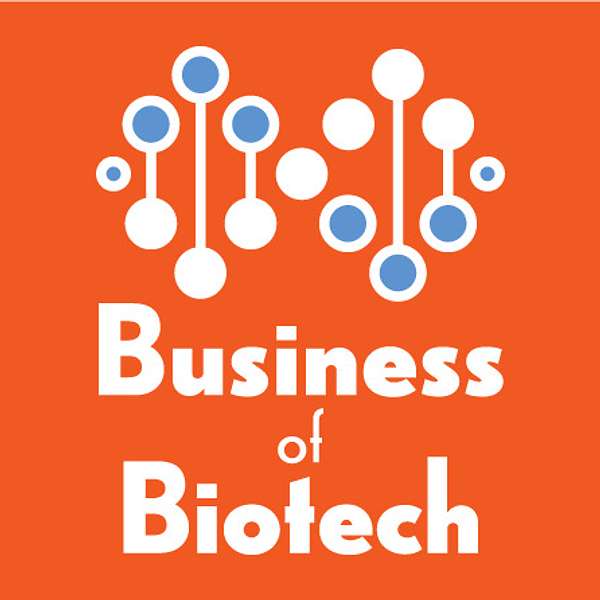
Business Of Biotech
The Business of Biotech is the pod dedicated to leaders of emerging biopharma firms. SUBSCRIBE to our new newsletter at www.bioprocessonline.com/bob. We bring you insight into organizational, finance and funding, HR, clinical, manufacturing, regulatory, and commercial challenges you’ll face as you navigate your company from an idea to success in the clinic and beyond. Each episode features guest commentary and best practices from accomplished founders and biopharma industry luminaries. The Business of Biotech is produced by Life Science Connect.
Business Of Biotech
High-Throughput Discovery With Bioptic's Andrey Dobry
We love to hear from our listeners. Send us a message.
Andrey Doronichev, known to many simply as Dobry, is perhaps best known for taking YouTube mobile. You heard that right. If it weren't for him and the teams he led, YouTube might still be a desktop experience. He went on to lead product strategy at Google before launching the startup Optic in 2022. What's that got to do with biotech, you ask? Well, it's Optic where he feels his greatest contributions are to come, and his sights are set squarely on disrupting the way we discover therapeutic targets. On this episode of the Business of Biotech, Dobry, Life Science Leader Chief Editor Ben Comer, and I explore the intersection of AI and biotech, discuss Bioptic's beginnings and its approach to leveraging advanced screening technologies for drug discovery, and address Bioptic's biggest challenges -- data access and industry skepticism.
Access this and hundreds of episodes of the Business of Biotech videocast under the Business of Biotech tab at lifescienceleader.com.
Subscribe to our monthly Business of Biotech newsletter.
Get in touch with guest and topic suggestions: ben.comer@lifescienceleader.com
Find Ben Comer on LinkedIn: https://www.linkedin.com/in/bencomer/
If it weren't for today's guest, you wouldn't have YouTube on your phone. At least, it's fair to say, you wouldn't have had YouTube on your phone way back in the late 2000s. Andrei Doronichev or Andrei Dobry as he's called made a big splash in Silicon Valley back then when he led the team that took YouTube mobile. We have him to thank for what's at once become what I call the most valuable tool in the shed when you're trying to troubleshoot a malfunctioning washing machine and a guilty pleasure of mindless YouTube shorts. Andre stayed on with YouTube after the Google acquisition, as head of YouTube Mobile and director of product management. He left the company in 2021 to try his hand at entrepreneurship, leveraging AI and image recognition technologies as his business mediums.
Matt Pillar:So what's a Silicon Valley superstar? Have to do with the business of biotech, you might ask. Superstar have to do with the business of biotech, you might ask. Well, andre's latest venture, a startup called Bioptic, sits squarely at the intersection of AI and biotech, and if he has his way, it'll create opportunity for biotech builders like you. Now I know what you might be thinking. Just about everyone launching a new biotech these days claims to sit in the middle of that intersection. Well, if it were anyone other than Andre Dobry, I'd probably pause before inviting them on the show, but this guy's really done some stuff, and now he wants to do some of that stuff in biotech, so the juice in this episode should be worth the squeeze. I've also asked my friend and colleague, ben Comer, chief editor over at Life Science Leader, to hang out with Andre and I today, so I'm excited about that and I suspect you'll be continuing to hear and see more from Ben in the coming weeks.
Ben Comer:Andre Thanks.
Matt Pillar:Matt, it's great to have you guys on the show, great to be together with you guys.
Andrey Doronichev:Thanks, matt. After your introduction I really want to meet this Andre guy Sounds like an awesome person.
Matt Pillar:Well, you're going to meet him today, and so is our audience, and I want to start with this departure from Google. Before you even started getting into the biotech space, what was it that kind of prompted you to move away from Google and start founding these tech startups? Why'd you leave and what have you been doing since?
Andrey Doronichev:Sure, well, first of all, before I joined Google, I actually had a couple of startups. So I joined Google in 2007 after selling my previous company, and it was a crazy amazing time to be in tech. And it was a crazy amazing time to be in tech and I was extremely lucky to not only be in the forefront of back then emerging mobile internet, but also I had a chance to launch something that lasted, that made sense and that makes sense even now as the most useful tool in the shed as you referred to it. That lasted, that made sense and that makes sense even now is the most useful tool in the shed as you referred to it. So this app that we started with just a couple of engineers and then we negotiated, agreed with Google to fund it and we launched first the Nokia version and then the Android version and then eventually, like I had the privilege to negotiate with Phil Schiller of Apple, to launch in the App Store. That one little app went from zero users to a billion users and was an incredible once in a lifetime opportunity to be part of that team. But, that said, I had an amazing run for 13 years and, very frankly, I just felt like we kind of solved a lot of big problems in tech and at some point I found myself sort of not being bored but feeling like we are sort of inventing new problems to solve, because the actual user pains for information access were like solved at large, at least in the domain I. I applied my knowledge to um and, uh, yeah, it was a good time to move on and see what else is there, um, what other things I can contribute, uh, to world.
Andrey Doronichev:And I spent some time reflecting and, you know, just taking a break after I left Google. And then, you know, it became clear to me that, first of all, it is really hard not to do something, even if you can, and second is that there was happening another revolution in tech that was machine learning, and that something big was about to happen. And then I really wanted to be at the forefront of this new wave of technology, this new wave of technology. So I started a company like a little private research AI research team with not very clear mandate. You know what this is was well, we probably going to build on our strength Like my co-founder co-founder said years of research experience in high throughput information retrieval systems, something similar to YouTube's screening technology that screens every video when you upload it and makes a decision whether it's a video about cats or it's some violence or some inappropriate material or whatever.
Andrey Doronichev:It's essentially a system that watches, quote, unquote every video billions of videos uploaded, and then very quickly decides what to do with it. So that's the content ID screening platform, as YouTube called it, and so we had expertise in those kind of high throughput screening technologies and we thought that there might be other verticals where this technology might be applicable. So we assembled a team. We raised some capital from outstanding investors Kleiner Perkins was our lead and Ray Locke participated in the round and many others. So with this team, we started just exploring different hypotheses. And here we are. Turns out that screening technology using AI is extremely useful for bio. So that's how you know. Eventually we turned into tech by a company.
Ben Comer:Andre, obviously a number of big pharmaceutical companies have used high throughput screening as a development tool, using a compound library. Was that the real connection that you found to biotech? I mean you could have gone into a number of different fields. You chose bio. Was there some other reason that you were interested in bio and human health, or was high throughput screening and the kind of adaptability between those industries a key aspect of it?
Andrey Doronichev:The honest answer and I guess I'll be giving honest answers today. The honest answer is both Like one, my personal interest, and you know I'm over 40 now. When I was 30, I really cared about entertainment and content and games and videos and it was super fun and I lived by this mantra that Silicon Valley lived by, which was move fast and break things, and it felt very natural. And for those 13 years at Google, it felt very natural to me. And then, like by the time I left Google, I realized that I'm passionate about other things now and one thing I found myself really interested in was health. And you know how I said we ran out of pains to solve in tech industry. Well, in health, there are literal pains for billions of people out there that need solutions. So, as a reflection of things that became more important to me at this stage of my life, this entrance into bio field feels natural. But also the honest answer is there was a bit of luck and what happened was as we built our screening technology and we were using it for all sorts of use cases, from assessing digital art and detecting fraudulent artworks to helping banks and financial institutions to detect AI-generated driving licenses when they're being uploaded during know-your-customer KYC flow. All sorts of things can be solved if you have AI that can literally screen billions of items in minutes or hours.
Andrey Doronichev:We've been approaching different industries and testing our solution in different places, and so I went to a conference to TED in Vancouver a couple of years ago, and as I was participating and chatting with people across different industries and academia who participated in the conference, I met this professor, dr Art Cherkasov, who is a professor of drug discovery, spent his life working on small molecules and peptides.
Andrey Doronichev:He is currently a tenured professor at the UBC, and so, as we were chatting, I described what we have and, yes, this is where this connection to high throughput screening came to be, because he explained to me that, hey, actually like we're having really hard times now that molecular libraries became so big like orders of magnitude bigger, he told me. I think he told me, hey, back when we were screening this program he was telling me about, we had to screen 2 million compounds and at this point there are libraries of like 40 billion compounds that are synthesizable. I have no idea how to screen that, what we're going to do, but probably there's a lot of great molecules out there, so we need new technologies that would be way faster. That would speed up discovery of active compounds among those libraries, and so that was how we decided to try applying our tech for this problem.
Matt Pillar:That's. It strikes me as I mean, yeah, there's luck involved, like you said, but it also strikes me as courageous. I mean it takes courage to pivot from one industry into any industry. I think it takes a special kind of courage to pivot from an industry into biotech. I mean that's a pretty significant pivot. What were you? I mean this is sort of a fuzzy question, andre, but what were you feeling at the time? Was it just straight confidence in the technology and its application in this setting? Or was there any trepidation at all, like hey, you know bio, that's science. Science is a little bit different from bits and bytes and feeds and speeds.
Andrey Doronichev:Yeah, to this day I'm questioning my life choices. No, it was really scary and it still is. I mean, the complexity of biology is like nothing I've ever seen in my life and I thought I worked in so much complexity in big tech. So I have enormous respect to scientists and life science industry, enormous respect to scientists and life science industry, and I do believe that there's a reason why so many industries already have been disrupted by tech. But I think tech bio we're just scratching the surface. This is just the beginning, because it's so complex that we needed so much pre-existing technology to make it possible to even approach this field. That said, to answer your question, I have a story.
Andrey Doronichev:When I came back from this TED, I was intrigued by the idea of applying our tech for drug discovery and it sounded really cool. The professor, dr Cherkasovov, was working on a prostate cancer drug. I was like oh, we can, you know we can use AI to treat cancer. Isn't it great? Like it sounds fantastic, right, but truth is, I didn't really take this opportunity seriously at first. I thought it was way too far and way too hard.
Andrey Doronichev:And at some point mid last year I was presenting to one of our investors, our partner at Greylock and there were three parts of the presentation, three hypotheses we went after with our technology and first two made a lot of sense and I mentioned them to you like a lot of sense and I mentioned them to you like the art detection use case and we had like revenue and customers. Then actually it was looking pretty good. We had the second test running with banks and AI generated content detection, which was also showing a lot of promise and made sense, especially given that that was the time when generative AI just started booming. And then the third I just threw in there and I was like hey, well, and we also have this third idea. That sounds completely crazy, but we could probably maybe use AI to find molecules and help those academia people and researchers in the industry to find drugs, like drugs for cancer. And I presented those three things and my partner at Greylock, david Thacker, he looked at me and he went you know what I think you should do.
Andrey Doronichev:The third one, the crazy one, the AI drugs one. I was like wait, what, how so? And he said, look, the first two make a lot of sense and you have good data and good founder market fit. Founder problem fit makes a lot of sense. But the last one is the one that gets your eyes to light up, and, if I know anything about early stage startups, it takes a lot of passion from the founder to actually make it to the end, because companies normally run out of stamina way before they run out of money and therefore I think this is the actual problem you care about. You should work on this one.
Andrey Doronichev:Honestly, to me it was shocking, and even after this conversation I did not immediately react, but it grew on me At some point. We had a very honest conversation with my co-founders, but it grew on me At some point. We had a very honest conversation with my co-founders and we just realized that, of all the things we could be solving with this technology that we have and generally we could be solving in the upcoming 5, 10 years of our lives, that there's nothing more important. Nothing more interesting than helping outstanding researchers out there to get to their goals faster and save lives and help people to deal with disease. So that's how we ended up here.
Matt Pillar:I mean that is fascinating. I guess the first question I have for you is what was the reception beyond that initial conversation with Greylock? You know your eyes are lit up, this is something we should pursue and then over the course of time, you know, probably in short order you expose your intent to the biotech industry, the community. You start talking to other investors, you start talking to potential. You know customers use cases. I'm curious about what the reception looked like.
Andrey Doronichev:It varied. Obviously, I got a lot of people were skeptical, right Like I. Just, in general, I found that life sciences industry is hard to penetrate for someone who doesn't have PhD in biology and doesn't have all the credentials in academia or industry. Like who am I? Some tech guy deciding to build some technology that would apparently replace scientists which, by the way, I never said I was going to do scientists which, by the way, I never said I was going to do. What I was saying was like hey, before that we were plumbers for ad industry and now we're going to be building tools for scientists. But anyway, the the initial reception varied.
Andrey Doronichev:However, there was a very important thing for us as entrepreneurs, as startup guys. You know you either see some traction, you see that you are showing some results. There was a very important thing for us as entrepreneurs, as startup guys. You know you either see some traction, you see that you are showing some results early on, or you're not. And that's what matters. And what we did. We started writing tests and you know we first, you know, indexed NMN's 40 billion molecular library and small molecule library and just started testing in silica our existing screening technology against a couple of targets that our scientific advisor, professor Chirkasov, had data for and we just started running all sorts of tests trying to see if the results even make sense. And once we started seeing that there is some light and actually looks like there might be some good results, it became sort of irrelevant whether or not people on the outside believe that we can do it. Truth is, we have technology, we know how to develop it, we have a process for do it. Truth is, we have technology, we know how to develop it, we have a process for building it and we have brilliant scientists who are willing to collaborate with us testing this technology and seeing if it can be applied to their programs. That is enough to start.
Andrey Doronichev:So around this time last year I did two things I sent an investor update to the rest of our investors saying, hey, we're pivoting to buy and here's why, and I explained the whole thesis. And two, I discovered your podcast and started listening to it to understand how to approach the industry, like really I was completely a complete newcomer. And of course, another thing I have to say we are privileged to have the investors we have, especially like Kleiner Perkins, who were the original investors in Genentech at round A, and Brooke Byers, specifically of KPCB, who was kind enough to kind of give me a lot of initial introduction to this industry. He brought me to Laguna CEO Forum where I met a bunch of great people who gave me feedback straight away and that was very honest, very kind of guards down conversations where people were receptive to the fact that I came from a different industry and I, like, didn't quite know what I was doing. And those conversations really steepened the curve and, you know, allowed us to quite quickly start orienting ourselves, at least initially.
Andrey Doronichev:And you know we managed to sign our first deals partnership deals in Q1 of next year, so Q1 this year, basically right. So just a quarter after like official pivot, which I think was super fast. And just generally, you know the speed at which we've been moving since we started Bioptik Bioptik is the name of our bio AI platform the speed at which we've been progressing is quite fascinating. Like, at this point we have 13 therapeutic programs across several partnerships in academia and industry and two in-house programs, and we are progressing rapidly. So I think you know, when you have some results it makes it easier to believe in yourself.
Ben Comer:Yeah, andre, I think it's. It says a lot about you and the company that you were able to attract some of these kind of blue chip investors, particularly given the fact that there's so much noise about AI out there. You know every new biotech has some sort of AI play. Was there a key attribute or a key piece of technology, or you know a key aspect of yourself and you know your founding team that you think helped to attract some, attract some of those investors and and a short period of time.
Andrey Doronichev:Yeah, I do have an outstanding team and I think it was the right place in the right time and we should not forget about this too. Like we raised our first round, we raised $11 million seed from Kleiner Greylock and others in the very beginning of 2022, which was the very peak of the venture industry, just before it became really sour and the tech investment climate, especially in AI space, at that time looked way better than looked way better than bio investment climate, which I didn't realize until after we pivoted into bio that actually the investment was actually quite quiet at that time, but in tech things were really peaking. So we got lucky with timing of our first round. But also, answering your question, of course, the biggest reason was the team. Like I had my tech pedigree, which of course, helps a lot when you approach investors, and also my co-founders are incredible AI researchers with like 50 plus prior deep neural nets that they trained from different companies, from Intel and Nivea to Pepsi and Nestle and whatnot. So, like they had, my co-founders had incredible experience before that working at the deepest level of ML AI research.
Andrey Doronichev:And so you know, yes, of course, every company right now is AI enabled. It's obvious Like every company at the moment is using internet right, like it wasn't the case back in the 90s. It's one thing that you're using some neural nets or you're training some models for a specific target, that you're working on specific therapeutic programs and you help yourself to progress this program forward with some computational tools. There's a whole different game of trying to build foundational technology that would be target agnostic, that would generalize across many targets and potentially many modalities and that would really change the game for potentially hundreds or thousands of biotechs out there, if it's successful. And that is what we are up to. We're trying to build something that would be an enabling technology for many, many programs across different targets, different indications and potentially different modalities too.
Ben Comer:In addition to founding Bioptic, you also co-founded Mesto in 2020, which is a community of Russian speaking entrepreneurs, and I'm curious about what the war Russia and Ukraine has meant for your business, if it's impeded you in any way, or what you might say about that conflict and its impact.
Andrey Doronichev:I know there is always some war and people suffering around the world, but for me personally, as someone who was born in Soviet Union I immigrated out of Russia 20 years ago ago. For me, this particular war has been the most heartbreaking, horrible geopolitical event in history. The vastness of this crime, the invasion of Ukraine, the destruction of its cities and the conquest of their territories all those things that Russia started in 2022, I cannot describe by any word by Well, first of all, we work with some partners who are physically in Ukraine right now, which means that you have to work around some horrific facts that sometimes there might be a bomb destroying their house or there could be electricity outages for hours and days. So that is obviously a huge impact. Apart from that, part of my team are Ukrainians, and some of my key people on the leadership team who are now, who are now in US, are actually came here as refugees from Ukraine. So that is also a significant factor, of course.
Andrey Doronichev:And besides, as I mentioned before the recording, I think the fact that this war began in February 2022 really forced me, as a founder, to start this company and raise capital way earlier than I otherwise would have, because you know we were playing with hypotheses, we're contemplating different product ideas.
Andrey Doronichev:Again, it became obvious to me that we're going to have to be in position to help a lot of people who would need help. Some outstanding tech talent AI talent from Ukraine and from Russia too, who had to flee from Putin's regime, will need to find their new way of living in different places. They would end up and they would need jobs, and you know we could help. So, in a way, you know, we have some outstanding ai people working for us, um, which otherwise we would have. It would be hard for us to hire because they were all employed in some tech giants or, like Kiev based startups, but because of the war, they needed a job and we were there to help. So, yes, it's a very complex topic for me, but the simple answer to your question of course this war had ginormous impact on us, both from business standpoint, from the team standpoint and from the personal standpoint as well.
Matt Pillar:And that, my friends, is a difficult question to pivot away from, tough to find a segue away from that one, so I'm just going to make it rough. You said something earlier, andre, that piqued my interest. You were talking about your partnerships, the number of partnerships that you have going, and then you mentioned a couple of in-house programs. Yes, what can you share about that, if anything?
Andrey Doronichev:I will try to share as much as possible. I cannot share some of the names because of the deals that we have that we require like marketing approval. But in broad strokes we have 13 small molecule programs we're working on at the moment, all preclinical, all are highly novel chemical matter, sometimes non-targets. Some of the targets are completely new. Roughly half of them are academia partnerships. So these are the first ones we started with and that was pretty much the way forward. I think of those investments from our side as sort of a way to test our technology. But also it's a marketing expense, right. So we in those partnerships we do all the computational drug discovery work, but we also synthesize chemical matter and then a partner would run their assays and test the compounds that we synthesize, and so obviously it costs money. But I think it's worth it because in case we are not ready to publish those results yet, but the results look really promising. So once those papers are out that's going to be a great way for us to market the platform.
Andrey Doronichev:But closer to the July last year we signed our first industry partnership and I think this is one interesting thing that differentiates BioOptic is that I do think we found an interesting angle into the industry which is different from what I've seen before, and that is partnering with venture creation funds or bio-investors to help them de-risk their asset companies like single asset companies that they invest in, or help them boost progress on those programs they invest in.
Andrey Doronichev:And as far as I can see, we are pretty unique in this approach. We developed a bunch of tools that are sort are investor-facing and helping them first identify opportunities, assess those opportunities and then actually help the research team within the program to move faster using our tools. And so that first deal that we signed in July, the first molecule out of 10 has been announced already. It's called Vinder Therapeutics and it's a $17.5 million partnership in milestones and royalties that are paid to us and it's an oncology drug, precision oncology. I don't think we disclosed or they disclosed the target, so I will not go there. So yes, it's academia, it's a number of industry partnerships and we try in different business models, like we have a joint venture with one of the oncology therapeutics companies. We do have this sort of milestone revenue more like traditional research collaboration deal and we do have two internal targets one in obesity and one in Parkinson's.
Matt Pillar:So yeah, those are internal projects.
Andrey Doronichev:Yes, those are fully owned.
Matt Pillar:Yes, yeah, those two, yeah, that's interesting. Yes, yeah, that's interesting. When you look at the, you know I picture this as sort of a winnowing down right. Like you start Optic, you've got a bunch of potential applications and multiple verticals. And then you get excited about the biotech opportunity and there's an immediate use case, an immediate application that you get to try out and test out. When you survey the biotech landscape and you open your mind up to applications beyond those that you tested early, that you played with early, what do you see? What do you see that you're compelled to perhaps pursue in terms of applications for your technology?
Andrey Doronichev:That's a great question Because, yeah, you know, I'm being very honest here we started with the use case that just fell in our lap because we had technology and we had someone who wanted to use this technology for HitID. And then, like later on, a few months in, you realize that actually that's how most of the first wave of AI drug discovery companies back in 2014 started, like AtomWise and Silica. Everyone started with sort of, okay, small molecules, hitid, we're going to do some sort of virtual screening technology that will be fast and whatnot, and so you know, our tech was. You know, it seems to be working really well. The truth is, it's a pretty crowded space and it is one of many use cases that need to be solved, and now that you know we've been at it for a while, we're, of course, expanding our offering to many more new ideas, like, as I mentioned, for example, by opportunity assessment and tools for investors is kind of a direct result of this better understanding of the landscape. All that said, I think it's pretty clear that we have not, as an industry, we have not solved any of those use cases completely, entirely. It's not like you have a fully generalizable model right now that enables zero-shot, de novo small molecule discovery, right, Like no or de novo zero-shot for anything actually, not just small molecules. So there's tons of opportunity at every stage. Um, but you know, as I, as someone mentioned to me, you know, if you want to speed up drug discovery and you're focusing on pre-clinical tools, it's like you're speeding up the trip from san francisco to london by speeding up uber to the airport and, yeah, like it makes sense, of course, like vast majority of time and money goes into clinical trials.
Andrey Doronichev:And you know, I do believe that eventually we will get to the point where we'll be ready to create like digital twins for at least animal models, and then like maybe at some point, like far, far far in the future for humans, and so that would be a whole different world. We're nowhere near that yet, but I think for now, realistically, there is a lot of opportunity. The initial hit idea sure, great, there's our technology, there's other technologies out there. Something has been happening and I think right now, with new neural net architectures like generative AI, wave of AI, let's call it this way we have a chance to make it way better. But I do believe that there's a lot of opportunity in multi-parameter optimization, so like key-to-lead and lead-opt phases. That's also preclinical but it's not solved and there's a lot of stuff that will be happening there and I see a lot of teams doing great job at this stages.
Andrey Doronichev:When it comes to clinical stages, I haven't touched it enough to understand it deeply, you know. But it's also clear to me that there's a bunch of low hanging fruits, like, for example, I've been chatting with someone about medical consent procedure when you're trying to enroll the patient in the clinical trials and you know it is really it's not solved. The user experience of this procedure is like really, really complex right now and I think you know it is really it's not solved. The user experience of this procedure is like really, really complex right now and I think you know it's a completely different type of AI technology. But if you imagine like a better designed AI based consent procedure, that could really change, make a difference, because you know I'm used to you know, at Google you're used to making a small change and creating like millions of dollars of value using this little change because the overall volume of customers is so big. So in clinical trials, that's where you know, 1% change, 1% increase in efficiency can actually mean tens of millions of dollars of value saved or in other words generated for the customer. So that is a huge opportunity too.
Andrey Doronichev:We are remaining in preclinical for now but, as I mentioned before, we're expanding beyond into opportunity analysis and detecting new investment opportunities for investors, which is an interesting different play Overall the industry. I think you know the next big frontier, like we're going to figure out chemistry with AI. I think that's pretty clear at this point. But figuring out biology is a whole different game. Right, when you design the molecule that theoretically, even like with wet lab validation, like in our cases, we're very proud that you know the molecules we generate in silica then work well in the wet lab and you run your assays and yes, it binds and yes, like the potency is there and you know you're excited. This is not a drug. And this molecule put in a living cell and the whole complexity of it and then in the living tissue and then in the living organ and the living body, is a whole different level of complexity which we're not even approaching yet, and I think you know.
Andrey Doronichev:The next frontier for the AI drug discovery industry, of course, is modeling biology and which is why I see a lot of my you know, some of the smartest people I met at Google actually getting there, like Dave Burke, who used to be the engineering manager for my YouTube mobile team back in the day and then he was the leader at the Android team, recently announced that he is joining ARK Institute to work on cell models.
Andrey Doronichev:Recently announced that he is joining ARK Institute to work on cell models. You know Steph, who used to be a product manager, stefan Grieken, who used to be the product manager, like fellow product manager at Google, and his partner, daniel Daicho, who used to be an engineer manager on YouTube Mobile as well. They co-founded I'm not sure if Daniel is co-founder, but they both are at Cradle and they are doing protein generation with AI. So my point is more and more people, who I consider some of the smartest people I have ever worked with, are moving into this field of solving biology with AI, which is, I think, the next level of complexity after chemistry.
Ben Comer:Yeah, that's fascinating. I'm curious about, you know, I guess, first your content recognition technology. Maybe you could give us a kind of overview of that and then also talk about the data inputs that you're using. You know, particularly with respect to chemistry, and now biology Is there. You know what kinds of biological data you know is coming in. You know how much is it accessible. You know, are there types of data that you want that you can't have? I'll stop asking questions right now and let you respond.
Andrey Doronichev:Sure, well, starting from the beginning, our screening tech the content ID as we called it was essentially a super high performance similarity search engine. So, in simple terms, it would enable you to index billions of items. Whatever it could be like for us it's all vectors, right, but it is a vector representation of some information. It could be an image, it could be video, it could be an image, it could be video, it could be a molecule. And then the way our platform was engineered initially was designed for speed, so that you can perform very quick searches and find similar vectors to the one that you are looking at. And, depending on what you encode with this vector, you can apply this technology for different content types. So initially, as I said, we did it for videos or for images.
Andrey Doronichev:But when it comes to molecules, this is where the term similarity search might be confusing, because in chemistry or in drug discovery, when people say similarity search, they mostly mean structural similarity, so you look for a molecule that is structurally similar to the one you started with. In our cases, we meant something completely different. So what we encoded as those vectors were activity profiles of molecules. So we took data initially from binding DB, which essentially, in simple terms would be a small molecule in the protein, a pocket of a protein that it binds to, and so that together would be an activity profile for us. We would encode it and then we would index this data and that would enable us to find, for like in some other larger data set, like, for example, n-means synthesizable compounds database. We could find a molecule, a small molecule, that would be completely different structurally from the ones that we've ever seen in our regional training data set but that, according to the neural net, would have similar activity profile to something that you care about, like, for example, think of a patent busting use case. Like you know, when we started Bioptik, everyone was crazy about GLP-1s, right, so great.
Andrey Doronichev:You log into Bioptik and you take a known uh legend for um or glp1, you put it into the system and and ask it to search um, let's say, a billion uh compound uh data set that either you brought as a library or you know something from one of our partners, like e-molecules or NMN, and what Bioptik would be able to do is to find, let's say, 100 molecules that would be less than 0.4 tanymota similarity with the original molecule that you put in. However, they would have similar activity to the same receptor, and that was the original use case for our very first technology, when we just straight up adopted our first technology to bio. Of course, since then we've built a lot of new tools, like our new model that we're really proud of and we're really excited about.
Andrey Doronichev:A structure-based model that we designed works using completely different principles at this point, which means that you can just input any protein sequence for your target. It could be a completely novel target. It could be a target with no 3D structure result actually, and the model would design a small molecule, or it would find a small molecule among the library that you would provide that would most likely bind to this pocket, and it will detect the pocket itself pocket automatically. It will understand the 3D structure automatically. So you skip the whole alpha fold or crystal structure step altogether and it just spits out a number of molecules that you would take to the wet lab. So now it's a whole different game.
Ben Comer:Yeah, and you mentioned several different databases that you're using to access that molecular data. Are you building a kind of proprietary database of bioptics own at this point? Are you still always trying to bring in increasingly more data from more sources? What's your view on that?
Andrey Doronichev:Well, data is a huge problem for the industry in general. Right, let's start there. What I didn't realize getting into bio was, you know, our thesis was we should be building AI for industries where there's a lot of data, a lot of capital and not enough native AI talent. That's how we started and the bio seemed like a perfect industry Clearly a lot of data, clearly a lot of money and, probably like vast majority of people who graduate with data science machine learning degrees, they do not go to Pfizer or Merck, they go to OpenAI at Google and Google. So it felt like, okay, this is an interesting opportunity for us to work on.
Andrey Doronichev:However, when I looked into this, we realized that the vast majority of data is proprietary and, in fact, it's not normalized. It's very often very noisy, there's loads of problems and I think even the bigger problem for someone like us who is trying to build a foundational target agnostic technology is that the kind of data collection and generation technologies that you need if you're working on a specific program or specific target or even a specific modality, that your approach to data generation is different from when you're trying to build something horizontal, and when you're trying to build something horizontal, you need a very diverse data set you need, like you cannot afford paying per data point as much as you would pay if you were working on a very narrow domain, around certain targets, for example. There's a whole new set of challenges in terms of data generation if you're trying to do it across a vast chemical space, vast domain. So it's not a solved problem. Like all of the binding DB that we initially used to train our first model, I think it was like less than 10 million data points. It's like I don't remember exact number, but it's something like around, let's say, 10 million data points. The number of tokens that was used to train GPT that was the first like truly generalizable language model was around a trillion tokens, right. So it's what? Six, five, six orders of magnitude, right? The difference we are nowhere near a number of data points needed, and that's just in this one particular idea of like binding right, but there's so many more parameters that you need to optimize. So it's just the industry itself does not have data.
Andrey Doronichev:Even if we could pull all the data from all the proprietary sources, at the moment I'm convinced we don't have enough data to actually build something truly generalizable and so like right now there's a new crop of tech biocompanies that are trying to solve this from different angles.
Andrey Doronichev:You know Dell libraries is interesting, technology phage displays interesting stuff, and I think there's a reason why we're seeing so much progress in protein design right now is because sequence-based data in many cases can be synthetically generated. Like you can actually pre-train models with a lot of synthetic data, which is a fantastic opportunity. It doesn't solve all of your problems, but our new structure-based model is actually using pre-trained parts. It's using parts of ESM, which was trained on billions of possible protein sequences. And so this combination of real-world technologies for measuring real wet experiments, but in a way that is economically viable, and combining it with either pure synthetic data or better scraped and better normalized and better classified public data from more niche sources like negative data from failed programs, for example, which is I think for now is still untapped opportunity Like combining all those sources together is probably like what a lot of companies like ours are going to be doing over the next decade or so.
Matt Pillar:Do you see anything afoot that might contribute to the solution to that dearth of available data, and when I say that I mean, like you know, any collaborative efforts, any industry-wide efforts to unlock some of this proprietary data or, to your point, some of see any influences that might not create a glut but at least reverse the dearth of data that we're looking at right now?
Andrey Doronichev:My honest answer is I don't know. I mean, there's been initiatives that look promising, like Melody. It sounded like an interesting way of federated learning, but I don't know if it like what was the impact of it? Um, for now, my personal bet is that it's still going to be a massive investment in data generation pipelines through automation, through new, specifically designed assets that would make it possible to do it, like at the massive scale, sequence-based data and maybe like building like a Google translate quote unquote, for, like you know, peptides to small molecules, like that kind of stuff, those ideas. There's like plenty of teams with ideas of how to build a data generation pipeline that would enable you to generate like billions or even trillions of new data points, and so my bet for now is that one of those efforts will more likely to succeed and actually start like generating new massive data sets across different targets, rather than existing data sets will somehow be reconciled and, you know, unlocked and whatnot.
Andrey Doronichev:There's too much headwind in the way this industry is set up. You know, data is gold and, just like the way Big Pharma is set up is such that it's not really designed for sharing data. At this point, like even for us, we are working with many customers right, but we cannot really use specific data points from customer programs to train our foundational model because there's an IP issue. Basically, all those data points that we can use to refine a model for a specific program and actually make it more efficient for this target or this particular molecule is not really contributing to the other programs, so we have to generate our own data. That's actually like clean IP.
Matt Pillar:Yeah, how are you doing on time, andre?
Andrey Doronichev:I'm okay, Like Joe Rogan, Joe Rogan me as much as you want, but yeah, I totally get it that at some point you'll have you'll have to cut it in pieces. So please stop when you feel like we should.
Matt Pillar:No, that's the direction we're going. We're going to turn this one into a three-hour long episode.
Matt Pillar:Good, I want to pivot a little bit to the landscape of AI players of several different flavors in biotech right now and get a sense for where bioptic fits and what you see playing out in this sort of nascent. I say nascent but I mean it's maturing rapidly, I think, at least in terms of volume and applications. You've worked your entire career in some pretty competitive high-tech environments, so I'm curious about your perspective on this. We've had on this show a number of notable and well-funded biotechs that are building on AI backbones. I think of companies like Recursion you know Chris Gibson's been on the show before which just acquired Accenture. I mean, that's a powerhouse there. Ben referenced, when we were chatting in advance of the episode, hong Kong-based in silico medicine. Another one Just a couple weeks ago Vic Bajaj from Foresight Labs was on the show and they just launched a new company called Zara, a biopharmaceutical development company called Zara, with a billion dollars in funding behind it AI-driven.
Matt Pillar:So there's that, you know. You look at like in the mid-tier, like flagship pioneering every company that they spin out these days has got an AI backbone and then further downstream, looking at like sort of the service, like application service providers, if you will. You've got a ton of these little, you know I shouldn't say little, but smaller sort of cottage industry of companies like ProFluent and Evolve. Their founders have been on the show as well and they're looking to provide AI as a service to biotechs. As it stands right now and then, maybe forward-looking a few years down the road, where do you kind of see bioptic fitting on this landscape?
Andrey Doronichev:Sure.
Andrey Doronichev:Well, first let's talk about my view on the industry in general. I think every pharma in 10 years from now will be AI-first pharma. To me it's pretty clear right At the end of the day, drug discovery and drug development is about working with vast amounts of data, making decisions and progressing and designing something using this data. We've been doing it originally, like science was done, hypothesis-driven way, where a human with a big brain comes up with some great idea and then collect some data to refine this idea, builds a very local, very specific model to sort of help steer in a close proximity to the original hypothesis and then either prove it or disprove it and move on. And we move from that world to the world where we do data-first research, where we're mining ginormous data sets and then nowadays we're creating some super smart foundational models that can generalize foundational concepts from those data sets and then start spitting out decisions that were not even human-driven. It's a whole different world we're approaching, and so to me it's pretty clear that human's ability, human brain's ability, to deal with vast amounts of data over the next 10 years will not evolve dramatically. However, our AI technologies will improve orders of magnitudes. So to me it's pretty obvious that, just like every retail became an e-commerce over the last 15 years, 20 years, similarly every pharma will be an AI company going forward.
Andrey Doronichev:Now, what does it take to be a great AI company? Well, I think to build AI advantage, you need three things. Very roughly, you need talent advantage, so you need to have people who are really good. Very roughly, you need talent advantage, so you need to have people who are really good at it. You need data advantage and you need compute advantage. And eventually, compute advantage means right now. For now, it means do you have access to chips? Eventually, it will mean do you have access to enough energy?
Andrey Doronichev:So, at this large scale, if you think of this from the top level, if the transition from today to the future of pharma is similar to the transition from old school retailers to today's retail, which is all e-commerce enabled, and you think of the number of enabling technologies and different kinds of companies that make this transition possible, you realize that there's a lot of opportunity In the next 10 years. There's that there's a lot of opportunity In the next 10 years. There's going to be a lot of different shapes and forms of companies that contribute to this eventual outcome of an AI-enabled pharma and sure, like in e-commerce, you had a fully vertically integrated players like Amazon, who started in the very beginning of this transition and were very different from retailers back then, and at that time brick and mortar retailers were laughing at them and then eventually, these huge vertical integrated companies became the massive dominating force, and so I think we're going to see that in pharma. So we probably will find some new big pharma that will be an AI-first company, and maybe Zyra is one of those companies, maybe Isomorphic will be one of those companies, I don't know. But again, drawing this parallel with retail, you also see that there's a lot of horizontal technology companies that enable different parts of the stack. You have tons of smaller shops run by e-commerce platforms like Shopify or using Shopify technology. You have the ginormous company Stripe that is essentially enabling all the payments industry and so on and so forth. There's so much in the stack to unpack and each one of those layers is a ginormous opportunity.
Andrey Doronichev:So back to your question. You know, yes, there's a lot of AI companies out there and they have all sorts of different shapes and forms when you double click into them. Some of them are sorts of different shapes and forms when you double click into them. Some of them are, you know, effectively a biotech that is using AI technology to progress, a number of assets they care about. Some of them are trying to be a horizontal platform, but let's, you know, let's say those were like the first wave of AI companies back in 2010, 2014. They just didn't have enough enabling technology back then, nor they had enough data or compute to actually deliver on that promise, and so we saw a lot of very disappointing cases where companies just did not deliver and did not get even with one asset into clinic over the last 10 years, and we'll know different examples here.
Andrey Doronichev:I think there's a new wave of companies right now that are relying on the modern AI technology that transform architecture, like the new generation of chips that enable way bigger sort of data processing capability, and that also they do their own high throughput data generation, and so I think this is like the current state of the art and there's a lot of excitement there, and some of them are trying to be like sort of a horizontal platform enablers, and some of them are also just using this technology for their own internal therapeutic programs, and so from now on, like in the future, as I mentioned. I do think that there's going to be more and more new like next generation AI companies that would actually try to solve biology and model cells and maybe even bigger tissues and organs using AI technology. We're nowhere near that yet. I don't know when it's going to happen, but there's also going to be a huge next wave, a big new crop of companies that will enable that at some point. All that to say is yes, there's a bunch of companies out there. I do think there's a lot of opportunity.
Andrey Doronichev:And then the question is how do you combine your talent advantage, your data advantage and your compute advantage vectors to create a meaningful proposition? In our case, we do have talent advantage, for sure. Just you know, we, as I mentioned in the beginning, we were lucky to be able to hire people who otherwise would have worked at Google or Intel, or our head of sciences from Atomwise, and we have people from Insilica, from Neuralink, from Apple, working in bioptic. It's a very strong team. We are working on our data advantage strategy. At the moment we have our own strategy that we're pursuing, but I'm not ready to disclose it yet. We do not have any compute advantage beyond comparing to Google or NVIDIA. We don't have our own data centers. We don't have our own chips, so that is the part where at some point, we'll have to figure something out. But we're relying on existing platforms and we're not limited by it for now and as a go-to-market strategy. I think we found a very interesting angle that actually enabled us to sign those first deals, like first commercial deals and you know, I'm really excited about what's to come next year, because this approach of trying to get to market through people who enable investments in single asset companies or smaller biotechs it's a meaningful strategy that so far worked well for us, bringing our talent advantage and our technology to their investments, to de-risk them and help discover new opportunities. All together seem to be working for us and we'll see where we end.
Andrey Doronichev:And one last thing I wanted to mention is software company versus more classic asset ownership. There's always going to be a debate, debate there. You know, when we started we as, as you can imagine, like I, come from consumer applications world of course we wanted to build pure software, play, um. But just, you know, learning more about this industry, we realized that the way to capture value is to have a share in the actual assets and selling results rather than selling services or software. So at this point we are. You know, the way we try to monetize is through equity in the resulting assets and I know that there are, like some very successful companies, who go pure software away. But we picked this path and for now it's working well for us.
Ben Comer:Andre, I wanted to ask a question about your internal pipeline. I think you mentioned a preclinical program with GLP-1s and in Parkinson's as well. I was curious how you chose those. If it had more to do with the data that you have access to or you know you're looking at therapeutic areas, obviously GLP-1s obesity and diabetes are hugely lucrative. Parkinson's huge unmet, need you know. What would you say about you know the choosing, I guess, of those programs and then if you have any sense of how far away you are from clinical trials of how far away you are from clinical trials.
Andrey Doronichev:Yeah, To clarify, we do not have GLP-1 program. We are working on TNIC target and we believe that there is. Our hypothesis is that it is a target that can be relevant in obesity indication and yes, Everybody these days is just associ, you know associating obesity with glp1.
Andrey Doronichev:Yeah easy yeah, yes, but, but there are other targets too. Um, um, yes, and and and answering your question, uh, how was how we pick those targets? Very honestly, just following business opportunity. So those are not completely novel targets right, the existing molecules.
Andrey Doronichev:For either of the targets we're going after, we saw an opportunity. We needed something to start testing our technology independently, without any delays from partners and whatnot. So we picked a couple of reasonably hot targets where we knew that that was different enough to test our technology in different ways and we knew that in case those go well, we can have a valuable asset. So we're pursuing them. We're going to solve whatever. We're going to predict clinical trials, one outcomes, toxicity outcomes at the early stages using our technology, and whatnot, like all sorts of things like that. Altogether, they are platform technologies that are target agnostic and that potentially make resulting biotech more capital efficient. And yes, matt, like you did mention that, maybe one day we found ourselves being a biotech. You know, someone told me a fellow tech bio founder told me once well, when tech bio fails, it becomes a biotech.
Matt Pillar:Is that how it works?
Andrey Doronichev:If you cannot sell your platform, you cannot like make your platform valuable enough to scale it the way you initially planning, like what we are thinking of right now then, yes, you end up just valuing your company at the value of the assets you've developed so far. And that's why there was a lot of disappointment with first wave of AI companies who really, really tried super hard to build a scalable platform and did not invest enough into moving their internal assets to clinical stage. And then, by the time it became clear that the platform itself is not monetizing, they didn't have any clinical stage assets and at this point, okay, they're not valuable anymore. So those huge drops in valuations that we've seen are driven by that. So we're trying to be somewhere we're balancing between the two because, yes, we are tech, bio, but we do try to own equity in all the programs that we're working on. And that's how we, you know, we try to seize the capture, capture the value that we're creating.
Ben Comer:Andre, I apologize if I missed this earlier, but did you and, to the point that you were just making, do you have an approximate timeline of when you might go into the clinic with your internal assets?
Matt Pillar:These are the forward-looking statements we ask for when there's no public relations or investor relations representative on the call with you. Yes.
Andrey Doronichev:And as much as I would like to boast about our uber-optimistic timeline and roadmap yes, of course we have a roadmap and we are pushing really hard to get there I do not think that it would be appropriate to make those statements in this goal. However, what I do want to say is that, at least with those two assets that we own, you know our goal to hopefully set a record timeline from starting the program to IND enabling studies phase, but you know that is yet remained to be seen. We are. We had a killer year. It's been super fast, and you know every advisor and every investors we have told me that it's unprecedentedly fast. You know how we got from where we started to where we are now. However, you know it doesn't mean that it's going to go as fast over the next year. I'm very optimistic, though.
Matt Pillar:Are there any specific plans that you can share with us for for 25? I mean, we're going to this episode is going to drop right around the turn of the year, so give us a, give us kind of a glimpse or a forward-looking hope or forecast right for for 25.
Andrey Doronichev:Sure, I can talk about the platform plans and that's the part I'm mostly excited about. Um, as I mentioned earlier, we have developed our new structure-based uh model. That is extremely promising, like think of it. It would enable things that, uh, you know state-of-the-art models like alpha fold 3 uh uh enable at the moment. But it's ridiculously simplified architecture, which means it's way more nimble. It's easier to deal with.
Andrey Doronichev:The way we describe it internally is, if you're following space industry and you might have seen this transition from Raptor 1 engine to Raptor 3 engine in SpaceX, where they dramatically simplified the design of the engine, removed a lot of tubes and components that were covering this original design, and that makes the whole thing way more manageable.
Andrey Doronichev:So this is how we think about Bioptic 1, the structure-based model that we're working on. So the plan for the next year would be, once we have all the data ready, to publish a paper on it and actually present it to the world in a more public way. But meanwhile we're using it for a number of programs to actually progress those molecules internally. So that's one structure and and publishing the paper around it, and two on the business side, you know, fingers crossed. I mentioned one deal that we signed last year, but we have a couple more exciting deals that are in the works right now, so I hope we'll be able to announce all of them sometimes, you know, earlier next year, hopefully, and actually like at this point, I could be less cryptic about our partners and people we're working with and the kind of deals we sign.
Matt Pillar:Yeah, what about internal growth, like company growth? Are you looking to make any strategic hires? Grow personnel or talent there?
Andrey Doronichev:Yes, we like we've tried, like we have an outstanding team at the moment and you know I try to move as fast as possible with a small number of humans employed as possible. Uh, that said, um, we are. You know, I feel like we're reaching the limit. So we're going to be hiring both on the science side on like a life science side of things, and also on the computational science, so AI researchers. We're probably going to start growing our business team too, but that I'm not sure about. And I think, like sometime next year, we're going to be raising the next round. We're getting ready to.
Matt Pillar:Yeah, very cool. Well, listen, we've been abusing your time, andre. I appreciate all the time that you've given us, but we do need to wrap things up. And you just brought up you were talking about the computational team and the biology team and I want to have you back on the show for a more, maybe a tighter, episode where I don't keep you for an hour and a half To talk about that, the marriage of these two things.
Matt Pillar:We've been talking about the marriage of computational minds and biology minds for a couple of years now, and when we first started talking about that, it was sort of like well, you know, you put them in the same room and you make them talk to one another. But now, as this concept has matured, you know, and you have companies like Bioptic that are actually bringing you know, onboarding this talent, I think it'd be fun to have a conversation about how we're consummating this marriage in real time. So if you're game for that, we're going to have you back on sometime down the road to talk about that and all the progress that we expect to see out of Bioptic in the coming year.
Andrey Doronichev:I'd be privileged to come again and talk about the marriage of tech and bio, because I think that's the most interesting thing that is happening in the industry at the moment. All the most interesting things that happen at intersections and the intersection of AI, which is essentially artificial evolution life, and bio, which is the actual evolution life. This intersection seems to be the most exciting thing there is.
Matt Pillar:Yeah, no doubt, and you're fun to talk to about it, so I appreciate it. Thanks for coming on, andre. Thanks, guys.
Ben Comer:Thanks a lot, Andre.
Matt Pillar:So that's Bioptic founder and CEO, andre Dobry. I'm Matt Piller.
Ben Comer:I'm Ben Comer.
Matt Pillar:And you just listened to the Business of Biotech. We're produced by Life Science Connect and we can be found on lifescienceleadercom and anywhere you listen and subscribe to podcasts, do that subscribe so you never miss an episode. Be sure to leave us some feedback on how we're doing, or a guest or topic suggestion, or just say hi, we drop every Monday. Keep it dialed and, as always, thanks for listening you.