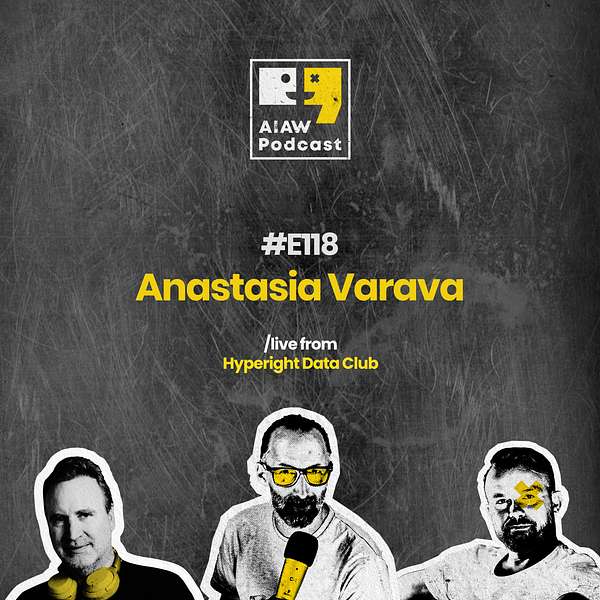
AIAW Podcast
AIAW Podcast
E118 - AI Innovation in Banking - Anastasia Varava
Join us in this week's AIAW Podcast - Episode 118, for an enlightening journey into AI innovation in banking with Anastasia Varava, Research Lead at SEBx and Data Scientist at SEB. Anastasia, armed with her extensive background in computer science and AI research from KTH, Stockholm, will guide us through a range of intriguing topics. We'll explore her journey and key contributions in her PhD research, delve into the innovations of the virtual assistant at SEBx, and examine the current and future landscapes of AI research in banking. Anastasia will also share insights on the WARA NLP Track, discuss the crucial role of AI in society, and give us a roundup of the latest AI news. The episode will further touch on the impact of the open-source movement in AI development and culminate with a thought-provoking discussion on the potential societal impacts of AGI, from dystopian concerns to utopian possibilities. Don't miss this comprehensive conversation at the intersection of finance and artificial intelligence, where Anastasia Varava sheds light on the exciting future of AI in banking.
Follow us on youtube: https://www.youtube.com/@aiawpodcast
Yes, where you have Yanle Kun, right he's a good, brilliant example. Right he's working with Mehta and at the same time, he's a distinguished professor, and this goes on and on in MIT and Stanford and everywhere. And we don't have that sort of legacy.
Anders Arpteg:I've been also trying to do the same to drive research happening in industry in different ways in my past. So I'm very happy to hear that you're also interested in trying to drive that agenda a bit more. But perhaps you can come back to also your new affiliation, so to speak, because you mentioned you left KTH and moved into SCB, but you still were there during your free time, right?
Anastasia Varava:Yes, I was there as a ghost Ghost researcher.
Henrik Göthberg:It's a classic. Some people you love the academic setting and I kind of not want to leave. You know, it's the people who never graduate as well.
Anders Arpteg:Yeah, some people never leave the student environment. We have the Matthias from Linköping. He's like 50 plus and he's still in student.
Henrik Göthberg:He's doing good work as well, but he has but still okay.
Anders Arpteg:So you were still there at KTH doing like close to research work, but on your free time, basically.
Anastasia Varava:Exactly.
Anders Arpteg:But you recently was appointed some kind of more formal.
Anastasia Varava:Yes, so now I have like the paper that basically is signed by both KTH and SCB and says that, okay, I'm an affiliated faculty in this school.
Anders Arpteg:I work on these topics, yeah, so it's a formal setup and what basically do you do as what's the formal affiliated faculty Is?
Anastasia Varava:that the proper name for it. Exactly that's what it's called. I know the title says nothing.
Anders Arpteg:But it is in computer science at least, right? Yes, what's the area?
Anastasia Varava:Yes, so I'm affiliated with the School of Computer Science and Electrical Engineering. But yes, my area is computer science. That's where I did my PhD, that's where my whole education is.
Anders Arpteg:Right and you continue to work with robotics or the area.
Anastasia Varava:Yes, yes, exactly. So I'm not a hardware robotics person. I'm more interested into how we can use algorithms to make robot move and do things. So, now that I started, I would like us to look a little bit more into how both foundation models can be used for robotics, but also how embodied agents can be used to train better foundation models.
Anders Arpteg:Kind of explore this overall, very apt and interesting topic. We had a couple of people from the robotics side here before and a lot of, of course, software people as well. To have someone that combines both of them, I think, is very interesting, super interesting. So with that, we would love to formally welcome you here. Anastasia Varavan, was that correct? Yes, thank you. Wow, and you are a research lead at SEB-X, right? Yes, this is called. You had a PhD, as we spoke about before, from KTH in robotics, or you can define it yourselves later more properly, I think. And you also associate with Vara, like the WASP Research Arena? Yes, and it's the media language or the NLP field.
Anastasia Varava:So we are Vara from Eden Language. Yeah, and I am one of the members of the core team. Yeah, so I'm basically core-run and it was a few other great people.
Anders Arpteg:So many interesting topics here to cover, but before we go into all of them, perhaps you can give a quick background about yourself. Who is really Anastasia? Who? How is your? What is your background? What is your interests? How did you come to be who you are today?
Anastasia Varava:I would say I'm a computer scientist broadly, if I talk professionally. So I like to see myself as someone in between industry and research. So you can say I'm an applied researcher at this point.
Henrik Göthberg:But then, before you go on, I just want to add that maybe one of the themes that we can kind of circle around, I think, is this how do we bridge the gap between academia, applied research in Organizations and actually, how do we get an even stronger, higher productivity out of? This I think this is a logical overarching theme today.
Anders Arpteg:I mean like I think I know this is close to your heart and it's close to our heart as well. Let's make sure to get back to that question, surely? Yeah, it's very interesting.
Henrik Göthberg:But it's really this CBX, r&d and CBX, then KTH and all this this and what Vara is trying to get that done and everything. Yeah, so please back to the. You know who you are, but I think that was just appropriate.
Anastasia Varava:Yes, so I'm an applied computer scientist. To put it in one sentence Maybe if you like, if you like boxes.
Anders Arpteg:If I may just interrupt quickly, do you think we can do basic research in industry?
Anastasia Varava:You can and, for example, deep mind does that but that requires quite a lot of investment, a lot of risk appetite.
Anders Arpteg:And I'm sorry to jump in this topic but I'm not sure I'm trying to keep bounce on myself to not go there already. But cool, okay. Applied researcher working both in industry and academia, then. But before that, can you just describe a bit? You know what's your interest. How did you come into the person you are today?
Anastasia Varava:How did they become a computer scientist? I mean, I was a computer science nerd back at school. So I come from Ukraine originally and I went to specialized school for people interested in math and mathematics and things like that. And as I was growing up in high school, I did more and more competitive programming at the time. So I re-elect algorithms and things like that.
Anastasia Varava:And then I was very natural to just go and study computer science. And then somewhere maybe in the second year of university, I got really interested in pure math. So I kind of navigated a little bit more to applied math, which was in between.
Anders Arpteg:But I think that's an interesting point, because some people come more from the programming side and then become perhaps more into math. But you came from the math side and then became yeah, I mean.
Anastasia Varava:I like to solve problems. I've never been interested in programming for the sake of developing product, so algorithmic computer science was originally my thing when I was a kid and then kind of added math interest to that, so that's.
Henrik Göthberg:And then you started in. When did you move to KTH?
Anastasia Varava:Yes, so I moved to KTH in 2014, I think, to do my PhD.
Henrik Göthberg:Yes, when you got started, you started your PhD. That's when you started it.
Anastasia Varava:First of October 2014. In KTH yes.
Henrik Göthberg:And is this? When you came to Stockholm, that was my first day in Stockholm. Yeah, first time.
Anastasia Varava:I remember it as if it were yesterday.
Anders Arpteg:Fantastic. Was that a positive or negative?
Anastasia Varava:experience. I was super happy. I was very young, I was full of hopes. No, I really like all of the topic of my PhD, and that was kind of the only reason why I moved to Sweden in the first place.
Anders Arpteg:Yeah, awesome. Okay, so you did your PhD, and that was with Danie from the starch in your lab as well, yeah. Yeah, yeah. And if you were to just try to describe briefly, perhaps, your PhD, what was it really about?
Anastasia Varava:It was about designing algorithms for robotics, more precisely for path planning and manipulation from the kind of geometrical perspective. So in reality I did quite a bit of computational geometry, a bit of computational topology To enable safe robotic manipulation and navigation among obstacles.
Henrik Göthberg:And so, in layman's terms, who can't follow this? Yes To tricky language. It's about making the robots navigate in a complex space.
Anastasia Varava:Yes, exactly, exactly. In cluttered environments, where you have Not killing humans, exactly, and have improvable guarantees that that's not going to happen, yeah, so basically finding the safe boundaries.
Henrik Göthberg:you know that this is yeah.
Anastasia Varava:Yes. Stuff like this Exactly.
Henrik Göthberg:And the core technique is geometric.
Anastasia Varava:Yes, what is that? Are you so? Computational geometry, so you work. This is going to be technical, please. He loves it. Yeah, I know, I'm not here to stop being technical, it should be technical.
Henrik Göthberg:I'm here to Can I see if I get it. That's all. Don't go technical, that's super fun yeah.
Anastasia Varava:So Okay, I will still try to. Topically A bit higher. Forget about me. So I work quite a lot with simple shell complexes, like geometric representations of simple shell complexes, to essentially model obstacles and generally spaces in which robotic systems are and kind of trying to understand how much objects can move with respect to obstacles, how much the robot can interact with them, whether, if you, for example, grasp an object, whether it's going to fall on the floor or not, whether a robot can move from one point to another. Like again with a guarantee.
Anders Arpteg:So just to give an example, basically you have some kind of environment. Yes, if you set up mathematically, essentially you just represent.
Anastasia Varava:Like you represent this room as you kind of model it, as a 3D model.
Anders Arpteg:You can see it like this and then you have a set of obstacles inside that room. Yes, and that's a part of your environment, right?
Anastasia Varava:All of those things are a part of your environment, and then you want to find some potential path.
Anders Arpteg:Yes, so it's kind of a doorstep.
Anastasia Varava:So, it's kind of a dual problem in a sense, because sometimes you want to restrict the mobility of the object if you want to manipulate it and in that sense you want to prove that that happened, so it cannot kind of escape from you, it cannot fall. But you can also consider the dual problem which is the robot wants to move from point A to point B and you kind of want to understand whether it's possible or not, so whether there is a path.
Anders Arpteg:So both these objectives can be solved with, say, method.
Anastasia Varava:Yes, exactly, exactly, exactly.
Anders Arpteg:And if we put it in simple terms, if you have a Tesla car that wants to move from one point in New York to another, for one you want to see can you stop it from actually going to the White House, or whatever?
Anastasia Varava:Yeah, you can see it that way. It becomes more. It's not maybe so much a geometric problem at this point, but yes, absolutely.
Anders Arpteg:But at the same time, if you wanted to go to the White House, the same method you used to avoid it can also be used to potentially prove that it can.
Henrik Göthberg:Yeah, but you made a good comment. When is the geometric approach most useful?
Anastasia Varava:So, when you interact with physical objects, what kind of restricts your mobility is physical obstacles. That's where geometry comes in. You can take it to a different level of abstraction and say that, okay, I don't have a geometric obstacle here, but, for example, if I'm a car and this region is forbidden for me, then I can also represent that as a virtual obstacle. So to say so, you can add those things as well. But yeah, originally we were talking about physical obstacles.
Henrik Göthberg:And where has the application of geometrical approaches? Are there any use cases, for example, where this is quite used today in different? I mean like? I have in my hand the lawnmower. Yeah, Robotic you know when is the geometrical, when has that sort of been mostly put in production, if I ask you like that, that you know of.
Anastasia Varava:Oh, in production, I don't know, there was like research, research.
Henrik Göthberg:This is research. This is okay, good.
Anders Arpteg:But you mentioned also of course you're working in more theoretical land here and not the practical world, at least in the beginning of your thesis what would you say? The usefulness, or besides the nice city, of having a mathematically provable solution to it? Can you elaborate a bit more? You know what can having a provable guarantee be useful for?
Anastasia Varava:So if you work with safety critical applications, I think it's fundamental that you can actually prove something, to kind of have the certificate that, okay, nothing can go wrong here. I think that's the main advantage of that.
Anders Arpteg:So even I guess you know, in real life, when you have, very like for one, partially observable environments and also dynamic environment where things change all the time, you can never have 100% observability.
Anastasia Varava:Yes, you can have some lower balance on how much you can move. Yeah, and because you cannot. So if you're working with really complex environments and perhaps your perception system is not perfect and perhaps things can also move without you being able to perfectly track them, but you can still have some sort of estimate for where for sure you will have obstacles and that kind of gives you an estimate, so that estimate can be conservative. If you want to again deal with safety, so you want to show that okay, if you're doing I don't know, automated surgery, like robotic assisted surgery, and you want to kind of really prove that something cannot possibly go wrong, or if you do a step-as-a-toddler's drive, for example, right, so you can kind of have a way to model this problem in a more conservative way and you show that this area for sure is going to be an obstacle.
Anders Arpteg:I think that's a clear case for it and being able to at least have some lower bound, as you say to ensure that at least we can say it won't be worse than this, so to speak, in some sense is a really interesting point.
Henrik Göthberg:But did you finish your PhD before you started SCB?
Anastasia Varava:Yes, and I actually worked as a postdoc for a couple years.
Henrik Göthberg:How was that?
Anastasia Varava:That was very different. I expected it to be exactly the same until day one when I started and suddenly I had like six or eight PhD students took a supervisor. So, then, the big difference is now you focus on your own research and now you're supervising seven different projects Exactly, and those students were rather junior as well, so they needed a lot of support. So that was not easy and all the context switching, but that was really good.
Henrik Göthberg:So the context switching of course is massive with six or seven people and then all of a sudden you're pedagogical.
Anastasia Varava:All these topics come really up front and a sense of responsibility.
Anders Arpteg:Okay, but at some point then you went, I guess, from the postdoc into SCB. In some way Can you just speak a bit more? How did that introduction to SCB come about?
Anastasia Varava:I don't think I would think of applying to a bank necessarily if I didn't know Salaf and Sin which we had on the. It was so fun, so we knew this.
Henrik Göthberg:Chris is an awesome person, of course, sal is an awesome person and Sal has been on this podcast, and if you haven't listened to that podcast, go and listen to Salafrancia's podcast. So how did you know? Salah is actually the real question.
Anastasia Varava:I actually knew her from the lab.
Henrik Göthberg:And how did? Well, she was also a ghost in the lab.
Anastasia Varava:Yes, you can say so, because she was supervising an industrial PhD student in our lab. So I knew that she was cool, I knew that she has a background in math and I just yeah, I just emailed her and asked do you have open? And turns out she did. Okay, so that was how it started.
Anders Arpteg:What was your thinking then? Because you still, you know, love academia in some sense. What made you still take the jump to SCB?
Anastasia Varava:Yes. So actually in my postdoc I did more applied stuff like more with machine learning, and then at some point I was thinking, okay, so now I spent quite some years in pure research. Now I want to actually see what kind of real applications are out there. And since I was never a hardware robotics person, I kind of didn't have this idea that I necessarily need to work with physical robots. So I was very open. I mean, I'm a computer scientist, right, so I was very open to learning about new application areas. And finance is an interesting one and it's also wouldn't have so many people with computer science background in finance. So it's a pretty interesting area to be in because there's quite a lot of unexplored stuff.
Henrik Göthberg:Sorry, is this 2018-19? When you started?
Anastasia Varava:Three years ago.
Henrik Göthberg:Oh, three years ago.
Anastasia Varava:Yeah, so 2021, I think.
Anders Arpteg:Yeah, and I also made a jump, you know, from academia, reluctantly, I must say I didn't want to leave either and I stayed after my PhD for a number of years and very, very reluctantly left to industry that found you know values in an industry that I enjoyed more. And I also saw some problem in academia. But I would like to hear your thoughts before I cloud your judgment, so to speak. If you were to say some perhaps negative aspects of academia, what would that be?
Anastasia Varava:I would say at the beginning of your academic career, if you really kind of go for it and optimize for traditional values. You have an academia, you need to travel quite a lot, so you need to do like postdoc or several postdocs abroad and then you start applying for faculty positions and that can also be all over the world, and that becomes difficult with your family and your life in general.
Henrik Göthberg:If you really want to do an academic career as a professor, it's quite.
Anastasia Varava:Exactly, and it's kind of easier, like, for example, when I moved here to do PhD. That was very easy because I was 22 at the time, so I didn't have any preference on which country to go to, as long as it was interesting. But then when you get older it kind of gets harder. So that to me that's the biggest problem, I think.
Anders Arpteg:Yeah, what do you think about the whole funding workload that you have to do in academia? Did you see that as a problem? Or perhaps you have enough funding anyway? Through.
Anastasia Varava:In Danny's lab. That has never been a problem. We had the opposite problem.
Henrik Gothberg:There's a couple of labs in the world that can say that.
Anders Arpteg:Yes.
Henrik Göthberg:A couple in the world.
Anders Arpteg:I think for most academia's out there they don't have that luxury.
Anastasia Varava:Yes.
Anders Arpteg:I'm well aware of that. What about paper writing, then, or writing papers?
Anastasia Varava:I love to write papers.
Anders Arpteg:Yeah, did you Some people go into the minimal public, publicly unit that you can write? Sorry for the horrible phrasing here there is this kind of sometimes pursuit of maximizing the number of papers you can write with a minimum amount of content in it, to be able to be accepted at some conference.
Anastasia Varava:Yeah, that's actually another problem, which is maybe like a problem of KPIs that you have in academia. If you need to maximize your age index by whatever means necessary, I think that's wrong.
Henrik Göthberg:But did you experience that, or maybe you? I left early enough.
Anastasia Varava:You left early enough, so I was left.
Henrik Göthberg:I think so. Something has happened here.
Anastasia Varava:It feels like no, it's more like I left early enough in my career, like I wasn't actually a faculty right. So I mean I did a postdoc for a couple years and then I was helping course-improvisant students but I never kind of did a proper faculty career. So I mean I was aware of those problems and I knew that if ever to stay in academia, I would have to deal with that. But that kind of wasn't my primary concern, because as a PhD student that's not exactly what you need to optimize for Is it a solvable problem or do you need to rethink?
Anastasia Varava:I think we need to rethink the system. Yes, absolutely.
Henrik Göthberg:And do you have any? If I'm not listening, do you have any fundamental principle ideas on how to solve it?
Anastasia Varava:First of all, I think we need to treat different, not just areas of science but maybe even sub areas of, for example, computer science differently, because if you work in, say, theoretical computer science, you will not possibly get as many citations as you can get in machine learning, for just publishing one benchmark that everyone cites, and that's just not fair. No, it's not fair.
Anastasia Varava:So we need to kind of treat those things very differently, and I think we need to when it comes to more applied computer science. I think we need to create a space that it's not necessarily even like where you work, is not necessarily even evaluated based on your publications, but more on the impact of the work you do. Right.
Henrik Göthberg:This is the topic, I think. Because I think R&D in a polite computer sense is going to be more and more relevant in the coming years, because the whole trend with the, with beating benchmarks, is really taking us down the rabbit hole of not doing novel research at all.
Anastasia Varava:Yeah.
Henrik Göthberg:Yes, it's stupid in terms to a fixed data set that everybody knows yes, and then that you know it's like it's a gamification. Exactly, is that research? I don't think it is research anymore.
Anastasia Varava:I don't think so either, and it's very frustrating to be super frustrated.
Anders Arpteg:Yeah, the hunt for point point one percentage on a bench Right and it's no real impact.
Henrik Göthberg:And if it include Andrew Eng and everybody else who says, like you know, real AI needs to work on the data and is lot of. When you have a fixed benchmark or a fixed data set, you sort of you're taking away the real world Exactly, ai problem Exactly From the whole research, exactly.
Anders Arpteg:But without. Perhaps you can elaborate a bit more. You know, I know, I know I can't really go into details about you, know how you perhaps use AI or your work to help, but perhaps you can speak in general terms a bit. What do you focus on? What kind of problems do you focus on in S, B, X?
Anastasia Varava:So in S B X? No, this I can actually cover a little bit. So we started the current iteration of S B X More or less when the hype was charged, GPT came out, and then everyone started calling us and asking oh, can I use to wait? Oh, the process.
Anders Arpteg:I was so frustrated in your organization.
Henrik Göthberg:So now I know what you do Sorry.
Anastasia Varava:But yes anyway so I was kind of really skeptical and annoyed by it for the first couple of weeks yes Weeks months for me.
Anders Arpteg:No, no, no. But then I realized.
Anastasia Varava:But then I realized that it's actually very good that people are interested because it can actually bring lots of value if you do it the right way, yeah, so what we ended up doing is kind of design and virtual assistance for our employees that would help them in daily work, yeah, but kind of, you know, through providing proper knowledge basis that are relevant for their work and doing fact checking and providing them references so they can actually click on the link and then you can go to the website and then you can verify the answer and things like that.
Anders Arpteg:Can we even go into our use and source models?
Anastasia Varava:We did a lot of benchmarking. Actually we compared, so we had several use cases and we compared different models in each of them. In some week unfortunately open A models for better.
Anders Arpteg:Unfortunately.
Anastasia Varava:Yes, I was rooting for open source. Yeah, but I still believe that open source is going to catch up.
Anders Arpteg:But you said open is better. I guess you mean like proprietary is better than open source, right?
Anastasia Varava:No Open source. I'm a strong proponent of open source.
Anders Arpteg:Yeah, but you said unfortunately.
Anastasia Varava:I said unfortunately, open A models.
Anders Arpteg:Why is it not? Fortunately because open A.
Anastasia Varava:Models are not open source.
Anders Arpteg:That is my confusion here. You said I thought you said open models.
Henrik Göthberg:Open A. Open A.
Anastasia Varava:Now I got it.
Henrik Göthberg:Sorry, I got it from the beginning, so I must be small.
Anders Arpteg:Open A Of course it's better than open source, but that's unfortunate, I agree.
Anastasia Varava:But I think this is going to change. I can't believe that.
Henrik Göthberg:I believe that Proprietary versus open source and large language models, because I think it's a huge topic, both technically interesting, but also societally interesting.
Anders Arpteg:But let's, yeah, but I add that as a topic for speaking, the pros and cons of open source and the Yanle Kun versus open A.
Henrik Göthberg:And I want to hear why you are proponent, why you wanted the open source to win.
Anders Arpteg:We can start there, but let's do it later Because I guess, also as we know, with the security demands it has on the data it is having, can't really send data over the internet to open AI or to yeah, yeah, yeah I mean the trick there is that you can still have open A models in a secure environment in the cloud that you don't interact with open A and directly, for example, use Azure, and then they also have. Those models there. Enterprise version of opening exactly and.
Anastasia Varava:Google Cloud provides the same, so like that problem per se is solvable, and then you can also deploy open source models in the cloud.
Anders Arpteg:I have to ask, though can you put bank data in Azure cloud?
Anastasia Varava:It depends on the sensitivity class. So we have a general strategy of moving to the cloud, but Google Cloud is kind of our primary.
Anders Arpteg:Google Cloud is the go to.
Anastasia Varava:Yes, but so, yes, we have kind of the long strategy of moving. I don't think we'll ever move all the data, but the majority at least, to the cloud. So that's the general vision. But then of course, I mean some data sets are like maybe not going to make it.
Anders Arpteg:Transactions of people's money, or you think that's going to be in the cloud as well.
Henrik Göthberg:But I think the core topic here is like I've worked with a button fall before and I think you say the way we framed it is that we framed it cloud first and then. So you basically you have to have a very structured approach for your use cases and data. And then you need to work very professionally with your data classification. Exactly.
Henrik Göthberg:Everything needs to be so that it puts a whole big, massive topic around computational data governance. In my opinion, like that, you cannot only do this theoretically on PowerPoint. You need to have your machine readable, metadata and all this stuff.
Anastasia Varava:Exactly, and we have a pretty big organization that is working just with that. At SCBX, we have the luxury that we do prototyping and exploration, so we don't have to work with sensitive data.
Henrik Göthberg:You're in the sandbox in this sense Exactly.
Anastasia Varava:Exactly so when we pick use cases, we make sure that we actually don't have to deal with sensitive data. Yeah, smart.
Anders Arpteg:Cool If we were to just elaborate a bit more about the very interesting topic of the virtual assistant. Do you call it that or do you have like a pet name for it?
Anastasia Varava:We have several, ok, but the virtual assistant of SCB, it sounds.
Anders Arpteg:I think so many companies are trying to do something similar. Yeah, a lot of struggling of course, but if you were to give some tips or some information about how do you go about building a virtual assistant for a company like SCB?
Anastasia Varava:So, first of all, it's not just like one generic virtual assistant. It's like several use cases that are technically similar. You have the same type of setup and architecture but are based on different knowledge sources and are catered towards different user groups. I would say the main thing is understand what your user actually wants. So work very closely with the business from day one and do kind of iterative prototyping. So set something up, expose it to the users, let them test it, collect their feedback and then improve. So those were the main takeaways and I'm really happy that we did it that way.
Anders Arpteg:I'm glad that you say that as a researcher as well. It's a perfect example of agile development. But also I guess there are technical limitations, that these kind of large language models are not easy to fine tune to the user data. And then you have different techniques. We had a podcast very recently speaking about RAG.
Anastasia Varava:Yes, that's exactly what we did as well, always. I think that's the good to approach. I'm not so sad.
Henrik Göthberg:We had the Jesper here from Volvo and we talked. The theme was from RAG to autonomous agents. You know where did we start the big hype in 2023.
Anders Arpteg:Everybody did RAG and where are we evolving to? But if you were to just give a very brief introduction for people that don't know what RAG is, how do you go about building a virtual system? That means you saw large language models and RAG.
Anastasia Varava:Yeah, so I can start with how to not do it.
Henrik Göthberg:That's a good one.
Anastasia Varava:So because that's kind of what maybe you may think about is, I'm just going to interact with language models directly and I'm just going to ask them questions about you know stuff that they weren't exposed to when training and kind of treat them as this large knowledge base. So that's a bad approach, especially if you ask it about something that is business specific, because they have never been exposed to that data so they will hallucinate.
Anders Arpteg:Then you can't put everything in the prompt either.
Anastasia Varava:No, exactly. So you need to kind of give it some context, right, and that context is essentially your data. So the easiest way to deal with it is if you don't even like, for example, want to find you on the model, right, so you want to just provide it your knowledge base, so it uses only that to kind of answer your questions. So you can essentially take your knowledge base, create embeddance and then do semantic search based on the question that you get from the user, compare it to the relevant documents that you provided, extract those that match closely and then kind of put them as a context to the language model and ask it to answer your question.
Henrik Göthberg:Because you did two things. Now you did the semantic search to take something down to a token space that you can fit into the prompt.
Anastasia Varava:Yes, exactly so. And then you provide this context that you extracted in this kind of retriever stage and you ask the model to answer the question. Given this context and as an else, Well summarized.
Anders Arpteg:Yeah, perfect. And you said knowledge base and I guess you know there's so many different terms here. But you have the language model. It's trained on a lot of external data world knowledge if you call it that, yeah, or do you have a preferred? Some people call it like world knowledge, or do you have a preferred name for what you call the thing that the whole large language model is trained on? Initially, you think world knowledge is a good term.
Anastasia Varava:I don't think so, because it's just a random data set that is very large, but it's still pretty random, random knowledge I actually don't like. Maybe it's an popular opinion, but I actually don't like to think about large language models as knowledge bases. I think what they really are good at and how they should be used is the language itself, but then kind of relying on them as a source of knowledge, unless it's like really common sense knowledge, I don't think that's a good thing, but it's an interesting angle here.
Henrik Göthberg:Are they actually good at knowledge or are they good at language?
Anastasia Varava:And you know, I don't think they're trained to be. They're not trained to be. No, they're not.
Henrik Göthberg:The objective of the large language model is to be good at language. The objective is not knowledge.
Anastasia Varava:Exactly.
Henrik Göthberg:And I think this is a misconception. We get a huge benefit. That actually has some knowledge, it seems, but the objective of the exercise is language, exactly.
Anders Arpteg:I have to disagree a small bit here.
Henrik Göthberg:Why Okay, okay.
Anders Arpteg:Let's do this. I think language is one thing. If you just know the grammar, that's certainly not enough. You need to have at least a common sense knowledge. Without that it would be impossible to write anything. So the common sense knowledge at least it has to know. It has tools to know a lot of other stuff that is potentially not related at all but could be so. It has a lot of irrelevant knowledge for sure. But if you really want to have the model used for something that is specific for your need, then you need to add something outside of that external knowledge. But to say that it just doesn't have any knowledge, I disagree with it. But let's be precise.
Henrik Göthberg:I think you need to be precise now, because what type of capability does it have? It has grammar capability, for sure. It has some sort of semantic capability or ontology type capability. So it can reason from the text that when you say the word so.
Henrik Göthberg:Reason very, very simplistically. Okay, Not reason, but it can understand from the context that when you use the word so, is it the so or is it something you see or you saw? So, okay, Is that knowledge or is that semantical understanding? I give it more than grammar. I give it more than grammar.
Anastasia Varava:But he's knowledge, then Exactly.
Anders Arpteg:I think it's a huge amount of knowledge that it actually does have, but it's not specific knowledge, it's certainly not all knowledge, but it is an insane amount of knowledge encoded in these large language models that, if you very briefly describe, you know, how can it understand if Paris refers to the city or the actor she is?
Henrik Göthberg:Yeah, yeah, the famous Paris Hilton, yes.
Anders Arpteg:Then of course it needs to understand the context, but I agree. You don't think I disagree to the concept of large language models not having knowledge. I think they have an insane amount of knowledge.
Anastasia Varava:They have it, but whether it's reliable or not, it's another question.
Henrik Göthberg:But let's reframe the question. This is an interesting one. Is the objective of the large language model built as an objective of knowledge, or is it the objective of being fluent?
Anders Arpteg:I think to predict the next word, which is trained on. It requires the ability to understand language and knowledge. It would be impossible for it to predict the next word without having a lot of knowledge. I would argue.
Henrik Göthberg:How would you say it? Because my point of view is that we get knowledge, or we get that, as a byproduct, but the coding objective of a transformer is language.
Anastasia Varava:Yes, I agree with you and I think knowledge is a byproduct. Like you predict the next word or the next token or whatever you want, just based on this distribution. Right, You're not actually optimizing for knowledge.
Henrik Göthberg:So you're optimizing for language and in order to do language really well, it has to have knowledge.
Anastasia Varava:Yes, You're optimizing for the sentence being statistically like being likely exactly.
Anders Arpteg:But the statistical argument. I mean you could argue the same for the human brain.
Henrik Göthberg:Yeah, you can Right.
Anders Arpteg:You can't say it's simply trained by using statistics. You could just as well argue that the natural neuron in the human brain is also just trained with statistics, potentially.
Henrik Göthberg:It's an interesting one, because I can fully see your point. But I think that you can technically also argue what is it optimized for?
Anastasia Varava:I disagree because, as a human, you can imagine things that have never happened.
Anders Arpteg:Right, the hallucination of large language models is amazing.
Anastasia Varava:No, but you can think of out of distribution kind of things.
Anders Arpteg:Okay. Do you think that large languages can extrapolate, or neural networks in general?
Anastasia Varava:Yes, to a degree, absolutely yes.
Anders Arpteg:But so can, you must, to a degree. Do you think they can extract a bit more than neural networks?
Anastasia Varava:I think so, I think so.
Anders Arpteg:I actually agree on that, but they can extrapolate to a surprising large extent. I would argue even for neural network or a large language model.
Henrik Göthberg:I agree with that too. I think it can do way more extrapolation than we understand even. I think that's sometimes when we are surprised today.
Anders Arpteg:Anyway, it's a very interesting philosophical question.
Henrik Göthberg:It's a philosophical question. Is it a problem, even If you flip it into and discuss this from a usefulness perspective?
Anastasia Varava:Is it extrapolation or is it interpolation?
Henrik Göthberg:That's a good one.
Anders Arpteg:It's a super interesting question. If you listen to some of the Machine Learning Street Talk podcasts they try to insist it's just interpolation. I would very much disagree with that. If you listen to Jan Le Koon, he of course says it's certainly not only interpolation. This is an interesting topic. If you leave in the course of dimensionality, as soon as you move up in dimensionality of any kind of vector or representation that you have what is really interpolation, then Everything becomes more or less an extrapolation. I would argue this becomes really philosophical. Now Fun. I think it's fun.
Anders Arpteg:It's certainly an interesting one. Have you listened to the Machine Learning Street Talk podcast at any point? No, I don't think so. He basically refers to a neural network as a hash table. A hash table saying if you want to predict what pixel it should be there, you find the two closest rows in a hash table and interpolate between them and then that's the prediction.
Anastasia Varava:That's a simplistic view.
Anders Arpteg:A very simplistic view. In my view as well, this is a topic because I want to come to the point.
Henrik Göthberg:Let's go philosophical. But I love when we go philosophical into the details. It's a little bit like you can go philosophical in math and in astronomy, but you can go and look at the big picture or you can look at the smallest quant picture, right? So let's be philosophical and granular.
Anders Arpteg:Should we?
Henrik Göthberg:go one minute more in this. No, let's keep it later, because I want to skip this now. I want to get back a little bit to before we start the pod. I asked you a little bit about how do you understand the research.
Anders Arpteg:Let's finish. The topic of virtual assistant, then we just spoke about you want to build a virtual assistant in SAP. You mentioned that. Of course we should build some kind of early prototype. You should get it quickly out there, test it with the users, get the feedback and then continue to iterate, which I think is an awesome tip and advice for anyone. Then you have some kind of large language model and you have some kind of rag. Can you go to any specifics? Can you say some of the models you have experimented with, like Mistral?
Anastasia Varava:or Lamatu or something. Yeah, mistral, actually haven't tested yet, but we compared models that come from OpenAir, obviously, models that come from Google and, if you, open source ones as well. But the whole point here is to kind of have this set up as flexible as possible so you can substitute models in the future.
Anders Arpteg:Of course it comes new once every week.
Henrik Göthberg:Exactly so. That's kind of the whole point to avoid this lock-in change. So you want an architecture where you can be a model, large language model, agnostic to some degree.
Anastasia Varava:Yes, yes, yes, exactly, and even for the retriever part, you can also be exactly.
Anders Arpteg:And the retriever. It's a lot of different, like vector databases or whatnot.
Anastasia Varava:You can put there yes and sometimes you can use it of the shelf, for example, and actually we started this very early, where of the shelf tools were not super nice and now they developed quite a lot. For example, the tools that come directly on Google Cloud Platform are now much better than they used to be six months ago.
Henrik Göthberg:And have you evolved in your thinking about how to do the rag or how sophisticated to do the whole rag setup or using knowledge graphs?
Anastasia Varava:Yes, exactly that's what I want to do next.
Henrik Göthberg:You haven't gone down the knowledge graph path. Not yet but I think this should be promising.
Anastasia Varava:Yes, absolutely, and I think for much more than just rag, much more than just answering questions.
Henrik Göthberg:Because now this is the point. Right, we started with rag and it's a hack. Yes, exactly. Can we agree it's a hack, but now, okay, we learn and it works. How do we make this more real? Right? And I think yes, perhaps some good ideas here, of course, where this is evolving, but the knowledge graph it still makes me to say that rag is a hack, but so is transform. It is a hack. You said it was a hack Sure.
Anders Arpteg:Okay, so can you elaborate how could a step moving from rags to more knowledge graph approach look like potentially?
Anastasia Varava:So you need to build a knowledge graph. That's kind of the fundamental part, and then you can kind of have this natural language interface on top of that if you want to query it or whatever you want to do. The cool thing that I think we can do now with knowledge graphs that we couldn't do before is that now it's going to be easier to build them Because again you can use language models to work with unstructured data, which again you could do before when you did all this like entity resolution kind of stuff.
Anders Arpteg:But if you take the knowledge graph and you think about the nodes and vertices in it, would the nodes be like the extension or intention? Sorry, let me be more clear. Would it be representations? That is, in clear text you can understand it. This is a person, for example. Or would it be more of a latent space node that represents?
Anastasia Varava:I think for us it's going to be real entities because it's not going to be single purpose. So you also want to kind of just treat it as a knowledge base where the user can also interact with it.
Anders Arpteg:Okay, cool, because yeah, so it's more okay, traditional sense of knowledge graph that Google have a knowledge graph. They have all the people and cities and things happening in the world. And then you're trying to populate basically this knowledge graph in different ways.
Henrik Göthberg:And, if I understand right, if you do the knowledge graph evolution, you will use it for the large language monsters part of the rag, but you can use it for other things as well.
Anastasia Varava:Yes, potentially for predictions, Of course, exactly.
Anders Arpteg:I'm very tempted to go into this topic again. It's good topic, no, but I had so many discussions about the knowledge graph approach versus not knowledge graph approach.
Henrik Göthberg:And you tried it early in Spotify and you didn't go.
Anders Arpteg:This is like 2010.
Henrik Göthberg:Exactly, but you didn't even go down that path at that time.
Anders Arpteg:But I, you know, for a very long time ago I gave course in like Sematic Web and Web Ontology and OLB and these kind of technologies that were supposed to be a complement to the normal web that we do have, and it was a machine version of the web, everything that I was supposed to be the knowledge graph for the machine, and it was very beautiful.
Anders Arpteg:It was very much using formal logic, this description logic kind of formalism, for representing the knowledge of the world in a like global kind of knowledge graph. It's super beautiful, super mathematically nice, I would say, but it never took off and it ended up being these kind of other solutions where you simply embedded small pockets of knowledge into HTML pages and never became this kind of interconnected knowledge graph machine readable for everyone. And if you, if anyone, refers to this kind of like formal knowledge graph Allah Sematic Web I'm sorry but I don't believe in it. If you believe in something else, like something that you build up which is more like I call it Sematic, but a strong word more less formal kind of knowledge graph than I do believe it.
Henrik Göthberg:But I think we have Stefan van Dien on the pod and he has. He was the evangelist at Neo4j before. And then I have Miko Klingval that is working with me at AirDocs and me. Miko uses a word where he thinks we need to figure out a way, that is schema. Second, we can't build schemas and everything. We need to be able to build schema as we go, and my understanding for knowledge graph value is more the latter. What you're saying, I don't think you can represent it. But to have a way where you, as you go, add the relations and you know people are working and then you okay, we do. The connection is more like, instead of having a tree structure, when you're trying to structure information, you have tags and you have Google search. So I think, I think very much. It's very much more organic. Understanding how the value of it is my view.
Anders Arpteg:I think what Neo4j is doing is actually very nice, because they are truly combining large language models with a graph that they are representing.
Henrik Göthberg:So that could be similar with your thinking. This is more organic.
Anastasia Varava:This is more schema, second ideas Exactly, and I think it should continuously evolve as well.
Henrik Göthberg:Yes, exactly, you cannot build it if you try to build a knowledge graph up front.
Anastasia Varava:No, no, no, no, no, come on, it will never work. And then you can exactly know and you probably have temporal aspect there- as well, and FASI association as well.
Henrik Göthberg:But I think the problem is when people are going all the way, almost fundamentalist, like the whole view.
Anders Arpteg:It's like a whole psych project. Do you remember or have you heard about the psych project? It's like something started in 1980s or something and it continued into the 90s and I think there are still some ramifications of it. But they were supposed to build like a common sense database, so everyone was adding like a car has four wheels and that is a rule added to the list and they try to add like a complete list of all the common sense knowledge.
Henrik Göthberg:And then there is a humanistic approach.
Anders Arpteg:in a probabilistic world I don't get it and it continued and it had like poor, sane amount of research money into it. And it never turned into anything useful, I would argue, but it's still not. I mean, it's basically abandoned to these days.
Henrik Göthberg:But we are killing it on the rabbit holes here, by the way, are we? Done with the virtual agents. Because I want to get back to the topic.
Anders Arpteg:Let's close this topic then. Virtual agents.
Henrik Göthberg:You see, we couldn't do it right. This is so fun.
Anders Arpteg:Okay, okay. Virtual assistant. Let's wrap it up here a bit. You have the kind of agile approach trying it out while you're working on developing it Flexible architecture exactly. You have the modular approach, trying easily to switch out models and rag approaches, potentially moving into some knowledge graph approach Perhaps we can just close off a bit or the use cases. Potentially you mentioned a bit with fact checking. I guess some of the session. Yeah, I mean, how would? You if you were to give some classical example use cases, what would it?
Anastasia Varava:be so I think one example is if you work, for example, on customer support. Yeah, so first of all, we do everything in GenAI for our employees because we want to have a human in the loop. It's kind of you know, a safe setup we don't want to put in front of the customer, at least not in the foreseeable future, but it's really about increasing employee efficiency. So it's kind of helping you to navigate through lots of text. For example, if you work on customer support and you have your internal instructions and you kind of want to quickly answer the question while being on the phone with the customer.
Anders Arpteg:Makes sense. I think that you know it's a very sensible approach. You know, still have the human in the loop. It's basically should be there and the AI should be there to empower the human not replace the human.
Anastasia Varava:That's what I say every day.
Anders Arpteg:Perfect, I love that. And I guess you know the number of use cases is. You know a large number of them. Fact checking, you know, is one of course.
Anastasia Varava:Fact checking is a part of RAG right. So it's like that's the because you I mean you kind of want to reduce hallucinations as much as possible, even though you have a human in the loop. You still want it to be useful. So, and references like from the retriever will also provide the actual references to the original documents and exposed them to the user as well.
Anders Arpteg:So if a customer calls into SEB and asks you know I can't get the bank ID to to confirm my loan or what not, then the the human that is sitting there answering speaking to that customer can double check with the assistant asking what could the problem be, and you get a list of answers Not in production yet. No, no, of course, what once it is hopefully.
Henrik Göthberg:But this is one of the clear cases, right, yeah, and we're working towards this. Yeah, I, we, I listened to. We had Tetra Pak on one of the conferences and of course, tetra Pak has huge machines like them when they build like manufacturing plants, for you know, and of course, then this is the same storyline. Like who the service technician? I have a problem with this machine and you know all this documentation about what part is this? You know it's the same. Right, that is such a logical problem to tackle. Should I, can I? Can I take one now, please? We had a very nice little discussion when you arrived. Anastasia and.
Henrik Göthberg:I think this is a good way to think a little bit about what is research in SEBX, and let me frame it a little bit more based on our conversation. It's like because SEBX, to some degree, is the exploratory approach and the incubator in relation to the mothership of all SEB. So so you gave me quite nice picture of like the stages, how you understand research and then how it moves into SEBX, and this I think this is this really frames what you do, and how you're thinking about this?
Anastasia Varava:Yeah, exactly. So we, internally at least, kind of think of it as a panel for, you know, potential topics that we might want to explore and that, by the way, applies not only to research topics, which I work with, but also to new business models, which some of my colleagues work with. And then we kind of have these different stages right. So stage number one is when you just monitor, so you see what's out there, what can potentially be interesting for us, what we can start experimenting with if that's promising enough.
Henrik Göthberg:So quantum computing, quantum computing is monitor. But is it an example like what? This is? Technology you monitor something, you keep a tab on it, but maybe not for production yet. Exactly, but it's still kind of good, but never Sorry. More positive than that. It's a good example, right, like it's a monitoring example, right.
Anastasia Varava:Yes, so for now it's monitoring for sure. Then if something is promising, you can move to the next stage where you have a hypothesis like, okay, I believe that this technology is going to solve this problem, and then you can start experimenting to kind of verify or disprove this hypothesis. And then if it's really applicable, like large language models, then you can move to the next stage where you actually build prototypes.
Anders Arpteg:So you know for me at least you know since I came from academia as well and I moved to industry, I tried to preserve the method of academia, so to speak, in industry and also building research teams in industrial companies, which was a big interest, or passion of mine at least. Sometimes it's a bit hard to argue for research because the gains of the value of a research team is much more long term than perhaps engineering teams are. But still, if the question then is, if a company is big enough, of course, then they can motivate having long term like research team in them. But I guess at some point it's hard to start motivating. You know, why shouldn't you optimize for the next quarterly report, kind of thing?
Anastasia Varava:I don't think you have that problem at your team.
Anastasia Varava:No, and we don't have that problem in the organization, thankfully. I mean, we don't do fundamental research. So first of all let's make things clear. So fundamental research is something you do at the university. What we do is R&D, so we don't. I mean we can publish if we want to and if you know, we kind of get some nice result as a byproduct of something we do, but we absolutely don't, you know, aim to publish. So we're not advancing research per se, we're applying research. So that's point number one. But I mean, if you only optimize for the next quarter, how are you supposed to have a long term strategy?
Anders Arpteg:Yeah, exactly that's my question, so I think it's. I am trying to promote and make people understand that actually having teams of look at the next, not quarter, not a year, but perhaps five years sometimes could be very useful as well.
Henrik Göthberg:But do you think there is? There's a cultural difference here. Let's say, a company like SAP, or a company like Scania that I've been working quite a bit with, or Wattenfall they have a. You know, when you have companies with a hundred year legacy, like they start behaving differently because they understand that, ok, there is another, there isn't. We need to have these different horizons in how we think about our business.
Anastasia Varava:You need to have forward looking leadership.
Henrik Göthberg:I'm not sure that is. I think it's quite unique when you you know you, you manage that because you win a high innovation, high tech company like Spotify or Paltorian. But I think there's there's there's some companies in the middle here. They don't have the long longevity, so they haven't seen it or experienced how close to bankruptcy.
Anders Arpteg:And I think so many companies are in this kind of financial like marginal kind of area where they have to save every dime they have more or less, and they are asking, oh, can we hire one more of these people? And they struggle not to and at the same time to say that you have a person that is not helping them fix the bugs that you have in the current system in production is really hard.
Anastasia Varava:Yeah, but I mean, if you're in that situation, it's very hard to think long term. Yes, no, it is, but I think.
Henrik Göthberg:Long term thinking is becoming harder and harder and my hypothesis this is a little bit like either. You have an understanding, deeply at the board level, of a company that has been around for 100 years.
Anastasia Varava:Because they've seen the macro cycles, they understand this, which we do, and we're grateful for that.
Henrik Göthberg:Or you have these real tech innovators. Who knows, we innovate or die, you know like. So they. Those two are sort of in the safe room here. I think that they understand the applicability of research. I think it's these companies in the middle that is really having a hard time with this.
Anders Arpteg:I think everyone should aim for having some kind of mental that the SAP has, that you need to have a balance you need. You can't just look short term. Of course you need. If something crash and you have to ever kind of you know catastrophe happening where they ransomware everything, then of course you go short term, you do everything you can to just fix the problem at hand. But other than that you need to have a balanced way where some work you do is long term.
Anastasia Varava:I think you should always have someone who is looking long term.
Anders Arpteg:Yeah, otherwise I would argue it's just a matter of time before you will be overrun.
Henrik Göthberg:But let's now add the curtsides, accelerating returns to this topic. So, when the innovation pace is increasing and when the productivity frontier is moving faster and faster and we get to a point that you actually need to unlearn and do it in, reinvent the way you do it, because otherwise the productivity gains is not even on the curve, yeah, I would argue, now that you know we are blurring what is R&D and what is business, what do you think? Yes, what is long term? I mean, what is really long term in this in the age of AI? 20 years is you know? Forget it, five years maybe?
Anastasia Varava:Yes, but then you also have quantum for example. Yeah, there is, there is, I would argue that's much less.
Henrik Göthberg:We can get into that. This is maybe even a topic for after after work.
Anders Arpteg:I'm sorry for you know, it's one of my favorite topics to speak about, but let's not go there right now.
Henrik Göthberg:But in a sense then there is some sort of ladder here. I was asking if you were using this sort of technical readiness level of zero to nine kind of model.
Henrik Gothberg:And I think you are. Yeah, we are, we just don't call it yeah.
Henrik Göthberg:I wasn't asking if there was a scientific sort of robust, but it's interesting how you understand that there is different parts to the task and objective of R&D.
Anders Arpteg:But if we were to try to phrase that one is the short term versus long term kind of objective. But I think there is another way to phrase also what the difference between R&D and research and let's even be more clear, science and engineering. Perhaps I have my favorite at least, like try to define what it is. Do you have any third way to say what's the objective of science and objective of engineering?
Anastasia Varava:It depends on where you are. Yeah, so if you're in a research lab, then or let's say, let's say like this if you're a pure mathematician, everything else is engineering to you.
Anders Arpteg:Yes, exactly Well said, I agree. And then, as you kind of move, more and more, sorry for all mathematicians out there, but you can say that as a mathematician yourself.
Anastasia Varava:I mean, I can relate to that, let's put it this way. But then, as you kind of moved more and more towards applications like, for example, where I am now, I still have very clear distinction between engineering, which is just literally implementing stuff where you know it's supposed to work but you need to build the system, and R&D, were you know it's not fundamental research. You're not solving the scientific problem, but you are kind of trying to figure out a way to solve an applied problem which, again, is not guaranteed that you're going to actually find a solution.
Anders Arpteg:So it's more answered. I took a bit of offense when you said engineering is just implementing stuff, because I actually do consider myself primarily an engineer.
Anastasia Varava:Yes, it's actually a lot of work. And then, like I don't think research can exist without engineering, at least in the R&D setup, and I love engineers.
Anders Arpteg:Yes, I do as well, and I remember some experiment podcast at some point and they spoke about the usefulness of science versus usefulness of engineering and I think you know neither can exist without the other.
Anastasia Varava:Yes.
Anders Arpteg:And let me try to give you my very brief definitions of them and see what you disagree with. But I think science and engineering is very overlapping, but they have a clear distinction in purpose. So science have the purpose of build knowledge and engineering have the purpose of building products or building value, I would argue. So that means that if you take Elon Musk, he claims he's the primary engineer. He wants to go to Mars. Everything that he used as a measuring stick, is how fast can we get to Mars?
Anders Arpteg:So that's like the value approach, that's like the engineering approach. That's saying, if I change the rocket engine in this way and I have to do science to know what it is, does it get me faster to Mars or not? That's the pure, like engineering mindset. I would say, how fast we can we get value of it. And if you take science, then you say I just want to understand how something works. I want to build knowledge that we haven't seen before, and I may need engineering to be able to do so, because I need to build a prototype, perhaps, that I can experiment with and see does it bring value or does it actually work or not? Sorry, I used the wrong word there. Does it actually work or not? And then everything you do you measure by build it actually build new knowledge or not? Yes. Would that make?
Anders Arpteg:sense to you in like definition of engineering versus science.
Anastasia Varava:Yes, exactly I think so. I think it gets a little bit more blurry when you move to an applied field.
Henrik Göthberg:Like in machine learning.
Anastasia Varava:it's like the discussion we had half an hour ago with benchmarks. That's where it gets blurry. Is this the same in robotics? Because again, you kind of want to solve problems, fundamentally in the sense that you want to be able to do tasks that were not visible before, but then you can also improve them right, so you can do them in a safer way, in a better way in general.
Henrik Göthberg:And then you have the other argument that you have the engineering mindset of Elon Musk. But you reach then to a topic where you really need to then go deep on. You know you get to a topic where we cannot solve it the old way. So we need to research our way through the engineering problem. So it's not like, oh, they are only, no, they're doing research, but it's actually the goal or the mindset. That is maybe one way in your definition.
Anders Arpteg:If you want to get to Mars. There is so many things you don't know.
Henrik Göthberg:You need to research that.
Anders Arpteg:And what do you do with things you don't know? You do science, you build knowledge, but you do the knowledge then in the for one, to build the knowledge and secondly, you use that knowledge to do the.
Anastasia Varava:I think, I think knowledge is the word of today's evening.
Henrik Göthberg:It is knowledge graphs everything Knowledge in launch language, but is this a purely fun and philosophical question or definition, or is it value and usefulness in these distinctions?
Anastasia Varava:See this problem solving, to be honest, like in, especially in more applied questions. It's, you know, it's. It's really like, first of all, can I solve this problem at all, Like whether the solution exists. And you can even like think about it mathematically. And then, if the solution exists, like, how do I find the best solution?
Anders Arpteg:And you know people are different people. Different people are driven by different needs and interests. For me at least, you know reason I actually left academia. To move between industry is actually the pursuit of value to see that the thing I spend time on actually brings value.
Henrik Göthberg:So in the end you kind of screw this knowledge thing. I want, I want engineering stuff, you are. You now feeling more like an engineer than a scientist?
Anders Arpteg:I still have the urge of it to spread knowledge, so I do still publish, not much, but you know it happens, you know. But I think at least you can spread knowledge when you build knowledge, because you do build knowledge all the time, every day of your life. But if you aim to spread knowledge in different ways, it can actually be to build a prototype.
Anastasia Varava:Exactly and, to be honest, right now I spread way more knowledge than I used to when. I was in academia.
Anders Arpteg:But you still build knowledge. It's just, it's not in the form of an academic, academic paper. It can be in other forms.
Anastasia Varava:You share. You share knowledge with people with completely different background and at least hope that it brings a lot of value, even without building prototypes. Everyone was just, you know. Explained, and then understandable terms, what the technology is and how it works.
Henrik Göthberg:Then is. Then is a small other angle on this, and I don't think it was Elon Musk who said, I think it was Steve Jobs who said it and now I'm almost swearing because we have this joke, so but he said something like and I'm paraphrasing that it's not until you start really building stuff, or you're really trying to work it out in real engineering terms, that you really get to the real problem and the real knowledge. And I think Elon has said something similar. Right? So I mean, it's the whole idea. You know we are, we are, we are iterating our way to Mars, we are building a rocket and we are blowing shit up, and we're expecting it to blow up, because that is where we learned.
Anders Arpteg:So I think this is also a topic around knowledge and it's like Jeffrey Hinton, you know. He said you know, he started as a scientist and still is a scientist to large extent, even if he was working at Google and still were very much an academic. But he said, you know, he wants to understand the human brain. That's why he got into AI, that's why he got into Sheila, that's why he got into neural networks, because he said, if I want to understand how the human brain works, I need to build one. Yeah, it's interesting.
Anders Arpteg:So he didn't build the neural network to have use for it. He built the neural network to understand how the human brain worked, and that's pure, exact, the definition of science. Interesting Right. Yeah. Then he come to the point that, oh shit, we built a neural network, or official neural network that isn't like the human brain, but it's better and it's scary, and that's why I left Google.
Anastasia Varava:It depends on what you mean by better.
Henrik Göthberg:It seems a part of the doomer camp, or not? How would you frame him, praise him? He makes a doomer comment, but is it part of the doomer camp? I'm not sure.
Anders Arpteg:No, I don't think so. He's a very sensible person, I must say, extremely knowledgeable as well.
Anastasia Varava:Do you?
Anders Arpteg:have any thoughts about Jennifer Hinton?
Anastasia Varava:I don't know much about him.
Anders Arpteg:We have spoken far too much about too many rabbits, so should we move into the VARA perhaps? Yes, I think we should go proper into.
Henrik Göthberg:VARA. I think it's a super important topic for Sweden actually.
Anders Arpteg:So, anastasia, please, what is the VARA NLP track or VARA to start with?
Anastasia Varava:in general, yes, and maybe we should move a little bit from tracks and a bit more in general.
Anastasia Varava:So it's something that is called the Warnenberg research arena. So it's essentially, and so first of all, wasp has several research arenas in different areas. So there is, for example, varro Robotics, varro Public Safety, so Varro Media Language is one of them, and the whole point of Varro's is projects, and they are really long-term projects that are funded by WASP. It's to create, essentially, a collaboration platform between industrial and academic participants to do exactly what we discussed, I think, at the beginning, so to help researchers find problems in real world that come from industry and help industrial partners to actually get support and solve in those problems and bring people together and do cool stuff together.
Anders Arpteg:Yes, yes.
Anastasia Varava:And Varro Media Language specifically. So traditionally we worked with. It kind of started with, I think, varro Language Before you go in there.
Anders Arpteg:I mean we should mention, I think, wasp in general.
Anastasia Varava:Yes exactly.
Henrik Göthberg:And there's a biggest.
Anders Arpteg:Swedish research program we have, but it is focused on research and it is focused on yes, it's focused on fundamental research, precisely In autonomous systems of NAA, of course.
Anastasia Varava:So could we summarize?
Henrik Göthberg:for someone who has not been deep down the rabbit hole. Wasp is originally a lot of folks on academia and research and adding PhD students. And Varro is now a subset where we are trying to go into this interface between industry and academia. So Varro has a sub-objective that is a little bit different to the whole. We need more PhDs in general. Is it the same or is it different?
Anastasia Varava:I mean, Varro also has PhD goals. You can also apply for PhD funding through Varro and you can also have an industrial PhD student through WASP as a general program. So there is no clear cut there.
Anders Arpteg:Yeah, okay, it's a bit of a overlap, but it's clear.
Henrik Göthberg:or more closer industrial applications, at least in Varro yes, yes, yes, exactly, but because when you read the, if you go in and read the documentation of the objectives and the steering letters, so to speak, I think there is a distinction between the WASP as an overall and Varro. I think really focusing on this intersect.
Anastasia Varava:Yes, in the sense that we have more people from industrial side kind of involved, definitely.
Anders Arpteg:Okay, so how did sorry? How did you get in contact with Varro and what is your role there today?
Anastasia Varava:Yes, so how did they get in contact? I actually inherited it.
Anders Arpteg:So, when. I started no long story short.
Anastasia Varava:Varro-Median language originally were proposed as two words. So there was Varro language and Varro-Media and then they merged and Varro language was actually initiated by SAP. So it was Nicolamok and Sela who kind of initiated that. And then it so happened that Sela left the bank and at the time there was another colleague of mine, kambis, who was kind of running the language track of Varro-Media language and then I joined the bank and I joined Kambis and then I kind of ended up being responsible for the language track.
Anastasia Varava:But I think now what we want to do is we want to rethink. We want to rethink a little bit the agenda of the Varro. So we're kind of in the process of defining strategy for the foreseeable future because we believe that just thinking about language is not enough anymore, because it kind of started before this whole progress and GPT type of models happened. So at the time it was maybe more relevant to talk about models for Swedish languages, something like that. But I think now so much happened in the area that now we can work with foundation models in general, and multimodality and embodiment, by the way, is going to be another track. So maybe we should do interesting name, I don't know.
Anders Arpteg:But it is combining media and language.
Anastasia Varava:Yes, exactly so learning comes from the natural yeah exactly. But I think we'll have more tracks. That's what I mean, yeah.
Henrik Göthberg:What is it? The embodiment? You open up a little bit Clemson on the specific topic there. Yes, exactly.
Anastasia Varava:So you can think of it as any kind of embodied agent. So it can be a physical robot or it can also be an agent that interacts with the world in a simulated environment, and there are kind of interesting problems on how foundation models and this whole kind of world of embodied agents are all played together.
Henrik Göthberg:So it links a little bit back to the hypothesis. We think, if we talk about large language models and RAG in 2023, the hypothesis, what prediction was that? Oh, we will start to talk more and more about autonomous agents in general in 2024, and it will be one of those words right, and you can see this now that how we, oh, when we open up the idea of embodied agents and all this it's many different dimensions in this. I guess quite clearly interesting that you are also seeing that in world, of course, yeah, exactly.
Anastasia Varava:Yeah, it was actually my suggestion. It also kind of coincided with my affiliation with KTH, because I was like okay, so it's very clear that foundation models are gonna make a huge difference in robotics in the next years. So how can we not involve embodiment? That's interesting, yeah, exactly. And then there is, of course, our robotics, but they are kind of, I think, a little bit different in the agenda. So we may collaborate with them.
Henrik Göthberg:But this is actually a topic on its own. What is the relationship between large language models and agents and robotics? Because for maybe, I think this, you know that we is this super clear to everyone.
Anders Arpteg:I guess it becomes increasingly overlapped. Right yeah, exactly why? Because you need to have a model that is not only working on text, not only working on a single language or not only working on a single modality or also working on agents or actions or also working on planning, and then everything becomes connected to robotics and other things. And you train everything in a single model, and now can you then separate out the things?
Anastasia Varava:It doesn't necessarily need to be a single model, I don't think I mean. So the most trivial thing that you can do if you start from the beginning is you can use your language model, which is just language, as an interface for the robot, right, so you can take human instructions, you can translate them into whatever the robot is doing and that just kind of makes it easier to interact with. That's a trivial thing. Now, if you have a multi-model model and, for example, you want to do planning in this kind of visual space, so you want to plan your intermediate steps, say images of your environment, that's where foundation models can also come in, because suddenly you can use them to generate your plan. And that's actually a pretty cool thing, because planning traditionally has been a hard problem, especially task planning when we have a lot of objects that you interact with because, of course, of dimensionality, essentially because suddenly the dimensionality of your space in which your plan kind of explodes.
Anders Arpteg:And we could go to another rabbit hole here of the. Have you read the JEPPA paper of Jan Likund, by the way? In seeing these kind of different components of a future, system? I don't know.
Anders Arpteg:But it does have different modalities of it. One of them is like perception, another is like a configurator to try to understand what's part of the brain or the network should do what. And you can have like working memory, which is something that doesn't exist in Chattivity but they actually added just like a week ago, or something Memory you know, the memory add, and so forth. So I certainly agree with that. We probably will have different modalities and parts of networks working together in some sense.
Anders Arpteg:But then the question is should it move to the end-to-end kind of training that Elon Musk is doing with the FSD in version 12?
Henrik Göthberg:But now we're going into rabbit holes, but I really like your summary here, because I was asking a question simply to get down to this story, because you can get into. Well, obviously this is the same right. But you can start from how do we create general AI, what is missing from a large language model in order to make AGI? And then we have the HEPA paper of Jan Lekund and we have the different. Well, what are the things missing? But then you can get into the actual. You can come in from, you know, from Elon Musk's point of view, when he's building Optimus. You know Optimus is building the robot, right, and it's a little bit like okay, for a robot to be functional, you need to be able to give autonomy and an agency for something. And how do we do that? And then, in the end, these topics converge. So I think going with embodiment and agents is actually, in my hypothesis, an evolutionary path to better understand. You know what is AGI, even stuff like this, but now we're moving into rabbit hole.
Henrik Göthberg:We were speaking about Bora.
Anastasia Varava:And then the dangerous one, which is AGI.
Henrik Göthberg:Yes, we moved into okay, back up again, back up again.
Anders Arpteg:If we just try to summarize it, summarize Bora, so we have the NLP track. It is expanding a bit in just minutes.
Anastasia Varava:Yeah, I prefer to talk about it in terms of foundation models, and I think that's what the strategy is going to be. And then we have partners from Geemen, so it's which partners are part of the Bora language? It's a really long list and I'm afraid to forget some.
Anders Arpteg:Some top names. Perhaps Spotify was part of it, at least before.
Anastasia Varava:Yes, spotify used to be, so we have electronic arts. I mean, we have us, of course, from the SAP side. We have SiloJoin and now we have Eriksson.
Henrik Göthberg:So it's quite a lot but yeah, I'm afraid I might forget someone, but is it more media companies or is it also more traditional manufacturing companies like Scania and them?
Anastasia Varava:No, scania, I don't think we have.
Henrik Göthberg:No.
Anders Arpteg:OK, but I guess in general this kind of Bora addition that has, how long has it existed, like 45 years or?
Anastasia Varava:something like that. It started before I joined.
Anders Arpteg:So I was part of that for a long while, but I think for five years.
Henrik Göthberg:But it's a lot of Bora in her new role, or has she sort of left this field area?
Anastasia Varava:We love to have her around, so she actually no, no, no, she's a part, yes, absolutely.
Anders Arpteg:Cool and I guess you are very much in favor of the Bora, since you have this kind of passion for industry, meeting academia in different ways.
Anastasia Varava:Yeah, exactly.
Anders Arpteg:And this is one way to.
Anastasia Varava:It's a very natural way, and it's also kind of a platform to figure out what works and what doesn't it's needed and what we can set up, maybe even in a more permanent basis in the country.
Henrik Göthberg:But moving into solutions or ideas for solutions so it's one of the topics here is, ultimately, how can we speed up from foundational research into real world application and commercial use? I think this is a core game here, and we think there is still a too big distance here and there are things that works and things that don't work. So can you give us a sort of your personal point of view of what is the sort of the blockers or gaps and what are the potential things we should work on to make this better?
Anastasia Varava:I think, what makes it a little bit difficult. So if you work in, say, theoretical computer science, then you don't have a problem because you just work in academia. But I think when you work in this kind of limbo of really applied problems, I think the problem that you run into is that if you want to do pure academic career, then you need to optimize for each index and excitation and all of that, and in that process you may be miss out on solving more real problems, because that doesn't necessarily lead to a publication rate, especially in the top. It's kind of orthogonal in a sense. So I think what we long term need to think about is how to maybe redefine this whole process and have more people working in R&D, not necessarily in a company per se, but kind of maybe centrally in Sweden, working with different application areas and things like that. I think that's what we're a little bit missing.
Anders Arpteg:Awesome, I was thinking. Were you connected to the Swedish AI Commission in a way? By the way, when SAP was right, no, no, no.
Anastasia Varava:It just happened to do a press conference to announce it at RPL and our lab, so that was just kind of fun, but I'm not involved in this work anyway.
Anders Arpteg:OK, but in general I guess you're in favor of and believe in AI will help the world and Sweden and our society in different ways.
Henrik Göthberg:This is a very simple question.
Anders Arpteg:How should we do it? How should we make the best use of AI? I think you have said so many good things already, saying that of course, we need to have some part of the foundational research happening, but it's not sufficient.
Anastasia Varava:Exactly.
Anders Arpteg:We need to bring it also to industry and we need to have them taking advantage of this and having their R&D and also their engineering work happening, but what is missing then, if you were to speculate a bit here, what should we do more? I guess Wasp is also helping in this, in actually funding this kind of research. Yes, exactly.
Anastasia Varava:So I think what we're missing is more jobs for people who want to have this either hybrid careers or work with applied research. That is a little bit, maybe longer term, than most companies are OK with, because, again coming back to your question, at SCB, for example, we're really blessed to have forward-looking leadership and it's really good that that's the case, but that's not necessarily the case for other companies. So you need to have another type of organization that is focused on applied research but, at the same time, has a broader horizon and longer time, yeah, longer time horizon.
Henrik Göthberg:But what are you envisioning here? Are you envisioning something that this government funded, or like that?
Anastasia Varava:In most countries it is government funded, exactly, but it doesn't need to be. It can also be private, and then you can. It doesn't really matter.
Henrik Göthberg:And then we have a given example and I'm not sure if that's the right one, but I mean, then we have an organization like RISE. In some ways that should kind of fill this topic, but maybe they are not really exactly where they need to be, or they have a slightly different agenda today.
Anastasia Varava:I think the problem usually starts from the fact that what sources of funding are right and what kind of your KPIs are, Because if you're supposed to work on real cases and deliver them to industry, then suddenly you're becoming very short term. If you are just centrally funded and are completely disconnected from companies, that's another problem, Because suddenly you're solving problems in vacuum. So I think it needs to be mixed.
Henrik Göthberg:So it's actually something with the steering model here and the federated joint Joint goals.
Anastasia Varava:Exactly so. You're not supposed to be fully profitable, because then you're not doing R&D, then you're just doing.
Henrik Göthberg:So one of the key topics here is that it's like a Venn diagram, and if they don't meet, and you have all the funding from this angle, we have all the funding from this angle, and they are both trying to throw money at the middle, where we need to kind of look at what is the entity that is in the middle of this and makes this. I call it federal or joint ownership in some ways. So actually rise doesn't have joint ownership and something that is completely commercial doesn't have joint in.
Henrik Göthberg:So there's another type of entity with some sort of joint ownership. Is that a summary?
Anastasia Varava:Yeah, I think so, because you need to have the flexibility of actually thinking about problems long term, but at the same time, you need to have a close connection to real world. So that's, that's the conundrum here.
Henrik Göthberg:So you can't really solve it in either camps, so there needs to be a camp in the middle. That is something that we don't really exactly have maybe it can be mixed funding. Mixed funding.
Anders Arpteg:And I agree to a large extent. If I just were to try to find some potential exception and I'm sure you agree with this as well is that most companies cannot do the more foundational research, of course, and it needs some more perhaps government funding or something else to happen, but a lot of research can happen in industry as well, and if you take the superscalars of the world, they do a lot of foundational research as well.
Anastasia Varava:Yeah, if you look at the meta team, for instance, or Google, yeah, but let us not forget that WASP, for example, actually comes from Privatsur's rate.
Henrik Göthberg:Yeah.
Anastasia Varava:Exactly, it's not.
Anders Arpteg:No, I find it's amazing, I mean that's another example of a tech, or at least a non-government agency actually doing a lot of foundational research and, frankly, it's a fantastic contribution to the Swedish society.
Anastasia Varava:Yeah, I mean, it's absolutely fascinating.
Henrik Göthberg:And I think it's offensive that the Swedish government is not matching what someone is doing, to be honest.
Anastasia Varava:Yeah, exactly, it's very sad.
Henrik Göthberg:I think it's super sad. I mean, ok, that we need someone to step up, like WASP, but not even trying to match, not even trying to be part of it. I find it offensive, to be honest. I find it really strange.
Anders Arpteg:But my point a little bit was that we do have some superscalars and they are super profitable, extremely the most profitable companies in the world literally and they also do more and more research and having even more money makes them able to do research even more long term. Yeah.
Anders Arpteg:And they perhaps are accelerating at a pace that is going the normal type of companies, and Henrik and I have just speaking about this for a long time. We call it AI divide in some way, and we can perhaps even more see it now, with large foundational models being increasingly important, that a very, very few number of entities in the world are able to train them. Is this something that makes you scared that it becomes normal? Universities can never train like a large foundational models these days.
Anastasia Varava:Oh, you can On Brazilus.
Anders Arpteg:It's not the scale of chattypity or it could never compete with the tech giants, kind of side.
Anastasia Varava:Yes, yes, but I believe that we'll have more data efficient and computer efficient models going forward.
Henrik Göthberg:So we should, but we should take it on and do this Do you think I mean today, we know it's not the case.
Anders Arpteg:I mean they have insanely more research than Brazilus has. Or even if you take all the European data centers we have combined, it's nothing even close to what OpenAI has or Microsoft has or Google has.
Anastasia Varava:Exactly, but as a pure researcher, I don't think you should be competing with big tech.
Anders Arpteg:Well, my point was that you are seeing an acceleration of the superscalers, that they are gaining more and more money, they are getting more and more knowledge, they are getting more and more value. This is a dangerous trend. If that kind of gap keep accelerating, it would be a concentration of power that potentially, is a bit scary.
Anastasia Varava:Yes, that's why OpenSource should be supported. Yes, good, this is a good topic.
Anders Arpteg:But I hope also that it could potentially reduce the importance of academia If they don't have the ability to do the type of research that a very few number of entities otherwise can do.
Anastasia Varava:But I don't think it reduces the importance of academia. I think that's actually a fundamental misconception, because it just means only one thing that the technology became ready for industry, which means that as an academic, you're supposed to think more long term, so just do research in other areas.
Anders Arpteg:Let's say that we should do the news section. I guess at some point we haven't done that. But there is one area now where OpenAI and SAM is investing insane amount of money now in something that is perhaps can speak about that shortly. But if this keeps accelerating they will have something proprietary that no researcher will ever be able to get insight into. They can't do research on it except through APIs. But really do any kind of introspection?
Anastasia Varava:Yes, that's the stupidity.
Anders Arpteg:And, yeah, it's a dangerous future. And should we do the news, by the way, I think, or should we? I haven't looked for news. Ok, but perhaps it's a good time to do the news then, in a way. What do you think, Eric?
Henrik Göthberg:I think we can stop here and, I think, take a reflection, because there's a big topic around the corner here, I think, to discuss how important it is with open source versus proprietary models, and I think it's somewhere where we can also question Academia should really be on the open source side as much as possible in order to you know, if we now want to pull our resources somewhere, we should really pull them into open source. But this is maybe then we take the news. Do we have the jingle?
Henrik Göthberg:otherwise we do. And now the AI news. And since Goren is not here, there is no jingle, goren, but here is the AI news of the week.
Anders Arpteg:Awesome, so for your.
Henrik Göthberg:It's time for AI news brought to you by AI AW podcast.
Anders Arpteg:So we've been trying this new thing during the autumn to have a small break in the middle of the podcast, and then we go back to the discussion and hopefully back to the open source question, which I'd love to discuss more with you, anastasia, about. But let's do a few minutes of some topic and the discussion, and then we'll talk about some minutes of some topic that we all speak about, and perhaps I can start because I have one connected to the very topic we just spoke about.
Henrik Göthberg:Yeah, so just, anastasia, if you have a topic of something you read in the news that you think is newsworthy. The last week more or less, so everything was happening so fast. So we thought, ok, what is the major headline news in the field of AI? If you have it, you have it, otherwise don't worry about it. I think I missed last week. Anders, you go first.
Anders Arpteg:Yeah, During the last week. I think it happened last week before this, but anyway, it's been one story that's been over the media quite a lot and that's at Sam Oldman, the. Now still again, the CEO of Open AI is apparently raising money for a new ship company and there's nothing new. We heard about Rain AI and it was like, ah, a couple of billions of dollars, Like, ah, you need 50 billion dollars for that.
Henrik Göthberg:But now suddenly they are speaking about raising five to seven trillion dollars which is an obscene amount if you think about the GDP of countries in the world.
Anders Arpteg:It is. It is absurd. I mean, if you take the, I think the market cap for Microsoft now is three billion. Apple is like, yeah, slightly below. And then you say they are raising this amount of money. You know, I'm an investor myself and if they are raising that amount of money, it should be valued at least four or five, perhaps 10 times this. If you take a company that's valued, let's say, five times, to have it in a low range to that, it's like 30, 40 billion sorry, trillion dollars. That's like the amount of all the publicly traded companies combined, but it's like insane amount of money?
Henrik Göthberg:Yeah, but why is he doing it then? Because then you need to unpack this. Is this used to marketing stunt? I mean, like the onslaught of comments on this obscene number, there are many angles to this. So how did you want to unpack that? Because that he said it. Good, is it a marketing stunt? You know what was your take on it.
Anders Arpteg:I really don't know and I hate to speculate this much, but I think it's such an important topic that we have to speculate even though we know nothing basically about it. But you know, Sam has himself commented on it on X, so it can't be completely rumored. But perhaps it's a marketing stunt.
Henrik Göthberg:But OK. So the backdrop is maybe important if you're not fully into this. It's like we were talking about the semiconductor business and that has been super concentrated to Taiwan and to actually one company in the world who has sort of such a world dominance. And I think during the COVID, in different ways we have seen how fragile the whole supply chains has been on this. So I think everybody grew up, if not before, so from the Scania to whatever company that we have a semiconductor shortage and in the end in the geopolitical powers. I think Sam is basically doing a very simple job. He's going to the people that has a geopolitical interest and say, hey, man, it's time to get into the game. And then, if you go back then and have sort of studied the semiconductor business and there are some really interesting books you can read on the history of semiconductors, the entry ticket into that game we are talking now building foundries and stuff like that, the foundational stuff to get stuff done, and this is massive. And then you add the pneumorphic to that story.
Anders Arpteg:But this is not the. This is the rainbow day. I don't think this is going to rain, Isn't?
Henrik Göthberg:the rain going to go pneumorphic. I think that the whole point.
Anders Arpteg:Yeah, sure, but I don't think the 7 trillion thing.
Henrik Göthberg:OK, you mean, the 7 trillion is your semiconductor in general.
Anders Arpteg:I don't know what it is, but I think it's more than rainbow day. But I think rain could be part of it, but I don't think that's the whole thing.
Henrik Göthberg:But I think the bottom line is that this is a geopolitical play by Sam Oldman, where he basically raises the super riches and the super powers, who sort of thinks we need to be in control of this destiny here and we need to dominate this, and then maybe those money makes sense. I don't know.
Anders Arpteg:Imagine going to some investor he's going to. He went to Saudi Arabia or Saudi Aramaic, the biggest oil producer in the world. They apparently invested in rain, I believe, but they were declined later by US because of geopolitical reasons, exactly. But to go to someone and say I want to raise 7 trillion dollars. And then someone what do you mean they have to have such a pitch deck to this one to say that you're going to invest more money than any company in the world is even worth today. I mean they must have. Then you can see so many conspiracies and theories about this. They are saying of course this is AGI. Of course they have now approved, if anyone going to put in 7 trillion. Of course they have been able to prove that they have AGI in house and that's why they're getting this. But that is just conspiracy theories.
Henrik Göthberg:We don't know if this is true, but in the end, if you take it all the way back, even if it's just a stunt, it works. It works because it raises the stock price of Microsoft, even it raises all this.
Anders Arpteg:What do you think, anastasia, about this crazy raising money on the investment?
Anastasia Varava:I'm on the cynical side.
Anders Arpteg:You don't think it's a stunt?
Henrik Göthberg:But I think there was some other interesting comments in relation to that. I can't exactly phrase the article I read, I can't quote it, but it was more or less like a little bit like what has the world come to when someone? How are we thinking philosophically as human species when we can, and how can we let the capitalist view on this dictate these topics? So something is fundamentally like there was an article that there is some fundamentally flawed thinking here, not precise amount, but more like what has the world come to?
Henrik Göthberg:I don't know if you read a couple of those articles as well. There was a lot of commentary on this. It's the person, if you think about it, it's the person of scene All right, let's leave that I have another one, but I almost let you do it, but I can lead into it. Openeye rolls out chat-gpt memory to select users. What is that all about, andes? Thank you for dropping that. I put the news on. But OK, so I can start it.
Anders Arpteg:But it's an interesting one, right? Yeah, it's very interesting.
Henrik Göthberg:I can start, but you need to take it up. So OpenEye has begun rolling out memory capabilities to select number of chap-a-t users. Ok, interesting, so we can now control the memory. We can put it off and on, but I think the interesting point that I think to some degree is missing in some of these comments is what it means. I think the articles I read now has done a pretty poor job in sort of wow, what's the wow factor of the memory topic here? So help me here.
Anders Arpteg:No, but this is back to the rag. I mean, if you were to guess what they have done, it's simply adding rag to chat-gpt and then putting that for every user and they put it to GPTS as well as separate memories. So in short, it's the, since chat-gpt can't be finetuned quickly and the only added or other way to go forward to be able to quickly adapt to what you have said recently is to have some kind of memory added to it. So this is perfectly the short-term working memory kind of thing that you add on top of the large language model that contain the basic knowledge. I don't think I say so, but it has short-term knowledge versus long-term knowledge than in two separate parts and of course, it's super powerful. It can remember. If you I want to always summarize the lay quarterly report from this company in this format, then you don't have to say it the next time, you just send it in and it remembers your preferences and whatnot.
Henrik Göthberg:And it knows your children.
Anders Arpteg:It knows everything you wanted to change, but it did have a feature, if I don't recall incorrectly, to raise memory. This was kind of interesting. You can, of course, turn it off If you don't want to have memory of what you have said before. You can turn it off completely. You can also say this I want you to memorize and never forget, maybe, but you can also go in and see what it has memorized and delete parts that you don't like. Imagine if I could do that to you, henry. I can go.
Henrik Göthberg:Henry, please remove these memories from the late nights in the podcast Exactly, you know the karaoke. Please remove this karaoke experience. It would be awesome. But Anders, you almost said it like ah, this is, you know, the short-term memory, or adding memory is a little bit about, you know you could call that is what we are doing with the RAG. So if this is a large language model, and when we add RAG, as we discussed before, it's a little bit like the short-term memory. Do you think that's the technique here?
Anders Arpteg:Or is it something else? I don't think that's short-term. Yes.
Henrik Göthberg:You think that's what it is. Do you understand that? That's literally what? When they say memory, it seems fancy and all this, but it's something that allows you to then put in your own text. I'm kind of sure of it. I'm not sure if you have it in your thoughts I haven't looked into it. No, I don't, because it's so interesting. Right, the technicians get a hold of it and we call it something you know strange RAG Retrieval of what?
Anastasia Varava:is it called Retrieval of augmented generation?
Henrik Göthberg:Retrieval of augmented generation, and then when the marketeers get a hold of it, memory, I mean it's obviously some kind of RAG-based technique.
Anders Arpteg:They simply have some passage of text that they or other type of content they remember over a session.
Henrik Göthberg:But and do you think people would like this? Because I can take another angle on this. Like that, it feels kind of safe. I feel like if I'm an enterprise, we have using them over there. But the RAG thing, I want to be having my own control and I do it, even if I'm doing it shitty. It's in my server, sort of thing. Well, now I'm gonna do my thing inside the other thing. So I think there's a question here. Will, okay, you can use the memory feature, but do you want to use the memory feature rather than do your own RAG at home? I think it's also a matter of you know, controlling your data here.
Anastasia Varava:Yeah, but so many people are not aware of that problem. I think yeah.
Anders Arpteg:And then maybe it's Gary the first time and we're first months, but then you get used to it.
Henrik Göthberg:It's gonna be so much more seamless, of course.
Anders Arpteg:It's like putting data on Facebook. You know it's Gary, but everyone does it.
Henrik Göthberg:Anyway, so the memory part. And then they launched that this is some sort of alpha beta. I guess it's not really out there yet for us to try, so we don't know yet. Good, okay, do we have one or two more news?
Anders Arpteg:Yeah, I think you know time is flying by.
Henrik Göthberg:Time is flying, so we had two news today.
Anders Arpteg:That's it Okay, or did you have something you wanted?
Henrik Göthberg:to do yes, did you have something that you?
Anders Arpteg:sort of caught you? No, I think.
Anastasia Varava:I completely missed last week.
Henrik Göthberg:Yeah, there are better things to do right.
Anastasia Varava:Or more urgent things to do More urgent things.
Anders Arpteg:Ah, man, cool. Should we move into before we go to the final kind of philosophical part? I guess we already had a lot of philosophical discussions.
Anastasia Varava:Yeah, about knowledge especially. Sorry, what About knowledge especially? Yeah, knowledge, yes, exactly knowledge yes.
Anders Arpteg:But let's go for the open source discussion, right, Please? I think that's a good one. Let's see how we can phrase it. I guess the question is we've seen OpenAI. If we start with that, they supposed to be open, supposed to have open source meaning code, open models, meaning parameters, open data, potentially meaning data trained on. But basically neither is true now for OpenAI.
Anastasia Varava:Yeah, none of that is true.
Henrik Göthberg:None is true.
Anastasia Varava:And it's hilarious that it's still called open.
Henrik Göthberg:Yeah, it's not the funniest cynical ah.
Anders Arpteg:So that's, you can laugh about that, and I saw some. Yeah, anyway, let's go there. It's kind of strange. And they also are a for-profit or capped profit company, as they call it but they certainly not are non-profit capped at least. Anyway. I don't think there is anything wrong with being for-profit, but then you should be open with it and say that you are driving your company for profit.
Henrik Göthberg:Yeah, but let's shop and in on the core topic now question.
Anders Arpteg:Yes, thank you, Eric. Okay, so that's potentially a problem with OpenAI, but in general, then we can potentially see a trend also with additionally large number of companies going proprietary, meaning not publishing their large foundational models to the general public. Let me start with that. Do you agree with that trend and what do you think?
Anastasia Varava:about it. I think it's a mixed bag, I mean. So I think you will have both kind of proprietary. I mean, OpenAI is not going anywhere, right, and there's going to be other vendors that have their own proprietary models, and I think that's fine as long as you have alternatives. So that's kind of where OpenSource comes in, because if you want to use OpenSource, it should be competitive enough in terms of performance so you can actually make that choice. That's yeah. I don't want to ban proprietary models, Certainly market for that. That's perfectly fine.
Henrik Göthberg:But, the core topic here I mean like let's add the Jan Likun argument here.
Henrik Göthberg:So, from a more societal point of view, language and I don't know how exactly Jan Likun is phrasing it, but it's more or less like this is something that for society, or how we understand language, shapes how we understand ourselves, and it shapes so much so obviously we need to have this open and contributed to by mankind.
Henrik Göthberg:This is the Jan Likun argument, that there is some fundamental topics that this needs to be open sourced like this. And then I have a third angle that we will have a guest here, erik Hugo, in a couple of weeks, who has a background in South Africa. He lives in Sweden, he's South African, but he has seen first hand, you know, the tech divide towards the developing countries and he is on a very, very strong opinion that if it wasn't for Linux, if it wasn't for the real open source, it did so much for the developing countries and basically it's impossible for 80% of the world to even think about paying for using chat GPT. So there needs to be something in order to not to build the AI divide, sort of in the macros topic. So I think this whole open source topic is also is huge.
Anders Arpteg:What are your main arguments for open source?
Anastasia Varava:It's to have alternatives really. So, like I said, it's the same as with software. So you want to have a model that is, you know, available to you. You can just download, you can find your own whatever way you want, you can do research again with it and you kind of want to not lock in yourself into like one of these big tech model providers. And I think the cool thing is that big tech is actually also fine with it, right?
Anastasia Varava:So if you say sorry like Google, for example, like their strategy. You can see how it has changed over the past six months. So if they start up a strain on their own models, then the narrative kind of shifted towards. You know, don't bet on the model but on the platform, and I think that's a healthy way of looking at it.
Anders Arpteg:Okay, and why do you think? Do you think it's because the potential abuse can happen if they do open source models? Or what do you think? The reason for Google to go platform?
Anastasia Varava:I think they realized that they cannot compete. Who is the open source community? And then there is no point in competing.
Anders Arpteg:Sorry, I misunderstood you. So Google, you said in the last six months have moved from what to what?
Anastasia Varava:From bet on the model to bet on the platform. That's the narrative now.
Anders Arpteg:Yeah.
Anastasia Varava:Which I very much like.
Henrik Göthberg:Can you decompose that? Yes?
Anastasia Varava:Yes. So what you can do, for example, as an enterprise, if you use Google Cloud platform, you can use either models that are trained by Google, be it Palm or be Gemini, or you can also use open source models in model garden, like in.
Henrik Göthberg:So what they are doing is they have their model, but they're opening up to using any.
Anastasia Varava:They're trying to be model agnostic, as long as they allow you, as a developer using their platform, to be model agnostic, and they expose open source models to you as well.
Anders Arpteg:If you turn that the other side around, they're basically locking you into the platform.
Anastasia Varava:Yes, but that's their business model.
Anders Arpteg:Yes, Okay, but it's not really moving in the open source direction. So I'm not saying that they have an altruistic motivation here.
Anastasia Varava:They need to do their business, and it's also understandable.
Anders Arpteg:But by Smetha. Then there was a for profit. Why are they still in the open source crack, you think? I think you know, as a lot of companies are filing, finding a lot of valuable business models based on open source code. There can be so many examples elastic search or whatnot, so many softwares that out there that are open source, and then you build some business model on top of it either some kind of enterprise license or some support service or whatnot but it's based on open source and by having it open source, it allows other people to help you develop it and it's actually very valuable, yeah, from a profit point of view, to have other help you developing your own product.
Anastasia Varava:Exactly, and this way you also kind of reduce competitive. Like you know, if you don't want to directly compete with, say, OpenAI training their own models, but suddenly you have a full range of alternatives, then OpenAI also will lose eventually the competitive advantage.
Henrik Göthberg:That's another, I think. What's the observation in the end and my take? If I look at sort of the classical example of analytics and how fast the open source has moved in terms of frameworks and what you can do versus what you can find in package application, we can go all the way back to self enterprise minor back in the day, and then when R really came into the university and it just exploded as a, so there was no way they could compete in terms of having. You know, do we think open source in the end sort of has the by the sheer numbers the upper hand, or is the ballgame different this time?
Anders Arpteg:Can we try to separate the now? What are the potential positive aspects of open source? I can think of a number of them. One is purely scientific. I mean, if you want to be scientific, you should open sources, because it's spread knowledge and it helps other researchers understand how does llama to work, by them having the ability to download it, look into it, examine it and do research themselves. It helps with research. So if you are pro research, you should be open with it. I mean, that's basically what science is.
Henrik Göthberg:You are open with knowledge right.
Anders Arpteg:So for scientific research or purposes, it should be obvious that you should put the model out there, potentially.
Henrik Göthberg:This is one core benefit.
Anders Arpteg:Another, I would say, is actually for profit. I think that there can be business models built on top, and we've seen a number of them where it is profitable to open source your source code and then you add a business model on top of it. So I think that can be argued as well not in every case, but for some cases. Then, I think what Jan de Koon is trying to fight all the time is the abuse argument.
Anders Arpteg:Now, this is what OpenAI and Google are all claiming they are not open sourcing because they are afraid about abuse of their model. Right, at least that's my take on it. They are afraid that they will take this model and do a lot of propaganda, they will generate a lot of sexually explicit content, they will do so many things that will build bombs and help people do terrorist attacks, and so many things that there could be or create a new coronavirus. I mean, there are so many potential abuses that you can use this incredible wealth of knowledge, knowledge that these models have and then the question is how do you prevent that? Either you close them up or you put them out there so people can help you combat it and find it.
Anders Arpteg:Yeah exactly.
Anastasia Varava:I think the latter approach is much better and again, it's kind of this whole demonetization of the whole area. That's something that is very close to me.
Anders Arpteg:But what do you think about the abuse approach? I mean what the question I mean is it would it be foolish of Google or, let's say, openai now has GPT-5 around the corner, or 4.5, or whatever it will call it, and potentially it's super powerful. Would it be negligent of them to simply put it out there and let anyone use it for whatever purposes they wanted to?
Anastasia Varava:I don't think that's going to happen. No, I don't think it would have, like I'm not a doomer, right? So I don't think that is a problem per se. And then again, they're not the only agents in the world who can train large models.
Anders Arpteg:Let's just be clear, but one of the few at least.
Anastasia Varava:Yeah, but you can have China training their own models. You can have China training their own models as well, and they do.
Anders Arpteg:Exactly. They put their own god rails and they are very careful about the resources.
Anastasia Varava:But if you're concerned about propaganda or Russia, that's inevitable. That's going to happen, and I don't think not. Open source and open AI models is a way to do with this.
Henrik Göthberg:It won't solve the problem.
Anders Arpteg:Exactly, if you were a Sam Oltman, you would open source GPT-4.
Anastasia Varava:That's a question about business model. I just think. I just don't buy into the risk argument.
Henrik Göthberg:Yeah. For him to stand on some high horses and arguing that this is better for mankind is bullshit in my opinion. Yeah, exactly, but he might have super legitimate resources to do it the way he wants to do it. But make no mistake, it's the business play ultimately.
Anastasia Varava:Yeah, they just say so.
Henrik Göthberg:Yeah, you say so this is perfectly fine as well, I think, because I think when you're trying to sort of hide the raw business truth on something that he doesn't really hold right, because you're not solving the problem, that way, it's very hypocritical yeah.
Henrik Göthberg:I don't buy it. I think it's quite easy to see through it and I think there is a place, as we said, for proprietary models and open source models. There are certain cases I'm sure what this makes sense or the whole platform, and you're locked in and this is terrible. But it's maybe way simpler For an enterprise example. It makes kind of sense because it's going to be really challenging in other ways to have it, I don't know. So there is a place for all. What about the core benefit that we need to solidarize?
Anders Arpteg:Let me ask you, henrik if you were a small man and let's say that you are not allowed to make any money from it, which actually is not, he has no equity in an open AI. But let's say that you had the responsibility of the profit of open AI and now it cannot make money, so let's say that it's impossible for them to use the argument of profit. Would you then open source or GPD4?
Henrik Göthberg:I would I mean like I actually I look at this more and more from the AI divide point of view. I look at more and more. I'm very influenced by Erich right now, who sort of understood. I think it's a real, real problem when we're going down a path where 80% of the world's population has no way of working with proprietary models because they don't have the financial means to do it. So I think to find models or to for us to sort of more heavily lean open source first, then, if that doesn't work, proprietary. I think this is better for mankind.
Anders Arpteg:So therefore I you know I'm not in the business position, so I'm, if I'm arguing from I'm just saying that the abuse argument is not something to be tripled with, at least, and I think it's very interesting what Jan Le Koon and Meta is doing and they've been successful so far. But they had a setback, if we go back a bit, when they build a language model for generating research articles, and they had so much backlash from that they had to shut it down quickly, but that they are still, you know, going back to it.
Henrik Göthberg:But let's be clear now help me out, Because Jan Le Koon's argument around abuse is that the safest way is to have it open, because then we can work on, then it's open for everybody to work. There will be people that are doing this for bad reasons, but now we can have more people working on finding the, the bugs, finding the holes and all that. And that's actually so, actually. So he's proposing that open source is actually the safest approach.
Anders Arpteg:That's his argument, but it's easy to see that anyone can then download the version and take this card rates away, because in the you know you see the problem?
Henrik Göthberg:Yeah, I see the problem, but there are clearly two camps where he has a philosophical view that he's safer and other people saying this is so powerful so we cannot let anyone look into it.
Anders Arpteg:I think in reality and this, but this is my personal- Let me ask you a very like provocative kind of question to you yeah, would you put a loaded gun in the sofa to your two year old kid?
Henrik Göthberg:But how is that relating?
Anders Arpteg:to our argument they would learn them how to use it right.
Anastasia Varava:It's a bit insulting to humanity, I think.
Henrik Göthberg:But it's. But can you? Can you use those kind of metaphors?
Anders Arpteg:If you have a large language models that tells you, you can ask it how do you build a gun or how do I build a bomb? This is one of the core like questions that you are used as an example. If that is given to any kind of person out there, even people with psychological problems or immature minds someone is going to do it. Yeah, Someone is going to use that gun to pull the trigger potentially, and that can be really dangerous.
Henrik Göthberg:So from this argument, then we shouldn't have open source models, we should have proprietary models.
Anders Arpteg:I don't have any answer.
Henrik Göthberg:I'm just saying but because is that? Why can't? Why can't you get exactly the same result from a proprietary model? Because they have to put safe guards in place, guard rails in place, or you mean like because when it's a proprietary model built as a product, there's a lot of there's a lot of things that can't remove it, yeah, and that can't remove it.
Anders Arpteg:That basically puts a lot of things you can easily remove it.
Henrik Göthberg:Okay, so open source you can remove safe cars.
Anastasia Varava:That's the argument. But is that really the biggest threat? Which is like just a random person, I don't know, some lunatic interaction with chat GPT and trying to ask it how to build a bomb and fail and because of the guard rails. Is that really the biggest problem?
Anders Arpteg:I don't know. You tell me like is that?
Anastasia Varava:the biggest threat. For sure, there are a lot of others.
Anders Arpteg:Exactly, Exactly, but still you know there will be a lot of abuses to it.
Anastasia Varava:Yeah, but. But. But those people can also Google for that information.
Anders Arpteg:But, but you have, yes, but that argument, you know the large language model is significantly more efficient in providing knowledge than a Google search is.
Henrik Göthberg:But, anastasia, what is your? Your fundamental stance on open source? You know, because I think you can arrive into, we should go open source from different perspectives. So what is your fundamental, what is your?
Anastasia Varava:entry point into why. Why, why this is good or why is the right way. So my main argument is demonapolization, because when you have just a few tech companies competing with each other on your kind of ultimately need to choose your poison between you know so here we don't have an argument, because I end up that this is the most, this is the biggest threat.
Henrik Göthberg:This is the bigger threat for mankind than if, if you, if you do the gun to a two year old argument. So for me it's like. It's like choose your poison.
Anastasia Varava:Exactly.
Henrik Göthberg:I'm choosing my poison, and I'm choosing it based on the same argument as you are, and I think it's about the AI divide, ultimately, for mankind, and I hope you don't think I'm against open source. No, no, no, we are still man. We are straw manning.
Anders Arpteg:I love it, we will, so it's my argument, you know, for open source would basically be sure you can try to keep some models safe, but it's just a matter of time if someone else would put it out there, so why not try to do it quickly?
Anastasia Varava:Because I know Andres.
Henrik Göthberg:I know he was still manning the argument because he is on this, is thinking exactly the same as us. But for the sake of discussion, I know what you're doing and I love it, but, but, but I think, if I'm, if I'm going to be super transparent, I think about this from Eric's point of view. Where we talk about taking AI apartheid, we're doing stuff that literally puts a large population of the world and we're putting ourselves in this monopolistic situation which has never proven good for mankind. This has led to revolution. It has led to really bad divides in society and economical, which leads to war which leads to geopolitical problem which is of much bigger scale.
Henrik Göthberg:And this is the ultimate. This is my end game, where I think choose your poison, and I would take all the different poisons in order to focus on getting this right.
Anders Arpteg:I think you know, just to end a bit with Elon Musk kind of. Sorry for this. No, but you know, completely removing the guardrails and regulation around these very powerful technology would be very dangerous.
Henrik Göthberg:Yes, I agree with this.
Anders Arpteg:So we need some kind of regulation because it will be very dangerous unless we try to minimize the risk.
Henrik Göthberg:But this is a really healthy argument, because if you fundamentally ideology, ideology wise, we say we must go open source, then we open up that kind of worms and then we understand what we need to do open source in the safest way. This is a good argument, because that becomes okay. What do we then need to do with open source for this to work in the best way?
Anders Arpteg:That's a good argument, that's a good discussion. Yes, but yes, so don't anyone thinks that we are, you know, in some kind of world without regulation, because that would be super dangerous with a so.
Henrik Göthberg:so it's an ideological top of open source. Then, from that standpoint, what do we regulate? How do we do it?
Anastasia Varava:Good yeah, regulating is one thing, but criminals can also train models if they have an free sources and if it's profitable enough.
Henrik Göthberg:Yes, this is true, this is true, it's a really good argument, really good.
Anders Arpteg:You're not going to ban GPUs.
Anastasia Varava:Well, they're trying to try.
Anders Arpteg:They're trying to, you know, have export control to China.
Henrik Göthberg:You know, because you get to the export laws around weapons and I can just imagine when you know with the whole AI Act you can sort of take this really wrong way that we need to have export laws on GPUs.
Anastasia Varava:And then you have a bookmark.
Henrik Gothberg:They have right. Yeah, nvidia did that. Yeah, but that's just a retro trend.
Anders Arpteg:But they will have their own GPUs. Sorry, but yeah, but they don't yet. This is a very other very interesting geopolitical political topic about, you know, TSMC and ASML and so forth, but that's and time is flying.
Henrik Göthberg:It's all ready, and it's in this vein that the seven trillion now makes sense for some people.
Anders Arpteg:Yes, yes. Interesting Okay great stuff Should we end up with the final question. Perhaps time has flown away and we are far above time limit here, but, anastasia, what if AGI becomes a reality? What if Sam Altman, seven trillion investments, come home and he builds something that is potentially an AGI? What kind of world do you think it will turn out to be? Will it be this kind of dystopian nightmare like the terminator in the matrix of the world, where machines are trying to kill all people?
Anastasia Varava:I don't see that happen in any time soon.
Anders Arpteg:Or do you believe more in the other extreme? I mean, you can think of two extremes here. One is the dystopian one, the other one is the super utopian one, where everything is awesome, we have a world of abundance. We have the world where we don't have any energy crisis, we don't have any hunger, we don't have any lack of education and humans are free to pursue the passions and creativity as they see, please.
Anastasia Varava:Yeah. So to start with, I don't think that's going to happen soon. So completely no, no no either, like I don't think.
Anders Arpteg:AGI is going to happen. Okay, do you have a prediction for that? What do you think?
Anastasia Varava:potentially Not in the foreseeable future. I'm very skeptical.
Anders Arpteg:In five years 10 years 50 years.
Anastasia Varava:Not in five years, but even if we were to hypothesize, I think the truth is always somewhere in the middle right. So because it never happened that, like we never had a complete dystopian scenario in history, I mean you.
Anders Arpteg:okay, you can, you can actually turn it, but it also never happened. No, but it was close potentially.
Anastasia Varava:Yeah, but but still, and now we're back. Okay and yeah. Utopia I definitely don't believe in because I'm not much of an optimist.
Anders Arpteg:That's a good one Okay.
Anastasia Varava:But yeah, I think it's, we'll just need to adapt it.
Anders Arpteg:Yes, I think that's a good argument, because humans and even Sam Oltenham said this once, you know he was asked the question, you know, will this not create a huge change in the whole world? And he said no. No, it will have a huge impact, yes, but humans will adapt.
Anastasia Varava:And we adapted so far to everything. Humanity still exists.
Anders Arpteg:And the future, where they have super intelligent agents. Actually, are you looking forward to that?
Anastasia Varava:I would like to see that, because I'm skeptical.
Anders Arpteg:You don't see the possibility of a super intelligent agent.
Anastasia Varava:I don't no, I mean, I don't think we're there no, no and. I mean sure I understand that open is absolutely not open about what they do, but just given the timeframe, I don't mind.
Anders Arpteg:I think 50 years. Your children, for example, if you take the Max Teagmark's argument, did you listen to a summer talk?
Anastasia Varava:Yes, and I was asked so many times.
Anders Arpteg:You hated it as much as me, perhaps, but what are you afraid about your kid's future?
Anastasia Varava:No, also he's a physicist.
Henrik Göthberg:I love it but actually, spinning on Max Teagmark his book Life 3.0, he paints a couple of different scenarios, like he's trying to build a picture of different scenarios. What different camps think about this? There's another discussion on sub-level okay, agi, yes and no. Then we have will there be some point in time where something goes a lot faster? So he has a scenario where actually we will actually probably get to AGI, but it will kind of be like I guess we passed it already, we didn't notice it almost. It's more evolutionary versus something where there is something happening where the speed really really exponentially goes much faster the fast takeoff, the fast takeoff. So it's the fast takeoff of intelligence or something like that. I mean, we don't need to talk about super intelligence, we don't need to talk about AGI, but we can talk about fast takeoff versus something that kind of resembles boiling the frog kind of thing versus what we're seeing now.
Henrik Göthberg:We're seeing chat GPT. It doesn't feel super fast in one way, but we have still it's still evolutionary.
Anastasia Varava:It's absolutely not fast if you think about it from the technical perspective, right? I mean it was fast for the general audience, because there was nothing and suddenly there was this.
Henrik Göthberg:But for everybody who followed GPT exactly.
Anastasia Varava:It was a very natural development. Yeah, it's just that they did right marketing. They exposed it to the general public for free.
Henrik Göthberg:They generated lots of hype, I mean sure, so in reality the fast takeoff scenario still feels kind of more unlikely than an evolutionary topic that we will reach there in some ways but we will not feel it.
Anastasia Varava:I think it's going to be absolute improvements because just the just life generally works.
Henrik Göthberg:And we will work on the next abstraction level of problems, right.
Anastasia Varava:Yes exactly.
Henrik Göthberg:And with this power. Now we have bigger problems. We can now yeah.
Anders Arpteg:Cool. I must still ask you know, were you not surprised by the scaling laws, by the ability for models to simply double inside and just continue to increase? Or you double the amount of data and it just continuously increase, increase in power and it doesn't seem to stop anywhere. Didn't that surprise you?
Anastasia Varava:I think it's a curious fact. I don't think it's much of a surprise.
Anders Arpteg:Okay, it was a surprise to me actually Interesting.
Henrik Göthberg:Okay.
Anders Arpteg:So how should we summarize your feeling about like an AGI future?
Henrik Göthberg:In the middle.
Anastasia Varava:Not happening.
Henrik Göthberg:No, no, no, Not happening in the near future, somewhere in the middle and evolutionary.
Anastasia Varava:Yeah, exactly, and humanity will adapt. Even if that happens, I'm with you, I think that's a pretty good scenario.
Henrik Göthberg:Actually, I don't think that's a dystopian, I think that's just like I think we'll be surprised by the pace.
Anders Arpteg:But so I'm more on. You think it's going to be kind of side here.
Henrik Göthberg:Yeah, accelerating it will. Accelerate, it will go faster. Yeah, it will.
Anders Arpteg:Anyway, I'm hoping that you can continue for some time, anastasia, and I hope we continue to speak about quantum computing, because I'm looking forward to speak more about you about that. But let's do that after the cameras have turned off, and thank you so very much for coming to this podcast. Very fun discussions.
Anastasia Varava:Thank you so much for having me.
Henrik Göthberg:So much fun. Thank you, thank you.