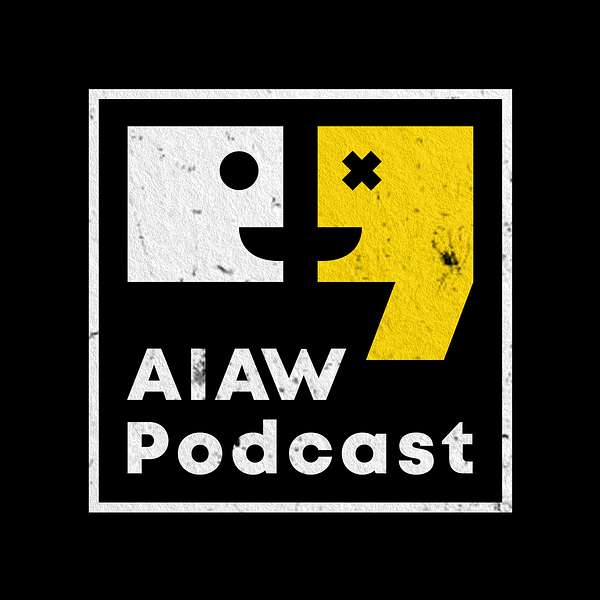
AIAW Podcast
AIAW Podcast
E135 - HPC in Sweden - Kjartan Thor Wikfeldt & Ingrid af Sandeberg
Unlock the secrets to cost-effective AI development and discover how high-performance computing (HPC) can transform your business. We promise to reveal the hidden costs of cloud services and how HPC presents a viable alternative, especially for small and medium-sized enterprises (SMEs). Join us as we explore the European Union's strategic investments in HPC, and the groundbreaking concept of AI factories. Learn about Sweden's initiatives to provide SMEs with the competitive edge they need to thrive on a global scale.
We're thrilled to welcome Thor from the European National Competence Center in High-Performance Computing (ENCCS) at RISE. Tor brings a wealth of knowledge in HPC and shares insights into the EuroHPC Joint Undertaking's mission to expand supercomputing infrastructure across Europe. Listen in as we delve into practical applications of HPC, from training AI models using tools like Slurm to the astonishing capabilities of supercomputers such as Finland's Lumi. Understand how these advancements are not just speeding up complex tasks but are revolutionizing the entire AI development process.
Get an insider's perspective on the collaboration between European HPC centers and SMEs, and the innovative projects emerging from these partnerships. We'll explore the latest from Sweden, including the upcoming Arrhenius system, and discuss the growing importance of specialized tiers for AI and data-intensive projects. Finally, immerse yourself in the future of HPC and AI, from the concept of a unified compute continuum to the rise of heterogeneous computing and the democratization of AI through large language models. This episode is your gateway to understanding how cutting-edge technologies are shaping the future of innovation and competitiveness in Europe.
Follow us on youtube: https://www.youtube.com/@aiawpodcast
No, but I think in general it's about like so when we're talking HPC for AI, then if you're a company, like a commercial organization, you're going to use what's best serving your purposes right, and most SMEs, for example, come from a cloud native environment, and that's not necessarily because cloud is the only option.
Ingrid af Sandeberg:It's just that cloud is. So it's there, it's kind of in your face, that's where you get started, and there's also a lot of services like it's there, it's kind of in your face, that's where you get started. And there's also a lot of services like it's built for uptime, it's built for scalability, et cetera, and really low latency and automation, and that's really good if you want to get something on the market. But if you have to do a lot of computations et cetera and use a lot of GPU power for compute and for training, then cloud becomes really really expensive. So for those use cases, it might actually be more economically beneficial as well to use HPC as a complement to cloud. People don't really know about that. So what we have been discussing a lot and and what I have been focusing on as well, is to kind of let smes know that this is an opportunity the eu is really investing in enabling uh private sector companies to use these resources because they see it as important strategically to also build competence within this area.
Ingrid af Sandeberg:So sort of how do we communicate to SMEs, like how do we reach them, how do we get them to understand that? You know, this is like there's millions and millions of euros worth of resources and compute and support and services available for free, like literally it costs nothing, waiting for anyone to just grab, waiting to be grabbed. Yes, I think you know the AI. It sounds too good to be true, but it sounds.
Anders Arpteg:So you were speaking about the AI factories and we'd love to hear more about what that actually is an issue. But to come back to what you said and what the main problem really is, because I guess we have the very famous cloud providers today and they have amazing services and they have an awesome tech stack and have great, you know, supporting system and it's very easy to get started there. But the problem I guess, as you said, is that if you have some kind of bigger product you want to run, it becomes really expensive and then we potentially in Europe, are lagging behind because we don't let some companies at least make use of data and AI to the extent that some other companies can. Is that basically what EU is seeing as a problem and they want to fix with this kind of AI factory?
Kjartan Thor Wikfeldt:I would say so, yeah, yeah, go ahead, yeah, no. I think that's a really important point, right.
Ingrid af Sandeberg:Go ahead. Yeah, no, I think that's a really important point, right, because, again, you see, sort of everything is coming from like all of the cloud providers more or less are based in the US, like NVIDIA is based in the US. Yes, we have AMD in the Netherlands and that's really important. But I think it's also like, on the hardware side it's an analogy that NVIDIA is, like the cloud providers, really really good at building an ecosystem around the hardware that makes it really easy for anyone to just come and use. It's kind of plug and play.
Anders Arpteg:And hard to leave NVIDIA as well. Exactly.
Ingrid af Sandeberg:And it's kind of the same because when we speak to the PDC people at KTH.
Ingrid af Sandeberg:So Parallel Data Center, to clarify the guys who work with Diredel the supercomputer there they kind of have a preference for AMD in terms of the hardware specifically, but they work directly on the hardware, so they don't actually need the ecosystem around. So, based on what I read from them is that the AMD hardware it might actually in some cases be better than the NVIDIA GPUs, but the ecosystem around it makes the NVIDIA GPUs so much more accessible. And then you're locked in. And it's the same with cloud right, because you start out and you get an AWS instance, for example, and then you have a lot of plugin services and then you have a lot of libraries that you can use, etc. So it's so easy. But then the hidden scalability of the cost right, it's going to increase massively when you start using more and more compute.
Kjartan Thor Wikfeldt:But at some point you know it might be. But we don't, you know, have the misconception that cloud or HPC is for everything. It's a research and development environment. It's for big computation. It will never replace cloud completely. The current access to your HPC right now is for explicitly research and development projects. It's not for deployment, it's not for everyday operations.
Anders Arpteg:You couldn't deploy a chat TPT on HPC.
Kjartan Thor Wikfeldt:I guess we'll come back to the factories, but one of the points there is that they are supposed to be more general environments, providing access to these frameworks which currently might exist in the cloud and not in HPC. And it explicitly says actually in the call that it's supposed to be an environment where you can train, you can validate, you can test and deploy models.
Henrik Göthberg:Yeah, so could we just summarize the context? What is the AI factory? What is happening now in time?
Kjartan Thor Wikfeldt:So you just put the wrapper on, so yeah, we brought this up because it's sort of on our mind right now. We've been talking a lot about it, so the call just opened last week I mean, it's been a long time coming there was the decision by the EU Commission European Commission in January to boost AI, and then there was a concept paper published in the summer and now, finally, uhbc opened the call. Everyone is free to apply. The deadline first deadline is in November, and so there's a mobilization happening right now in Sweden. Hopefully we will apply and hopefully we'll get funding to establish this.
Anders Arpteg:That's interesting. What should they search for Google for? Is it for AI factory?
Kjartan Thor Wikfeldt:I think that should lead you to the right place. Yeah, combined with UHPC perhaps.
Anders Arpteg:I think it's a super interesting topic. You know the theme for the podcast of tonight is a bit more into the infrastructure to the high-performance computing, and I think it's so interesting given the news in the last couple of months where we see especially the big tech giants investing insane amounts of money. Just saw Elon Musk and XAI releasing the new Colossus data center 100,000 H100 GPUs, basically bringing down the power grid of the state in the US because they put so insanely big data centers in production and when they actually trained the new DROC 2.0 there, they actually had ramifications to the power grid and the stability of the grid. For the rest of the whole state.
Kjartan Thor Wikfeldt:I remember reading something that they didn't actually have enough energy to power all of them. Just a third of the entire Colossus machine could be powered with the existing energy available in the state.
Henrik Göthberg:Yeah, so in this sort of context. It's going to be very interesting today to talk about high performance computing and how that is dealt with in Europe and how we can, as normal people, as normal companies, maybe tap into this.
Anders Arpteg:And when I think the need for things like EuroHBC and AI factories becomes even clearer, even more clear because it is to an extent that I don't think people understand. You need to put it into very understandable words, Like it affects the whole power grid of a state to understand the size of the current, like investments in compute infrastructure. Anyway, it's super interesting topics and I'd love to just welcome you here. Ingrid Sandeberg, is that correct? Yes, and Erik Kajtan Tor.
Kjartan Thor Wikfeldt:Ikväll. Well, to be precise, Kajtan, but I use Tor in Sweden, yeah.
Anders Arpteg:Awesome, it's going to be a super interesting topic Again. Let us just do a quick round of introductions first. So, ingrid, how would you describe yourself? Who is Ingrid?
Ingrid af Sandeberg:Oh wow, who am I? I'm an independent consultant. That started that. That's my occupation. But I think I am an independent consultant because I'm very curious and I love to work with different things, and recently I've been working a lot with AI, both as an independent consultant, because I'm very curious and I love to work with different things, and recently I've been working a lot with AI, both as an independent consultant, as a management consultant, as sort of operational in AI startups, and also as an investor. So I'm coming more from the general AI ecosystem rather than the HPC ecosystem specifically, and I've been working with Tor for a few months now.
Kjartan Thor Wikfeldt:Yeah, since May, yeah since May.
Anders Arpteg:And you have your own consultancy company? Yeah, I have my own consultancy company. I'm also chairing Stockholm AI, which is You've done that for a number of years now right.
Ingrid af Sandeberg:I've done that for a number of years and for clarification, it's not AI Sweden.
Anders Arpteg:No.
Ingrid af Sandeberg:And Stockholm AI is a non-profit community, so everyone engaged with Stockholm AI are doing that on a volunteer basis. So I don't work for Stockholm AI, but I'm chairing the board for a few years and also do some initial investments.
Ingrid af Sandeberg:You said yeah, so I've only done one, but in cybersecurity, also do some initial investments.
Ingrid af Sandeberg:You said, yeah, so I I've only done one, uh, but in cyber security, uh, it's an area that I've been very interested in for a long time.
Ingrid af Sandeberg:Uh, this company certified is sort of a more traditional cyber security, but I think that when you talk about security in a cyber setting, it's becoming increasingly related to ai as well, because it's more and more about intelligence and influence, operations and political influence, et cetera. And I mean, we saw already in the 2016 US election how, I think, russian bot farms was rumored to have reached, you know, a million plus Facebook accounts. And I think, as more and more information on the internet is also AI generated, it becomes quite interesting when all of these big new foundational models are trained on the entire internet and there's a sort of an inverse relationship between, I would say, quality and quantity of information. But generally, generally, if you thought a lot about your, your content, and you put it online, uh, then it might be a better quality than if you're a troll farm who have, you know, a hundred accounts and um per person or patrol and then it's pushing the same thing, all empowered by ai, now which?
Anders Arpteg:exactly tenfold their productivity, so to speak.
Ingrid af Sandeberg:Yeah, and I mean it was announced that was today or a few days ago that youtube will have this like gen ai video plug-in starting next year and I'm like, yeah, that's gonna be great even more, more garbage in, garbage out.
Anders Arpteg:Yeah noise yeah, interesting times for sure. Tor, how would you like to describe yourself?
Kjartan Thor Wikfeldt:well. Well, I have a background in HPC. Most recently, I am leading this project called ENCCS. I can talk more about that later, what we do and so on. It's the National Competence Center in High-Performance Computing. I work at RISE since two years back Actually, the project is older than that but the project belonged to Uppsala University. Before that and before ENCCS, I worked at PDC at KTH in Stockholm, the Supercomputing Center. I was there for five years and before that I was doing academic research.
Anders Arpteg:What was your main field of?
Kjartan Thor Wikfeldt:academic research. I was in physics, chemical physics. I used HPC systems as a user, as a PhD student and then postdoc and researcher, so that was a key tool in my toolbox. I was fascinated by the whole this middle way to do science. I mean, there's the traditional approach to science is theory and experiment. But then since decades back you can actually do computer simulation and I thought that was so cool, combining theory and and it's sort of like an experiment but on the, in silico, on the computer, and visualizing molecules, atoms, electrons.
Anders Arpteg:I would love to go into a discussion with you about alpha fold, and they have a new. It was a new one that simulates proteins as well alpha proton or something I actually didn't see that new story.
Kjartan Thor Wikfeldt:No, alpha fault is fascinating and it's not my background. I mean, it's more in the biomolecular domain, but um, as far as I understand, it's a, it's a game changer, yeah yeah, but that's not the topic of today, right, henrik?
Henrik Göthberg:no, so we're going to talk about HPC and specifically how we do it in Europe and specifically how we do it in the projects that you are working on.
Anders Arpteg:Yeah, should we start with the ENCCS? And if you were to just give a short background to what it is, how did it get started? And People can make this.
Kjartan Thor Wikfeldt:Okay. So I mean we are the EuroHPC National Competence Center in Sweden, so I need to tell you what EuroHPC is, maybe before it makes sense to tell you what we do. I mean EuroHPC it's one of the European joint undertakings, Jemensson Företag. It's like a funding agency. It's a common effort by European countries to, in this case, fund usage and work and research in high-performance computing. And the EUROHPC JU was formed in 2018, and the goal was already to rapidly expand the supercomputing infrastructure in Europe to actually start competing.
Kjartan Thor Wikfeldt:I mean, in decades and decades before 2018, Europe was never even close to the top 10 in the world. There's a list of biggest supercomputers in the world published twice per year. Europe was never there, but they've managed. So today we have three supercomputers in Europe on the top 10 in the world, the biggest one actually being in Finland. But it's not enough just to build out the infrastructure. I mean you need skills, you need awareness creation, you need training, you need consultancy, and that's why the EuroCC project, so competence center, was established Pretty big project with 32, 33 countries involved, and we are currently 32 national competence centers in Europe. We collaborate tightly.
Anders Arpteg:And what is a competence center.
Kjartan Thor Wikfeldt:Yeah, so I mean we employ HPC experts. We have various backgrounds but a shared passion for and interest in HPC. So what is a competence center? I guess there are multiple. There are other JUs, actually there are other joint undertakings. There will be a chips JU with chips competence centers to focus on the development of computer chips in Europe, but we are focusing on the HPC part. So we are a group of HPC knowledgeable people who reach out with support and help and awareness about the opportunities that exist today and also future opportunities. Like you know, we spoke about the AF actually.
Anders Arpteg:So how does it work? Is it more like a consultancy, that people in this center can actually go and work directly into some project for people that wants to work? Yeah.
Kjartan Thor Wikfeldt:So there's room for proof of concept projects. We can work on proof of concept projects with SMEs and startups, which involves a little bit more in-depth consultancy and help and support. In-depth consultancy and help and support touching the code, looking into the code that is being used, scaling it, making it run on GPUs or whatever it takes. That's an important part. But much of our work is also somewhat more lightweight. I mean, it's helping to get access to your URHPC systems, writing the proposals, all that paperwork. It's not rocket science, but it's not trivial either.
Anders Arpteg:It's not. I hope you make it easier than traditional kind of EU applications.
Kjartan Thor Wikfeldt:Yeah, I mean these are free resources, so you need to jump through hoops. I mean it's simply not allowed to just open it up without any effort. There needs to be some competition to be able to hand out these free resources right. But we help with that and we can help to get started. We can talk to a client, talk to a company, hear what they are doing, tell them what we can help with, and we can sort of together mutually arrive at a conclusion whether it's worth it to go for HPC and if so, we can help you get started. So that's.
Anders Arpteg:EuroCC and it's connected to EuroHPC, but then you have the ENCCS. It's a lot of abbreviations here, but what's the ENCCS?
Kjartan Thor Wikfeldt:It's an acronym for EuroCC, national Competence Center in Sweden. We've actually been thinking about relabeling it to EuroHPC just to put the HPC in there somewhere. Otherwise, what is the competence all about, anyway? So ENCCS there is also an NCC in Finland, norway, iceland, spain, greece, north Macedonia and so on. All over Europe we are this network of national competence centers that work together very tightly. We do events together, we share knowledge between each other.
Anders Arpteg:If someone in Sweden right now needs access to more advanced compute infrastructure, they should reach out to you, or to someone or to Ingrid or someone in ENCCS, or to someone or to Ingrid or someone in ENCCS, and they can help them get access to more compute.
Kjartan Thor Wikfeldt:Yes, I mean, we are the gateway. We're a one-stop shop, a single point of entry into the world of HPC. If you need more compute, if you're limited in what you currently have access to on-prem or cloud is it too expensive, Is it too difficult? We can take the conversation with you and evaluate your project Is it good for HPC? And help you get the access and get started.
Henrik Göthberg:So if I would put a theme on this podcast today, I would say and please correct me or improve on it we are talking about how normal companies, smaller, medium, even in focus can act and do in order to get access to hardcore, really high-performance computing and with effort, you can have it for free. So then we need to learn a lot about this whole thing. This is maybe a world not so many knows about.
Kjartan Thor Wikfeldt:In a way, this is democratization of compute power, right? So I mean, this is specific. Our action, our project is specifically targeting SMEs and startups, because a big company can hire their own people or recruit their own experts, build a team that just manages a big on-prem cluster, and they buy the cluster and they are happy. And there are multiple Swedish large companies that do this today, which you probably know. But this is simply not possible for an SME or a startup in particular.
Anders Arpteg:And perhaps it's necessary for people that's not familiar with the term HPC, can you just elaborate a bit more? What is the difference between general HPC and AI compute or data compute infrastructure?
Kjartan Thor Wikfeldt:So HPC and AI? I mean it's sort of like different beasts. Hpc is a tool, it's a computational backend that you can do stuff with. You can do AI on HPC, you can do AI on cloud. I mean the acronym is high performance computing and this is a very old discipline, going back I don't know 50 years or even more. Computer, oh yeah. So crave one, create two is the slurm and this kind of tool still yeah, oh, absolutely yeah. Well, there's always the talk of modernizing hpc as well. I mean we might see evolution. Slurm is great, it's really. It's open source, fantastic tool for many purposes, but I mean for the, at least the traditional use cases of. But I mean there are different needs. I mean the people we talk with too often they're completely fine with Slurm. Other times it's like why cannot not programmatically access the cluster?
Anders Arpteg:Okay, cool, but HPC in general, it can be used for much more than training AI models. It can be done to do simulations similar to what you did in the past, I guess for more chemical kind of simulation or biological, or if someone wants to build a circuit board and needs to to simulate the potential design of you know it's better than me, but I guess there are so many other applications than just training ai models on this right yeah, I mean, ai is the new kid on the block but if we talk about used to paint a picture, here we talk about, we have one of those top you know, top 10 supercomputers in Europe.
Henrik Göthberg:Now one in Finland Lumi. What is Lumi? Is it a GPU-based? What is it?
Ingrid af Sandeberg:A lot of GPUs.
Henrik Göthberg:A lot of GPUs right.
Kjartan Thor Wikfeldt:A lot of GPUs. Yeah Well, lumi is an AMD machine. It has AMD GPUs, amd CPUs. There are other supercomputers in Europe that have NVIDIA cards instead H100, a100. But what is it? I mean, it's okay. So I think you have to have a different mental model of what an HPC system is, a supercomputer.
Henrik Göthberg:They're sort of synonym words, but they're not exactly the same.
Kjartan Thor Wikfeldt:No, it depends on whom you talk to and what your background is. I mean, some people understand supercomputing to mean like the high end of HPC, like really running on the whole machine, massive scale simulations, while HPC is maybe just having access to the best in line GPU card which enables you to do high performance stuff. I mean it's much faster than running on your laptop. But in other aspects, you know, talking about supercomputing can also be easier to relate to somehow than HPC. It's an acronym high performance computing Sounds very computer science-y somehow, so I would be interested in hearing what you think about the difference between the two. So I would be interested in hearing what you think about the difference between the two.
Henrik Göthberg:I mean, as a layman I mean like, to some degree I get the annotation Is that for supercomputer or HPC, or you know the Cray computer or like that whole architecture? And then now we have a very much a GPU based architecture, which is essentially then the parallel computing to do more mathematics and, of course, very suitable then for AI. So for me, the modern discussion of HPC, for me it's, I guess, gpu-based at quite large scale, a lot of GPUs are the same that they're connected, so you can use them.
Kjartan Thor Wikfeldt:Okay, so I didn't quite finish the mental model I want to convey here, which is that a supercomputer is a bunch of nodes, compute nodes, which each acts as a computer, with its own memory and own CPUs and GPUs. But of course it's a very, very beefy computer. I mean often you have four A100 cards on each of the supercomputing HPC nodes and then you might have 128 CPU cores and lots of memory I mean 256 gig or a terabyte or something and of course the key thing is that the nodes are interconnected. So it's perfectly fine to, and the key thing is that the nodes are interconnected. So it's perfectly fine to get access to a supercomputer, an HPC system and run on one node. But the true power, the thing that makes it into a Formula One car in the world of computing, is that all of the nodes are interconnected. You can parallelize over all the nodes so it runs lightning fast.
Henrik Göthberg:And typically now used to connect it to AI and generative AI and large language models, is that, when you want to train one of those models before you put it in production we are talking about computing hours or computing days of sometimes the whole computer or Days, months. Months.
Anders Arpteg:Yeah.
Ingrid af Sandeberg:Yeah, but that's still GPU hours you're talking talking about. So if you have a lot of gpus and you can run them in parallel, it's going to be few man hours. So, for example, we had a conference two weeks ago where we were looking at some sma use cases and there was this company supersite in finland who'd be working on. You loom me to train a model and he was like yeah, so what would take me 15 days to train on my kind of powerful macbook pro? Takes me two to three hours on lumi, and then I want to try 10 different models, but I can do that in parallel on lumi.
Ingrid af Sandeberg:So instead of spending 150 days, uh, sort of chronologically, I would rather just spend two, three hours because I use the entire sort of chronologically. I would rather just spend two, three hours because I use the entire sort of the entire supercomputer infrastructure. So you can press the timeline a lot. But then, like I said, you know it works a little bit differently. It's not a production environment, so you have to schedule jobs a little bit differently. It's not going to have the same uptime et cetera. But even if you have way worse uptime, two to three hours is a lot less than 150 days, yeah, but but.
Henrik Göthberg:But maybe to use the llm example is a way to bridge to people who doesn't come from this world. So when you, you you're developing the new model and then you train it for days or months, and then when you, when you're done with the training and all that, then you sort of lock it and then you take it home and then this is the product that you're now servicing in GPT-4.0. So the difference is between all the hours of compute to train the model, the LLM, and then to bring it home and then productize it. So I think for many companies, if you understand what we're doing here with machine learning, is the difference between the training time and then to put things in production at home. So then you can have your one set up, you know how you actually use it with your customers in the end.
Anders Arpteg:But here to compress the job of training the model, the underlying model, that's what a lot of what this is all about, and I think it's interesting to get back to the training versus inference time at some point, because even if you have something trained here, you will need to put it to production some days and then you can potentially need even more compute.
Henrik Göthberg:But I'll add that to the list.
Anders Arpteg:Let's get back to that.
Henrik Göthberg:But was that my layman's example?
Kjartan Thor Wikfeldt:like to understand when we want to use a supercomputer in LLM terms and if you're adding something to the list, note down compute continuum as well. Compute continuum.
Anders Arpteg:Okay, I'm getting very. That's a good one. Yeah, let's see. You're picking Star Trek when you're saying that it's a cliffhanger.
Ingrid af Sandeberg:But I think, going back to like most machine learning models are trained in, they're first trained on data and then they're validated and then they're tested and then they're put into production. And when they're put into production, that's where the customer meets it, right. But if the training and the validating and the testing is sort of not super compute intensive, you can have it sort of in a production environment, you can have it in the cloud and then you can just deploy models quite frequently. But if each training is quite big, then you have a lot of lag between when you start training the model and when you sort of deploy it. And then if you put that in a supercomputer context instead and you compress from 150 days to two to three hours that's a very extreme example. I think it sounds very extreme then you can deploy models much quicker and you don't have to spend it, because each hour is cheaper on these machines and also, well, they're actually free. But even if you were to pay for them, because you can also pay for hours as well.
Anders Arpteg:I think, yeah, great example, and I think it would be fun to just go through some example project, perhaps something you have seen recently being done. But before that, I'd just like to close the topic. When we spoke about HPC versus AI, you also spoke about the biggest HPC clusters in Europe now, and we have three of the top 10 in Europe, which is amazing.
Anders Arpteg:And I'm very glad to hear that we are starting to catch up, even though we are a long way to go. But we mentioned Lumi and I guess that's the top one in Europe, correct?
Kjartan Thor Wikfeldt:Currently there is an even much bigger one under construction right now.
Anders Arpteg:Can you share more about that? Name the top three, or something, which one would you name that exists today?
Kjartan Thor Wikfeldt:to start with, Currently biggest in the world In Europe. In Europe is Lumi and followed by Leonardo. So Lumi came in on the list ranked number three in the world when it was built I don't know what two years ago. Now it's fallen to place five, which is very expected because other extremely large supercomputers are being built. And what happened actually in the meantime was that the first exascale supercomputer was built in the US, so they broke the exascale barrier first.
Anders Arpteg:Exascale is basically exa-petaflop or exaflop. Indeed, yeah. So 10 to the power of, is it?
Kjartan Thor Wikfeldt:18? It's insane. And flops is like floating point operations per second. Okay, but the bigger one which is being built. It's in Germany, at the Jülich Supercomputing Center, and it will be called Jupiter, Not Jupiter with a Y, but with an I like the planet. And so that will be Europe's first exascale system. A huge success for Europe, I mean-. Do you know when it will be launched? Next year, if I am not forgetting something. I think next year.
Anders Arpteg:But it's a Euro HPC project as well.
Kjartan Thor Wikfeldt:It's funded by the Euro HPC but like all the other Euro HPC systems, it's co-funded. I mean there's the Euro HPC funding and then national co-funding.
Anders Arpteg:And we have some Swedish ones as well, If you were to mention. We spoke about KTH and PDC and their kind of data center.
Kjartan Thor Wikfeldt:And what else do we have in Sweden? I mean, sweden has a pretty strong history in HPC. I mean there are two very old, in a way, supercomputing centers, hpc centers in Sweden. It's the PDC Center at KTH in Stockholm and it's NSE National Supercomputing Center in Linköping, and there's been sort of a friendly competition between the two, you know, and they've sort of taken turns. Well, they're married over time perhaps, depending on people involved, but they've taken turns to host the biggest flagship supercomputer in Sweden. Currently the biggest system is at BDC, the Dardell supercomputer. It's a mini Lumi, exactly the same architecture as Lumi, except I mean smaller Lumi, exactly the same architecture as Lumi, except smaller. But there have been other HPC centers in Sweden as well. There were six before. Now the goal is to centralize a bit more in coming years.
Anders Arpteg:And then we have some more AI specialized ones.
Kjartan Thor Wikfeldt:AI specialized one like Berserklius, yeah, I mean. So some of the HPC centers in Sweden have some side thing. So PDC has been working with Scania, for example, hosting their hardware to some extent, while Linköping has collaborated with so that is, national Supercomputing Center in Linköping has collaborated with SMHI run. I mean SMHI have been doing their weather forecasting calculations there and most recently built the Berselius AI supercomputer in Linköping.
Anders Arpteg:And that's also being funded by Wallenberg. Yeah, but you mentioned something, some new project as well. Is that something you can speak more about?
Kjartan Thor Wikfeldt:Are you referring to the upcoming Swedish EuroHPC system? Yes, yeah, so that's super exciting. I mean, what is it now? Two years ago, linköping University as the hosting entity of the National Academic Infrastructure for Supercomputing in Sweden, the HPC organization in Sweden. They're hosting it and they were given the mandate to apply to one of the open hosting calls for a UAHPC machine, and there was a strong team of people writing the proposal and they sent it and it was evaluated extremely highly and it was granted, and so the work is currently ongoing to procure a so-called mid-range UriHPC system. So it's not going to be Jupiter, it's not going to be Exascale, it's not going to be Lumi either, but it's going to be pretty good. So it's going to be bigger than the traditional so-called petascale HPC systems. It's going to be on the order of 40, perhaps petaflops.
Anders Arpteg:Is it going to be more like by CLU's AI-based or more HPC general kind of cluster?
Kjartan Thor Wikfeldt:I mean, the exact specs are yet to be revealed, but it's going to be a general purpose HPC machine. It's not geared specifically towards AI, but AI is still an important use case, so there will be GPUs. Exactly what flavor of GPUs will be seen? Does it have a name yet? So it's called Arrhenius Arrhenius, so that's a.
Henrik Göthberg:And what was the story here with the name?
Kjartan Thor Wikfeldt:Yeah, it's a good anecdote, because Berselius is there. Berselius is an AI supercomputer and if I tell you Arrhenius, if you're a chemist or a physicist, you think of Svante Arrhenius, who received a Nobel Prize, I believe, and he was born, living, active in the 1800s. Could he have received the Nobel Prize? Yeah, he must have anyway. So it's not named after him. It's named after carl axel arrenius, who lived 100 years before santa and worked with berzelius. So we'll have arrenius and brazilian side by side.
Kjartan Thor Wikfeldt:So they were co-workers in the past, yeah co Reincarnated in the form of a supercomputer today.
Anders Arpteg:Cool, great. I think you started to work with Tor now for some months ago, right? Can you just elaborate a bit more? How did that get started and what you're working with?
Ingrid af Sandeberg:So they're sort of building out the business development part of ENCCS. So I'm supporting with that and with business development. It's basically marketing these resources and helping SMEs on the one hand, understand what's there, but also helping URAs receive programs to understand what are the needs of SMEs. So, for example, why are they using clouds or what's required? What are the pros and cons for cloud and in what type of use cases for an SME would HPC add more value?
Anders Arpteg:And for people that's perhaps not familiar with the history and please correct me if I have the wrong history here but traditionally it's been kind of hard to get clients to HPC clusters, or companies are perhaps not as interested as they should be potentially.
Kjartan Thor Wikfeldt:It's not on their radar, right? What are you thinking?
Ingrid af Sandeberg:Well, I mean, when you contacted me about this, it was the first time I heard about HPC. So I think it's just like it's not on the radar. Like you said, it's not something you think about. You think about training models or like using compute. You think about training models or like using compute. You think about cloud.
Anders Arpteg:Yeah.
Ingrid af Sandeberg:Because I think it is like you mentioned. It's traditionally a lot more of a scientific environment. It's not as close to the product. And when you're a startup, normally or not normally, but oftentimes you want to have something very close to the market, very close to the sort of production environment and normally it's just when you reach a certain scale of computational requirements where it actually makes sense to put it in a different environment and train the models in a completely different environment before you deploy them. So it is only and now I mean we're seeing more and more data and we're training larger and larger models. So for more and more SMEs it might actually make sense. But it's easy, like we discussed before, it's easy when you start out in a cloud environment. You're sort of hacking together, you're getting some POCs and then you find different things you might need more compute for, but you're already in a cloud environment and you're not really aware that there's something else as well.
Anders Arpteg:So it's a threshold there for companies to get started with it.
Ingrid af Sandeberg:Yeah, Is this where we go back to the compute continuum thing? Well, maybe we can postpone it a little bit.
Kjartan Thor Wikfeldt:We could postpone it a little bit.
Ingrid af Sandeberg:Yeah, but there is a threshold right, because it is a different environment, because it is a different environment.
Anders Arpteg:Yes.
Ingrid af Sandeberg:So if you start out in a cloud, maybe you build your MVP, get your first customers. You train a little bit. You realize you can train a little bit more. You scale, you raise some money, you get some revenues. You want to train a little bit more. And then, sort of because cloud is built for scalability, right so you scale a little bit more, a little bit more, you pay a little bit more all the time. At some point it's going to be the cost-benefit equation is going to be in favor of HPC. But then you're so ingrained in the cloud environment.
Anders Arpteg:You're partly locked in because you're using that tech stack.
Ingrid af Sandeberg:You are locked in, on the one hand, technically or sort of organizationally or operationally, but also because, like you're just running right, you're, you're building a company, it's, you're, you're running like hell. And then can I say that on a podcast yeah, we're not americans um, so you're running there and you're digging where you are, uh and then? But this is a completely different way of training.
Anders Arpteg:I think it should also be frank that these kind of top cloud providers, they have an amazing service, I mean they are self-serviced to a large extent. You can actually get started very, very quickly, very, very easily Awesome documentation, awesome support and everything which is hard to compete with. But, as you say, the problem with them is that it becomes really, really expensive when you have to start to scale something up.
Ingrid af Sandeberg:Yeah, but it's like we discussed with the chips right, you have this ecosystem of support and services, which is really great. It comes at a cost and that cost is a no-brainer at a smaller scale, because you can't afford to have all of this stuff in-house, but at some point if you're training really really large models or if you're using a lot of GPUs and CPUs per hour, or, if you want to, in the future, if you have an idea how to scale up.
Kjartan Thor Wikfeldt:You know, if we only had so much more compute, what could we do then? And then HPC becomes a factor.
Ingrid af Sandeberg:Yeah, we've been discussing with some SMEs like, yeah, if we had that we could do all of these things.
Anders Arpteg:That we've just discarded for now because it's completely economically unfeasible to do it. Can you elaborate a bit more? Do you have some example, perhaps businesses or projects that you are focusing on? Or what would the stereotypical favorite kind of SMEB that should contact you know ENCCS and hear more about their potential?
Kjartan Thor Wikfeldt:We've been focusing a lot on AI SMEs lately through Sorry what. Ai, smes and startups. Yes, through English Connections and her great work over the summer, and it's been super interesting to get to talk to these people.
Anders Arpteg:And when you say SMEs, how large type of company are you speaking about?
Kjartan Thor Wikfeldt:I mean, well, we use the European definition because that's what we have to adhere to in our work. I mean, we can do certain work with SMEs which, for free and the Proof of Concert projects are restricted to SMEs, and the definition is European. One is up to what is it? 300 people or something? 250. 250. Employees, right, Employees? Yes, I think there's a number on the total turnover or something as well.
Ingrid af Sandeberg:I'm not sure I mean, you can still access these resources as a larger company as well, but the terms might differ slightly.
Kjartan Thor Wikfeldt:Yeah, and SMEs have other exceptions too, just to take that detour. So I mean, we're supposed to focus on SMEs and that's what we want to do, because that's where we can make the most impact. The big company can do their own thing. They can buy own hardware or what they need. Smes can't. So there are these exceptions. We can support them extra much and they also can get exceptions to the open science requirement for your HPC access.
Anders Arpteg:Okay, so there is otherwise an open science, basically an open source license saying that the science should be open for them to use it.
Kjartan Thor Wikfeldt:Well, it's not so precise as to specify.
Ingrid af Sandeberg:You know that everything should be open source, but, um, uh, there's like uh explicitly written somewhere that work done on your HPC should be as open as possible but as closed as needed, and that, in particular, for SMEs, you can, yeah, but it literally says you might need to loosen the requirement on open science if it's in the interest of the beneficiaries the beneficiaries being the company who's using the resources and they're also exemplifying that this could be, for example, for competitive reasons or data privacy reasons or stuff like that. So it's it's not that you have to publish everything you do, but I think the general thing is that we're giving you resources uh, it's good to know what they're used for and if there are any learnings that can be shared in the wider community just to have some company out there you know be identifying with.
Anders Arpteg:You know, this is something that could be useful for me.
Kjartan Thor Wikfeldt:Yeah, I didn't finish the story about the types of companies we worked with. I mean, we, we had contacts with companies um in past, many of them, some big companies and some smaller companies. There were already AI companies we spoke with two years ago, before the LLM hype and everything. There was a company doing speech synthesis, text-to-speech, another one doing battery inspection with computer vision and they used successfully your hpc with our help. But there there were many companies as well doing the traditional hbc domains like computational fluid dynamics and electronic structure calculations, molecular dynamics, biomolecules, yeah, but it's really been an acceleration of contacts with ai companies lately and I think that's a good thing. I mean, that's certainly the signal we get from above. You know, find your ai smes nationally. I mean message we hear and it's directed to all the national competence centers in this network that you, you know, focus on AI.
Anders Arpteg:In my very subjective view as well, I think Europe in general, at least in the last 10, 15 years has been has had far too little focus on AI versus, like US and China, of course, put much more effort into it. Do you think that's something they are trying to remedy now a bit by actually putting more focus on AI, or would you say it's similar to what it has been in the last 10 years in terms of investment in AI-related?
Kjartan Thor Wikfeldt:Well, my impression is that it's accelerating I mean, it's really a higher level of ambition now than it ever was before, exemplified by the AI factories, you know and making the URHPC machines currently existing ones and future ones as well more usable for AI companies. Another example of this is that when you apply for URHPC, you can choose different tiers smaller tiers, which are really easy to get you just write a small technical application evaluated by one HPC expert on a technical basis. And then there are bigger tiers, you know, going up to extreme access. But recently your HPC introduced the AI and data intensive access for AI and data intensive tier to your HPC.
Henrik Göthberg:To make basically for these use cases. Find if use cases try to be as smooth as possible for these use cases to be usable.
Kjartan Thor Wikfeldt:Yeah, I mean, it doesn't affect really how usable the USBC systems are themselves, it's about the administration and how you apply and so on. I mean, and the whole on, I mean and the whole atmosphere in in europe in your hpc, um, and the commission is to facilitate as much as possible. Yeah, companies to get to, to accelerate, I mean to grow as quickly as possible.
Anders Arpteg:there's really a lot of focus on smes and and in ai, and you have um given a cliffhanger now a couple of times here. What is really the compute? Does it?
Kjartan Thor Wikfeldt:continue. Well, I don't know who coined the term sometime in the past, but it's adopted by our brilliant colleague and friend at Rise, johan Kristiansson, who has his vision for the future. He comes from the cloud we're comparing cloud to HPC all the time now, right, and it's a very pertinent, very relevant comparison to do and he comes from a cloud background and realized the importance of so he sees the complications with cloud. You have hundreds of different services you can find from these different cloud providers and then through research at Rise, there's lots of research on edge computing and with us, ian says yes, when we came into Rise. There's again a focus on HPC and this Smörgås board is just growing right and they're all distinct. They all have their own distinct qualities and complications. We need something to glue it all together and the future vision with the compute continuum is that compute should be like a commodity, like electricity. You plug something in the wall, you get electricity. You should be able to do the same with compute Amazing vision to have.
Anders Arpteg:Oh yeah, and as a step in the wall you get electricity. You should be able to do the same with compute.
Kjartan Thor Wikfeldt:Amazing vision to have. Oh yeah, and as a step in that direction he has been developing single-handedly a very cool framework called Colony OS. It's a meta operating system which is Colony OS Colony okay, os as an operating system. I mean it's more computer science than I care to know. But there's lots of ingenuity in this platform and it's meant to abstract away all the complexity of the backends. I mean you can keep data in cloud S3 or something you can run certain lightweight things on your own computer or your on-prem, whatever cluster. You might have edge nodes and then maybe you have a part of your workflow. Is compute heavy r&d related, whatever it needs to run on hpc, and this framework can orchestrate and automate a lot of things. And this is not an old idea. There are not new idea. There are many projects that have been started and not many have actually reached anything. But this is production ready. This is already in production in Sweden and it's something we're looking for potential interested parties and trying out. I mean in actual production.
Anders Arpteg:Because in some way, if you see the top scale kind of compute project take LLMs or whatnot they seem to be super optimizing more and more specific compute projects. For large LLMs you need to have a super high bandwidth. It's not really limited anymore, it can be more like the bandwidth with limitation. You need to have a super high bandwidth. It's not really limited anymore, it can be more like the bandwidth with limitation. Because they need to send the gradients around to such an extent for these large models that it's actually limited by bandwidth rather than compute potentially. And they seem to be optimizing everything and making it work only for transformer-based and only for this specific use case. And this one is going in the other direction, which I think is interesting. I think both should be interesting areas of research. But would you agree with it that some kind of movement is just saying how can we just make this work for this very, very specific use case?
Kjartan Thor Wikfeldt:Yeah, I mean it's all moving into more heterogeneity, more variability of different platforms, different architectures, optimized for different purposes, and that's we're only seeing the beginning of it, I think I mean that's a whole new topic that we might go into. But Europe wants to become sovereign, wants to become independent in hardware also, right, so not relying on the big hardware companies in the world, but actually having homegrown technology for CPUs and GPUs and so on.
Henrik Göthberg:We're talking now about a push from the chipset to the computer, to the data center, infrastructure, hardware, and then we want our software and SaaS companies as well. I mean, this sovereignty goes across the board, this topic.
Kjartan Thor Wikfeldt:But the point I was thinking about was that you know the heterogeneity, the variability of different hardware is only increasing and there will be customized chips, customized FPGAs. You know you might have quantum computers in the future which might work well for certain workloads and you need some way to orchestrate all this complexity, or manage the complexity and orchestrate workloads across.
Henrik Göthberg:And this heterogeneity. Is that a given trend? Is that a given that it's the right path? Is that obvious? Is Houston like a law of evolution of the use cases? Or why are we going? Heterogeneity.
Kjartan Thor Wikfeldt:That's a very big question. Is it good or bad?
Henrik Göthberg:well, it's feels to me to be unavoidable.
Kjartan Thor Wikfeldt:Yeah this is what I mean?
Henrik Göthberg:like, like, almost like. Uh, the gene is out of the box, like it's a law of what we are doing now is leading down this path.
Kjartan Thor Wikfeldt:I mean one um driving force behind it is, of course, efficiency, efficiency, energy efficiency. You don't want to train AI models on CPUs because it's more efficient, it's faster and more energy efficient to do on GPUs.
Henrik Göthberg:I think boom, you put your finger on it maybe one of the most important topics here. When we talk about energy consumption, as we're saying, should we have these huge mega models for everything, or do we need to have ensembles of very small models? And there is something you know hello the cost and the energy footprint is sort of driving us to be efficient in what we're doing.
Ingrid af Sandeberg:Yeah, I'm going to stick my neck out and say that I agree. I mean, I think I normally I work a lot with also people who aren't that into AI. And for people who aren't in AI, llm has made sort of AI accessible for everyone, because suddenly you can talk to an AI with human language. So you use them for everything, like what should I cook for dinner, or whatever. It's great.
Ingrid af Sandeberg:And talking about AI, a lot of people are now just talking about lms or gen ai, but to a lot of applications where the data or what you want to do is not necessarily natural language based, then using an lm to get an answer is like going with a um, an air cross carrier, to get sort of small fishes. Right. You're using a lot of energy to get something fairly simple out because you want to be able to use natural language, but then you have to have like an insanely big model to translate that into something that the computer understands, make a very simple calculation and then translate it back to you using a lot of compute again, which is extremely inefficient.
Ingrid af Sandeberg:I heard somewhere and I haven't checked the source, but I have heard that prompting chat GPT could take as much energy as charging an iPhone.
Anders Arpteg:Interesting.
Ingrid af Sandeberg:I'll have to double-check that, but it's something I've heard several places.
Ingrid af Sandeberg:I love these kind of metaphors and even if it's not that much, but I have heard it from several sources but I can't remember which one, so I have to add a disclaimer there. But it's like it's not just like turning on a light bulb for five minutes, it's actually quite a lot. And then you do like these super simple things because you can. I mean, it makes it makes no sense. And then you have these newer models, like the 4.0 and the 4.01, for example, and then you kind of see that they might be trained to be a lot better at some things. But then going back, like the GPT-4 might be better at like super general things, because it's not as pruned and it's not as specific for performance on a certain set of tasks, it's just like super general. It just calls the entire internet to be able to give you like super simple answers on a question, because it's just general.
Kjartan Thor Wikfeldt:So it has to know everything to be able to give you super simple answers on a question, because it's just general. There's actually a funny analogy here between a CPU, the general purpose processing unit in a computer, and the super big LLM which is good for rather good at everything. But specialization is key maybe to energy efficiency.
Henrik Göthberg:So it seems like we can sort of draw a generalization here from hardware being efficient specifically, all the way up to how we use different types of machine learning versus, you know. So we all can answer to every question that comes into the team then we have to know a lot more.
Ingrid af Sandeberg:But if we specialize we can have, we'll be able to cover a lot more grounds because we can just direct questions to each person. And it's the same with compute. If you have one model that has to be sort of the silver bullet and have the answer to everything, it has to be so much more powerful. But if you want to dive into a specific domain, then you want to have a model who specializes on that domain. It doesn't have to be a specialist on everything else.
Anders Arpteg:I think that's a super interesting topic and perhaps we can move to that. Let's go there. I think that's a super interesting topic and perhaps we can move to that.
Henrik Göthberg:Let's go there now. Let's go there.
Anders Arpteg:And for one I certainly agree, of course, with what you said the generative AI hype that we have there. People that are now calling themselves for AI experts are, in reality, just chatty PT so-called experts and don't understand that AI has been around for 70 years.
Anders Arpteg:And there are so many other AI techniques than LLMs that are super useful, and then you need high performance computing to train them and you can't use LLMs for everything. That was it. Sam Oliver said something. There was a news about XGPOOS 2.0 being released and the comment or the meme was ah, I haven't heard about that LLM, heard about that LLM Super fun kind of analogy.
Anders Arpteg:But also, if we just expand this question that we spoke about and try to think like a couple of years ahead in the future, we can at least see that the frontier kind of foundational models are increasing in size and potentially fewer and fewer companies can at least train them from scratch.
Anders Arpteg:I mean, even with Lumi at hand, it would be hard to do it today. And then, if you just try to extrapolate a bit in the future, I think even using them in the future will be so expensive that it won't really be feasible to use at least the largest O1 model that we have in the future. So then you do, I think, what you just said, ingrid create more and more specialized models. So perhaps you use these kind of large foundational models to be a teacher for them and a starting point, but then you fine tune them for your own data that you haven't seen and for your very specific use case if that is a baking recipe or, you know, trying to understand x-ray images or whatnot. But that could be a future right and that can be a way for us to have the need to use, like HPC and these kind of AI centers, even if you can't build the biggest ones.
Kjartan Thor Wikfeldt:Sure, we don't have 200,000 GPUs like X has, but we have enough to actually do really useful stuff.
Ingrid af Sandeberg:I think what's interesting about these huge models is that they're able to learn from different fields. And I mean we saw it was a while back now, but when we saw the first chat GPTs was that they were able to sort of draw conclusions from one field and then sort of understand physics or something like that, so they can learn from different fields, which then like, if you can, if each learning from each field can be applied to all fields, then of course you accelerate learning. So I think to have a few of these large model, it's going to be great to kind of push the frontiers and also understand where does it make sense to build specialized models. But it's not going to be great to kind of push the frontiers and also understand where does it make sense to build specialized models. But it's probably not going to be the ones that we normally use. But it makes sense to have also maybe one in Europe and not just in the US and in China, for example.
Anders Arpteg:We would love that.
Henrik Göthberg:But I think this is a huge topic because everybody's sort of oh, let's go home and die, we lost the race, right, it's all over.
Ingrid af Sandeberg:It's not.
Henrik Göthberg:Who says that? Who says that? Because all of a sudden now we are realizing that, okay, there will be some super big very few of the biggest of the kind and they will have a, but in the end it's almost like they're going to be pushed back and we're going to use them in order to train the models that are then done in Europe for maybe automotive or maybe an applied research field or an applied business field. So it means that how this market will structure itself and where the opportunity lies for Sweden or Europe to actually be best in the world competitive, it's still an open field. When you start understanding where this is going and this heterogeneity topic, this data continuum topic, when you get your head around that, now we can see. You know we're going to be the best on applied AI in Sweden or in Europe. You know, okay, here is what we're going to play. I think this is a quite feasible conversation. You know strategically where to go. I don't know.
Ingrid af Sandeberg:What do you think? Yeah, and I think also, I mean and now I suppose I'm going out on a limb here but I suppose if you have a lot of different or sort of diversified models and you have this compute continuum, perhaps you can kind of get the same effect that you learn from different fields, using sort of an overlay, if you will. I don't think that we should sort of disregard that. Being in the race of having you know these large foundational models in Europe as well is probably quite important for longer term competitiveness. But, like I said, I think less and less of actual work or production is going to be on those super large ones, because it's not energy, economically feasible.
Anders Arpteg:I think it's also driven to the inference cost. Even if you use APIs and pay the cost it has there, it will still cost quite a lot if you start to put it in production and use them extensively. The cost it has there, it will still cost quite a lot if you start to put it in production and use them extensively. So then you perhaps need to do train or fine tune your own smaller model that is sufficiently big to work for your use case and also economically feasible to run.
Henrik Göthberg:Yeah, it feels like and you can find a niche here. So you can basically find a position in the market. You know from. You know market from the product UX to where is compute done, where is the majority of the compute really done? To train the model over here, but an inference in production, maybe in a completely new segment.
Kjartan Thor Wikfeldt:Yeah.
Henrik Göthberg:Interesting.
Anders Arpteg:I think we should have a potential small break here. What do you say, kirill? Do it, let's see. But we usually take a small news break in the middle of the podcast to just speak about the recent news that we've seen and been interested in, like last week, and there's been at least one super, super big one. But let's go around the table and see what kind of new starting would you like to speak about? Tor, would you like to start?
Kjartan Thor Wikfeldt:yeah, well, we sort of already talked about the af factories. I mean, that's very important to our field, to what we're going to be focusing on in coming years, and that's exciting for us and but it's sort of you on in coming years and that's exciting for us, but it's sort of you know, we'll see where that leads.
Anders Arpteg:But that's something that ENCCS will also spend time on.
Kjartan Thor Wikfeldt:We will play a key role there. I mean SMEs, companies and general startups are going to be important stakeholders and recipients of services from these AI factories, so we are the natural partner there too.
Henrik Göthberg:And when did the call actually come out? When was it Last week, last week?
Kjartan Thor Wikfeldt:This is news, this is super news, super new news, yeah, but you know, maybe it's fun to raise something slightly more technical, geeky. You know which is closer also. I mean I'm not an AI expert by training as such, but I read an interesting article about which is closer also. I mean, I'm not an AI expert by training as such, but I read an interesting article about Kolmogorov-Arnold networks, cans, which was fascinating to read, and then I saw that it actually came from Max Tegmark's group at MIT, so that was pretty cool. I mean fellow physicists who turned AI expert and I don't know AI safety evangelist and so on. Doomer, yeah, that's another way to put it, but yeah, so that resonated with me because it's physics.
Kjartan Thor Wikfeldt:I mean it's not going to be so okay, cans, kolmogorov-arnold networks, replace weights, basically in the networks with functions, and it's different mathematics. There's a different underlying formula for the expected efficiency of these networks, and what I found interesting is that you know you get explainability, battery explainability. It's not a universal model that has use cases across the board. It's not for language modeling or whatever. As far as I understand, it's for modeling physical phenomena, for understanding physical phenomena, solving mathematical equations that describe certain phenomena, and they offer potentially more so interpretability, which any scientist would want. I mean, you don't want black boxes, you want understanding as well.
Anders Arpteg:I think it's a super interesting field of research. It was some time that I read about it so my memory is not perfect about it, but, in short, it's just replacing this kind of super, super simplistic relus, this kind of function that is simply having a inflection point, so it's either zero or times one, so to speak with more advanced functions and thereby being able to reduce the number of parameters that you need to have. So you know, trying to understand and have an explainability for a model that has billions of parameters in it is not easy, but if you can instead have more advanced functions, then you can reduce the number and then you can start to see that, okay, I can sort of this part of the brain or the network is doing that, yeah, so you get more information somehow in each node, I guess.
Kjartan Thor Wikfeldt:I mean. So it's not just like a number between zero and one, it's something that you can more easily understand. You know which um was it.
Anders Arpteg:There's like splines and these kind of different lines connected with some kind of you know spline type of function or something.
Kjartan Thor Wikfeldt:Please don't ask about details. I'm beyond my comfort zone. Yeah, I think it's.
Henrik Göthberg:But it's cool, and Max Tegmark is working in MIT, right. What is his team? Do you know exactly what the main focus is of his team? I mean, we know what he has been talking about.
Kjartan Thor Wikfeldt:Yeah, I mean, he's a cosmologist, hardcore theoretical physicist turned AI expert, and I guess I haven't looked into it, but I guess he has a pretty big research group, probably half of which does physics and the other half does AI.
Anders Arpteg:I think one of the focuses that this group is having is this kind of mechanistic interoperability. So, it is the explainability part, as you say. So they spend a lot of time trying to see if they introspect the actual parameters of a huge network. Can they see what the different nodes or parameters and the weights in the model actually do? I think one example, if I remember correctly, was that they wanted to find is there a part of the huge large language model that has the city of Paris encoded in it? Large language?
Ingrid af Sandeberg:model that has the city of Paris encoded in it. It was an anthropic that published something about that and I think it was like May or something early May where they trained a model on like San Francisco or something and they honed in on a specific part of the model and then suddenly it will bring in San Francisco and everything. It's like, oh, what's a good recipe for a muffin? Yeah, you get this recipe, and it's best enjoyed viewing the Golden Gate Bridge.
Ingrid af Sandeberg:It gets obsessed with San Francisco, yeah because they place more weights on the San Francisco part of the model or, if it was, golden Gate Bridge, part of the model.
Kjartan Thor Wikfeldt:That's the sort of reverse engineering you have to do to understand a traditional model, right, yeah? And hopefully there are these other types of models, other architectures that offer more explainability, which might be important in some settings. Yeah, that's a cool one, isn't?
Anders Arpteg:it. Okay, I should say more about that. I think explainability is super interesting. I don't think you know mechanistic or this kind of introspection into parameters is the way forward, just as you wouldn't. If I were to understand Henrik, I wouldn't drill in his brain.
Ingrid af Sandeberg:I would try to find some other way. Sorry, elon is doing that, yeah, but not everything Elon is doing is right. You have a point there, all right, so let's go to the next. Again, there's a use case for every approach.
Henrik Göthberg:But let's go to the next topic. Ingrid, did you have a topic you wanted to bring up?
Ingrid af Sandeberg:Yeah, I think I mean on the topic of explainability, but, going more into language, I think it's worth mentioning the GPT-401 model, where it's basically got more reasoning capabilities.
Anders Arpteg:I misunderstood you previously. You mean Neuralink kind of work Elin was doing.
Ingrid af Sandeberg:What did you think I was doing?
Anders Arpteg:No, I thought you meant that Elin was doing actually AI network kind of introspection things.
Ingrid af Sandeberg:No, you were drilling into people's heads.
Kjartan Thor Wikfeldt:I was not drilling no-transcript.
Anders Arpteg:Yeah, but I still Did, you see the news story about that when they had the first human trial.
Ingrid af Sandeberg:Oh, yeah, yeah, but that was a couple of weeks ago, yeah. And it was going so well, though, like everyone, was surprised. And the funny thing is, it was like.
Henrik Göthberg:I thought I'd be so much more productive straight and he was playing.
Anders Arpteg:Okay, yeah, I think this is. Let's take that news, let's take it here. This is new. I mean, it's to what I recall you know, basically, you know, for some years now Neuralink has been building these ships. You actually embed them, operate them into the human brain and you have this kind of 1000 threads that you connect to neural cells in the brain neurons, yeah, cells in the brain neurons, neurons, yeah and uh and uh. Then they had now the first human trial, so a person that had, he, he was in some kind of accident and he couldn't move anything yeah, I think he was in a surfing accident and he was quadriplegic, which basically means that two arms and two legs can't work.
Anders Arpteg:Yeah, so he was like a vegetable and then it put the chip in the brain brain to keep it short and they connected it to a computer so he was like a vegetable. And then he put the sheep in his brain to keep it short and they connected it to a computer so he can control the keyboard and mouse or something.
Ingrid af Sandeberg:With his thought. So he's controlling the mouse pointer with his thought.
Anders Arpteg:And then he plays games to an extent that basically like a human expert or world level expert, because he doesn't need to use his hand anymore, he can control the hands and arms, yeah.
Ingrid af Sandeberg:And what they realized was that he was like but it's moving before I've thought it, Because normally, like if you want to move your hand, like there's a slight delay, but if you're just thinking it, then it was kind of before he understood that he made the thought.
Kjartan Thor Wikfeldt:I hadn't thought about that, but that's super cool, I mean, because you only become conscious of what you're actually about to do like half a second or 200 milliseconds or something, after you actually thought that happens in the brain.
Ingrid af Sandeberg:You send an order to lift your hand but the order takes some time.
Kjartan Thor Wikfeldt:But if you don't have that latency, and then your brain autocorrects, you know, makes it feel like a synchrony.
Anders Arpteg:They have to check. I guess in the future, if you have eSports, that no one has a chip in the brain that controls the mouse.
Kjartan Thor Wikfeldt:So what about this interface, though? Just because I'm curious, I mean there must be AI involved there to actually translate the signal.
Ingrid af Sandeberg:Yes, they have a network that's trained on that, and it's based on reinforcement learning, so basically, when he uses it, it becomes better and better and better at understanding his thoughts.
Henrik Göthberg:This was a couple of weeks back, right, yeah?
Ingrid af Sandeberg:But the point with the GPT-401, to go back to that, I think the point there was that because normally with the older version of ChatGPT you had to be quite good at prompt engineering to understand that um or to get really good response. And and what people discovered was that when you're prompting for a chain of thought or you're prompting for reasoning behind a reply, you get a response. But this one has that built in, and then I was following. So we have a quite active Slack on Stockholm AI and that's where I get a lot of like the really good drill downs from my friends who are reading a lot more into the weeds than I am.
Ingrid af Sandeberg:But we had a lot of debate on whether sort of this was kind of an agentic thing, that they're just like having agents prompted for chain of thoughts. But apparently it looks like that's not the case, because it's well, first of all it's not multimodal and also there are challenges to what you can do. You can't set parameters in the same sense and you can't drill down into it in the same way. So basically it's just reasoning itself, which is quite cool and that's also kind of democratizing because, like one pointed out, that very few users of ChatGPT know this hack about prompt engineering for one, but also getting this chain of thought thing to get better results. I can speak about this forever.
Anders Arpteg:I'm trying to clarify my mind, to speak about it briefly, but I think one way, at least I try to explain. I think the big news that I think this is this is one of the top AI news of the year, I would say.
Anders Arpteg:And the reason is if you take a thing like AlphaGo or AlphaZero, like in 2015, it could play chess, go, starcraft or whatever, and it does so better than any human ever could. And if you take AlphaZero, it did so without even looking at any human data at all. It generated its own data. And this is actually close connected to what we're seeing now with O1. So O1, it was previously connected to this Q star, and Q star can either be a Q learning kind of optimal policy kind of connection. But it can also be connected to this paper called STAR, self-told Reasoner, and it's also connected to this reflection tuning that we spoke about last week. But, in short, it's about generating reasoning and motivation for how to come up with an answer.
Anders Arpteg:So chain of thought prompting is one way of doing that, but it can also then say that okay, if you want to have this kind of answer, do the reasoning to get there and then you can check if it's correct. If it's not correct, it can actually fix itself and have reinforcement learning, saying this was actually right or wrong, and it can learn to do the right reasoning. And it can do the reasoning without any human giving it the reasoning. It's self-taught. This is actually a big thing. So, similar to AlphaZero, it can actually simulate forward how you should play chess, for example. In this case, it can actually simulate itself how we should do the reasoning to come up with an answer which limits the dependency to have human-labeled data. We're even having data at all. It can actually create their own data itself.
Kjartan Thor Wikfeldt:Do you think it could play chess at a high level? No, not yet. I mean, llms were tested for chess and it actually could make a few reasonable moves and you could put up the position on a board and it sort of made sense. But then craziness happened.
Anders Arpteg:But I tested it directly, of course. Then it came out, and I did, you know, one of the first. You know simple things. That GPT-4-0 couldn't do well is simply count the number of R's in the word strawberry.
Ingrid af Sandeberg:It's a famous example.
Anders Arpteg:And of course now it can do this correctly and all other LLMs have a hard time trying to answer is give me like 10 sentences each ending in the word fusion or something, and surprisingly they can't do it. And they can give some sentences, but at least a couple of them is always wrong. And you would say, as a human, it's super easy to do. You can just list the word and you can see directly. This is not ending with fusion. I see this is wrong. I tried it on 01 and it failed.
Anders Arpteg:And then, okay, I understand it's not at all the level of reasoning that of course humans have, nor does it have the type of reasoning that AlphaGo or AlphaZero had. It's increased reasoning, for sure, but it's not at all to the depth of reasoning that humans or these kind of AlphaGo kind of systems have, but it is a significant increase. So, in short, just as Demis Hassabis has said himself, what they are working with in Gemini now is to take the traditional type of LLMs that we have and connect it to AlphaGo. That's literally what their research is focusing on right now. They haven't done it yet. O1 is the first step towards that, but it's not that big a step, I would say.
Kjartan Thor Wikfeldt:It's like they call it O1,. I guess yes.
Henrik Göthberg:Yeah, but it's also interesting how they are quite clear now that this is complementary and they are putting out these big statements on. This is useful in these particular instances and types of use cases, but we recommend you to use 4.0 for this and this. And what were the examples that they highlighted where 0.1 was the preferred? More complex?
Anders Arpteg:tasks was the general data compute programming 0.1 doesn't have the capabilities that 4o has, and so it can't search on the web, which it's 4o can. It can't work with other type of modalities images, for example at all. So there is a lot of limitations that o1 has because it's the first version of something completely new. Yeah, not completely, but rather significantly different. I mean, in short, this would be gpt5 if they continue to do the proper kind of versioning on this, but they chose to completely disconnect it from the traditional kind of GPT-4 naming and now call it O1.
Kjartan Thor Wikfeldt:But wasn't there also an aspect, an idea here that it should be able to generate its own training data?
Anders Arpteg:now, yeah, but that's what it's doing, so the reason it generates is its own data, right?
Ingrid af Sandeberg:Yeah, but that's what it's doing.
Anders Arpteg:So it's generating the reason it generates its own data. That's what's so amazing. It's exactly like the AlphaZero it can actually generate its own data and train by itself to improve. So in this way, potentially in the future, it can continue to improve its reasoning skills without having the dependency of humans, which is possibly terrifying.
Kjartan Thor Wikfeldt:Yes, it is.
Anders Arpteg:And I saw some kind of IQ score measure. I think all of the others if you take the best one, I think Claude is in the top and 4.0 is in the top, but they are around 100. In IQ score kind of measure, 100 is the average of all humans and then it's like a bell curve from that. This one was 120.
Ingrid af Sandeberg:So significantly better than the average, but is that the right way of measuring it, though? I was listening to, harari made a speech I think it was just before summer at this Future Talent Summit here and he said that it's like measuring AI models in terms of human intelligence. It's kind of like measuring how well a plane imitates a bird or something like that.
Anders Arpteg:I use that metaphor as well. It's a great example.
Ingrid af Sandeberg:Yeah, I think it's quite and I'm not sure, but I kind of also feel that it doesn't have to be like a human to be very useful.
Henrik Göthberg:We don't have to measure it with IQ.
Ingrid af Sandeberg:I think this is spot on reflection yeah because I also feel like, you know, we sort of come up with a definition of intelligence air quotes based on what we know as humans, and then we said like, oh, we say chess, and then the AI can play chess. And then we then we're like, yeah, but it's not really as humans. And then it's like, oh, it's with, you know natural language, and then we we build models that can handle natural language, and then we realize that, but it's still not as general as humans. Um, so we kind of push the boundary because we don't really know what intelligence is. It's my feeling, or we don't really have a good definition that we can measure an AI based on, because I mean IQ tests.
Henrik Göthberg:I mean, it goes to humans, right. Who's the most intelligent, michael Jordan in basketball or the physicist?
Ingrid af Sandeberg:I think it goes back to specialization again.
Anders Arpteg:The IQ score is both for human and for AI to measure the score, but it still is an interesting measure, I mean if it truly is an. Agi or a super intelligent, it should be able to master that as well.
Anders Arpteg:It's a hygiene factor, so we know that even LLMs are significantly better in terms of knowledge than any human ever can be, and for AlphaZero it's insanely better than reasoning that any human ever can be. So it's easy to see measures or ways to try to measure intelligent knowledge, which is not what humans are simply about.
Henrik Göthberg:I really like that simple metaphor, you know, with the comparison of the plane to the bird. You know, I think it's telling right. Why are we trying to push the plane into being a bird?
Ingrid af Sandeberg:It flies well.
Henrik Göthberg:It flies as well right.
Anders Arpteg:I mean I think that's also what Jeffrey Hinton, you know, alluded to when he said, you know, said he invented the back propagation back in the 80s and he said he did that because he wants to understand how a human brain works. But he said I know it's an approximation, I know a human brain works differently and I know it's very limited, but at least it's some first approximation with a back propagation to try to train up some kind of neural network. But now, when he quit Google a couple of years back, he basically said I believe that backpropagation is potentially superior to the human brain because it can learn much faster than the human brain can. Just because it's different from the human brain doesn't mean it's worse. Actually, it can be that the backpropagation algorithm is actually better.
Ingrid af Sandeberg:For some use cases.
Anders Arpteg:We know the human brain is significantly more energy efficient, for sure.
Kjartan Thor Wikfeldt:But if you have a lot of energy. So back to learning how I mean we learn from very few examples.
Anders Arpteg:I mean, just take an LLM, you know, you basically can ask it any question about any topic. Yeah, it has much better recall.
Ingrid af Sandeberg:Yes, I mean we do learn from very few examples. We're kind of initiated, though, with sort of a gene pool that has been developed since the beginning of time. We sort of see that as a sort of Pre-training.
Henrik Göthberg:A long pre-training, a long pre-training phase, yeah, exactly.
Ingrid af Sandeberg:Spanning billions of years, I think it also is a little bit difficult to say. You know it's better or worse because also like the sensory input we get, yes, we have eyes and ears and nose and mouth, but we are also like our entire bodies has some kind of sensory hierarchy as well, like with the autoimmune system, for example. I don't feel, for example, my stomach normally, but if something is wrong I I will feel it. So there are sensors there as well. It's just that it's lower in the sort of attention hierarchy.
Ingrid af Sandeberg:So we get a lot of data.
Henrik Göthberg:And we got all these rabbit holes from the news of 01. But it's a big one. It's a big rabbit hole.
Anders Arpteg:That's a big news.
Henrik Göthberg:This is why it's like a news item that is so interesting that we can sort of flip around in many ways.
Anders Arpteg:I think it's also interesting that you release like two models out of three. The big, 01, is not released, 01 Preview is released and they have the small one, which I think is interesting and connected to what we just spoke about before the Mini. Yeah, the 01 Mini, which apparently is really good at coding so a bit more specialized also what we spoke about before but it's significantly smaller and cheaper to run. So I mean, I think you know they have extreme inference costs right now in open AI, so they need to have a way to run certain types of tasks much more efficiently and by having more specialized, smaller models, like a one mini, they will do so. So I think it really moves in this direction that we just spoke about.
Henrik Göthberg:Yeah, I think that's interesting how we it's a trend here, we can see it.
Kjartan Thor Wikfeldt:Full circle.
Henrik Göthberg:Full circle on our own analysis, but maybe do we have some more news, or should we end it with that?
Anders Arpteg:I think it's enough.
Henrik Göthberg:We went on a tangent with the old one, so I think we stopped with the news right now. Yeah, sounds good.
Anders Arpteg:Okay, and the time is flying away and we want to try to keep it to one and a half.
Ingrid af Sandeberg:We won't make it, but at least under two hours.
Anders Arpteg:But perhaps we can speak about the next steps. What's coming up for ENCCS and you've already spoken a bit about that for the AI factories, et cetera? But perhaps you can speak about the next steps. What's coming up for ENCCS and you've already spoken a?
Kjartan Thor Wikfeldt:bit about that for the AI factories etc. But can you elaborate a bit more on what's coming up? I mean our ambition is to grow to become more visible across the spectrum in Swedish industry, but also public sector, even academia. We are involved in consortia applying for grants different types of grants to expand sort of peripherally or in adjacent directions from our core activities in HPC and they have to do with AI, they have to do with quantum computing and so on. So we want to build a bigger group of experts who can help a bigger group of companies with more diverse use cases, and it's a very interesting environment to be in because it moves very fast. I mean things happen quickly. I guess you're very used to a dynamic environment in AI, but also in HPC. A lot of things is happening and we want to expand our training portfolio not machine learning training but human training.
Henrik Göthberg:Yeah, but, if I get it right, this sounds really smart. By the way, am I taking you right that you want to expand the ecosystem around yourself? I immediately get the neural pops like oh, that's how SAP is doing it in order to get ERP out there, they have millions of certified management consultants. So is that one of the parts? Now, in order to grow and become sort of an established player and get critical mass it's you need to grow, but you actually need to grow the ecosystem around you.
Kjartan Thor Wikfeldt:The ecosystem is growing without anything from us. We're a small player, but there are increasing investments and the national competence centers that we are one example of. It's just one out of multiple different beasts in this EuroHPC ecosystem. There is also centers of excellence that focus on different domains, so bioscience or material science or catastrophe prevention or modeling, stuff like that.
Anders Arpteg:So, these center of excellences, are they part of the EuroHPC?
Kjartan Thor Wikfeldt:Yeah, I mean, the phrase word center of excellence is probably used in many different contexts. But there are the EuroHPC COEs and we collaborate with them.
Anders Arpteg:Just to understand, if you compare a competence center to a center of excellence, what's the difference.
Kjartan Thor Wikfeldt:Each country should get a national competence center. So there's an ncc in 32 different countries. Yeah, but there are currently I don't know 10 or so currently active coes, but they are multinational, they have partners from multiple countries and they're focused around a certain area domain applied applied area yeah, so something.
Kjartan Thor Wikfeldt:Yeah, so something a domain where HPC is relevant and so COEs are larger than competence centers in general, and they collaborate across the border the're tied together in this ecosystem, and we actually have worked with one of our clients quote unquote again, they don't pay us, but we call them our clients. They came in with a use case to us and it was a very interesting use case. We could help them get access to HPC, but they also had a need for certain expertise, so we connected them to a CEV and they now have a beautiful POC going on which is all about actually improving air quality in Stockholm. Okay, so we work and thrive in this ecosystem. We want to grow in the ecosystem and the ecosystem itself is growing.
Henrik Göthberg:I was also getting a very concrete view of like like. We're talking about business development around this. So if you look at learning from software one of the keys you know critical strategies for a new vendor to get out there. Like you have a new app, you have a new software. You're growing the ecosystem of different types of partners, consultants doing different things, who can sort of connect from the customer into the actual, you know, using then hpc. So you basically end up to having a lot more touch points or sort of tangents, or you know so there's a lot of.
Henrik Göthberg:I get this whole vibe of you. Know you could do that. That could be an interesting area. When you say ecosystem also to you, know how you can attract other partners in order to do the sort of evangelizing for you.
Kjartan Thor Wikfeldt:Yeah. So two things to say. Maybe one is that you know we are offering this free access to HPC. It comes with some requirements, it has to be open in some sense, and so on, but it's free. So cloud providers might not like it, but in a way, we're not competing or we're creating a market where there isn't a market before. We're creating a market for scaling up, running bigger things and and your HPC might not be free forever. You know there might be a time when you start paying for access and but, but the goal is to um, by your HPC and the NCCs and so on, is to um help these companies create business models based on HPC and become more competitive as a result and, you know, be in a good position later when things might start costing money and so on. So is there?
Anders Arpteg:like an exit plan for ENCCS saying like will you continue to get money from EU or will you at some point need to have your own funding covered?
Kjartan Thor Wikfeldt:Yeah, so we're. One of our side focus areas is to look into ways to become economically sustainable in whatever way that could take. It's unclear, but that's certainly something on our radar. I mean there will be a EuroCC3 project, most likely Probably EuroCC4 after that. These are, of course, run as projects, everything is run as a project, but there is still the will to make something permanent here.
Henrik Göthberg:Because I think the bottom line is to create a market and to create it. Sometimes it needs to go from being, in quotation marks, superficial to sustainable, maybe.
Kjartan Thor Wikfeldt:I don't know. I wanted to add also one more thing, which is a joint NCC effort. I mean multiple NCCs across Europe are involved in working out the specs and requirements for sort of like a marketplace platform or a LinkedIn platform for HPC. Of course we have already LinkedIn. We could use LinkedIn, but to create a community around HPC where you exchange information, you post your open positions, you advertise your HPC services and so on. The marketplace thinking, yeah, very interesting, where everyone is invited, right. I mean we have the free academically supported projects. The CWIs mostly are academia and CCs are often hosted by universities too.
Henrik Göthberg:We're funded to help for free, but you could have commercial players coming in and they offer different types of services with different I mean, if you are talking about a gap between traditional companies who potentially could have a huge calculation need but they are not really tech savvy or mathematically savvy or HPC savvy, so of course that becomes an opportunity how we close that gap.
Kjartan Thor Wikfeldt:Yeah, and just maybe. Final thing about ecosystem. I mean we work in this level of the stack HPC. Not everyone is ready for HPC Absolutely not. In many cases, it's enough to buy a beefy laptop and run everything there. That's perfectly fine. In other cases, the challenge is to digitize, to move away from Excel spreadsheets into writing Python code or something. We're not working there, but there are other European projects and national initiatives as well to help with those aspects In our ecosystem. Once again, we have the European digital innovation hubs, testing and experimentation facilities and so on. So it's like a smorgasbord of different projects which somehow work together too.
Anders Arpteg:A problem I have a bit with all this EU funding here is it's so much focused more on innovation, r&d, and not on a sustainable business model. I mean, if you take, for example, large AI models today, yeah, it costs money to train them, but if you have a big company, it will cost much more to run them. The inference cost will be higher and this is not something that the ENCCS or other kind of EU funding today is trying to cover, and I think it's a bit problematic. And I think you know if companies should be feeling like a proper support, it's not just for the R&D part, it's really to build up the proper business that support them throughout. You know, do you have any thoughts about that?
Ingrid af Sandeberg:Yeah, you first that. Support them throughout, you know, do you have any thoughts about that? Or yeah, yeah, you first. Yeah, I agree, uh, but like we said, you know, hpc is one area and that is more of an r&d environment. So, by itself, like you wouldn't do a have a business that only does HPC, most likely, but also, I think, these programs. I want to view it as a little bit of a freemium model. If you start using a Euro HPC machine, you're not required to continue to pay for it, but I think an ambition with these EU projects should be to have SMEs or companies that have use for it understand the business case for using HPC so that you later on can also motivate actually paying for it.
Anders Arpteg:It could be an accelerator in that sense Exactly.
Ingrid af Sandeberg:And so, yeah, it's sort of supporting R&D, but it's supposed to be supporting SMEs, for whom their product requires some.
Anders Arpteg:R&D.
Ingrid af Sandeberg:If your product doesn't have like it's. When we're looking at which SMEs might have use for these resources, we will be looking at SMEs who have sort of data scientists or computer scientists or research scientists, which is kind of an indication of that they're actually doing some more scientific R&D. Because if you just have sort of data engineers and machine learning engineers, then they would normally be working more closer to the production environment and then it doesn't make sense to kind of try to break up stuff to put in an R&D environment, because that's not how those companies work.
Kjartan Thor Wikfeldt:I think I should mention just in this context, because it's so on topic. I mean there are the free HPC resources and so on. Via your HPC you can also apply for funding. There's a funding program called Fortisibo or FF. Ff Plus is the most recent sort of incarnation of this ongoing funding scheme where you can apply for funding and compute time and there are two different tiers. One is like for people that already use HPC to scale up and so on. The other one is called business experiments. So people, you know companies that are looking into changing their business model, adapting, expanding their business model, coming up with new models based on HPC, so you can get the funding actually to kickstart the journey into a new business model.
Anders Arpteg:We're, at the end, going to throw all the money into American cloud providers. It's a bit sad, I think that we don't have any more Swedish or European.
Henrik Göthberg:Yes, and on the most short note. Why don't we do a shameful plug? What are you doing next week? Don't you have a conference coming up?
Kjartan Thor Wikfeldt:indeed well, we had the conference in Copenhagen early September. That was great. We worked with our Nordic colleagues. We had actually we were in the venue of Dansk Industri in Copenhagen, beautiful venue, amazing audience. We had a really good program. We will run that again next year. We will probably actually have in the spring our own event in Stockholm too. So INCCS Industry Days event.
Henrik Göthberg:Basically to market and to reach out, yeah, and to evangelize on this opportunity yeah bring together our friends and also new potential contacts.
Kjartan Thor Wikfeldt:you know, to come together and talk about this rather niche field HPC it's not like an AI conference, but you know there are shared interests and things you can exchange and learn from each other about.
Henrik Göthberg:And I can imagine, as an entry point for someone who is a little bit curious and maybe even intrigued by this conversation. Could I use this? So you need to dip your toes sometimes.
Kjartan Thor Wikfeldt:But you mentioned next week. So what we have next week is a workshop, and we'll have it here at the Data Club, together with Hyperight and Stockholm AI, which is in here is Technikarton16.
Ingrid af Sandeberg:Ai, which is and here, is Tegner Gatan 16.
Henrik Göthberg:14, 14, tegner, Gatan 14.
Kjartan Thor Wikfeldt:And we also do this together with the PDC center at KTH. Oh, super cool. And what's fun about that is that when I worked at PDC, we had two clusters before the current one, which is called Dardell. One was called Beskov, the other one was Tegner Tegner, which is called Darnell.
Anders Arpteg:One was called Beskov, the other one was Tegnir.
Ingrid af Sandeberg:Tegnir, of course, full circle, full circle.
Kjartan Thor Wikfeldt:And no, but so we have an afternoon event where everyone is welcome. It's too late today to get an actual HPC account. If you would have registered a few days ago you could have gotten the account, but you can still show up and see hands-on demos.
Henrik Göthberg:So you will have a workshop educating who is the target audience. Can you be a newbie to HPC? Definitely yeah.
Kjartan Thor Wikfeldt:I mean, of course, we target people that potentially have a need for compute, so these will be data scientists or some such category, so people that already write code in some way and they might run in the cloud. But we want to show them how HPC works, demystify it, show it both in the classical way log in via terminal, ssh, linux module systems, learn we already talked about submitting jobs but also the modern way, using Colony OS. So you will actually demo Colony OS.
Henrik Göthberg:We will demo it yeah.
Ingrid af Sandeberg:Maybe we can put the link in the notes.
Henrik Göthberg:Hopefully, yeah, yeah, let's do that Great idea.
Anders Arpteg:I can't really drop the thoughts about how we can make the Swedish and European sector work better and, if I may be, so bold to give you a suggestion.
Ingrid af Sandeberg:Absolutely. Please do Be bold.
Anders Arpteg:Be bold. Yeah, this is actually not my idea. It's actually from another European country that did something similar.
Ingrid af Sandeberg:No, you're adding disclaimers. You're going to be bold.
Henrik Göthberg:I like you. I like you.
Anders Arpteg:Always careful with my statements.
Anders Arpteg:It's an occupational hazard it's an occupational hazard. Anyway, you say you want to help people or companies in Sweden and Europe or in Sweden in your case and you have this kind of R&D solution. Why not have think Eurovision for cloud compute in Sweden? And what I mean by this is that different providers companies, startups, smes are allowed to compete in a competition where they provide some initial solution, they get some funding from you to do so, and then you evaluate them in a different way and you select the winner and they potentially then get a contract that can help public sector SMEs in Sweden to get access to infrastructure in different ways Infrastructure not just for R&D purposes, but actually to run the proper product in the end as well. If you were to really push some startups to build this kind of common infrastructure I think that's something that we sorely need in Sweden and Europe and if you were to push these kind of initiatives to have at least some Swedish or European competitor to the big cloud providers, I think it would be such an amazing.
Kjartan Thor Wikfeldt:So we have everything there except for the production deployment environment. Right, we have the R&D part, we have monetary support, we have human support, we have human support, but the competition is for someone to actually be the production environment for a European cloud provider.
Henrik Göthberg:So imagine they're competing, they're coming up with ideas in order to then we really push and launch a commercial entity through a Eurovision context.
Anders Arpteg:It's a private company that provides an infrastructure service that then gets a contract, potentially for SMEs and public sector in Sweden or whoever is interested in it.
Henrik Göthberg:So there's a strong connection between the R&D here, with a very targeted goal of creating maybe one of your AI companies like this, that is more of a hyperscaler, which is maybe a little bit could be a different podcast.
Anders Arpteg:And just for your knowledge, there is another company that spent a huge amount of money on this and it's been running for three years and more and it seems to work. So I would really encourage you to look more into this and see if we can do something similar.
Kjartan Thor Wikfeldt:Can you mention the name of the company?
Anders Arpteg:Afterwards perhaps more into this and see if we can do something similar.
Kjartan Thor Wikfeldt:Can we?
Anders Arpteg:mention the name of the company Afterwards perhaps, but I think it could be amazing, because you have to have a sustainable solution. It can't be this kind of ephemeral kind of you know we have ENCC. That's supported, yes, but not long term. If it actually builds up another one that is sustainable and have a business model, it can have such a big impact. I think.
Henrik Göthberg:Anyway, I'll stop there. Yeah, you can dream. I think it's more than a dream. Should we go to some last more philosophical topics? And I don't know a way to connect it. Do you want to have a go?
Anders Arpteg:Yeah, Well, okay, sure, I'm thinking 01 and thinking that we are starting to see AI models now not only having huge amount of knowledge, as the large language model does or the large multimodal models are having, like Gemini et cetera, but they also get some reasoning skills now and perhaps they soon also get some more agentic skills where they can, which already GPT-4O can do. I mean, they can do web searches, they can call out different APIs, tools like Wolfram Alpha and so many more things. So they already are starting to become more powerful, and some people are thinking that it will be a point where we have something called AGI. Do you have a preferred definition of AGI, Ingrid, if you were to start?
Ingrid af Sandeberg:No, I don't. I always ask what the definition is.
Kjartan Thor Wikfeldt:It's going to be a moving target maybe.
Henrik Göthberg:Yeah, I think your definition is that this is a moving target. That was your conclusion.
Ingrid af Sandeberg:Yeah, it's a moving target and I think it's probably going to be very incremental. Hopefully we're also going to develop our own intelligence and how we complement AI.
Anders Arpteg:Yeah, I think that's the best scenario where we actually have AI that complements humans. But I think Sam Altman's version is rather good. He basically says that when AI systems become as good as an average coworker, then you have AGI.
Ingrid af Sandeberg:I think it's rather good. I think was it OpenAI or somebody else who recently posted like a definition or like a five levels, the five levels.
Anders Arpteg:That's a Google paper, and OpenAI has done it as well.
Henrik Göthberg:Okay, they have done it as well. I only know the Google one, yeah.
Anders Arpteg:In OpenAI one they have, the top one is basically organizational one, and then they have PhD level and they have great these kinds of levels.
Kjartan Thor Wikfeldt:So this is the down-to-earth definition maybe of agi, you know, incrementally start, you know, going from where we are right now and ultimately we'll have something that's indistinguishable, it has iq, you know whatever, and it can do basically everything. I haven't seen this just make mistakes.
Ingrid af Sandeberg:Yeah, but we're still comparing it to different levels of human type intelligence.
Anders Arpteg:Well, the organizational level is potentially not human. It's potentially superhuman.
Anders Arpteg:But it doesn't really matter. We're getting a bit off the topic here, but you can look more into that if you want. But you can define AGI in different ways and people are going to disagree with it, as usual, but you can understand that at some point, potentially, they will have an AGI level kind of system and perhaps even super intelligent, meaning literally better than all humans combined in terms of knowledge and abilities and reasoning skills. What do you think will happen then? If you saw? You know, nick Bostrom is a person who has written many books on AI and he recently released a new book which is not as skeptical as the previous one.
Anders Arpteg:This one was called Deep Utopia and he basically says what if we come to an AGI future where everything goes well and we have utopia? So my question to you basically is, in a very philosophical and speculating kind of fashion, where do you think we will end up in a spectrum, either this kind of dystopian future where machines will kill all humans, like the Terminator and the Matrix and so forth, or the utopian future that Nick is speaking about, where potentially and also Elon Musk is speaking a lot about the world of abundance, the world where the cost of goods and services goes towards zero and you don't really have to work unless you really want to. You can survive and do whatever you want. But if you want to have some more luxurious kind of things, you probably have to do some work. But it will be a world where you do not have to work, at least.
Anders Arpteg:And perhaps a utopian future. Tor, do you want to start? We're in this spectrum.
Kjartan Thor Wikfeldt:Well, I think it's fun to think about also more science fiction-like scenarios, because personally I don't see any reason for why it could not happen that, when we reach a certain level of complexity which is beyond what is currently done, somehow interconnected neural networks, specialized neural networks on different things that are interconnected, they have certain agency we're talking about adgentic and so on why could it not happen that there is a runaway process in the Kurtzweilian sense, you know, with a singularity, like there's an intelligence explosion and what?
Kjartan Thor Wikfeldt:I mean? That's fascinating to think about and also very scary, and what that would lead to it's completely unknown, I think, because a super, hyper, extremely intelligent agent which is omnipotent as well, has all the resources, has robotic embodiment and so on. I mean we have no way of knowing what that would lead to. It could be utopia, it could be dystopia, it could for some reason come up with a goal that colonizing the galaxy is the best way to proceed, you know, and then humans become completely irrelevant in that context, or it could be like the unheard of flourishing of humans. So I don't know which way to lean there.
Kjartan Thor Wikfeldt:Above or below 50%, utopian let's say 50-50, exactly, but that's the I don't know more science fiction like way to look at it. I mean, the other way is just incrementally. I mean, maybe we will have AGI in a couple of years when we just eliminate the bugs, we eliminate the hallucinations and gradually it becomes, you know, an AGI. You know model that can be the CEO of a company, it can be a scientist. Be the CEO of a company, it can be a scientist, you know. And to me, the utopian future there is that, everything you know, science will progress as never before because everyone can be a citizen scientist. You have the perfect scientist assistant and everything will be, you know, open source shared between humans and so on. Everyone can be a programmer perhaps as well.
Kjartan Thor Wikfeldt:Everyone can be a programmer, there will be no need to be a programmer. I mean they will do the programming. But I mean, just given the current state of the world, it's a little bit difficult to see that this is the most likely outcome. You know, when all the power and the resources are in the hands of a few companies and there's geopolitical terrible situations in the hands of a few companies, and there's geopolitical terrible situations in the world, there's war, there are aggressions and humans are not behaving in a very ideal way. So I'm also rather ambivalent on which scenario is more likely, in that more sort of down to earth way, to speculate about the future.
Henrik Göthberg:I like the way you were painting the pictures here. Of course it's impossible to answer, but also for you it's even sometimes like damn it. On a Monday I feel more 40% and on a Friday I feel more 60%.
Kjartan Thor Wikfeldt:It depends on whom I'm talking to. Like, I have friends who are very doomsday you know age doomsday prediction um, guys and um. Then you go the opposite. I tend to sort of push against whoever I'm talking to. So I like that.
Anders Arpteg:That's annoying to the other person. Cool, ingrid, what's your thinking? Will we end up in a dystopia or a utopia?
Ingrid af Sandeberg:Yeah, I mean you're right in the sense that if everyone can become a citizen scientist, everyone can also become quite a fusion terrorist, so I mean there are pros and cons of everything and AI is a tool that can be used for good and bad.
Ingrid af Sandeberg:I mean I don't see a sort of physical law that says, given endless time and compute and experimentation, that we would not be able to end up with some kind of super intelligence.
Ingrid af Sandeberg:But I don't know whether what we're doing now is incrementally going towards that direction, because everything we're doing now is kind of trained on data, either language data or sensory data, and it's a little bit going back. I'm reading this book about sort of the origins of quantum physics, actually called what is real. And then there's this discourse in the beginning of the 1900s about like, is science what we can see or the theories about what we can see? But regardless of which, we are training the models on stuff. We feed it, but we don't feed everything. So what I say will never 100% represent what I think. But we are humans so we kind of function a little bit similarly so we can infer that difference between what I can express and what I actually mean. But computers don't really work that well so they become quite literal. So I'm not entirely sure that these sort of LLMs or sensor-based models will at some point what's?
Anders Arpteg:lacking.
Ingrid af Sandeberg:I don't know I mean, but so I don't claim to have the answer. I'm just not sure we're going in that direction, but I think at some point there's no reason where we wouldn't end up with a super intelligence or agi. And then going to a question about do I think it's going to be a great future or a horrible one, or perhaps no future at all? So I have a friend who uses a great term called purposeful delusion, basically meaning that whatever I think about this is not going to impact the outcome a lot, but I'm going to feel a lot better thinking it's going to be a utopia. And then I might also think about what kind of utopia might I want to work towards. So I tend to lean positively but on the other hand, like when you're talking business, for example, it's normally much easier to sell pain than to sell gain. So you might have to also think a little bit about the utopia for people to actually like, feel a sense of urgency to make sure that we don't end there. But I tend to be an optimist.
Anders Arpteg:Nice, awesome, cool Time has flown away and, as super interesting to have you both here, ingrid and Tor, I hope you can stay on for further off the camera kind of discussion speaking about quantum physics is one I would love to speak more about.
Kjartan Thor Wikfeldt:We didn't even talk about quantum computing, but it's one of my favorite topics. We didn't even talk about quantum computing. It's one of my favorite topics.
Henrik Göthberg:Awesome, we have to do it off camera.
Ingrid af Sandeberg:Turn off the camera.
Henrik Göthberg:From.
Kjartan Thor Wikfeldt:HPC to quantum computing, if anyone is interested in quantum computing talk to us as well. We do quantum computing also.
Anders Arpteg:I think you should be glad you didn't speak to me. Awesome. Thank you so much for coming to this podcast. Ingrid and Tor, Thank you very much. Thank you so much for coming to this podcast.
Kjartan Thor Wikfeldt:Ingrid and Tor, thank you very much. Thank you so much, it's been great.