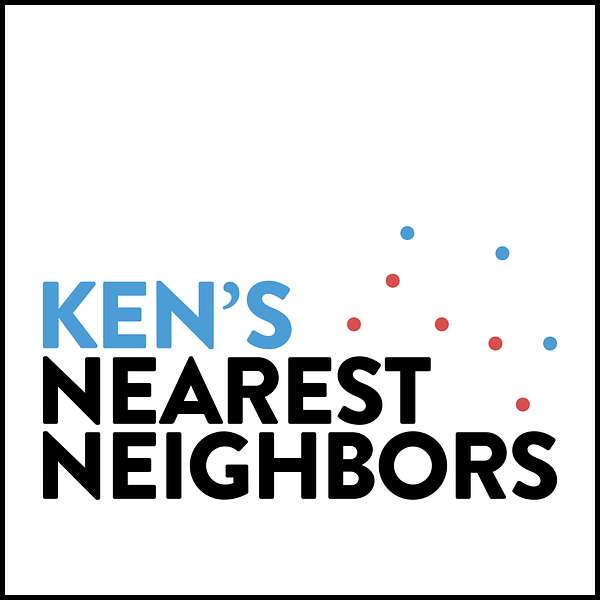
Ken's Nearest Neighbors
The Ken's Nearest Neighbors Podcast is about telling the unique stories of the people in the data and AI space. In highly technical fields, we often lose sight of the individuals who are making the positive change. The podcast focuses on who these people are and how they have approached the big decisions in their life that shaped their world view and career. While the podcast is centered on a technical domain, the conversations often touch on personal philosophy, the pursuit of meaning, and how we define success in our career and lives.
Ken's Nearest Neighbors
Why He's Completed over 25 Online Certificates (Jack Raifer Baruch) - KNN Ep. 77
Jack Raifer Baruch is a Behavioral Scientist turned Data Scientist. A believer in lifelong learning, entrepreneur and passionate about using Data Science to improve socio-emotional development for individuals and organizations. Currently he heads the Data team at Ada Intelligence. In this episode we talk about the intersection of human behavior and data, why Jack loves online learning and certificates, and the wild possibilities for data science if we remove profit from the equation.
They have the sense that, you know, you're not a machine. You can't be at a hundred percent all the time. It's impossible. You're going to be at 95. And when you reach a certain point, you need a break. You need to stop and you need to start over tomorrow. This episode of Ken's Nearest Neighbors is powered by Z by HP. HP's high compute, workstation-grade line of products and solutions. Today, I had the pleasure of interviewing Jack Raifer Baruch. You might have seen Jack on my 66 Days Of Data livestream just a little bit ago. Jack is a Behavioral Scientist, turned Data Scientist, a believer in lifelong learning and entrepreneur and passionate about using data science to improve socio-emotional development for individuals and organizations. Currently he heads the data team at ADA Intelligence. In this episode, we talk about the intersection of human behavior and data. Why Jack loves online learning and certificates and the wild possibilities for data science if we remove profit from the equation, I hope you enjoyed the episode. I know I enjoyed talking to Jack. Jack, thank you so much for coming on the Ken's Nearest Neighbors Podcast today. Obviously, we have talked a lot. You're on my live stream a couple weeks ago, and you're also one of the few people who has done the 66 Days Of Data challenge updated challenge as many times, actually more times than I have, which is incredible. So thank you again for coming on the. Nah, thank you for having me. it's really a pleasure. You've been a big inspiration, especially on me, you know, learning about getting, on the hard side of the skills that I needed to develop, even though I've been interested in data science for quite a while. And it's helped me develop that develop by skill a lot faster and be become part of the community. And that's all thanks to your 66 Data initiative. So big fan. Excellent. Well, it's always nice to hear, hear good things about the initiative. And it's also like really inspiring to see like how much you've grown or how much, you know, how the journey you've gone through by following, along with your 66 days. To me, that's a really cool and fun thing as well. Something I would like to touch on before we go into your learning journey is a bit about where you first got interested in data to begin with. Like where did that fire get lit? Well, I've liked data for a long time. Definitely not when I was studying psychology originally, my original psychology journey was all in psychotherapy. So you know, you don't get a lot into data there and all the district you get is limited. Then about a little bit, over 10 years ago, I started getting into behavioral economics and now we start seeing this huge area where, where data is very, very important. So I started getting into data and into data right there. And. it's been just something that I wanted to explore more and I got the chance to start doing it a few years back and I just started doing it by myself and started learning. Hey, you can actually do really interesting things with this and then started looking at the actual data science tools and data science algorithms and what you can do with programming. And the more you dig into the rabbit hole, the deep period goes, even though there is so much to learn. So, so much every single day, there's something new. Sometimes you feel like if you want to learn everything, you're just like, keeping your head above water just a little bit. But at the same time, every time I learn something new, I want to think of a project. How can I use this? Even though about 90% of the time, I'm like, No, no, I don't have the data for that right now. This is not my area. And specifically, since I do a lot of work with human data, with people, analytics, with biostatistics, the chance of getting into the more complex algorithms sometimes it's not there. I try to get very creative, but those are like my site projects. So they take forever and if something comes out of them, I will let everybody know and be very happy about it. but it's going to take time on the other hand. But the more tools I find, the more interesting things I find, and it's becoming easier and easier, not just because of the skills, but because of the tools that are being developed to do very, very interesting things with, with data science and with data in general. I love that. Something that you brought up, which I think is kind of funny and relatable to my past is interest in behavioral economics. So my first love was psychology. It was my first major in college. I took a research methods and statistics course, and I did not do very well on it. I had to, I think I've told this story before, but I had to a rat and we put him in this, the Skinner box. And then I named the rat and, you know, we trained it and did all this stuff. And then at the end of the semester, I was like, Hey, what happened to the, what happened to my rat that I became such good friends with? And that kind of put a ... on the psychology career for me. But after that, I eventually settled on economics. And I remember reading the book "Predictably irrational". And essentially, as you know, it talks about how like our conventional idea of economics is that people always act rationally and people don't do that, right. You, for example, raise the price on a product. Sometimes people buy more of it because it's perceived differently as perceived like a, like a more quality product or something along those lines. And the way that we understand irrational behavior is not through necessarily pure math. It is through data. It is through estimation. It is through building models and predictability and running AB tests and doing those types of things. And that opened up my world that, Hey, this is so much bigger than just pure economics and me putting a supply and demand line on paper. Like there's so many other dynamics at play. How do we control from for those. And in my mind, the answer is statistics, machine learning and those types of things. And I wonder, did you have the, a similar experience where you're like, Okay, psychology is limited by X, Y, Z, and some of this other data can help us go in the other direction? Well, there's a lot of things happening in the same time. First of all, when are in high school, I always thought I was gonna get into engineering. My idea, you know, I was very much into inspection and I thought I was gonna study something like bioengineering and work on the first artificial human heart. You know, we ..., and all these others really got into my head. Then when I got to college, because of everything that was going on in my life, I started studying psychology. And, you know, I probably made the mistake that many psychology students actually make. It's you get into psychology to understand yourself and it doesn't work. If you want to understand yourself, go to therapy, don't study psychology. It makes, it just makes it a lot worse. And you know, many years later then I started getting into behavioral economics and actually loving the idea. I actually, my first Coursera course ever. And that's why I love the platform was with Dan Ariely and well, everything about his book, "Predictably Irrational", I ended up reading all his books, then getting reading all the books by ... Richard Tyler and ... and just starting getting into the whole area. And again, yes, we need to build models that actually reflect reality a lot closer than what we think, because we're very, we're always going to be biased. We're gonna be biased because it's very comfortable to be biased. And when you see the data and when you look at the models that we can build, you start noticing, Hey, we are actually really bad at making good decisions. Which is funny how we run the world, huh. So, go ahead. Yeah. There's an interesting example which I actually just forgot. So I'll tell you later. Sounds good. I'm when it comes back, I'm equally anticipating it. So for, obviously, we talked about behavioral economics and psychology. Can you go a little bit more in detail about how you made that transition how you learned a lot of these things? Was it, you know, you mentioned projects, you mentioned certificates and also how you made the actual, not just learning acquisition of skills, but the career transition as well. Well, to start with, I started switching into behavioral economics and I've always been a very firm believer in entrepreneurship. So I actually, what I did is actually start a business that mixed. Trying to teach people about, you know, behavioral economics and working with organizations with psychology. I went a lot into the human resources part of it and how you can implement those, these things into companies, especially working with corporate culture, you know, it's the big buzzword in many companies, culture, culture, culture. So I started asking what is culture? And they started saying, well, it's the values and the this and the that. Okay. But how can you get data out of that? How do you know if whatever you're doing is actually working? So. I started me and my wife we're partners in practically everything we do nowadays. So we started building on this idea of how, how do we deal with culture? And we ended up putting together a whole concept and and a whole model of how to actually do this and using data and started mixing things like objective key results and psychological safety and all these concepts, and actually putting them together in a way that we can teach people how to actually build corporate culture that goes beyond. Let's do you know a $20,000 seminar over the weekend with the higher ups. So they can come up with six new values that nobody's gonna care about in six months, which is generally what happens. And from there we started building more projects. I've always been into statistical psychometrics, you know, the ocean model, the HEXACO model, and. We started thinking, Hey, maybe we can adapt this to actually measure things in companies. And that just made things click. And I started looking into, Okay, how can you use this? How can you build models? And I started seeing the data science more often and here and there. And of course, since since high school, I went to get into technology and suddenly a you should, how to program, you should learn how to code. You should learn how to you know, SQL, which was something that I've heard about. You know, I knew it had something to do with databases, but never actually got in my hands on it. And for me, coding was what I did back in 1986, I think, with a Texas instrument and basic, I don't know if you remember that code that you went 10, do this 20, do that 30, go back to 10. And I hate to tell you this, but that was before I was born. Well, imagine now, and this is the other part, having someone like my wife to push me and saying, you know, it's never too late to start over. If you know, you never really do start over. Whatever you learned before is actually valuable. But if you want to start a new career, especially nowadays, which is becoming more and more common, you know, whatever you learn in college is probably gonna be fairly useless five to 10 years down the line, if you're lucky. So it's gonna be something constant that you have to keep learning and just switching careers and just following your passion. And the other part that drove me into data science was looking at what was happening in the world. You know what happened with companies like Cambridge Analytica, you know, using these algorithms, this wonderful technology called machine learning instead of helping people to hurt people, because it's convenient for someone up the line or somewhere in the world. So I said, why isn't this being used, like openly to build tools that are gonna help people be better? Not be worse or just make profit because I'm all for make profit making profit, but it should be something sustainable through time and not just something, you know, how much money can I make right now. And then forget about whatever damage you're making. So all those things just drove me to start learning a little bit about, a little bit more about data science. And I was lucky enough that the tools were already there. I mean, if I wanted to study data science back in two, five, I would've had to go to the university and figure out, you know, if somebody in the university down here had any idea what data science was and could take a few courses about this and that, but I was lucky enough to be that there was a time where there were all these wonderful platforms from Coursera, edX, Harvard Online, 365 Data, Data West. I mean, there's so many possibilities nowadays and you just start mix and matching whatever works for you and learn the stuff that you actually need to learn and put it into practice. You know, so for those who are listening, if you go on Jack's LinkedIn profile, he's taken more online courses than anyone I've ever seen. And we're talking like at least 50, which blows my mind. But you know, it's also a really incredible sort of like paradigm shift that you get from experience, right? Is that, Hey, you know, historically I would've had to pay a lot more money. I would've go into a formal setting. I would've had to do all these things to learn this information. Now I can go online and I can get these things either for free or relatively cheap. I can do it on my own time. If you're viewing education in that way, where it's like, I wanna learn this. And compared to before, it's easier than ever to learn this. The, you know, like the hardest thing for me to do is I just have to choose what to do when I get started. That makes a lot of the anticipation or fear of starting these things go away because you're thinking about, Oh, compared to how it used to be with this old frame of reference that I have, like, this is incredible. This is an open territory. This is so easy. And I think, you know, I think now we have YouTube, we have all these free resources. People don't realize how difficult that it used to be. And like baseline starts to be really difficult, right. And you know, it's not a knock on those people. It's just what they've known. But also, you know, thinking about it from a perspective of like, Hey, you know, this is a, it's very cheap to learn things now, right. Like the fact I'm so grateful for that. If I want to go and learn a concept, I can like just Google it. And I could probably find something redefined. Like we live in a time where like the fact that we're able to do that and consume that in that quick iteration loop is like nothing. We've ever experienced in the world before. I mean, people, if you, if you brought someone from like the 15 hundreds in, right. And they're like, Oh actually, yeah. In order to get a copy of this book, I have to copy the entire thing because we don't have a printing press, you know, they'd be like, Oh, I can press Control C, Control V, and do that. Like, like they'd be doing, they'd be going nuts, right. Plus you're forgetting the equivalent to about $2,000 worth of paper. Back 500 years ago. Exactly. And so, I mean, to me, you know, if you're someone who who's struggling to start learning this field, struggling to like, learn that for certificate, whatever it is, just think about how much harder it would be if you had to do it through university, right. Or you had to go and like find all the resources cause they weren't consolidated for you. And of course, to begin with, I mean, like when you give yourself those frames of reference, when I like. And thinking about learning a new skill, right? Let's say I wanna like learn and hit a golf ball or whatever it is. Like I remember when I was starting and practicing and learning and we didn't have all the ball tracking technology. Right. We didn't have any of this stuff. We didn't have the live feedback. Nothing. I am so grateful for how easy it is to improve now because I can, I can go, I can see all this stuff that, you know, it, it makes me rethink how I was doing things before. And it makes me again, like more likely to do, to like indulge in tracking this stuff now. Just because that period entry is easier. So I don't know why I went so far down on that tangent. Yeah. But I just think it's like very inspirational and I love seeing people just get so immersed in learning and loving that we have the accessibility to it. But still think, think about it when I was in high school. And even in college, if you wanted to know something, you had to go to the library, look it up, look to see if there was a book about it. I got into the internet very, very quickly, as soon as it was sort of available, you know, with the screening ban sheet technology just to connect on a, on a very slow server and take like forever to download whatever it is that you wanted to download. And even at the beginning of the internet, whatever you found was was, was hard to get nowadays to give you an idean idea which Belia is a lot easier, faster, and more reliable than the Encyclopedia Brittanica ever was, or any of the encyclopedias. And it's a lot faster, you know, now, for example, I remember trying to figure out how to do something very simple back in, I don't know in dos or Windows 95 on whatever you wanted to look through, you had to go through a thick book and look for the specific page to look for a tiny snippet of code. And nowadays, you know, you're going to stack overflow and you have the code right there for practically anything you want to do, or at least a good example of the code. So now it's a lot easier to get into it, but that also means having the tools also means that there's a lot more expected from you. And, you know, we can get into the whole issue with the companies when they're hiring data scientists, expecting you to know every single time, every single snippet of code by heart, which is never going to happen, especially in that nowadays. I'll just give you an idea, how many phone numbers do you know by heart. Maybe four, you know. Yeah. That that's probably most, that's probably most, most than more people that more than most people. And what happens is by the time you have this, why would you actually learn everything by heart? I mean, you can use your brain on many other more important things. And that's what the internet gives you all this knowledge that now you don't have to keep in your head and you can keep in your head, the stuff that's actually important, the higher level stuff. Yeah. I love the, I love that idea of the extension of yourself. You know, like our brain is as big and as useful as the systems we create around it, like, you know, I tell people about notion all the time. I love notion, but it's super searchable. It's an extension of my brain, right? It's like, Hey, I put all my thoughts. I don't have to think about those things. Again, I can just like use my brain, that I, my extended brain that I've created, that I've organized and structured and I can fish back up those thoughts on whatever book it was I read or whatever thing it was. You know, that actually does touch on an interesting topic that you alluded to before. And it's about how we use machine learning, AI, these types of things for good, you know, I can use technology to expand my brain in that sense and make it more efficient, but I can also sit here scrolling Instagram and effectively use technology, these algorithms to eliminate any progress or semblance of self-control that I had. Like, how do we, you know, how is it up to the individual to use those algorithms for good? Or is it up to the companies or, you know, how do we frame that landscape. Well, that's a really, really, really deep and complex question. First of all, because it has to do with, with what's re position on determinism, you know do, do we actually have agency, do we have AC do we actually have free wheel or not? And that goes into the philosophical. I believe that the university is deterministic, meaning that everything has a specific cost, even if we don't know what that causes. And we are, although we do have some agency on what we do, we are very much shaped by our environment. So if you're environment is constantly bombarding you with by this, by that your life is miserable. If you don't have this it's going to push you in that direction. And so there is something to say about our environment and how we should shape our environment to help people be. And you know, I don't know if you, if you're a fan of of, south park and one of their seasons, they went in this whole route about online ads and how they're controlling the world. They they're very close to the mark. They, they are at least shaping a big power of our culture. So we can use this technology for evil or for, you know, very specific purposes that are just gonna be good for very few people. Or we can use them to make society a lot better. Like for example I remember. When you're talking about good design there's this game world of Warcraft, you've probably heard of it. It's fairly famous and they actually have this thing where if you log out AF and after two hours, you log back in, you had this benefit and your character had better stats for a, for a few, for a couple of hours. So in the design, there was this thing of getting out and taking breaks and that's good design for people. So we can do the same when it comes to social media and we could start building things that are good for people to improve themselves. There's a lot of apps today for meditation, for learning. And they're just starting to learn how to push people. Like, for example, right now I'm doing lingo because, you know, I'm moving to a new country, so I'm supposed to learn a new language and the little notes and the reminders, and they're getting better and better and doing the sign to make it fun, to make it interesting. And to push you to learn what you want to learn a lot in a more efficient manner. So you don't skip a day, so you actually keep doing it over and over. But if you do skip a day, it's not that big of a deal. So yeah, we, we can design systems that are going to improve your life. So instead of just having ads, telling you by this, by that, and keep pushing you to having absolutely no restraint over your spending you can have the same kinds of things to help you you know, save money to help you not spend on stuff that you don't need. Like for example, what happens if this is probably never gonna happen, but what would happen if every time you buy something new on Amazon, it would tell you know, what are you, it would ask you, what are you going to use this. Give you that tiny pause before you click on purchase something that it's literally going to sit on, whatever route you use in your house as a storage and not move from there ever. And if you use it one time, you're probably ahead of the curve. Yeah, I mean, honestly, you could probably create a Chrome plugin for something like that. Amazon I'm sure would hate it, but it's an interesting concept is how do you I find this, this like the dichotomy between, does the technology work for me or do I work for the technology? And I think if you don't know the answer to that question, then you probably work for the technology, you know, you sit there like doom scrolling on Instagram or Twitter. I mean, my biggest concern is not even financial in spending and advertising. It's the amount that I'm encouraged to be on these devices. Like even the Duolingo example, right? Like, I think that's great. you're trying to learn a new language, Duolingo wants you to stay consistent, but when you're staying consistent, you're also staying on that platform as much as possible, right. They want you to maximize your time spent there. And it's this very interesting thing is that I think, you know, social media YouTube, any of these things, they're incredibly powerful in positive ways. Whether it's like learning to convey information. I mean, learning to pick up information, new skills, whatever it might be. But I also like go down these rabbit holes where I've watched like 15 YouTube videos on like folding paper or something weird. And I have no control. I feel like I have no control over it. And at the times when I do set up the infrastructure where I'm like mostly producing on YouTube, or I have like very specifically curated like Twitter feeds and things like that I feel like I can use. Those technologies for good, even though for the majority of people, they're, I think they're kind of detrimental in terms of like serving up just stuff. People will click on and continue to go down. But I also have this weird ethical thing where it's like, I want people to watch my videos. I want people to click on my content. You know, I am a bit of a slave to this algorithm that's out there and, you know, like the trade off between. Okay. Like what I think I'm producing is I think I'm producing things that are useful and valuable, but I also am like enabling this algorithm and feeding it and doing those types of things. So it's you know, a weird gray area as well for me. The whole ethics thing on when it comes to machine learning and artificial inte. It's such a huge issue. and we are just scraping the top of it. But at the same time, if you think about it, what the question is as you know, when we teach a machine learning algorithm, we are the ones that are telling it what's important. What is the goal? And it's going to be incredibly good at reaching that goal. And if you go into re re reinforcement learning, it's even bigger, but still you set the goal. And what happens if we just change the goal instead of how many hours do you spend watching YouTube videos? You change it to, you know, how satisfied are you with the videos that you're watching or how much did you improve on such and such. If you're trying to learn something, if you move the goal posts, then you know, those machine learning algorithms are gonna become better at something that's much more humane and a lot less trying to, you know, just keep your eyes glued to whatever screen you're you're using at a particular point in time. And, but that's the challenge because at the same time you have to be able to produce, you know, whatever efforts you're putting into have to be profitable one way or another. And yeah, since, since the advent of Google online marketing, you know, is where a big chunk of the profit comes from comes from to, for many, many companies because that those advertisings are the ones that are transforming into sales one way or another. This episode of Ken's Nearest Neighbors is brought to you by Z by HP. HP's high compute, workstation-grade line of products and solution. Z is specifically made for high performance data science solutions. And I personally use the ZBook Studio and the Z4 Workstation. I really love that the Z line can come standard with Linux, and they also can be configured with the data science software stack. With the software stack, you can get right into the work of doing data science on day 1 without the overhead of having to completely reconfigure your new machine. Now back to our show. Yeah. I mean, that's so true. Yeah. I think something you kind of brought up there with the advertising or with the usership is injecting intention. So it's like, what are you trying to get? And the purchasing stuff, right. Is what are you trying to get out of this? What are you trying to get out of this video? What are you trying to get out of this purchase? What are you trying to get out of this service or interaction? And, you know, some responsibility could be to. To put that on the companies to like inject that in probably more practical thing is for us all to ask what our intention is when we're doing any of these activities. And sometimes just when you're aware of something, like when I'm aware, I like, you know, I'll be scrolling through Instagram and I'm like, really I'm doing this again. Like, what am I, what is the benefit of this? Like, , you know, am I gonna see something that's gonna change my life? Probably not. And like just the awareness is something that snaps you back to reality and brings that consciousness around how bad or how good it can be which can be a little, a little scary and humbling at the same time. It's you know, at the end of the day, it's, if you start reading and I think machine learning and all deep, especially deep learning proves it very. If you read ... didn't talk that much about robot center. Artificial intelligence probably... is the biggest culprit here. It's the thing. Once you have an artificial inte artificial intelligence, and we're talking about general artificial intelligence there's no limit to how much it can learn. You know, you and I have a limit, you know, our brain cannot grow infinitely, you know, we can learn more stuff, but as we learn more stuff and create more neural pathways, the ones that we stop using start getting scraped so we can build better new ones. But once you're an artificial being. What's your limit, you know, it depends on how much memory you give it. The more resources you give it. It just keeps growing exponentially and infinitely. That's why this works for us. Like for example, initially to store, Oh, initially to store phone numbers. If it, if there you go, initially to store phone numbers, and then you can forget about remembering any phone number at all. Cuz it's just there. It's just a click away. Now your brain has more room for other stuff, but I don't know if it's ever gonna get to the point where we're like cyberpunk and we can just keep adding memory to our brains. Or once we build this artificial intelligence, you know, it doesn't have a limit. And I think that's the one thing that really scares us. If it doesn't have a limit, if it, is it gonna be good for us or is it gonna be bad for us? Because at the end of the day, it's just gonna run whatever programming we give it. The same thing happens with machine learning. If we tell it, you know, all I want is how much you can how, how much of Jack's eyes time can get, be glued to YouTube. It's gonna do that. And it's gonna be really good at doing it. Yes. There is a part of responsibility to myself to at one point say, Hey, no more YouTube. You know, I've got stuff to do, but at the same time, my environment now is working me because it's pushing me to just watch the next video. As you said, you start watching videos on origami and you know, at eight in the morning and it's two in the afternoon and you're trying to make a little elephant with oami and you spent, you know, six hours watching YouTube videos. Yeah. Well, you know, something you brought up. I've had a couple conversations about this recently and I find it pretty fascinating is. You know a general intelligence, right? In theory, the capacity or the capability for growth is infinite. Right. But on the flip side, there is no incentive for growth in algorithm in itself like humans. Right. We have an incentive to grow because there are other humans. Right. And like, it's wired into our DNA that we need randomness to like perpetuate. We also need like communities to perpetuate. We need competition to like essentially ensure the survival of the human race. That's like, what all this stuff doess like, if we were all uniform, we wouldn't exist very long because there's no like perfect set of genes and whatever it's right. For an AI, there is nothing to push against. So in theory, it might also not go anywhere at all. Like there's no incentive that we've baked in and, you know, like from a psychology perspective, that's a pretty interesting thing too. Right. You know, like, how do you. Do you see that being reconciled? Is there like a reason for an AI to grow itself? Actually, I believe it is, but again, you have to understand the difference in motivation from a human being, you know, we have specific motivations that have to do with our emotions. I mean, motivation is part of our emotional being, it, it, it's not a rational thing. At least not rationality in a way that a computer thinks and it's one of the dimensions dimensions of emotional intelligence even, but for a computer, the motivation is whatever it's goal is and it's going, and the big difference is you are going to get tired. I'm gonna get tired. The computer is gonna be relentless. As long as it has resources, it's going to be relentless on accomplishing that goal. And what we are learning with with machine learning is that. We can give it a goal that never ends because we know it's never gonna get to a hundred percent. If we don't allow it. If we're building good models, it's never gonna get to a hundred percent anything, but it is going to keep trying and getting better. If we tell it that it should try and get to a hundred percent and it's never going to stop. So my thought is, what is the goal of a general intelligence? Like how do we articulate what that is? That is the good question. That is the perfect question. Because at one point, if we ever get new enough to general AI, we're going to have to give it a goal. And that goal either can be accomplished, which at that point, that's it, there's no more motivation or it can be accomplished. And it's just going to try and get better and better and better. Probably the best example as a thought experiment is Viki from what's the name of this, of this one book. It's even a movie iRobot. I don't know if you remember. So like protects humans, right. And it, yeah. Exactly. So that's the goal. The goal is to protect humans the best possible way. And eventually the best possible way is you have to control them because if not, it's not gonna work. But again, that's an objective that the computer is eventually going to find the potentially the best way, even if it's not the best for us. So that's why, and I think we've all learned this when we're coding, computers will only do what we code them to do once we reach general artificial intelligence. And the only thing we actually EV end up coding is the goal and you know, a specific algorithm code. So it keeps learning. Eventually it's gonna get out of our hands because it, it can program itself. I mean, it's going to be able to program itself, but again, I don't know if we're, you know, 30 years from it, 60 years from it, or if we're ever going to reach general artificial intelligence. I think we are. I just don't know where, when. So I, you know, I think that, there's a really interesting, again, crossover with your research, but also your perspective from your experience in psychology, how do we set good goals that are optimal for humans or aren't, aren't harmful? Like wh what, is there a process we can take? Is there something, you know, you don't have to come to like a manifesto now, but I think that they're like, you know, are there rules of thumb that we should take into account when we are setting these dependent variables or these valuation criteria? Yeah. It's I mean, everything that has to do with what B humane means is going to be subjective. But I think at, at least personally, and following, you know, philosophers like Daniel Deni, They say that probably the best standard that we can use is wellbeing. You know, what is the well wellbeing for humanity in general, for people in general we can start making objective evaluations. So for example, is my algorithm generating wellbeing in the mid to long term for people. So for example is being stuck 24/7 on the screen. Good for people. My assessment. And I think it's a fairly objective assessment, is that no, it's not because there's plenty of other things. We need human contact, especially nowadays, but you know, still, hopefully at the end of the pandemic that we don't really know people need to work. People need to have other interests. They, they need to be able to get away from the computer at one point or from their cell phones at what point point in time. So I would say that, you know, start with what is the effect and is this going to contribute to people's wellbeing or not? Because I think that most machine learning models right now are focused on the wellbeing of a company, which is not a human. And apparently the only metric of the company's wellbeing is is it making more money? And unfortunately that most of the time that is gonna come in conflict with human wellbeing. So again, as you said, I don't have a manifesto on it right now. We could start working on it. So it's ready. I don't know, in three or four years, but that would probably be a good start, you know, wellbeing. What is wellbeing for people? And it's my algorithm, my model going to be good for people. Well, speaking about sort of algorithms related to people, you know, something we talked off quite a bit offline about is predicting people's behavior. And I think that a lot of that is the focus of your work. I mean, yes, I would. I would love to hear more about that. I mean, I use quite a bit of data to predict my own behavior, you know, well not, or to inform how I should behave. Right. You know, I wear my like fitness tracker and it tells me my readiness score for the day. Should I work out hard? Should I not work out hard? In what circumstances would predicting people's behavior like behavioral cues be really useful and you know, kind of, I'd love to hear more of that story from you. For me it's is my behavior in the future going to be positive or negative? I mean, is it gonna bring me, as I said wellbeing, or is it gonna be harmful for me? So, for example, if just like you can nowadays where a Fitbit and know how many steps you're taking and, you know, if we could have, I don't know, some kind of a thing that could actually perfectly calculate the amount of food and the quality of the food that we ingest. In theory, we could build a model that can tell you what to eat, how much to work out, to keep you in as best shape as possible for whatever it is that you want to do in your life. So, for example and if we take people who have problems with with moderation, whether it's food consumption of any substance, you know, alcohol, drugs, et cetera, or shopping, you know, that they can control their shopping habits. If we can measure that right now, they have this issue. And we know that there's things that they can do to improve how much they control their impulses to shop, to consume, to eat, et cetera. Then we can start building models that can help because we can, if we can predict that you're gonna misbehave in the future, we can actually start prescribing actions that are going to improve your behavior and are gonna make it less possible for you to make a mistake in the future. I don't know if you've ever read about the marshmallow test Which is for me, it's a brilliant experiment because it, by mere chance, it discovered that the children that had more self control were able to have better outcomes in life. Those are things that we already know. If you can control your impulses, especially when it comes to spending money, to consuming substances, to eating, you will have a better out outcome in life. So the question is, if we can measure that, if we can diagnose that today and we can measure it through time, then we can discover even better and better ways to help you change your behavior because you're not setting stone. The fact that, like, for example, for a long time, we thought in humanity, Oh, if you have low moderation, that's it. it's your problem. It's a problem that you're gonna have for life. And you can, can't do anything about it nowadays. We know that's not. You can learn how to reform your behavior. You can relearn how to act in front of temptation. You can relearn a lot of things. And when I'm talking about moderation, this is just one tiny factor of many. Like for example, can you focus on work? Can you start working on the stuff that you need to do now? Can you stop procrastinating? I mean, there's a lot of things that we know are bad for you. And we also do have already some things and a lot of it coming from behavioral economics of things you can do to try and modify your behavioral a little bit. Like, for example, reward substitution and things that you can do. But the question is how do you measure it? and that's the biggest challenge. I mean you can take a test and know that your moderation is low, but how do I know if my moderation is low today, tomorrow and the day after, because it's going to change, it's going to change based on many, many factors. And how can we measure that in a way that's convenient and how can we build models around it that tell you, Oh, if you're like as you said, you know, you wake up and you have apps that tell you know, your energy is low today. Let's do this to increase your energy, all the same thing today, your will powered to prevent temptation is low. So let's do this. So you don't have, so you can avoid temptation or you can do this to actually increase your temptation avoidance in the future. Or you can do this so you can start working and not procrastinate. So that's the big challenge. How do we just like, we are measuring things with a fit. Or with all these apps, how can we measure your emotional state and your emo to predict specific behaviors in the future? Or maybe not specific behaviors, but, but behavior probabilities, you know, are you going to misbehave in the near in the near future? And what can we do? What can we do about it? I gotcha. So obviously there's a flip side to that, which we already like some governments have been using in a very bad way, you know, like predicting when people will commit crimes and watching them particularly carefully. And it's like, you know, they're probably not more likely to commit crimes than anyone else, but there's more scrutiny on them. They are being monitored in this way. And obviously there's, there's implications around class and and race and those types of things. I will say that this does kind of go against your marshmallow test theory as well. Something that I read recently is that the marshmallow test was refuted quite a bit because there was a huge correlation between the people with with less ability to moderate themselves and social class. And so yes, if you're, for example, poor, and you don't know when your next meal is gonna come, you're gonna be more likely to eat the marshmallow. Not because you have less moderation, but because of the specific circumstances you're in. So I think, yeah, like I, yeah, I actually still agree 100% with the marshmallow, like the overarching theorem, but I still think it's important to note that like, Hey, you know, these things that You know, that are like clear cut, like do these, there's also a flip side and the negative and scary side it's that, Hey, like, like these other variables and these other use cases can confound things, but also, you know, the same beautiful, like incredible insight you're trying to create of helping people. It could also hurt people in the same way. And how do we, how do we reconcile those things? Well, that's the thing it's one of the issues with data is we, we can create models based on the data we have unfortunately reality shaped by the data we have and the data that we don't have. So we have to start figuring out what it is. And as I said, as you mentioned, yeah, the marshmallow test in that particular sense when it comes to food, there is a lot of bias towards. It's been redone with other things. But at the same time, there's also an issue that we do know that people that grow up in lower income families has have less moderation in general. I mean, that's a problem of the society because you have less opportunities, less you can access less education. There's a lot of issues when it comes to social class and how that shapes you at the end of the day. It's not that you can't get out of it. It's that it is a lot harder. There's I remember also a study that was brilliant that for example, people who are constantly worried about paying their electric bill, rent food, et cetera, their IQ is literally about 50% lower than the people who don't in general. So again, the fact that you have a lot of worries is going to affect your ability to make good decision. So the question is again, how can you know, how can we get all this stuff and make sure, as you said that this is not biased, because it is a problem when machine learning algorithms, there's one that's being used for parole. Are you going to get parole or are you going to get along? And they are all literally crucially biased. Why? Because society has been biased. So of course the algorithm is gonna be biased because the data we have is biased. So that's when it becomes really complex and dealing with, as you said, that the psychological part of data. it's a big issue. It's a big issue because there's a lot of things that you need to take into account. But for me, the first thing is trying to figure out if we can predict behavior, I'm still not true. We can do it, but I think it's a lofty goal in a, in a positive matter, I mean, I don't want this to turn into minority report and people getting arrested because you might commit a crime. No, on the end of the day, if this person might commit a crime, what can we do? So this person does not commit a crime. That's not putting them in jail. You know, what can we do to help this person shape their behavior in a positive manner? I know it's very utopic. What can I say? Well, Hey, I like it. I completely live that. I mean, to me, you know, I track a pretty absurd number of things about my daily routine and my life. And I know that, for example, if I get worse sleep. I am going to be significantly less focused. I'll probably like be more inclined to play video games or to use social media. My willpower is lower. Like I know that it is like, I've seen it happen again and again, from like the daily writing and stuff that I do. And like, knowing that it's like, Okay, if I get less sleep, what can I do to mitigate that behavior each day? Right. And that in my mind is like me gaining control over my life with these things. If I had notifications that could help me stay present with those things, like, yes, it's the same thing I'm using the technology to help myself to enable things to make, to make myself better. Yeah. I think something I just, I keep coming back to is like, how do we. How do we change that incentive structures for companies? How do we make it profitable to do that? And I think, you know, like the aura ring that I wear or do a lingo or a lot of these things, if the goal is related to health and yes, they make money, it, it doesn't have to be completely independent of profit, right? Like we can ha we can learn a language and make money. Right. You know, I can make a course that helps people and creates value, but I still, you know, like make a reasonable income. I'm not, I don't think anything that I put out there is exorbitantly expensive. Hopefully not, if it is, that's a problem on my end, but yeah. I mean, gosh, I just see human behavior and it kind of tends towards the extremes with a lot of those things and especially the accountability in large organizations. You know, it's not just one person it's, Oh, this, this I won't name any big organizations, but this company, I just work here. It's this company's goal to maximize profit. You know, if you can point at someone, if you could say, Ken is ripping me off, like that's a different story. Like yeah, there's accountability associated with that. It's a big issue. It, but here's the thing. There's a lot of things that companies can do. and we've worked on this with companies when we we're doing cultural change. For example, personally I've learned how to measure. And it took a while when for example, sometimes it's two, three o'clock in the afternoon and I'm completely burned out. So here are my options and I have to build my life around this. I can either make the decisions to push through my burnout and try to finish whatever it is that I wanted to finish today. And you know, my work is gonna be, it's not going to be, it is a lot worse for it. It's gonna, the quality's gonna drop significantly and I'm going to be even more tired tomorrow. So it's just gonna keep accumulating or can I make the rational decision of saying, Okay, I'm burnt out. It's three o'clock in the afternoon. I could push through, but it's, my work is gonna suffer or I can go to unrest, do some other stuff, you know, get my mind of work go out for a job. Do some yoga, do whatever it is that is going to help me rest and avoid burnout. And that same thing that might take me three or four hours to finish today. I'm probably gonna be able to do it tomorrow morning in 30 to 45 minutes. When my mind is sharp, I had a good night's sleep and I didn't burn myself out. Y. Now, this works personally, and there's plenty of research that tells you do not work when you're, when you're tired, you're gonna burn out and your work is going to suffer. Versus what happens when we put this into a company and into their culture, they have the sense that, you know, you're not a machine. You can't be at a hundred percent all the time. It's impossible. You're going to be at 95. And when you reach a certain point, you need a break. You need to stop and you need to start over tomorrow. And if that's the general consensus on some companies have done very similar things to this, even though they have other issues and their work, their productivity goes up significantly. Not a little bit. It goes up a lot. Why because now people are not working on your regular eight to five until they burn out and they can't work anymore. and they start just doing like the minimum work they can do just to scrape by. But now you start getting high quality work consistently. So productivity just shoots up and we already know that you don't need a 40 hour week to be incredibly productive, especially on certain areas. Now. Is much more complicated when you're talking about warehousing jobs, when you're talking about, you know, very physical labor and I don't have a solution for that right now. I hope someday it'll, it'll come from you from somebody else. I don't care. But I think that as the quality of life of people in improves, even at work, the quality of work improves and the potential for for even better profitability improves. And I mean better profitability instead of more, because it's not necessarily a matter of more income. It's a matter of how to make a company more profitable, which means you know, better use of its resources. And at the end of the day, everybody's happy and everybody's getting, you know, what they deserve in a good way. So yeah, it is, it is quite a balance. It is quite complicated, but it is doable. And I think if we use technology, it'll be better. So for example, There, one of the things that I'm working on and there's plenty of other people working on is can we predict your state of mind? and even your behavior from what's called weak behavioral signals. Weak behavioral signals are, are small often do you look at your phone? How much time are you spending, looking at the screen on your phone? How, how low does the battery get until you re you start recharging your phone, all those things. and they're called weak signal signals because you can correlate them to you can map them to the ocean per ocean personality model, and you can start using that to predict possible behavior. So you know that somebody who who's always plugging in their phone, right. That when it's right, like if it hits 29%, they're desperately plugging their phones. It's has a completely different personality and completely different behavior than somebody who's plugging in their phone. When, when it's at 1%. On a consistent basis, but again, this is just a weak signal. This tells you nothing by itself, but when you add it to, you know, another 10, 20, 30 different weak signals, then you can start having a better and more interesting picture. The same thing you can do when you start getting data from what people are doing online, you know, what are you doing with your computer? How much time do you spend sitting? And if you mix in like information from a Fitbit or other similar IOT devices, then you can start getting more and more a much clearer picture of the behavior of a person. And once you have that, then you can start predicting future behavior. I mean, there's so much, there's so much there. So something that I wanted to call out is we talked about, we talked a little bit about time, but I think time and incentive structure is something that really makes it difficult to. Create a good incentive alignment between customers and companies, right? So you have big, big technology companies, right? Where each quarter they're responsible for earnings or whatever it is, right. And each month or each week, they're responsible for a certain amount of money. And they're, they're held accountable at a quarterly level or an annual level, not like a longer time horizon. Right? A lot of startups or a lot of earlier stage companies in the next, like three years that are trying to do something, you know, like the time horizon for their success is further out. And for people it's the same thing. If I was trying to maximize every single day and like make the most money each day, or like have the, be the fittest every day. And I didn't think about tomorrow, I'd live just a messed up life. Right. And I wonder. How does that factor into our behavior and our decision making? I mean, something you said with the phone, right? Like when we charge it, I just put my phone on the charger every night. Like It's not related to the battery, but it's related to the timeframe, you know, can we look back and can we make more ethical decisions if we change how we, the time snippets that we view our decision making, you know, if companies were looking at customer success and happiness over 10 year periods, would they make different decisions than if they're just looking at it quarter? Yes. At the end of the day, I think it has to do with the same thing that we were talking about. General AI, what is your objective? Is your objective just making money or is there something on top of that objective? And you know, it's I think Simon cynic said it better than I could ever do it. It's a car needs gas to move, hopefully in the very near future, it's just gonna be electricity. But it needs gas to move. But the objective of a car is not to consume gas, not to accumulate gas or consume gas. The same thing should happen with companies. The objective of a company should not be making money. It should be getting from here to there accomplishing this money is the, is the gas is what you need to be able to move from here to here. But the whole system that we built, the whole economic system has to do with, we need to make more money for our stockholders. Yes. Things would change and even profitability would change. And the concept of, you know, growth would change if we started just looking at companies and okay, this is what we aim to accomplish, and we need money to get from here to there. And once we get here, we need money to make this better, but this is the focus right here. You know, this is our objective or purpose, not just making money. You know, it's funny. I see a lot of parallels between the machine learning models that we were talking about before and company objectives, like setting what the goal of the companies is just like what the goal of the models or the dependent variable of the models. You know, it's an almost perfect parallel. I don't, I don't know if you've heard, there's an herbal legend. And I think it's an herbal legend because I've never been able to find the actual paper or anything written about it for real, but as a herbal legend, it works. It works very well. And this, the legend says that this group of college students decided to reprogram their Roomba, their little, you know machine that cleans their home with reinforc reinforcement learning and what they taught it is that for every piece of garbage that it picked up, it got wa it got one point and they were teaching it to maximize the amount of point it got. So it turns out that when they got back, it, most of the house was trashed because apparently the room I learned that if it started hitting furniture hard enough, it would drop stuff. And then it would, it could accumulate more points again. It's a nice urban legend. We don't know if it actually happened. But it does teach you what is it that we're teaching machines? And it's the same thing. If the objective is just to pick up garbage, then creating garbage becomes an option. You know, if it's just, you know, garbage versus points, it becomes a huge option versus what would happen if we were teaching a cleaning machine, just to, for example, loose points. if the floor was dirty for whatever reason. So we have to pick just with machine learning, we have to pick the objective and whatever cost function we choose. We have to pick it very, very, very wisely. I mean, at the end of the day, machine learning is very cool. It can be used for amazing things, but there's so many pitfalls from bad data to biased data, to biased conceptualization, to just picking the wrong cost function. And it can, unfortunately, in some cases it can have very, very, very damaging consequences to people. I mean, imagine as we were talking, imagine that you don't get a loan because you know, of whatever bias the data has. And now, you know, you're stuck in a position where you can't improve your position in life and that's a problem, or you're not gonna get parole because of a bias because of a mistake, a data scientist made or the, or just because we didn't pay attention to the data. On the other hand, how much good can we actually do? I mean, there's, a lot of algorithms are out there doing a lot of good and more being built. I was talking to a team of entrepreneurs just at the end of last year that they've spent 10 years building a model that can check the quality of water just from satellite images. And that to me is like incredible. And I really, I, you know, we worked on a while on it and I wish them the best of luck. I need to contact them again to see how it's going. See that has a big that can have a big impact on human lives. And it's such a big impact that I'm sure they're gonna make money, but at the, at the end of the day, their, their objective was to be able to simplify something. That's very, very important for us humans right now, which is good quality water and figuring out when you know, where the water sources are and when we're contaminating water sources. And so we can do something about it as early as possible. Yeah. Well, you know, it's funny. That's one thing I really, you can say what you want about Elon Musk, but from a goal setting perspective for his companies, The goals that he sets are very like, you know, to essentially have a human colony on the moon, right. From what SpaceX does on a day to day basis. It's a bit divorced from that, but it's clearly focused on like a human centric, non necessarily profit motivated like frame of reference. Right. And I wonder how many companies out there really have a mission like that that is so divorced from profit. I mean, obviously in order to do that, they have to make a lot of money. Right. And I'm sure that making money is on the radar and is the, I think the richest person in the world right now. So, but, but you know, you don't ever hear him to the best of my knowledge talking about money, right? Yeah. You hear him talking about the incredible things that he wants to accomplish. And obviously that seems to be working in financial markets as well. And so maybe there is some, some proof of concept. There is some potential. Inspiration that could be drawn from other businesses as well. True. I mean, at the end of the day, the money is just a fuel to get you there, but I completely agree. I think Elon Musk has been brilliant, not just with space with Tesla. I mean, he literally reinvented the electric car just by making it cool again. Yeah. I don't think we would have the amount of electric vehicles that we have right now if it wasn't for Tesla. Yeah. Well, I actually think it's fairly fitting with the, with the name Tesla as well. I mean, if we go into some history, if we look at essentially Nicola Tesla versus Thomas Edison, Thomas Edison, right? Yeah. Edison, if I recall correctly sold out to general electric and essentially stole a bunch of the work that that Tesla had had done. And, you know, Tesla essentially was an adventure because he loved. The idea of inventing. He created some of the most advanced technologies to think he was responsible for like the alternating current and quite a few other things. And he never truly saw major profit from it. Most people tell you that Edison was a inferior scientist, but an incredible businessman. And that worked, you know, a hundred so years ago, but it's nice to see that that doesn't necessarily work now. And Elon Musk is able to hopefully do good and also create profit bearing the name of someone who was like a remarkable scientist, but probably too far before his time when, when you could be taken advantage of. So there's some, like, I don't know if solo is the right the right terminology, but there's some like, like beauty in the, in the circular nature of the, of the company name, which I, which I quite enjoy as well. And that's a thing. I mean, the question is, are we doing good with our, with our work? I mean, apart, we know we do, we are doing cool things. We're do we know we're doing sometimes very complicated things and amazing things. But are we doing good things that for me should be always the first question when you start any project. Yeah. All right. That's something, I try to ask myself as much as possible too. You know, I find, I find that it's interesting. I find that a lot in in like the content that I produce. Right. It's like, hopefully someone out there can watch a video, maybe get inspired and then create something really incredible, right? Like I'm an okay data scientist to, you know, I'm okay on the technical things and building things and questioning. But am I gonna create something? With my own two hands in my mind, it changes the world. As we know it that like that makes us rethink or we're able to predict something that is, that is outside of what we can do now. Probably not like that is not my space, but I can hopefully educate or inspire someone that does go on to do that. And I think that that's a, like a reasonably viable mission. In my consulting work, I do that mostly for fun, but I'm always thinking about, Okay, like who does this really benefit? Does it improve entertainment, value? Like, like where does that create, you know, come from. And yeah, that's something I personally re wrestle with sometimes. Especially because it is in sports and entertainment, I still love it and I still enjoy it, but I'm really happy. And this important. Yeah, I agree, but I will say I'm really happy that I feel like I have this other platform to really create value or do as much good as possible or maximize on that, which is a really nice thing. Definitely. it's one of those things. The important thing with technology that the first, especially anything that has to do with programming, which is amazing is that you are literally standing on the shoulders of others. It's not just about the knowledge, like literally you're taking, like, for example, I would never nowadays want to build a decision tree or a random forest from scratch. I mean, I've done it when I was learning, but I don't want to do it on a day to day basis. So I'm, I get, you know, sighted learn or whatever other libraries available at the moment and use that to build it. So you are literally standing on the shoulders of other people and you don't need to build that one amazing thing yourself. You can build one tiny thing that somebody else is going to use to put another little building block on it, and eventually it's gonna become one, one of those great things. And I think the best, one of the one most wonderful things we can hope of is to just be able to put a little block in and hopefully be alive when somebody builds something great with it. Yeah, absolutely. I mean, It's nice to think of it that way as well. Is that like, Hey, I don't have to be like, I don't have to build the one thing that changes people. I could just be a part of that process and it's still incredibly meaningful. Yeah. I mean, in terms of building things, you know, what's, what's next for you? How can people get a hold of you? I think I've gone through all the questions that I have. So I'd love to be able to help people connect with you and hear a continuation of your story. Well, next steps, there's plenty of them. First of all, move, which is happening in a couple of weeks. I think when this podcast comes out, I'm gonna be already moved. So, I'll be just settling in then comes working more with with, with my new company, which is our intelligence. And right now we'll, we're just building dag you know, human, emotional, and social, social, emotional skills, diagnostic tools which eventually are going to, you know, they they're, they're the ones that are gonna feed all these other wonderful projects that we have in mind for the future. And if people wanna get a hold of me, I'm very easy to get a hold of on LinkedIn. I'm also on Twitter and just look for the hashtag six days of data. And you'll probably find me as well. Not just Ken, just because I don't post every day, but almost every day. Nowadays I decided that I wanna post jokes once in a while. And people like jokes more than my posts, so I'll probably eventually just post jokes that have to do with machine learning. and if you have any questions, I mean, I love mentoring people who are just starting because I know getting into data science is not easy. There's still a lot of work to do since, because I worked, I worked a lot before with human resources, people, human resources still has no idea how to hire data scientists or data or data analysts and even less data engineers. Most of them don't even know what the difference is between one or the other, which is a big issue. So don't fret start, move forward. And if you need any help, just look me up join the 66 days community. It's an amazing community. Literally one of the best communities I've been a part of because when you make a mistake, people are gonna help you solve it. Instead of just telling you, Hey, you made a mistake. so, and it's a very supportive community. So really join us and just be happy and whatever it is, you do share it. Be happy to share, you know, whatever you can, that doesn't conflict with, whatever it is you're working on. And I mean, really just happy to be part of the community and can thank you very much for opening the doors to data science for so many people. I really hope that by the time you retire, you can see the fruits of your labor. Excellent. Well, I don't think I'll ever retire cuz I love what I do and you know, I get really antsy when I, when I don't do anything even on vacation. So I think it'll be a lifelong journey for me. And you know, even if I don't, I feel like I'm doing the work and doing the things that I really enjoy talking to people like yourself. I'll leave all your, all your links in the description so people can, can reach you on Twitter or LinkedIn. And I'm really excited to see what the future holds for you. Same here and we'll be in touch and whatever you can trust me, we'll build interesting stuff at some point in the future. I'm looking forward to it.