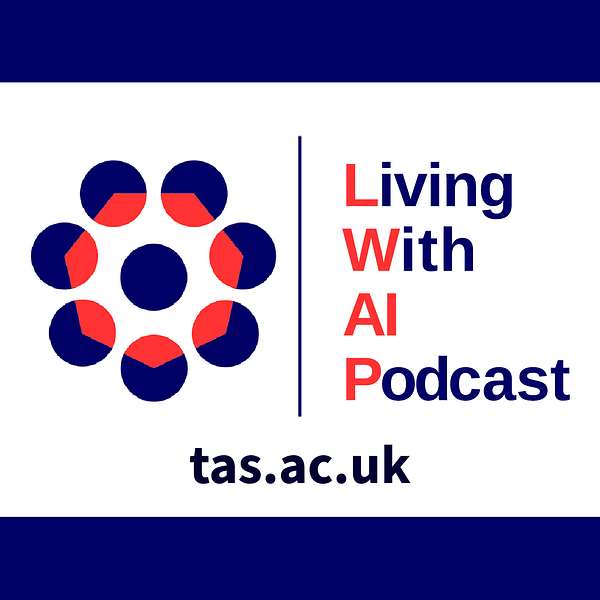
Living With AI Podcast: Challenges of Living with Artificial Intelligence
Living With AI Podcast: Challenges of Living with Artificial Intelligence
Trusting AI With Our Wellbeing (Projects Episode)
In this 'Projects' Episode we've chosen a few projects that tie in to the theme of Wellbeing
Kaspar Explains Dr Marina Sarda Gou – PDRF, University of Herts
SafeSpaces NLP: Dr Tayyaba Azim, PDRF, University of Southampton
A participatory approach to the ethical assurance of digital mental healthcare: Christopher Burr, Ethics Fellow, Alan Turing Institute
Imagining robotic care: Stevienna de Saille, Project Co-I, Research Fellow, iHuman, University of Sheffield
Industry Partner: Melody King, Self Help Products Manager, Samaritans
Podcast production by boardie.com
Podcast Host: Sean Riley
Producer: Louise Male
If you want to get in touch with us here at the Living with AI Podcast, you can visit the TAS Hub website at www.tas.ac.uk where you can also find out more about the Trustworthy Autonomous Systems Hub Living With AI Podcast.
Podcast Host: Sean Riley
The UKRI Trustworthy Autonomous Systems (TAS) Hub Website
Living With AI Podcast: Challenges of Living with Artificial Intelligence
This podcast digs into key issues that arise when building, operating, and using machines and apps that are powered by artificial intelligence. We look at industry, homes and cities. AI is increasingly being used to help optimise our lives, making software and machines faster, more precise, and generally easier to use. However, they also raise concerns when they fail, misuse our data, or are too complex for the users to understand their implications. Set up by the UKRI Trustworthy Autonomous Systems Hub this podcast brings in experts in the field from Industry & Academia to discuss Robots in Space, Driverless Cars, Autonomous Ships, Drones, Covid-19 Track & Trace and much more.
Season: 2, Episode: 2
Trusting AI With Our Wellbeing
In this 'Projects' Episode we've chosen a few projects that tie in to the theme of Wellbeing
Kaspar Explains Dr Marina Sarda Gou – PDRF, University of Herts
SafeSpaces NLP: Dr Tayyaba Azim, PDRF, University of Southampton
A participatory approach to the ethical assurance of digital mental healthcare: Christopher Burr, Ethics Fellow, Alan Turing Institute
Imagining robotic care: Stevienna de Saille, Project Co-I, Research Fellow, iHuman, University of Sheffield
Industry Partner: Melody King, Self Help Products Manager, Samaritans
Podcast production by boardie.com
Podcast Host: Sean Riley
Producer: Louise Male
If you want to get in touch with us here at the Living with AI Podcast, you can visit the TAS Hub website at www.tas.ac.uk where you can also find out more about the Trustworthy Autonomous Systems Hub Living With AI Podcast.
Episode Transcript:
Sean: This is Living With AI, a podcast where we get together to look at how artificial intelligence is changing our lives. In many cases it already has. AI is everywhere. Intelligent microwaves, voice activated assistance, even organising your photo albums. I’m your host Sean Riley and this is the Trustworthy Autonomous Systems own podcast. There’s a whole season of podcasts already up there so check out our back catalogue, the links are in the show notes. If you search TAS Hub, you’ll find us I’m sure.
We’re recording this on the 23rd May 2022 so bear that in mind as you listen with your robot pals. This episode is one of our project episodes where we feature a few TAS Hub projects grouped around a theme. Today’s theme is trusting AI with our wellbeing. We have four researchers joining us and a representative from industry. So Marina, Tayyaba, Chris and Stevie are our researchers and Melody joins us from industry, well actually charity I think is probably more accurate there but we’ll come to Melody in a moment. What I’ll ask each of you to do is just introduce yourself and the name of the project.
Then after we’ve gone round the room as it were, we’ll listen to a bit more detail about the project before we chat about this theme of AI and wellbeing. So just for no other reason than you’re on the top left of my screen, Tayyaba, can I start with you.
Tayyaba: Yes. Thank you, Sean. I’m Tayyaba Azim and I’m actually working as a research fellow in AIC at the University of Southampton. I’m currently working on the Safe Spaces NLP project which is actually looking at behavioural classification of people who are suffering from mental health problems. So it’s been over nine months on this project so we are actually working on a different kind of NLP models which are actually trying to classify different kind of behaviours. So hopefully we will get to know more about the project in the podcast.
Stevienna: I’m Stevienna de Saille, I’m at the University of Sheffield and I am the research lead Co-I for our project which was imagining robotic care, identifying conflict and confluence in stakeholder imaginaries of autonomous care systems. It’s quite a mouthful. I had to put it up to read it. So my PI is Dave Cameron and so basically it’s a social science project, it’s a pump priming project that’s attached to one of the nodes of TAS, the reason node. What we were looking at is literally what it sounds like in the title is how do people imagine robotic care might be provided.
Chris: My name’s Chris. I am an ethics fellow at the Alan Turing Institute which is the National Institute for Data Science and Artificial Intelligence. I’m also the principal investigator or project lead for a project that is looking at the ethics of digital mental healthcare ranging from the sorts of smart phone apps which perhaps the listeners will be familiar with, all the way through to more novel contemporary forms of digital or data drive technologies such as virtual reality for mental health therapy.
Marina: Good morning. My name is Marina Sarda Gou. I work as a research fellow at the University of Hertfordshire. The project I’m working with is called Kaspar Explains and we basically use Kaspar which is a social robot to interact with children with Autism and help them develop their visual perspective.
Melody: I’m Melody King. I am the self-help product manager from Samaritans and I’ve been working on our self-help application and been involved in the project that Chris was talking about about ethical assurance in digital mental healthcare.
Sean: Fantastic. So Tayyaba, can I start with you. Can you tell us about the project then?
Tayyaba: Okay, so has I just mentioned, I’m associated with the Safe Spaces NLP project. The project has been running since September 2021. We are actually looking at the behavioural classification of online discourse at the platform which is offered by [unclear 00:04:17] UK’s leading mental health forum and it actually provides mental health support to young people. So we are actually collaborating with this platform in order to actually get a data set so that we can see different harmful behaviours.
So the project is actually looking at different kinds of linguistic markers in that discourse and we are hoping to actually identify those behavioural markers in the discourse that can help us identify different kinds of mental health problems. So at the moment we’ve got a data set of 200,000 posts and we are actually developing graph based NLP models for this particular data set. In order to actually ensure trust in the system that we are trying to develop, we are actually looking for human in the loop approach where we would like to actually take humans into account.
So we will be using active learning and adversarial learning type approaches so that we can actually develop trust in the system that we are building. Also it’s important to note that we are also taking into account the [unclear 00:05:36] actually has moderators and counsellors who are actually looking into the content. They are not only moderating the data that is uploaded on the forum but they are also providing emotional support to the people who are sharing their experiences and who are actually looking for emotional support.
So we are also engaging with them so that we know whether the system that is being developed is trustworthy enough for the [unclear 00:06:06] staff or not. So far we have actually done some social linguistic analysis with the help of the social scientists who are involved in the team. This isa actually an interdisciplinary project like a lot of other interdisciplinary projects in the UKRI TAS Hub.
So with the help of social scientists who are involved in the project, we have actually done some social behaviour analysis on the interviews that we have taken from [unclear 00:06:40] moderators and counsellors and the clinical experts who are in their team. So we have done with the social behavioural analysis and at the moment we are in the process of developing and refining the graph based NLP models.
Sean: Fantastic. So we’ll go to Stevie. Imagining robotic care, is that the short version?
Stevie: Yes, definitely.
Sean: Tell us about it.
Stevie: Okay, so imagining robotic care came out of an earlier project that Dave Cameron and I did together along with a much larger group of 14 people so including roboticists and we worked slightly with IBM. What was coming out of that, we were looking for good methodologies to involve disabled younger people in stories about what might robotic care look like because most of this is aimed at elder care. That’s really only about half the care using population.
So from that, some of the questions that were open, we realised that everybody comes into these kinds of encounters with something in their head, something that they’re imagining as a robot for care, a scenario but we have no idea what that is. So that’s what we wanted to get at with this project. It was one of the short pump priming projects and we took what we call the health social care ecosystem meaning all the various people that are going to be involved in one way or another making this happen.
So we spoke to carers, we spoke to care users, we spoke to social workers, to academics in care, disability studies, human robotic interaction, to roboticists and designers and to members of the public. Sorry, did I say care commissioners, and counsellor, NHS social workers, all of that. Sorry. Then we also spoke to large numbers of he general public segmented into 20 year ago groups. It was really simple. For the focus groups we used Lego serious play, which is a really fun way to do this. I’m a trained Lego serious play facilitator so that was a natural fit.
The idea of Lego serious play is that it allows everybody equal input in a situation where knowledge might be uneven or power might be uneven. It’s a really good way to surface tacit knowledge tacit values and what you do is there’s a series of warmup exercises to get people used to what you’re going to ask them to do but effectively, all you’re going is you ask a question and people have a set period of time to build a model that helps them formulate a response to the question. Then they share the story of the model with each other.
Then depending upon what you want to get out of the actual workshop, then there’s a directed discussion. So for our purposes, the directed discussion was a little bit more like a traditional focus group at that point but the questions that we were asking were to simply have people design a scenario in which a robot is giving care to someone. We deliberately did not define either robot or care because we wanted to see what they were going to actually come up with.
So we got these fascinating stories about fascinating models but really what’s coming out is what that looks like from their vantage point within that health social care ecosystem and what they see. So there were some, I think real surprises to us actually, the social workers and carers were really positive about the idea of taking some of the burden off but it wasn’t so much a physical burden as I think partly a mental burden because the system is so stressed right now that they know that they’re not able to provide good care.
So the robots that they were imagining were robots that would take certain elements of those stressors off them to help improve what that experience was going to be like for the cared for person. Another thing that we noticed was that older people tended to come up with really fascinatingly interesting scenarios that would allow them to go and do and be, very much the opposite of this passive elderly person stuck in bed with [unclear 00:10:57]. Younger people tended to default towards the older care scenario which really quite surprised us.
We thought it was going to be the other way around. So at the moment we’ve coupled this with depth interviews with certain targeted people, very specific expertise that we wanted to be able to mine in more depth. So the project just came to a close about six weeks ago now and so we’re still analysing this giant pile of data because in a very short project really just gathering the data is all that you can do. So hopefully to have something shortly but what we’re noticing about trust in particular, because that is the topic of this podcast, is that there’s varying layers of trust and that trust can break down anywhere along the way.
So roboticists are normally framing trust as do I trust the machine to do what it’s support do and not hurt me in the process. There is that at the centre, sure, but then there’s a wider idea about what is the presumed purpose of this robot, who has provided the robot. There is another circle that’s more about the control of the functions of the robot, who has control. Do I have control? Is somebody else doing that? Further one on handling of data. Then in the more generalised atmosphere of trust I guess, it’s just trust that automation will drive beneficial social change.
So at any point if you don’t have trust in any of those then you’re not going to have trust in even the most beautiful perfect trustworthy robot. In think it’s that outer circle that’s where our work is lying. We’re stuck with these inherited systems, the broken social care system that we’ve presently got. That is the system into which this will be deployed. It’s not going into some idealised system. So where are the sticking points within that system and how do we make sure that anything that is automated and provided to people isn’t actually going to be used as a cheap substitute for actual care.
Sean: That’s fantastic. Chris, can you tell us about the ethical assurance of digital mental healthcare then?
Chris: Yes, happy to. So I’ve been working with quite a few different people both within our own research team and across the public, private and third sector including organisations like Melody’s, the Samaritans. What we’re trying to do is understand the different ethical values and principles and goals that people prioritise or perhaps care about when they are thinking about the social and individual impacts that not all data driven technologies are having.
This can be quite an inclusive motion, the type of technologies we’re considering, of course obviously the Trustworthy Autonomous Systems hub is focusing largely on those that make autonomous decisions in way or form, often that includes some form of machine learning or AI, a little bit like the natural language processing that Tayyaba mentioned a moment ago. But also a range of the apps that are just replacing otherwise in-person services that say healthcare systems like the NHS would have been providing, so cognitive behavioural therapy provided by apps, of which there are now many.
It’s a bit of a wild, wild west for these technologies at the moment and the different norms that govern them change quite drastically as we move between the public, private and third sectors.
So we’ve been trying to get a handle on this and understand what people prioritise, what matters, what harms or benefits may accrue from the use of these types of technologies and have been developing a methodology known as ethical assurance or trustworthy assurance as a way of helping those involved in the design, development and deployment of these technologies, understand for instance where certain ethical decision making, junctures crop up throughout a typical project lifecycle and what actions they can take to mitigate harms like the potential impact of bias.
So for instance if you were thinking about the goal of fairness, something that often is overlooked in favour of something like clinical efficacy, we think about does this particular system have a positive impact on some health outcome or on some measure or metric of wellbeing. But a second and quite important notion behind that is who has an increase in health outcomes because the way that these technologies are impacting society is not at all equally distributed.
So for instance one of the first case studies we looked at which was actually in the domain of UK higher education, so working with a fantastic researcher, [unclear 00:15:51] who’s been leading on this project, we actually spoke with a range of university administrators across I think about 12 UK universities as an exploratory research and we also ran a couple of workshops with about 40 students. The reason we focused on students as a way of understanding for instance what these individuals care about when it comes to whether to use a piece of technology for mental health or wellbeing or whether to avoid it.
What did they use as values to evaluate or what principles, sorry, did they use to evaluate whether to use the technology. It was an interesting case study to look at for universities because first of all, three quarters of mental health problems begin by the age of 24. The study performed by the Office for National Statistics found that almost two thirds of students in the UK reported a worsening of their wellbeing and mental health between March 2020 and the following autumn obviously, well not necessarily as a result of the pandemic but clearly quite a strong correlation between the events.
Also a lot of universities as a way of trying to ensure their duty of care throughout those times when a lot of students were engaged with each other and with lecturers, with university services, a lot of them obviously were doing so remotely. So some of the services that were being offered, much like in the NHS by universities to support their students with their mental health and wellbeing were delivered through digital technologies.
To make sure that people had access to the help they need, obviously that was done very quickly but we wanted to try and take some time to think about whether or not issues like fairness, accountability, the sustainability of these systems were not necessarily clear on what the long term impact of many of these new technologies are going to have. Sometimes the studies that evaluate whether or not they’re effective or performance in some way will look at it over a course of say three to six months during a research study but trying to get reliable results over say one year, two years, three years can be a very, very difficult thing especially in the context of current funding for research in the UK. So we wanted to try and understand that.
The methodology that we’ve developed provided a very structured means for different stakeholders to start putting together a case for what they’ve done while designing, developing and applying some technology and why for instance some action supports a particular goal, for instance maybe trying to ensure that complicated machine learning modem, why the outcomes or the decisions made by that model are both interpretable to say a healthcare professional but also explainable to the end patient because again in the context of mental health and wellbeing, having a good understanding of for instance why some decision, why a choice to maybe follow some form of treatment or to make a particular recommendation is being given to a patient can be a very pivotal part of their self-care, of their healing process.
It’s something that can be very subjective and therefore requires a lot of understanding, a lot of informed decision making on both the part of the healthcare professional and on the user. So although we explored a lot of that in the context of universities, we’ve also just finished a couple of workshops with stakeholders from the public, private and third sector as well, including Melody who is obviously on this call, to try and understand for instance some of the challenges that they also have on the other side of the table, putting together these technologies and thinking through what can often be pretty complex, ethical questions.
So the hope is this methodology and an online interactive platform that we have built to make it a little bit easier for people to put together so called assurance cases which are forms of transparent documentation that they can use and have been carefully considered in the context of these novel technologies, this platform should hopefully speed up that process of reflection, deliberation and make it more inclusive of the wider group of stakeholders that are often impacted by these technologies.
Sean: Thank you, Chris, thanks for that. Marina, could you tell us about Kaspar Explains?
Marina: Yes, thank you. So basically we’re working with children that have Autism Spectrum Disorder because they usually struggle with visual perspective taking. So this skill is just basically the understanding that other people might see different things that we see, so basically understanding that others might have different viewpoints and perspectives from ours or that maybe two people that are looking at the same object might be seeing different things because they have different viewpoints.
So for [unclear 00:20:42] that’s very easy but for children that have Autism, it’s actually not that easy. It’s difficult for them. One way of helping them is actually introducing explicit causal explanation in their interactions and for that we are using Kaspar which is a social robot that has been specifically designed to interact with children with Autism. So the robot’s face and behaviours are specifically designed for that type of interaction. The first thing that we did was to analyse, we did a retrospective study to analyse what type of games, scenarios or behaviours illicit the most causal explanations. I was the main coder for that retrospective study.
So we had a lot of videos from previous studies and we coded all these videos to see what games or scenarios had the most causal explanations and with the results, then we designed the games, introducing the causal explanations to Kaspar. Right, now what we are doing is we’re sending out questionnaires with the videos with this causal explanation to teachers and also staff members at the university to evaluate those types of explanations, to see which explanations are satisfactory and make the robot trustworthy.
Then from here what we are doing is we are having a collaboration with King’s College London to make these explanations a little bit more automatic, to make Kaspar a little bit more autonomous even though we are always using our Wizard of Oz approach, the difference between what we are doing right now and what we want to do in the future is that the researcher needs to press less buttons during the interaction with the chilren.
Sean: So, Melody, could you tell us about your role in the Samaritans and how they’ve been involved with the TAS Hub.
Melody: Sure. So I’ve been working at Samaritans for the last year or so on the self-help app and effectively what we’re looking to do is find ways to support people with making decisions about their own healthcare, their own mental wellbeing and provide people with the ability to find things that might make their lives better, that might make them happier but one thing that we always have to be very aware of is that generally the people that come to Samaritans looking for support and help are often in crisis or they’re often at the more severe end of the distress scaled so they can’t always make decisions for themselves.
They can’t quite take the cognitive load of some things so you have to be very aware that whatever decisions, whatever information we put in front of people, they may be in different mental states and more or less able to make decisions. You don’t want to take away any of their ability or their right to choose but equally we want to be able to give them as much support as possible. So when the Turing Society, when Chris and Ro approached us to ask if we’d be interested to take part in research about ethics around the area of digital mental health, it was fascinating for us to see all of the different aspects and different approaches from different organisations because it’s very easy to approach it from one perspective.
We want to help people, we want to make it as easy as possible for people to be able to find information. We also want to be able to look at what’s helping people, what people are using more often, what people are finding beneficial but you need to be careful that when you’re using information about what people are saying this is good, this is helping me, that’s their information too. So there’s ethics on all of the different sides of developing mental health support and Samaritans is always known as somewhere that you come for confidentiality, for safety, for trustworthiness and it’s something that as an organisation, it’s almost the most important thing, that you don’t break that trust.
So the work that Chris and Ro have been doing around trying to make it easier to almost make the ethical decisions that we’re making explicit rather than implicit, rather than assumptions, rather than of course I’m not going to do something that would hurt people or that would harm them or put them at risk but actually putting it down in black and white and being able to link the evidence of this is what I’ve done to make sure that I have not done this or so that other people can look at it and actually spot our biases because it’s so hard to see your own unconscious biases.
But to be able to put it in front of people so that they can see but you haven’t thought about this, will that not impact someone else, that’s so vital for me as someone who’s been given the privilege of being able to help people in this way, it’s been a real eyeopener but also it’s something that really takes us forwards.
Sean: Superb. I mean I’m thinking of those popups that we get all the time now, are you really sure you want to do this. I’m getting that kind of sense when things are explicit. Sometimes that can be seen as people covering their backs though. I mean this is not for that though? This is maybe for trying to learn from things and move forward?
Melody: I can’t think of anything worse than doing things in an attempt to cover my back. That sounds a bit, I don’t know, something that people would say if they were covering their back I suppose but for me it’s about making sure that people, it’s not so much about are you sure about this or are you sure about that, it’s about people making sure that we ourselves can be confident that we have done everything to the best of our abilities.
Sean: Sure. I wasn’t for one moment suggesting that you [unclear 00:28:02] but we know we get do you want to accept cookies, are you sure you want to do this?
Chris: The point you raise about the privacy policy is a really good point here, Sean. It’s why we’ve been so keen to speak with people like Melody and organisations like the Samaritans because when it comes to the use, the design and the use of these technologies, often you do find that people have very different objectives, very different perspectives on what matters and organisations like the Samaritans are very well-trusted, they’ve got a good background and history of doing the right thing for people in distress.
But often those types of actions do come into conflict with other values. So for instance when it comes to privacy and an attitude that I think is increasingly important and common and represented by things like cookie questions is that people want to have an understanding, have control over their data but unfortunately other organisations like Facebook, Google, etc, have contributed to a bit of a culture of distrust around how that data gets used. This can often spill over into the context of other sectors like the public and the third sector.
We’ve seen this recently in the last couple of months where charitable organisations working with researchers who are often handling sensitive data such as that extracted from conversations with people who are experiencing distress and don’t necessarily want to spend I’m reading through 20 pages of your privacy policy only to find the answer to one very, very specific question, that’s not the right time to be asking people to go through a bunch of legal [unclear 00:29:42].
So as a result, knowing how to navigate that trade off between doing good and respecting something like data privacy or respective people’s rights to informed decision making is not an easy thing to do in the context where other organisations have made it very difficult to trust people because of slightly sketchy practices and also where we’re involving new technologies that are not often well understood.
So we really want to try and help contribute to transparency and accountability by making some of those assumptions explicit but also creating an opportunity for people to feed back into it so that other organisations know for instance what they need to change and it’s less about a call out culture, more about trying to create a situation where more voices are part of that participatory design process that we need so badly.
Melody: You said that very well.
Sean: It is a very broad overarching topic we’ve got here but there are still points here where different projects I can see link in. So for instance the safe spaces for instance, Safe Spaces NLP I mean is specifically about looking at forum posts but it is analysing language, isn’t it, and therefore there are links here to looking at how people are feeling or trying to work out how people are feeling, which can be a bit of a dangerous thing, can it, Tayyaba?
Tayyaba: Yes, totally agree to that. So basically in the Safe Spaces NLP project, we’ve also taken into account the interview data. So these interviews are actually with the moderators and also with the counsellors. We’ve actually talked about the challenges that they’ve experienced while providing emotional support to the community. I mean we’ve learnt from their experience, from all the interviews that we’ve gathered.
So basically the project is not only taking into account the textual data from the forum but it’s also taking into account the experience of the workers so basically the [unclear 00:31:52] staff because they are the ones who are actually interacting with the community. Their emotional wellbeing is also very important. So when we interviewed them we actually found out the mental health problems because the content they’re actually exposed to is sometimes disturbing or its actually a stigmatised topic which is not very often talked about.
So we also learnt about the challenges of the mental health problems that the staff itself is experience. So basically within the roles that have been identified, I mean there is one role, I mean [unclear 00:32:46] staff role so within the role that each stakeholder has, we’ve actually learnt about the challenges each on is actually facing. Basically when we talk about [unclear 00:33:08] trust into the systems that we are building, we also have to take into account whether the system we are developing, is it actually helping the staff and at the same time is it also trustworthy enough for the users the community.
I mean they are the ones who are actually going to receive the service at the end so are they actually satisfied, do they actually think that system is trustworthy enough to share the information, that they want to share with the community or they want to actually seek support about. So we learnt about all those challenges and this is what the social linguistic experts in the team gathered /information about and they’re actually drawing more insights from it at the moment.
Sean: Thinking of those experts in, did you call it, what was it, socio? I’ve got it wrong.
Tayyaba: Social linguistics.
Sean: Yes. I was thinking of the Kaspar project there because of course the difference here is perhaps in NLP, the Safe Spaces NLP, you’re inferring what people might be feeling whereas you’ve got the opposite almost with Kaspar where you’ve got to be really explicit and you can’t allude to things, can you? Would I be right in thinking that, Marina?
Marina: Yes. So actually I wanted to mention that because we of course- so they are all children, are participants so they cannot consent, it’s their parents. So we have their parents’ consent, however we always are on the side of caution because these children might not express that they are feeling distressed so what we do is the minimum sign that they make if they are not participating or if we feel that they are not liking it, they just go with their teacher, go back to class.
I think that’s very important to always be on the side of caution even though we have the parents’ consent because we don’t want to harm anyone. In fact when I’ve been there with the children and interacting with them and with the robot, I’m just thinking that maybe we have one out of five or six participants that we need to just bring them back to class because we might think that maybe they are not liking it or they are not participating or they just want to go back to class. So it’s better to be on the side of caution when you’re dealing with vulnerable participants.
Sean: Definitely. Just to bring Stevie in here, have you got a similar thing when you’re dealing with such a wide spectrum of people and care because you mentioned it in the beginning there that actually people assume we’re talking about older people here when we discuss robotic care. But actually there’s quite a spectrum of people who need care.
Stevie: Yes. We wanted to bring in the lived experience and that’s one of the interesting things about using narratives but also trying to get, I mean our project is different from the others in the room right now because you’re all working with something tangible whereas our is purely imaginary. That has both benefits and drawbacks obviously because some of the robots that people are imagining obviously will never be built.
Sean: Except in Lego.
Stevie: Except in Lego, yes, and maybe not even in Lego some of them. So what we’ve tried to do is to draw out the underlying values and what is happening under the surface. We’re not really looking at what they are necessarily wanting the robot to do. But two things that really surface, and I was thinking this particularly Chris was talking, sorry, Christopher, whichever, very often what is imagined and we have a reversal scenario so they start with one scenario and then we ask them to reverse it and so it might be a happy scenario at the start, it might be a scenario or pure horror because we’re looking for what is the crux, what is the thing upon which that turns.
One thing I really noticed, and there’s some age dependency in this in that for younger people apps are just more normal anyway. But if the robot is really just a tablet on wheels and not really providing anything more than an app or a suite of apps, what is the purpose of this expensive hardware. So that is one question. I think in a way, in terms of ethical allocation of scarce resources for development, that might be something that needs to be looked at at a higher level, at a funding level. But the other question is to what extent are we throwing our requirements for human contact away by just providing an app.
I think a lot of the people, it was the classic scenario. It’s like it can tell me when I have to take my meds and it can calm my children and it can do this and that and it’s like yes, but you can do all that with your phone. They’re like yes, I can do all that with my phone so what’s the point of the robot then. They’re like don’t know. You can’t see my face but I’m making this I don’t know. That’s the pathway that I would fear more than anything. I feel like there’s a lot of really good working happening in terms of ethical implications.
There’s the very, very sticky area of to develop an algorithm and a robotic is really just an embodied AI, you do need mass and masses of data to train the algorithm and that data will be coming from the people that are adopting these robots and that will be very, very personal data that will have to do with their health, that will have to do with their mental wellbeing, that will have to do literally with the physical layout of their home if we have it in a home, in a private home.
All of these are all questions that I think need to be worked out in this kind of [unclear 00:39:46] period that we have at the moment where we haven’t really developed useful robots yet. A lot of things are still in the preliminary, the design phase where I’m a real proponent of participatory design of getting people in even before you’ve put the research proposal together, if possible, to see what’s really the best way to achieve a beneficial outcome for the most people.
The engineering mindset, the way that mind is disciplined isn’t always quite thinking along those lines, that’s more of a social science mindset. Engineering tends to think more along the lines of what money is on offer and how can we capture it and then what can we do with it that would be good rather than the social science which goes the other way which is here’s what I want to do, now who’s going to pay me to do that. So we do a lot of work trying to bring these things together and trying to bring the social science elements in and the difficulties, help engineers learn to start to wrestle with some of these difficulties because they’re difficult.
It’s very easy when you’re in a place that’s outside your own knowledge experience or your own knowledge realm to just say that’s your job, not mine. But values do get built into design every single step of the way with the decisions that are made. So we need our engineers to be also better equipped to incorporate those questions. It’s not just us and it’s not here’s the money, just build a robot and we’ll tell you whether it’s ethical. That’s not a good idea.
Sean: You mentioned values. We’ve got a really broad group of projects here ranging from vulnerable young people, people with Autism, people at their absolute potentially lowest ebb, calling Samaritans. One thing that really has got to be brought up in terms of trust is the training data that the AI that you’re potentially all relying on uses, what do we do about biases in that AI training data? What do we do about anything that we may not have foreseen. How do we close the loop on that? Anyone got anything to help with that potential question?
Stevie: I mean it is a real problem and there has been a lot of talk about first of all diversifying the people that are writing the algorithms, that helps a bit. But we noticed that as hard as we tried, it was very hard to get diverse focus group samples except for the public sample where we had actually hired an agency specifically to make sure that we had an ethnically diverse sample, that we had a balance of male and female and that we had roughly the same number of people in the different age groups.
But I think you have to work the opposite way. I think when you talk about bias in algorithms because particularly an algorithm that has to do with mental health, what that algorithm will read as normal is so crucial. We need to actually start, I think, with the smallest demographic of people in this society and bring them in at the start to help try to understand how that might work ethically rather than we have a room full of white people and let’s just add some people of colour and now we’re diverse. That’s not going to do it. That’s really not going to do it.
We know that the general demographic of the people developing this are relatively young white men who are from very educated backgrounds. So let’s reverse that scenario looking at the social strata of this country or of any country and say okay, let’s start with the people who are most likely to encounter the app or the robot but have the least power and the least advantage and the least ability to get in, to get their feet under the table where the design decisions are made at an early enough space so people who can test those algorithms very early on, do they respond properly, do they not respond properly.
People who can help, we don’t see things that white is normal, white is normalised, it’s invisible. Male is normal, it’s invisible. So unless you are actually inhabiting a different social aspect, this is partly why we’re talking to people whose job descriptions are so different within this ecosystem because what that all looks like to a social worker is really different than what I looks like to a low paid carer, it’s really different from what it looks like to the council person who’s in charge of the funding and has to provide x amount of services for x number of people on a budget that’s rapidly dwindling.
Chris: If I may just jump in here and echo a few of the points that Stevie has said on this. I think it’s good to differentiate between a few different types of biases. There’s lots of ways we can differentiate between bias but we’ve been running a course at the Turing on responsible research innovation for PhD students and early career researchers. One of the thing we draw attention to there is that there are three categories of bias.
I think your original question, Sean, highlights the fact that an important part of mitigating, first identifying and then potentially mitigating bias in training data set is thinking about the statistical bias, so potentially whether or not the data set is representative or whether or not the groups are balanced. But statistical bias is one form that goes alongside cognitive and social forms of biases. Cognitive biases are going to impact all of us and they’re going to potentially problematics different actions throughout a research or a development project’s lifecycle at very different stages.
This is not something that can simply be solved by going and collecting more data because ultimately it is us that are the source of that bias. It’s our own cognition that is impacting on that. Then Stevie also drew attention to the plethora of social biases hat pre-exist any choice. I think this is a massive field. I think one thing that I just wanted to echo from what Stevie’s point is that when it comes to the use of technology in these areas, it’s important not to use technologies like machine learning or AI as a hammer for which we need to then go searching for nails because when we do that, we don’t reflect upon whether or not the actual application of that technology is going to exacerbate pre-existing biases.
Which is why I actually think also work where we’re doing futures design thinking like Stevie is doing are so important because it creates that space for people to have hopefully a more diverse, inclusive discussion about what we want from technology and how we want it to impact society albeit in a liberal society, the point about a liberal society is that we accept there’s a plurality of ways to live a good life, to bring us back to the wellbeing point. That doesn’t necessarily mean that there’s not going to be consensus around the things that we really, really don’t want.
I think futures thinking conversations can be very, very helpful both in identifying what we don’t want but also recognising when certain domains are just not the right place for deploying technology. I think in the context of mental health and wellbeing that’s certainly going to be the case a lot of the times where we don’t want to be trying to replace people but we want to maybe enhance or augment the decision making of human professionals. So I just wanted to echo a few points and also just add that clarity on the different types of biases and hope that it’s helpful.
Tayyaba: Christoper has actually categorised all the biases very well so he’s covered my point but I guess I would again like to emphasise the fact that biases do exist in the data set. I mean for the kind of system that we are developing when we want to use such a system as an intervening mechanism, it’s important for us to understand the cultural biases as well because we are actually trying to help the communities so I might not be the right time to intervene in a particular community and it could be the right time for another community.
So we need to understand the differences between the different groups we are dealing with. So I would just like to emphasise that when you actually deal with the real world data set where you have textual data or maybe some other kind of data from different kind of communities, the biases do exist and we have to take into account that bias when we are putting trust in the AI system. So we have to take into account that.
Marina: I just wanted to add a small comment on that because there is a thing that maybe we don’t think about and that may be including philosophers in the development of these algorithms and these apps or these software because they are experts on this type of situation. I think that actually would be very useful and something that we need to think about.
Sean: Definitely. Obviously the TAS Hub has got a massive variety of different disciplines which is one of its strengths I think. Melody?
Melody: There was something that Christopher had said about augmenting not replacing in some aspects with this and I thought that was something that really tied in quite closely with what we’re doing and how we’re trying to think about how we can deploy technology but also keep the services that are already there. Things like the self-help app or other ways that we might look to support different communities and support different subsets of people and different subsets of needs, it’s not replacing what’s already there, it’s merely seeing that there is a gap where we could better be supporting a community.
As an Autistic person, calling the Samaritans phone line, which is what everyone knows us for, isn’t something I can do. However, I can access support through written word, I can access support through an app. So having that conscious thought underlying everything that not necessarily one size fits all, not necessarily replacing and also being aware of the bias, that was part of what I badly said earlier around explicitly making things clear.
It was explicitly making clear what biases you have been aware of in the work you’ve done so that you can ensure that you are taking them into account and trying to make it so that the users, the individuals don’t have to worry as much about the fact that you might have forgotten that actually they are an individual and that they may be in a culturally different marginalised group but they also might be in the neurologically diverse marginalised group and they may also have a gender difference that means that they are.
So all of these things combine to mean that their experiences are different from any one of those individual categories. It’s why this work is so important for me because I, who I am, only have this one experience.
Sean: This makes me think that with human in the loop it’s hopefully a bit easier. I think total autonomy in this would be quite scary, quite worrying. So with Safe Spaces and with Samaritans and various of these, we’re talking about a system to aid a human to respond in the best way I think. That sounds really positive. I don’t know where I’m going with that but if anyone has anything to say whatsoever, feel free to dive in right now. Stevie, I’m going to hope that you’re going to throw me a life raft of some sort here. Stevie?
Stevie: I don’t know if I’m going to throw you a life raft or a weight that’s going to sink you. But I would say that it is, when I speak about diversity, it’s also diversity of desires for what is a good life within particular age groups and the bias that we tend to have towards older people in this society anyway is well just for me the horror would be you just leave the older person, whether or not they’re physically still capable of doing things, alone in a room with something that’s basically an Alexa on wheels.
I see a lot of push towards going down that way which always worries me with the there’s an app for that. With the CBT app I think that Christopher was talking about earlier, my first thought was that’s great particularly because there’s a two year wait now to get actual therapy but that shouldn’t mean that you don’t then get the human to talk to in the same way that the automation should not be taking people out of the loop. It can transfer what you’re doing, the carer gets to talk to the person rather than doing the dishes but yes, I think for me that’s the biggest fear.
That’s really the biggest fear is that we fix social problems with technology rather than actually looking at the much cheaper, much more pleasant, much more humane social changes that we could make to organise the way we treat each other and the way we look after each other.
Sean: So my digital assistant is telling me I need to take a break and walk around to up my step count so I think that’s about all we have time for this episode. I’d like to thank our guests this week. So thank you Marina.
Marina: Thank you, Sean.
Sean: Thank you Tayyaba.
Tayyaba: Thanks very much Sean.
Sean: Thank you Chris.
Chris: Thank you and nice to meet everybody for those who I haven’t spoken to before, really interesting conversation.
Sean: Thank you Stevie.
Stevie: Yes, same. It’s lovely to meet you all and thanks a lot Sean.
Sean: Thanks so much Melody.
Melody: Appreciate it, Sean.
Sean: If you want to get in touch with us here at the Living With AI podcast, you can visit the TAS website at www.tas.ac.uk where you can also find out more about the Trustworthy Autonomous Systems Hub. The Living With AI podcast is a production of the Trustworthy Autonomous Systems Hub, audio engineering was by Boardie Limited. Our theme music is Weekend in Tattoine by Unicorn Heads and it was presented by me, Sean Riley.
[00:54:33]