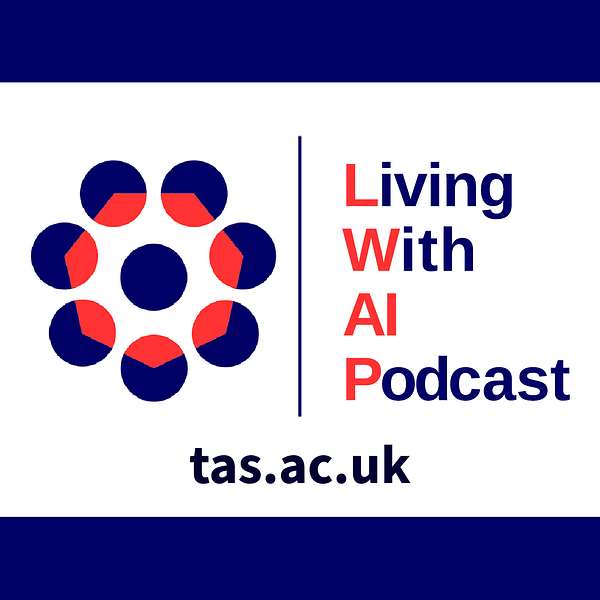
Living With AI Podcast: Challenges of Living with Artificial Intelligence
Living With AI Podcast: Challenges of Living with Artificial Intelligence
Can Machines Care For Us?
This 'Projects Episode' discusses a few TAS Hub projects grouped around the theme of whether we can trust machines to care for us.
Project: Trustworthy light-based robotic devices for autonomous wound healing
Sabine Hauert – Project Lead Contact Reader of Swarm Engineering, University of Bristol
Project: COTADS
Michael Boniface – Project Lead Contact, Director of the University of Southampton, IT Innovation Centre
Project: Trustworthy Autonomous systems to support healthcare experiences
Nils Jäeger – Co-Lead Contact, Assistant Professor in Digital Technology and Architecture, University of Nottingham
Industry Partner: Dr David Crepaz Keay, Head of Applied Learning, the Mental Health Foundation
Podcast production by boardie.com
Podcast Host: Sean Riley
Producer: Louise Male
If you want to get in touch with us here at the Living with AI Podcast, you can visit the TAS Hub website at www.tas.ac.uk where you can also find out more about the Trustworthy Autonomous Systems Hub Living With AI Podcast.
Podcast Host: Sean Riley
The UKRI Trustworthy Autonomous Systems (TAS) Hub Website
Living With AI Podcast: Challenges of Living with Artificial Intelligence
This podcast digs into key issues that arise when building, operating, and using machines and apps that are powered by artificial intelligence. We look at industry, homes and cities. AI is increasingly being used to help optimise our lives, making software and machines faster, more precise, and generally easier to use. However, they also raise concerns when they fail, misuse our data, or are too complex for the users to understand their implications. Set up by the UKRI Trustworthy Autonomous Systems Hub this podcast brings in experts in the field from Industry & Academia to discuss Robots in Space, Driverless Cars, Autonomous Ships, Drones, Covid-19 Track & Trace and much more.
Season: 2, Episode: 9
Can Machines Care For Us?
This 'Projects Episode' discusses a few TAS Hub projects grouped around the theme of whether we can trust machines to care for us.
Project: Trustworthy light-based robotic devices for autonomous wound healing
Sabine Hauert – Project Lead Contact Reader of Swarm Engineering, University of Bristol
Project: COTADS
Michael Boniface – Project Lead Contact, Director of the University of Southampton, IT Innovation Centre
Project: Trustworthy Autonomous systems to support healthcare experiences
Nils Jäeger – Co-Lead Contact, Assistant Professor in Digital Technology and Architecture, University of Nottingham
Industry Partner: Dr David Crepaz Keay, Head of Applied Learning, the Mental Health Foundation
Podcast production by boardie.com
Podcast Host: Sean Riley
Producer: Louise Male
If you want to get in touch with us here at the Living with AI Podcast, you can visit the TAS Hub website at www.tas.ac.uk where you can also find out more about the Trustworthy Autonomous Systems Hub Living With AI Podcast.
Episode Transcript:
Sean: Welcome to Living With AI, the podcast where we look at how artificial intelligence could change our lives. AIs are all over the place already but where can it go from here? I’m Sean Riley, host of this Trustworthy Autonomous Systems podcast. This is season two of the podcast so there are loads of episodes you can go and download from season one. Links will be in the show notes. If you search for TAS Hub you’ll find us anyway I’m sure. We’re recording this on the 23rd May 2022 so bear that in mind if you’re from the future.
This episode is one of our project episodes where we feature a few TAS Hub projects grouped around a theme. Today’s theme is can machines care for us. We have three researchers joining us and a representative from industry. We’ve got Sabine, Michael and Nils, they’re our researchers. David is joining us from industry. So what I’ll ask you to do is ask each one of you to introduce yourself and the name of your project and we’ll listen to a bit more detail about the projects before we chat about today’s overarching theme. Now for no other reason than you’re top left, Sabine, please can you just give us a little intro.
Sabine: Hi, everyone. I’m Sabine Hauert. I’m an Associate Professor of Swarm Engineering at the University of Bristol. Our project was about trustworthy, light based robotic devices for autonomous wound healing.
Michael: My name’s Professor Michael Boniface. I’m a professor of information systems at the University of Southampton and director of the IT Innovation Centre. Our project is called COTADS where we’ve been working with patients, carers and clinicians to co-design trustworthy, autonomous diabetes systems.
Sean: Fantastic. Nils?
Nils: Hi, I’m Nils. I’m assistant professor in digital technologies and architecture and I’m one of the co-leads of the task project Trustworthy Autonomous systems to support healthcare experiences which we also abbreviate to TAS for health.
David: I’m Dr David Crepaz Keay. I’m head of applied learning at the Mental Health Foundation. We are an NGO. We’ve been working in the mental health field for over 70 years. I have a particular interest in how we apply technology to public mental health and particularly the ethical and practice issues that are raised by that. I found the Trusted Autonomous Systems project really, really interesting and engaging. It sits alongside our values based approach to public mental health. I work closely with Nils on his project.
Sean: Excellent stuff. Thank you very much. Sabine, can you tell us a little bit more about this light based healing? It sounds absolutely fascinating.
Sabine: Sure. This project came about because for the past three years we’ve been developing a robotic device that allows us to use light to eliminate lots of little things, so those little things can be bacteria or cells or microrobots. Based on this illumination, something should happen to those little things and then we can image them using a camera to see what’s happening. So that allows us to control these micro swarms using a robotic device.
So with this pump priming, we were exploring whether we could use this robotic device called the Dome to essentially control cells in your skin for wound healing. The idea is that if you could wear a device that used these little lasers to basically activate your individual cells, could you then help drive the wound closing and could you be looking at those cells to basically improve the way you illuminate them on the go? If you go a step further, would you do some form of machine learning to help you find two in the illumination to the specific wound that you’re looking at and could this, just the fact that it’s closed loop, the machine has control of what it illuminates, would you be comfortable with this?
So there’s a whole bioethics envelope to this which is would people be comfortable wearing a device that zaps your cells essentially, helps drive a medical procedure and learns from that process to improve over time?
Sean: Did you have any findings?
Sabine: Yes. It was a great project. It’s a one year project so it’s quite intense. Ana Rubio Denniss, the PhD and the postdoc working on this project, did a lot of work along with the biology team, with Eugenia Piddini and [unclear 00:04:19] to essentially figure out first of all if we could put cells in our dome. So we managed to get our dome working in an incubator growing real cells, basically observing wound healing. So that bit is a proof of concept that we were looking for.
We also managed to use imaging to basically see whether we could zap the right cells right at the edge of those wounds. That’s something that we could do. We changed the light source of our device so that it was zapping cells at the right wavelength because you can imagine it’s not just any light that needs to dry out these cells, it’s a specific type of light. So that bit has also been implemented and is working. On the bioethics front we’ve done use case studies. So we’ve done interviews with the public, interviews with clinicians to understand what they think would be useful in terms of a device for wound healing.
I think that was really interesting because in general it felt like there was excitement. So there was a need for this device if we develop it well and that was really encouraging. But the develop it well, I think is going to be the key going forward, is how do we actually implement this. The final piece of work, so we did the proof of concept that we could grow these cells, image them, basically zap them. We did the bioethics work to understand what would be useful and how we could drive this forward. We did modelling work with [unclear 00:05:40] looking at how we can do machine learning and how we can model these cells.
We also did wearables, little props of wearables that we could put on your arm or stick your arm if them if they were a desktop device, which are just props. They’re 3D prints with lasers so you can imagine what it might look like but sort of next steps. So all the proof of concepts, I think a lot of it is there so good pump priming.
Sean: Fantastic. Michael, can you tell us about COTADS then?
Michael: Yes. Thanks, Sean. So increasing we’re looking at how we might use artificial intelligence to support the management of long term conditions. So there’s a lot of data that’s been collected about people’s activities in relation to their health. You may have seen on the telly for example companies like Dexcom who help diabetes patients manage their condition. They have an app. They can monitor their blood glucose control. There’ll be some thresholds that you can set, some warning signs to help those but these are fairly simple things.
We were looking at how you could use machine learning to make predictions about people’s blood glucose control and how those predictions might fit into the life of someone with diabetes. This is common for many other condition too, whether it’s COPD or other conditions that patients have to manage. The challenge is how do you get patients, carers and clinicians involved in the design of an algorithm. What sort of steps do you take them through so that they can understand the data that’s being collected and why it’s important.
How does the machine learning model work in a way that can be explained to them because one of the routes to trust is to be able to explain and communicate how a prediction is made and how it fits into the lives of those who depend on it. I mean today, much of the care is based on clinical oversight but can we get to a position where we can trust the algorithms themselves to make predictions and for those who have disease to make decisions based on those algorithms.
Sean: Linking back to what Sabine said about closed loop, that diabetes and closed loops, I mean it’s potentially highly dangerous, isn’t it, if-
Michael: Yes, indeed. I mean we weren’t focused on closed loop systems which is the insulin pumps. We were very much thinking about how can people integrate predictions into their lives. What we found is that we actually used what would be a data science tool which we call a computational notebook. This is way of describing an algorithm and how it works but we introduce some user interface elements into that.
So the people who are interacting could visualise their data, they could look at graphs. They could even play with an algorithm and see which inputs to the algorithm might affect the associated risk, and even discuss what additional data might make sense. What we found is that not only did it help the data scientists understand diabetes and what it means to live with the condition, it created compassion. It’s not a case of just sending an alert, thou will react to an alert when it’s made. Life’s not like that. It’s much more nuanced. Knowing when people, and how predictions can fit into the lives of those with those conditions was incredibly important.
Sean: Excellent. Thank you very much. Nils, could you tell us about the healthcare experience project you’ve been working on?
Nils: Absolutely. Thank you. So our Trustworthy Autonomous Systems to support healthcare experiences actually now it’s a little bit of an addition because we looked at that particularly in the home. So how do people manage their specific conditions at home and how could Autonomous Systems support that. So we looked at devices that are in the home, to support decision making about health and wellbeing. The idea here was to look at how monitoring of general health and wellbeing in the home might be supported by specific devices.
We had, from a previous project, a smart mirror that we thought was actually a good idea and a tangible system that could illicit some thoughts in people in how a system might monitor their health, so the smart mirror, as you look at the mirror, the mirror looks back at you and could potentially assess certain medical parameter. We engaged with non-clinical users but vulnerable groups. So we had two PPI groups, patient public involvement groups in multiple sclerosis and stroke rehab groups. We engaged them in the system and showed scenarios of the system at work to them.
So we created short videos that show both a positive and a negative case just to trigger some understanding of how such a system might actually be installed in a home. That was to start to understand what attitudes people might have to these systems and how they might be using them in their decision making around their whole healthcare experience. This healthcare experience would include not only the patient but also the carers involved.
So that could be carers in the homes or family members for example but also then the healthcare professionals like a GP, like a physiotherapist and all the issues around how do you share data with the relevant people, what data does your family need to know? What data does a specialist need to know? Do you want to share all the data? So all of this was kind of included in our scenarios and we ran multiple focus groups with this, a little bit like a copout because we actually wanted to do physical mirrors in people’s homes but because of Covid we had to resort to our videos so we couldn’t really test the device itself.
I think having videos and online meetings did a reasonably good job in getting a similar result. We looked at particularly the shared values that people might have around this. So there’s obviously trust in the system. Does it monitor the right things? Does it really the correct conclusions? How much efficacy has every stakeholder in that process? So I, as a patient, can I control how much data I’m share and with home? How is privacy handled? Are all people at all times able to consent to the data sharing? Do the systems facilitate personalisation?
Not everybody with say a stroke experiences exactly the same thing so there are very different situations which people experience. So we assembled quite a wide ranging team consisting of people with backgrounds in mental health and wellbeing, human factors, human computer interaction, clinical psychology, health sciences and then I did the home part from the architecture side.
Sean: I think that’s definitely one of the strengths of any of these TAS Hub projects, is bringing in all those different disciplines, isn’t it? So just turning to you, David, can you tell us about what you do with the Mental Health Foundation and how you were connected to this project Nils has just told us about?
David: We’ve been really interested in how people engage and are engaged with services and service providers pretty much through all of our history. So the key issue for us in this kind of endeavour is what’s the role of individuals, families, broader members of the public when it comes to using and applying and developing his technology and how do we make it trustworthy and acceptable to broader people. Certainly for a lot of us, so much of this just sounds like science fiction.
Even just sitting here listening to colleagues with projects I’m not involved in, it sounds like the kind of thing that’s science fiction. So how do we then engage people in this in a way that has real meaning for them, that they can teach and learn from the people developing these autonomous systems? So instantly we see things differently. So the first thing people would say is okay, this mirror, this smart mirror in my home, what’s it doing for people who just go past it who aren’t the person this is connected with? Does it switch off its smart thing or does it start analysing other people’s health?
Then is it going to start randomly sending health alerts to my visitors because they’ve glanced in the mirror to tidy up their hair before chatting or between chats. Then it suddenly discovered that they’re at elevated risk of something. We’ve done similar projects around self-management and peer support to the ones that Michale has mentioned although in very low tech ways but we were just starting to think about what are the consequences of systems taking over those business of alerting your peer support group that you’re having difficulties when you are no longer able to do that because of the condition.
So if, in the case of mental health, if you become so distressed that you can’t actually articulate your feelings to someone, at what point is it legitimate for, for example, a wearable device to alert your family, your friends, your clinicians to the fact that you may be in trouble and need some help? That raises a whole range of issues. Then finally we found that there’s been more and more online apps that are designed to support your mental health and unfortunately, a lot of them are simply harvesting data that are being used to target vulnerable people with things that haven’t gone through the rigorous development and scientific analysis that these projects have but actually in some ways sound more plausible than laser guided wound healing.
It’s how do you increase the mental health and health literacy of a broader population so that they can engage in these discussions. Then also, how do we work with cutting edge scientists to give them the language to communicate that effectively to people who would be really interested but would be frightened that they’d just feel ignorant and stupid if they were in the room with someone who’s talking about these things. So I guess we see our role as trying to constructively create conversations that are genuinely useful to broader public and to those developing these solutions that are going to be absolutely vital to sustainable, in our case, sustainable mental health as the world gets more complicated.
Sean: It seems to me that signposting is important here. Maybe things need a bit like a kitemark or something to say this has been endorsed by, I don’t know. What do we think about that idea? Is that the sort of thing we’re thinking here, trustworthy autonomous systems recommends?
David: That would really help. The challenge for us is who do people really trust? If we look back at all the discussions around for example vaccines and so on that we’ve had over the last two years, different people have- we haven’t yet developed the skills. When I grew up you got your information from libraries, teachers, universities and they were doing the quality assurance of information for you and doing some of the curating for you. These days we can all find these things but the skill you need to actually curate that into sensible understanding and knowledge so that you can take part in these things becomes much more difficult.
Sean: Or ascertain whether it’s a trustworthy source, yes. Nils?
Nils: Yes. Just on the back of that, so from our data, some of our participants or people from our PPI groups were actually saying that they’re generally positive regarding these systems, as long as they’re useful of course. But would for example say that yes, I like this system to generate say a treatment plan or certain set of exercises but I want this to be ultimately driven by a person. So I want a person in the background who has a look at this and ultimately confirms that that’s the right way to go.
So just having a system that completely independently makes decisions. At least for our participants, that wasn’t quite at that stage yet but as David said, maybe with increased literacy around these issues, we move towards that in the future.
Sean: So a human in the loop is still a preferred option for a lot of people. Michael?
Michael: Yes. As you were talking, David, I was thinking about some of the lessons we had from the COTADS project which was about this bringing together of human learning and machine learning. These things are intertwined. Your machine can learn but it’s only useful if it allows you to reflect and make sense of the world you’re part of. So that combination of scaffolding, literacy and learning and e-learning is going to be really important to allow our population to participate, to make use of these sorts of systems and I’d say participate in the design of the systems because we want a broad and diverse base represented in the data. We want a broad and diverse base involved in the creation of solutions. But to do that we’re really going to have to work on education.
Sean: There’s a data set issue here as well, isn’t there, the idea of rubbish in, rubbish out. If you’ve got training data that the AI is learning from, is that something to be concerned about as well?
Michael: Indeed. So part of our co-design series, one of them was about bias and fairness. We selected a data set which was a US data set and we presented the demographics and the data. Of course it was primarily white population of a certain socioeconomic group. We did that just to show the fact that there is bias in data and the fact that we needed equal representation and access to the technologies.
Sabine: The theme of human involvement in the automation of the system came up also quite a bit in our discussions. So to a certain extent the potential patients we spoke to were excited about the potential to help treat chronic wound healing or help avoid scars. They had loads of ideas of how it could be used and having the nitty-gritty of how he system functioned didn’t feel like it was necessarily something that they would dive into but something about regulation.
You were mentioning a stamp of approval. I think with these devices we’ll have to figure out is it a medical device, is it a skin care system? How do you take into account the machine learning? So hopefully doing this properly at the start will help us put in place some of this accreditation that at least makes the systems plausible in terms of being safe for the patients to use them. Then many of them said it needs to be part of a treatment plan that involves the doctor and the doctor has oversight of how the system is working, which then raised the question should it actually be home or should it be in a medical setting.
So loads of questions really around how this should be deployed. I think it’s encouraging they are open minded. So the sci-fi angle didn’t actually come through in many of our discussions but we need to understand to what level we involve the right actors.
Sean: One of the things when you were describing it, straight away having visions of Star Wars and all these sort of things where we’ve been watching for decades these robots coming up and fixing the humans, by fixing them or by installing bits of robots in them. David, you wanted to say something before.
David: I just wanted to build on Michael’s point about diversity and different backgrounds because this is really, really important. It’s one of the things that we’re seeing more and more. So I do quite a lot of work in the genetic space and the big biobanks that all the genetic evidence come from are predominantly North American and North European.
If you look at the genetic makeup of the population of the globe, there are small places like Africa and Asia that contribute at least a little bit to the human population but aren’t there represented in those biobanks. We’ve already seen in America some of the way facial recognition systems have been used are racially biased and that’s causing problems. I know here’s been some brilliant work done in natural language processing but I have no idea how good that is in terms of dialects and languages.
But I think Michael’s point about making sure that when we’re doing this broader engagement, it is diverse and it engages with whole sections of populations. In terms of trust, this isn’t anything new. So young black men in the UK hugely distrust our mental health services. There is some justification for that and there is some excessive legend involved in that. But in any case, that’s not about not trusting systems that are digital, that’s actually about not trust systems that are human led.
So having someone you trust from your community navigating you through this, is something that we’ve had to get used to, certainly in mental health and certainly in diversity. So the skills are out there, it’s just a case of making sure that this doesn’t feel like yet another first world white endeavour that leaves out those in the world with the highest level of need and actually the most to gain from doing this properly.
Sean: It needs to be, we were talking about whether to trust technology and digital systems. We’ve had a couple of instances come up recently of people over trusting digital systems. So there was a story I read on Twitter recently about an elderly person who had an Apple watch. He was having a heart attack but because the watch didn’t notify him he was having a heart attack, he thought he was absolutely fine. Now I don’t think this is legend but it could be, but you can see where that might be a problem or where problems like that might occur. I mean what do we do about that? I man where do we set this appropriate level of trust? Yes, Michael?
Michael: In the end when you have such systems that are critical, so for example there are regulations emerging around software as a medical device, it comes down to digital safety. You need to make sure that, okay it sounds like that example, should you use your Apple watch to check whether you’re having a heart attack or not, that’s probably down to education and the right purpose of use. But I think in the end it’s mechanisms within the systems that provide safety and they need to be designed in.
Sean: Agreed. We did have a previous podcast guest who was talking about robotic surgery and they seemed to suggest that in an established area like prostate surgery, that people were choosing the digital option over the human option because actually the results were better. So it might just be a question of getting experience. I mean I was thinking there of Sabine and the light healing, that actually once it’s a proven technology and people see that it maybe outperforms let’s say putting a plaster on a wound, that people will chose it over. What do you think to that Sabine?
Sabine: We see this with autonomous driving as well. I think there’s an over trust in the system and as a result people use the car in a way that it probably shouldn’t. It’s not ready to be used yet so they think it’s fully autonomous and it’s not. It still needs that human oversight. But ideally, I think I would choose the robot car over me, definitely as a driver, in the long term. I think that is the aim for some of these technologies if some of these specific tasks can be done better through automation.
But I think it’s this pivot point, at what point is it clear that the technology is ready? That’s something that we need to make sure that we’ve got right before we do that otherwise we undermine the public confidence in these systems.
Nils: One of our patient groups, the group with multiple sclerosis, some participants said that they were somewhat worried to actually become over reliant on the technology. So not in the sense that they wouldn’t trust the technology to do the right thing but because of their condition that over time gets worse, they were afraid to get too much help too early, to lose independence of certain activities and abilities to do things earlier than they would without having that support.
So I think any kind of automated system, artificial intelligence would have to be able to find that right balance for each individual and have this nuanced approach to avoid the overreliance on the technology, whether or not someone trusts it.
David: One of my favourite examples of where just everyday technology has been really, really useful for a group of people, we do some work with people living with dementia. There’s a group called the dementia enquirers who lead their own research into things that have helped them. One of the groups was looking at Alexa and similar devices.
What they absolutely loved about those devices was it didn’t matter how many times they asked them what the weather was going to be like today or where they were supposed to be today, it never got cross, it actually just got quicker at answering the question. Whereas the people they lived with would get more and more cross every time you asked the question. That was a real cause of conflict. So the complete patience that these devices had to be able to answer these actually really quite important questions, so am I wearing the right stuff to go out today, am I supposed to be at home because someone’s coming to see me today.
All this stuff that well actually frankly I rely on a piece of kit to deal with, that is life saving for people with dementia, is also relationship saving because that piece of kit does it really well with complete patience and certainly saves marriages.
Michael: Yes, that’s a very interesting point, David. Touching on what Sabine was saying, I mean Sabine, you were saying about things being for a specific purpose and bounding that purpose which was quite important. So it was a function that the machine was giving you. David, you were giving it a quality, so infinite patience. It made me think about the question for the whole session about whether machines care.
My view is machines don’t care. They have characteristics that are somehow useful in some circumstances but in the end, humans care. If I’m using a machine to help me with my disease management, I’m caring for myself but I’m getting support for it. Humans care for each other, not machines, would be my argument.
Sean: So machines don’t care, people using machines care?
Michael: Yes, indeed.
Sabine: I love that and I think that’s the important bit of their job, is the caring I think in these medical sectors. The machine in this sense, for our device, they’re able to do things that are potentially super human further down the line, that you couldn’t have a human do in real time unless you have a doctor who is looking at your wound under a microscope over 48 hours and controlling thousands of little pixels of light. So I think it’s also important to see places where we lack a capability that maybe we could design a specific technology for.
Nils: I think Michael and Sabine raise really important points about there is a space and a function for each system. I think our participants also pointed this out. So they don’t necessarily want a system that solves all their problems at once but they want the systems that they are using, and some of them are using quite a lot of systems already like daily reminders and smart watches and things, they just want them to work together to help them in their overall care and treatment plan.
So all of these systems feeding into something centrally that then potentially could be picked up by a clinician who then aggregates that data and makes sense of it and then creates a much more fitting treatment plan I think is what they’re looking for.
Sean: If these machines take over from maybe the drudgerous part, drudgerous, is that a word, anyway, the drudgery of maybe paperwork and organising things and the admin side of things, then that could really help. I mean there is an element here as well of yes, the machines, perhaps we can say almost with certainty don’t care but if they give the impression of caring, is that a problem in its own right? But maybe that’s a question for another podcast. David, you were going to say something. Sorry, I interrupted.
David: No, not at all. I think one of the things that we’re touching on is that there are complementary skills that these systems have and that humans have. I remember some of the trials on reading breast scans. So what appears to be optimal for reading breast scans is once machine and one person. My understanding is that the machines are really good at spotting anomalies and the people are really good at identifying whether those anomalies are serious or just artefacts.
So having a person and a machine look at those breast scans, the mammograms, seems to be optimal at the moment because they bring different skills to that reading of an image. That’s really quite exciting and that gives you that, you’re trusting the machine not to miss stuff because machines are really good at not missing stuff, and then you’re trusting the clinician to draw the conclusion from those anomalous images that they might just miss if they’re looking at thousands of images.
So there are some really good complementary skills and that may be the kind of thing that we could talk more about. So we’re not saying it’s one or the other, these things bring different skills. Whether that’s patience or pattern matching or whatever on the case of the machine and compassion and the detailed understanding that Sabine’s identified that a surgeon can bring but they can’t possibly do it to everybody 48 hours a day.
Sean: There’s always this question that gets raised whenever roboticists are involved is oh, aren’t the robots going to take our jobs? Actually what we seem to be coming to the conclusion is they will just help people do those jobs more efficiently and more quickly. Am I on the right lines there?
David: Well I mean the sat nav in our car has done a really good job of making our map reading arguments redundant. Honestly, it’s not perfect and it still makes errors but rather fewer than two people, one map in a moving object do.
Sean: So we’ve got something to blame then as well, right.
David: Absolutely right, yes.
Sean: But that then interestingly makes me think of the kind of idea of potential overreliance with sat nav. I think there’s a potential, certainly for people who are maybe first time drivers, for relying solely on something to tell them where to go and not having as many, I mean this is all guesswork and anecdotal, I’ve not to got the evidence for this but would there be potentially problems with people not being able to do things the old fashioned way?
David: You definitely don’t need to say that’s not evidence. Anyone who’s been driving in rural Buckinghamshire and relied on their sat nav and doesn’t look at signs that say bridleway, is going to be in serious trouble. I’ve done it myself. So it’s not a substitute. Again, it’s back to this what are people good at and what are machines good at and really understanding that kind of balance.
The real advantage the machines have is that they can learn from much bigger data sets than people can. I think that’s why they’re so good at the pattern spotting because they can absorb far more images in a fraction of the time, far more data than we can. So they are really, really good at that pattern spotting.
Michael: I mean it’s an inevitable trend really, Sean. We go from electricity to the internet to mobiles, to information and prediction being a critical infrastructure. In the end, it’s an evolution of our network systems that we will in the end depend more on. We have to make sure that when we’re building those systems, they’re reliable, safe and trusted.
Sean: Agreed. Hasn’t Wi-Fi become part of Maslow’s hierarchy of needs now or is that just a wish list at a conference?
Michael: I was thinking about the burden of technology. There’s a concept called treatment burden. If you’re having to be reliant on an app or interacting at certain times of day or filling out a form on a mobile phone, that comes with burden that can impact quality of life. We need to be very careful that when we’re designing these systems, that we minimise that.
Sean: I think there’s an element also of addiction on these things. We feel like we need when perhaps we don’t always need as well as it being a burden to actually do some stuff. Then there’s the distraction of clicking on the next app along and going I will check my Twitter feed.
Nils: We had an interesting maybe contradiction of maybe the setting, the understanding of how the system might work in that our participants on the whole understood very well that say a smart mirror or systems in the home that could monitor you day in and day out would provide you with a very, very dense set of data that you otherwise wouldn’t be able to communicate to your clinical professional. So obviously there’s a lot of data available.
Arching that and maybe detecting very small changes over long periods of time was seen as a very positive thing. On the other hand, and here’s maybe where the trust comes in, both in the existing institutions and those systems, they were very concerned that whatever data was being collected by the system, if that was communicated in the wrong way to the wrong say Department for Work and Pensions, might negatively affect their benefits.
So there are I think advantages and disadvantages of these systems that we just, as Michael said, we need to design so that people can really trust them so that if I have a bad day, that that doesn’t immediately get reported to my insurance company, my employer, whichever system there is and that then has some detriment to my wellbeing overall.
Sean: Conversely yes, if you have a good day that it doesn’t look like you can do these things all the time or whatever, yes.
Nils: Exactly.
David: We need to get much better at explaining these data and privacy issues. It simply isn’t good enough to get longer and longer lists of stuff that you just simply click yes, I’ve read and agreed to the terms and conditions and that’s it, your data belongs to somebody else. If we’re serious about building trust, we need to move away from this idea of consent is a one off thing to it being a dynamic thing, actually a highly dynamic thing. We need to get much, much better at articulating the risks and benefits of this data sharing.
I think we can start doing that in peer groups. So part of the self-management and peer support you need to manage your mental health or your diabetes or your chronic pain might also be part of the skill is actually understanding the data and understanding how to share, safely share and provide data and building that into our peer support and self-management system so that it’s not a separate thing, it’s actually part of it. In the real world it is part of it because that’s what we build these systems on.
They’re built on that data and how it’s understood and manipulated. So it’s not a separate thing that you just tick so that we can get on to the care developing, it is part of the care developing and it is part of that trusting, caring relationship that clinicians want these tools to support.
Sean: Just time to thank all of our guests today. So thank you very much Sabine, thank you Michael. Thank you Nils. Thank you David. Thanks for your time and thanks to everybody for listening. Make sure you subscribe to the podcast so you know every time we upload a new episode. If you want to get in touch with us here at the Living With AI podcast, you can visit the TAS website at www.tas.ac.uk where you can also find out more about the Trustworthy Autonomous Systems Hub.
The Living With AI podcast is a production of the Trustworthy Autonomous Systems Hub, audio engineering was by Boardie Limited. Our theme music is Weekend in Tattoine by Unicorn Heads and it was presented by me, Sean Riley.
[00:42:19]