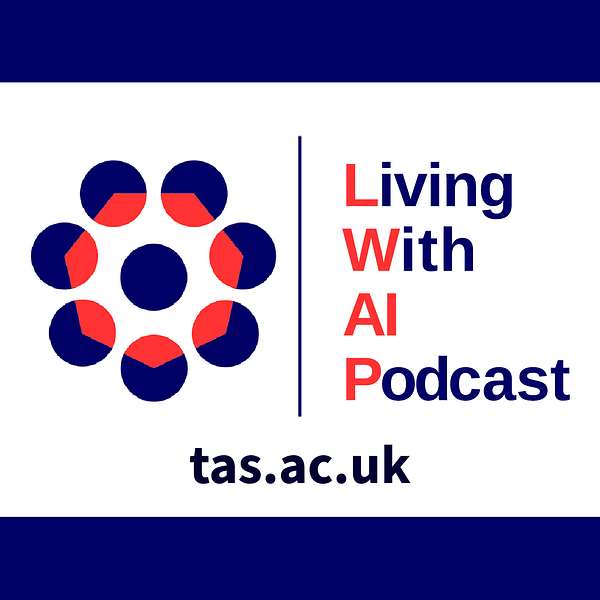
Living With AI Podcast: Challenges of Living with Artificial Intelligence
Living With AI Podcast: Challenges of Living with Artificial Intelligence
Is AI a Fair Judge? (TAS Hub Projects Episode)
Is AI a Fair Judge
A projects episode where we look at a few of the projects that the TAS Hub (Trustworthy Autonomous Systems Hub) has funded:
Mapping Contracts and Licenses around Large Generative Models: private ordering and innovation
Lilian Edwards (James Stewart)
Trustworthy Autonomous Recommender Systems on Music Streaming Platforms
Peter Ormosi
Principles for Building Fair and Trustworthy Autonomous Fraud Detection in Retail
Danni Zhang
This podcast was recorded on 9th May 2023
Podcast production by boardie.com
Podcast Host: Sean Riley
Producers: Louise Male and Stacha Hicks
If you want to get in touch with us here at the Living with AI Podcast, you can visit the TAS Hub website at www.tas.ac.uk where you can also find out more about the Trustworthy Autonomous Systems Hub Living With AI Podcast.
Chat GPT was used to create the below:
Lilian/James intro:
"In this episode of the Trustworthy Autonomous Systems Hub podcast, we're joined by Lilian Edwards and James Griffin, who are part of the team working on the project "Mapping Contracts and Licenses around Large Generative Models: private ordering and innovation". Lilian unfortunately has laryngitis, but she'll still be sharing her insights with us to help understand the legal and ethical implications of using large generative models, and how contracts and licenses can be used to promote responsible development and use of this technology. James may also jump in to offer his perspective and expertise. It's sure to be a fascinating conversation, so let's dive in."
Peter Intro:
"Next up on the Trustworthy Autonomous Systems Hub podcast, we'll be exploring the world of music streaming platforms and the increasingly sophisticated recommender systems that power them. Our guest today is Peter Ormosi, who is part of the team working on the project "Trustworthy Autonomous Recommender Systems on Music Streaming Platforms". Peter will help us understand the complex algorithms and data-driven decision-making processes behind these systems, and how they can be designed to promote trust and accountability for users, artists, and platform operators alike. Whether you're a casual listener or a devoted music fan, this is a conversation you won't want to miss."
Danni Intro:
"Our last guest on today's Trustworthy Autonomous Systems Hub podcast is Danny Zhang, who has been working on the project "Principles for Building Fair and Trustworthy Autonomous Fraud Detection in Retail". In this project, Danny and her colleagues are tackling the complex and sensitive issue of fraud detection in retail, where AI and other automated systems are increasingly being used to identify suspicious behavior and transactions. But how can we ensure that these systems are accurate, reliable, and fair, and don't perpetuate biases or discriminate against certain groups? Danny will help us navigate these c
Podcast Host: Sean Riley
The UKRI Trustworthy Autonomous Systems (TAS) Hub Website
Living With AI Podcast: Challenges of Living with Artificial Intelligence
This podcast digs into key issues that arise when building, operating, and using machines and apps that are powered by artificial intelligence. We look at industry, homes and cities. AI is increasingly being used to help optimise our lives, making software and machines faster, more precise, and generally easier to use. However, they also raise concerns when they fail, misuse our data, or are too complex for the users to understand their implications. Set up by the UKRI Trustworthy Autonomous Systems Hub this podcast brings in experts in the field from Industry & Academia to discuss Robots in Space, Driverless Cars, Autonomous Ships, Drones, Covid-19 Track & Trace and much more.
Season: 3, Episode: 1
Is AI a Fair Judge
A projects episode where we look at a few of the projects that the TAS Hub (Trustworthy Autonomous Systems Hub) has funded:
Mapping Contracts and Licenses around Large Generative Models: private ordering and innovation - Lilian Edwards (James Stewart)
Trustworthy Autonomous Recommender Systems on Music Streaming Platforms - Peter Ormosi
Principles for Building Fair and Trustworthy Autonomous Fraud Detection in Retail - Danni Zhang
This podcast was recorded on 9th May 2023
Podcast production by boardie.com
Podcast Host: Sean Riley
Producers: Louise Male and Stacha Hicks
If you want to get in touch with us here at the Living with AI Podcast, you can visit the TAS Hub website at www.tas.ac.uk where you can also find out more about the Trustworthy Autonomous Systems Hub Living With AI Podcast.
Episode Transcript:
Sean: Now this is season three of the TAS Hub podcast so there are plenty of back episodes for you to binge on, the links are in the show notes. If you search TAS Hub you will find us. TAS is the Trustworthy Autonomous Systems Hub and it is a UKRI funded programme with a vision to enable development of socially beneficial autonomous systems, trustworthy in principle, trusted in practice by the public, government and industry.
And we are recording this on the 9th of May 2023. And shortly we will meet today’s podcast guests. This episode is one of our project episodes where we feature a few TAS Hub projects grouped around the theme and today’s theme is AI and it being a fair judge.
So joining us today on the podcast are Lilian and James, Peter and Danni. So what I am going to do is just ask each of you to give us a brief introduction with your background and the headline of the project you are involved in and then after we have heard from each of you we will get more detail on each of the projects.
So I am going to ask Lilian to start, I know James is here just in case your voice gives out but Lilian if you kick off and then we will see how we get on.
Lilian: Okay, my name is Lilian Edwards. I am professor of law innovation and society at Newcastle University. Unfortunately I have got laryngitis today. And our project is on mapping contracts and licences around large generative models. So we are talking about private ordering and about self-regulation of large mostly image and language models.
Sean: Fantastic, thank you for that. Peter?
Peter: Hello I am Peter Ormosi. I am a professor of economics at the University of East Anglia. Our project was about trustworthy recommender systems in the marketplace. And we had a specific focus on creative industries and music markets but it was really a general overview of the impact of recommender systems on how suppliers compete on platforms.
Sean: Thank you for that Peter. And Danni tell us about yourself and the project you have been involved in?
Danni: Yeah, hello everyone my name is Danni Zhang and I am a research fellow at Southampton Business School. I have worked on product retail projects for more than three years now, so we have different kind of projects focused on product returns. So one of our small projects is to look at the implication if retailers try to use autonomous returns decision system in retail industries. To not only to look at fraudulent returns but also the general returns as well, thank you.
Sean: Thank you Danni. Okay, so this might be a little bit predictable but because of the subject of your project Lilian, and I know James is here and I haven’t intro’ed James so maybe we should come to you in a moment James. But I went for a little help in devising the intros so I asked ChatGPT to give me an introduction to Lilian and the project that you have been involved in. But then I also went in and told ChatGPT that you had laryngitis and that James had come to help you. So let’s see how this works, right, I haven’t read this so bear with me.
Okay, here’s how it starts. ‘In this episode of the Trustworthy Autonomous Systems Hub podcast we are joined by Lilian Edwards and James Griffin.’ It’s not James Griffin is it, it’s James Stewart, well that’s a good start isn’t it? It’s made that up because I just said James. ‘Who are part of a team working on a project mapping contracts and licences around large generative models private ordering and innovation.’
‘Lilian unfortunately has laryngitis but she will still be sharing her insights with us to help understand the legal and ethical implications of using large generative models and how contracts and licences can be used to promote responsible development use of this technology. James may also jump in to offer his perspective and expertise, it’s sure to be a fascinating conversation so let’s dive in.’
Lilian: It’s a bit cheesy.
Sean: It’s very cheesy.
Lilian: Also I am allergic to the use of the word ethical.
Sean: Ethical, it’s in there though. I didn’t tell it that.
Lilian: I don’t do ethics. I do legal.
Sean: Fantastic. Well tell us about the project then now we have had a taste of ChatGPT’s expertise as it were?
Lilian: Right, our project is about self-regulation of large or generative models, sometimes called foundation models. A background to this is that I think everybody knows that the world has gone mad in the last four or five months. We have got used to image models such as Dall-E 2 and Midjourney and Stable Diffusion then along came ChatGPT, which of course took the world by storm. And now we are in the middle of a kind of furore of various countries including notably the European Union, trying to find solutions through regulating, through regulation, to the problems that we now know come with these models.
They have enormous advantages, they are great fun, they are really good for innovation and industry but at the same time there are numerous now well documented problems. And the output they give out tend to be biased and they are often stereotypical, they may contain racial or gender stereotypes.
Some of the problems that have been identified include the fact that these models hallucinate, they make up stuff, you know, they make up fake news. They make up inaccurate information. We are also worried about their capacity to be used to create abusive material or to create for example non-consensual pornography or non-consensual sexual images, sometimes called revenge porn.
And there are increasing worries about the content that they hoover up to make the model. The model has to hoover up enormous amounts of data which is usually trawled from the public internet. And so what we are increasingly finding is that it is hoovering up copyright material, which is really upsetting a number of people, including artist groups and stock photo companies. And it is hoovering up personal data right.
So much of the data that is out there on places like Wikipedia or Reddit or other types of social media will refer to us, will be personal and some of it will be very sensitive. The material that we put into prompts for example can sometimes be very sensitive when we ask about things relating to our health or our sex lives or our social lives, right.
So these are some of the key problems that we will always try to grapple with right now. And one of the places we can look is an existing legislation notably in relation to copyright and in relation to protection of personal information, which is called Data Protection. Many of you will have heard of the GDPR, the General Data Protection Regulation, which we took from Europe, at least at the moment, which protects our personal data.
Another approach which has had a lot of publicity is to create bespoke legislation to deal with these problems and in Europe we are very slowly going through the process of creating something called the EU Artificial Intelligence Act, okay.
What has had much less attention or almost none really, is the fact that there is a tried and tested way of regulating services or products which is by contractual terms, right. So if you think about something like Facebook then there is a great deal of work in which academic scholars have looked at the terms and conditions of Facebook or at the privacy policy of Facebook. Or at the guidance notes and principles that Facebook puts around its products and services okay.
And from these you can see what Facebook itself says it does about things like copyright and harmful content and you know using personal data and what rights it gives its users and so forth and so on okay. So there has been for a long time, a decade or more, a degree of scholarship around looking at self-regulation, what is sometimes called private ordering, yeah.
But large language models or large image models they are so new that this scholarship hasn’t developed and it seemed like it was being sort of forgotten in the rush to address the problems, you know. So the idea behind our project was to kick off really a whole new era of looking at the self-regulatory aspects of these models and we wanted to do an initial mapping exercise.
So it’s very much a pilot project. As we got into it we have discovered that the amount of work to be done, you know interesting work, is gigantic okay and we only had a three month starter project, right. But what we tried to do is we tried to take a sample, so we tried to take a sample of different types of models.
So we had some large language models, we had some large image models. We had some text to audio and video models and we also tried to look at a sector of downstream deployers. So companies that were using mostly the API into ChatGPT to create new services which would have new contracts and new licences and so forth right. And we actually looked at the legal services sector for that, so that was one way we cut it.
[00:10:23]
Another way was to try and get a global perspective so we didn’t want to just look at the UK or the EU. Obviously most of these programmes, these models are coming out of the US so that was a key jurisdiction and is legally very different than the EU. And we also wanted to look if we could, at China and even Russia. So we managed to look because we had someone on the team who spoke Mandarin, which was very helpful, we managed to look at some Chinese models and I think one Russian model plus some from the Commonwealth.
We also wanted to look at different sizes of products. So everyone has heard about ChatGPT, most people have now heard about Google getting into this market but there are lots of small players as well who often are doing, you know, innovative things, open-source for example. So a very different kind of world and so we had a mixture of large, medium and small enterprises.
That all gave us already a very complicated matrix you know, so in the time we had, which wasn’t that much, we did manage to overlap some of these. So we did end up with I think maybe about twenty perhaps models in the end or products where we looked at a variety of their documents.
And again there are a variety of documents so you end up looking at terms of service, you end up looking at copyright licences, you end up looking at privacy policies. A lot of these larger services have guidelines and guidance attached to them so there is a kind of shadow legal system to some extent around some of these products, particularly Open AI and Google, so we looked at what we could.
And then we just really tried to draw out some very tentative early conclusions from looking at this sample, you know. And we particularly emphasised I suppose looking at issues around copyright around personal data protection and interestingly also around dispute resolution. So for example it turned out that most of the American services require consumers to go to mandatory arbitration which is something that is much less commonly imposed in Europe and indeed it turned out in China and South Korean, we also had a South Korean example.
So that was our basic design.
Sean: That’s a fantastic kind of summary of the project. It sounds like you got a lot done in three months. So I am just going to come on over to Peter now and I did the same ChatGPT intro so I do apologise. I feel like these are not designed to be read out but I am going to read it out and I am going to try not do my best DJ voice for it.
‘Next up on the Trustworthy Autonomous Systems Hub podcast we will be exploring the world of music streaming platforms and increasingly sophisticated recommender systems that power them. Our guest today is Peter Ormosi who is part of a team working on a project Trustworthy Autonomous Recommender Systems on Music Streaming Platforms. Peter will help us understand the complex algorithms and data driven decision making processes behind these systems and how they can be designed to promote trust and accountability for users, artists and platform operators alike.
Whether you are a casual listener or a devoted music fan, this is a conversation you won’t want to miss.’
Now look I am sure that we won’t be going into all of that stuff because ChatGPT has a habit of making things up as we have just heard. But anyway Peter tell me about the project?
Peter: That’s brilliant thank you so much Sean. I have to say this would have been a really good introduction to what we were planning to do in about twelve months’ time, twelve months ago. But the thing is when we started off we had this idea to focus on music recommenders. One of the reasons was that these are very sophisticated recommenders, these are recommenders that people engage with on a daily basis. And we had some expertise in the economics of music streaming so it kind of seemed like an obvious place where we could start.
However, as soon as we started talking to our partner, which was the Competition and Markets Authority in the UK, it became clear that there are some underlying issues in more general, in a kind of more general perspective in the relationship between recommender systems and the market place and how these recommenders impact competition in the market.
So we thought we should probably take a step back and take a more, take a broader view. And of course then we did spend some time looking at music recommenders so hopefully I will be able to give you something on that as well.
So to start with something of a motivation, quite similar to what Lilian was using to introduce their project, online platforms and the regulation of online platforms is a very hot topic in policy. If you think about the EU’s digital market sector, digital services act in the UK, the creation of the digital markets unit, there is a lot of discussion about how to regulate or whether we should regulate online platforms. And maybe to take a further step back to just explain to some of the listeners what we mean by online platforms.
So these are virtual market places that bring together the two sides of market, supply and demand. Now customers on these platforms typically face a huge range of choices. If you think about music streaming most music streaming platforms would host over a million songs and podcasts. If you think about YouTube they have over eight hundred million videos as we speak today. But you can think of other areas such as retail if you think about the range of items and products that you can buy on online retail platforms and so on.
So to evaluate these choices customers have got a really difficult task because they cannot survey the hundred million songs or the many millions of items that are available so they have this really big search cost and they also have a big decision cost. So to help customers online platforms deploy a recommender system and these are fantastic because they reduce the search cost, they reduce the decision cost of a customer and you get recommended something that hopefully you will like. So it’s fundamentally an information filtering mechanism that is designed to provide the recommendation that the platform thinks fits the customer’s preferences the best.
However, and this is a big however, platforms have an information and symmetry problem because they don’t know what exactly their users or their customers like. They try to make a prediction and they make this prediction based on data that’s available to them. And if there is bias in the data that they use and if there is potential bias in the way the recommenders work, then the recommendation will be biased. And by the way when I say biased we mean something that is not the optimal recommendation that should have been recommended to the user.
So in economics we use the word bias to mean something else but in the computer science literature this is the understanding is if something is not the most preferred choice of what something, it wouldn’t have been the most preferred choice for the customers.
So okay why do we care about this thing? Because if there are these biases on the platform it can have all sorts of implications. And there has been enormous literature looking at some of the implications, looking at the demand side, looking at whether these recommendations are relevant at all. So this is kind of, it’s a big part of the literature in computer sciences.
There have been studies on fairness, which is more related to today’s conversation, and most of these studies will look at fairness for example to do with things like gender representation in the recommendations. So you might have seen studies about all sorts of gender biases in the types of music that are being recommended by music streaming platforms.
[00:20:12]
Some of the studies have looked at racial biases, there have been studies looking at biases based on users income and so on. Where we came in this story is our focus was much more on fairness in relation to the competition between the suppliers who are selling their products on the platform.
So if you think about music streaming, these are the music companies who want to come to the platform, they want to sell their products on the platform but because of certain biases they might not ever be able to get into the recommendation sets. Other artists might have a much easier job to get into the recommendation set. And sometimes these differences might be similar to what the differences that we would see in a normal market.
Sometimes these differences and these biases are disproportionate and this is where we start kind of paying attention. And why we care is because maybe it’s not so obvious if you just think about music, but if you think about retail or retail platform, if the suppliers don’t compete on a level playing field, i.e. if competition is hindered because of these recommender system biases, it will have an impact on prices. It will have an impact on choice. It will have an impact even on things like incentives to innovate.
And there I can refer back to music, if only certain types of music get recommended, where will be the incentive to create something new if you want to? We want to hear, listeners want to hear new music, things that, you know, things that are so revolutionary that they will change the way the music scene works. Now if we have recommenders that are so biased towards the status quo innovatively because they are trained on data that comes from the status quo, then we have a problem. Because that’s the sort of outcome that I think most users or most listeners would agree they wouldn’t want to see.
So in much of our work what we do is we assume that platforms are user centric, we kind of introduce this terminology. And the reason we do that is because economics, there is already a kind of growing part of literature which looks at self-preferencing platforms and that’s more of an issue maybe if you think about retail platforms, if you think about Amazon, think about allegations that they might have been self-preferencing the products that are vertically related to them.
Now we didn’t want to go in there, we did some work on that but we said okay let’s assume that the platform is user centric, they don’t have these kinds of biases and see if these biases still cause a problem. Okay, so recommender system biases could be a problem for competition and what we find in our work is that certain recommenders particularly which have more biases tend to lead to more concentrated markets. They tend to increase entry barriers, increase homogeneity in the recommendations that are being made.
And like I said, this is the case even when the platform is not self-preferencing so the platform is user centric and it’s to some extent a lot of this is actually intuitively understandable. Entry barriers for example if the recommender system is trained on data all items that are being sold, let’s say on songs that are being played on a platform that are already available, then it will be really hard for a new item to come in. A new item especially if it has got very different features to come in and get on to a recommender set simply because the recommender doesn’t have data on these items.
So we find that there is these inherent biases have got very strong impact on competition. And we did various studies so we brought in methods from economic theory, we have got a paper which is a theoretical user that uses a theoretical model to look at this problem. We have done loads of simulations, that’s one of our main papers which is coming out as a working paper probably within the next week. And we have done empirical work and that is specifically on music streaming.
So there our question was whether early exposure to large editorial playlists increases your, increases the chances of having a recommended that might be struggling with popularity bias. So again the intuition here is simple, if you think about the large editorial playlist those playlists get out to millions and millions of listeners and as an artist those are the playlists you want to be on because that’s how you can increase the revenue that you receive from the platform.
Now if you get exposed on these playlists early on it means that you are already accumulating a large amount of listening and the recommender learns from this and it associates it with popularity. And therefore it is a self-perpetuating cycle and it would be more likely to recommend those songs in the future.
Whereas if you come out with something new and you don’t feature on these playlists, maybe your song is very different. And we do all sorts of metrics to try to match similar artists with similar songs, then just purely down to the fact that you are not being exposed early on you will be much less likely to be recommended later.
And finally a part of our team, which were a team of lawyers and sociologists, were looking at more from the perspective of how to regulate this or whether we should regulate this? And we kind of came to a conclusion that although the area that we, most of us work on, is competition policy and competition law, competition law is probably itself not going to be effective so other types of regulations might be needed.
And I would just highlight one of these and the type of these and that’s a requirement to make algorithms more transparent, more explainable. And these are things that are already envisaged in the EU, there is a platform to business regulation, it’s also in the EU digital services act to require transparency. Because even if there is no, by law there is no infringement of competition laws, it doesn’t mean that behaviour doesn’t impact on competition.
And especially if we care about the longer run then it would be very important to have some sort of solution. And requiring transparency, requiring the understanding of whether there is a bias at all is an important first step in ensuring that.
So I will stop here and maybe some of these issues will come up in our discussions. But I would really like to direct the listeners of this podcast to our work and I think if you Google my name and recommender systems you will probably find our web page where we post all of our working papers, thank you.
Sean: Thanks very much Peter. So our final guest today and this is ChatGPTs words not mine so apologies Danni, apologies in advance. ‘Our last guest on today’s Trustworthy Autonomous Systems Hub podcast is Danni Zhang who has been working on the project Principles for Building Fair and Trustworthy Autonomous Fraud Detection in Retail. In this project Danni and her colleagues’ - I don’t know where it’s getting this from so apologies if you haven’t done any of this.
‘Danni and her colleagues are tackling the complex and sensitive issue of fraud detection in retail where AI and other automated systems are increasingly being used to identify suspicious behaviour and transactions. But how can we ensure these systems are accurate reliable and fair and don’t perpetuate biases or discriminate against certain groups? Danni will help us navigate these challenging questions and explore some potential solutions for building fair and trustworthy autonomous fraud detection systems. Stay tuned for this important and thought provoking conversation.’
Sorry Danni, just tell us what you have been doing in your project?
Danni: Thank you. Yeah, actually this kind of information part of it actually aligns with our project purpose, so to give you a bit of background about our project, so as you may notice that currently there is an increase in online shopping. So retailers have to deal with more returns and a new type of fraudulent returns which are having a significant impact on retailer’s bottom line.
[00:30:07]
So as a result we kind of have to try to think about a new interventions in the market, so this is something we call autonomous returns system. So it is a real time behaviour based system that decides whether to allow the return of a product by using predictive algorithm and a model to identify and deter fraudulent behaviour or abusive returns behaviour in stores, online, warehouse and in all call centres, so this was used across all return channels.
So the developed algorithm are based on customers past shopping and returns transaction history. And of course the system will continuously to learn and adjust its decision based on the customer’s ongoing returns behaviour and the data, the new data put in to this algorithm.
So although this system is widely recommended in today’s retail industries, but we still don’t know much more about the deter information and the trust perceptions from both retailers and customers sides. So in order to address such knowledge gap we used two methods. One is we did fifty interviews with large retailers and the technology companies who provide and develop such sort of systems from the UK, US and Canada to get that understanding and also try to explore what kind of the potential of the issue might be involved in such a system.
Then we also did a survey, customer survey with four hundred and eight-five UK customers to get a better understanding about customer’s perceptions and any concerns in the system involved in their daily returns life.
So just to give you a general key results we get from our interviews. So through the interviews we found, we grouped them into four scenarios in which retailer planned to introduce or had already introduced in the practice based on such a developed algorithm.
So the algorithm was based on the data to divide customers into different groups. So the first type of group is the group who returns quite frequently such as seventy per cent returns rate in the past three months and they are suspected of returning a used item. So the system decided okay, this group of customers they cannot return by post anymore they have to return to stores, that was a recommendation by the system.
And another group of customers was forty-sixty returns rate which indicated an algorithm for returns being of change of mind. So the system decided okay this group of customers maybe not very conscious when they are doing shopping so they are going to send warning messages to alert this kind of group of customers to ask them to reconsider their returns behaviour in the future. Or for some very serious situations the system will start to request a restocking fee so say like five per cent of the price of the returned item, so that’s the second group.
The third situation, sorry the third group is for returning fake item or wrong item or just an empty box. So in that situation then the system not only denies the return, at the same time this group of customers actually are banned from the further purchase by deactivating their account or any associated accounts. Because if you remember the system can identify this group of customers by looking at their address, telephone numbers, so that is the situation.
Then the last situation we identify is actually the good customers. So for the good customer if they have a relatively lower returns rate, say like ten per cent and they always return the item in good condition. So the system decided okay we are going to give priority to refund this group of customers and also offer discount codes for the future purchase as a reward.
So overall the system is not only to detect fraudulent behaviour but at the same time to enhance the good customers returning experience and reduce unnecessary returns and protect our environment as well.
So one of the key benefits that were heard by our interviewees is by using such a system they can make sure, the retailer can make sure all the returns decisions are consistent across all channels for all customers. So i.e. a customer will receive the same results regardless of which store or site is visited or who is working at the return desk.
On the other hand during these interviews we also identified issues which we think retailers or regulators should pay attention to, one is data quality. So like Peter just mentioned, the data is very important for when you build your algorithm. So we found for a few large retailers they actually have not developed a good data collection system and for some of them they don’t even record specific returned items information.
For example they only record this group of customer they returned fifty pounds,, without any other information such as return reasons or the type of product. So this can lead to the inaccurate unstructured and unclear data which then biases the algorithm and potential discrimination as well.
The other issues are the transparencies. So the transparency is quite a concern we found through our studies, so this is because the data used by the algorithm to calculate a decision, returns decision, is not transparent to the customers. So our research team tried to look at some large retailers’ websites and although we do notice that some retailers they said that they might deactivate your account if you return badly. But they didn’t disclose like how they make such a decision, what can affect the action they considered, so such issues could face a legislation problem.
Then we also looked, compared lots of American retailers consent with UK retailers consent as well. So interestingly North America retailers they demonstrate, they express more consent by using this kind of algorithm based on autonomous returns decision system. And we think one of the potential algorithm because there is quite a famous low cost against using such a system in the US. So the retailer in the US who use such a system actually are accused to cause an unfair and restrictive returns policies.
But for the UK retailers most of them they don’t share this consent and they think such a system only affects bad customers so not for the general good customers. For time reasons I will cut it short and give you a bit more about the key result of our surveys.
So our surveys, so the most indicated finding is more than eighty per cent of participants they express they are very happy to shop with the retailer who are using such a system as long as the retailer will follow the policies and give more information about such a system. So in other words the consumers seem to be more open minded and accepting towards such systems in general.
The ones that are not happy with such a system are the most or frequent returners so I think that is not a surprise result. Although we cannot really say all these customers are fraudsters for sure, but it seems to align with the previous conclusion from the interview that such a system only affects the quote, unquote, bad customer. So we also, in the survey, we are also looking at eight ethical factors regarding using such a system compared with a human decision maker. So the factor will look at transparency, bias, privacy, accountability, etcetera.
Again for time reasons I will highlight the transparency issues. So the transparency was rated lower than all other factors, so that means customers generally showed indifference towards transparency provided by autonomous return system compared with human decision makers.
[00:40:00]
And also based on our open questions included in this survey we found that the customer will want to, sorry, the customer thinks if retailer wants you to trust the system they want to know more about how such a system will work. And what kind of factors are included in the algorithm and provide some detailed information of how customers can appeal or combat a decision if necessary.
But of course bear in mind such information is due from the customer perspective think about it, we can’t rule out the possibility that the fraudsters can work around such a system with this kind of information, so it is kind of a dilemma.
These are the key results I would like to share in here but of course this is just a very short project, four months project. We have done a lot and try our best and our research team is still looking for more funding to try to build a framework around this topic, thank you.
Sean: Thank you very much Danni. And just a quick reminder that all of these projects are available to have a look at on the TAS Hub website.
The one thing that strikes me there is that we have got obviously three very different projects all kind of hinging around this idea of, you know is AI a good judge, but I think we have got kind of different sample sizes in each of them. You know if you look at sort of the music streaming it’s millions and millions of tracks are streamed whereas obviously in your surveys you are talking in terms of hundreds Danni there. Is this going to be a big issue do you think? Is sample size really important? Does anyone want to have a go at that?
I don’t know, it’s just what strikes me. Peter I will go over to you.
Peter: Okay, thanks. I think it’s a really interesting point. And it kind of, to some extent it chimes with what I wanted to ask Danni is that in their studies they are looking at respondents from all over the world, people with very different preferences and finding differences across the countries.
So it kind of poses an interesting question whether how we think about fairness in AI and how much of it is a global thing and how much of that will have these localised pockets of the way we think about fairness? And actually if you surveyed a representative sample of people all around the world you would find that people have different ideas about what is fair, what would be required in terms of fairness.
So I think in that respect it’s just because it is a different sample size it kind of, yeah it still raises really interesting questions. And maybe you know sometimes looking at a, looking at the picture from our perspective, which is a much more kind of general picture, you might miss those interesting differences that you actually are picking up Danni in your survey.
Danni: Yeah I have to mention that the survey, the consumer survey we did is based on the UK, so we are only focusing on the UK customers not US customers. But I agree maybe if we want to do a further study in the future we can also look at US customer, what they think about it.
But based on the retailers, so our interviews we found out that most American retailers they are more focused on the customers satisfaction and their reputations as well. That is something that I would like to mention in here.
Yeah I agree for the survey we only focused on the UK but maybe there might be a slightly significant difference in perceptions between US and UK and maybe in other countries as well.
And also from my experience in this two year products returns research project, I found out culture and other things, so like you mentioned we might also need to think about the culture aspect as well.
Sean: And it’s sort of the same with the bias isn’t it? It’s a question of perspective where you are standing and how you view something is whether something appears biased or not biased. I think certainly in news if you are getting it right and you are being unbiased then the people on the right think it’s left leaning and the people on the left think it’s right leaning.
Lilian I think you were going to say something were you?
Lilian: Yeah I was just going to say that our project, except at the very, very beginning of its conception, was never meant to be quantitative, it was meant to be an idea generator. As I say we had a number of categories that we wanted to explore, you know, we wanted to see if the terms and conditions, the terms of service of say a large language model looked very different than those of a large image or AI art generating model. We wanted to see if things looked very different in the US than the EU or indeed in China or South Korea or New Zealand.
The answer to all of these was very largely no, which was interesting. I think one of our main takeaways was that almost everyone, except maybe the very biggest players like Open AI and Google who are using huge libraries on the stuff, conditions that they have developed across multiple products. Almost everyone I think was using already existing licences and already existing privacy policies that they have probably taken and adapted from say social media services or cloud services or internet service providers.
And so what we actually saw, in a funny kind of way, was much less variation then I would have expected. But that in itself is an interesting finding because what it means is that the terms we saw don’t actually fit very well to the product or the service that’s being delivered, right. So noticeably we saw a great many terms and conditions that related to the data that is input by the user, the customer right, the person who signs up to an account, so for ChatGPT.
We saw almost no reference to rights or obligations of people who are not someone who had set up a customer account so you might say third parties. Now you might say that’s not their business because they haven’t got a contract with them but in fact when you talk about things like personal data the service provider has got duties to be transparent about what they are doing when they gather data. Or indeed when they gather copyright words arguably, which, you know, is scope to people who are not in a contractual relationship.
So I think what we were finding was yes sample size was not our main issue frankly. It wasn’t that kind of project. It wasn’t a classic kind of empirical market survey type thing that would be at least an eighteen month project, probably a three year project. And that was at the point where we started looking six months ago when there were relatively few models on the market and now there are hundreds if not thousands.
And it’s also worth remembering that this isn’t a matter of talking to say a hundred people and getting a response to a one page questionnaire or something. Each of these models had terms of service, privacy policies, guidance, principles that went on for pages, you know, twenty pages, thirty pages. It took the work of one RA over about two weeks generally to look at two or three of these services. So if you start to work out the numbers on that you are talking a hell of a lot of time basically.
And I think our takeaway was this was never a three month project so we will be looking for more money too.
Sean: It reminds me of a project that some University of Nottingham researchers did ten or so years ago called Literal Outing which was comparing terms of service to great works of fiction and then giving you your required level of education to understand said things. So for instance, it’s a fantastic marketing thing to be able to say it was Faustian from a very literal sense to sign up to Facebook or iTunes or whatever it was.
Peter over to you, you were going to say something I think?
Peter: So I had a question to Lilian which really interesting project and I look forward to looking into some of the detail of what you have done.
My question is, so the regular though landscape is changing very fast and new regulations are coming out, new regulations are being discussed. There is so much uncertainty in the air both for businesses who have to anticipate what will be the regulation next year or in two years’ time. Also for regulators who have to anticipate what will be the practices that we want to regulate in two years’ time. And maybe by the time we actually implement something it will have already been too late.
So I do wonder if from your perspective of looking at these terms and conditions, how much you see these different businesses and very different, there is a wide range of different sizes and from different countries, whether there is sort of anticipation, or any sort of anticipation of this change in regulatory landscape or are the big guys more adaptive and is it reflected in the terms and conditions or not?
[00:50:20]
Lilian: Yeah that’s an interesting question. Again it has to be said right we have looked at a very small sample quickly so you know take everything with a grain of salt. But my general impression is what you kind of expect if you were a bog standard transactional lawyer as opposed to someone looking at exciting AI, which is you are seeing people use boiler plate texts that they have used a million times before right.
I saw dozens maybe of these that were using a very similar kind of copyright statement to Facebook, you know which is you keep your copyright but we retain some kind of co-existing global non-exclusive perpetual licence. There is a form of words that I saw again and again, so that’s one point.
And I think the other one is that lawyers or people who are stealing from lawyers, will always try to cover their backs, you know. So they weren’t trying to engage with what the DSA might say or what the EU AI Act might say, they were trying to reduce the scope for their own liability as much as possible and wait for someone to sue. Which as you well know consumers almost never do, right, even in America, which is so much more litigative, you know, people still rarely sue.
And actually one interesting point in a few of these terms, and this is why dispute resolution is interesting, was exclusion of class actions. Now again that might not hold up in Europe or in some countries but why not stick it in? So that is what we saw over and over again was all the ability falls on users. Users must indemnify the service if they involve the service in copyright infringement, you know. You have to go to mandatory arbitration run by the service right, guess who they find in favour of? You can’t get a class action which is your cheap way to do it, you know.
And sometimes they just ignored types of law they didn’t like right. So we found with some of the smaller ones that they just didn’t mention data protection. They all mentioned copyright because they are used to this form of action under the US DMCA, Digital Millennium Copyright Act. Which basically says if you don’t provide for notice and takedown then you can be done, you can be done for copyright infringement, so they all know about that. So they all have kind of DMCA notice and takedown type provisions in but lots of them didn’t actually mention data protection.
Or those that did often were only referring to California which has data protection like provisions. So they don’t seem very well clued up a lot of these services that if they get used by people in Europe or sell into Europe then they are going to be subject to European data protection laws you know.
So essentially it’s kind of watch this space for the litigation but again most people don’t litigate and if they do most people settle. We have got a couple of huge copyright cases coming through the pipeline in America and that may kick off. What tends to happen is one of these big cases happens and then it settles, maybe it doesn’t, and that may kick off a frenzy of altering standard terms and conditions. So that would be very interesting to come back and look at as well.
But yeah the whole thing makes you slightly cynical. It’s the difference between legislation created by democratic institutions which take into account a variety of interests and legislation created by a company’s lawyers who try to cover the company’s back.
Sean: I have one final bit that I can read from ChatGPT to round this off. Are you ready for this wonderful bit of prose? Okay, ‘That brings us to the end of today’s Trustworthy Autonomous Systems Hub podcast. We have had a great discussion with Lilian and James about their project, Mapping Contracts’, and obviously James didn’t get the chance to speak but you know ChatGPT doesn’t know that, ‘around Large Generative Models.
Peter with the Autonomous Recommended Systems on Music Streaming Platforms and Danni on Principles for Building Fair and Trustworthy Autonomous Fraud Detection in Retail. From the legal and ethical considerations around AI and biometric data to the’ . So there was going to be a fourth project in today’s episode and I told ChatGPT that it had not made the cut and it is still mentioning it, so you know, we have problems.
Anyway sorry back to ChatGPT’s stuff. ‘We have covered a lot of ground today, thanks to our guests for sharing their insights and expertise and thanks to you for tuning in. Be sure to subscribe to the Trustworthy Autonomous Systems Hub podcast for more thought provoking conversations about the future of autonomous systems.’
It has obviously been fed a lot of these kind of spiel from various kind of Reddit sites and whatever it is of people doing this but anyway. It does remain for me just to say thank you to all of you for being part of the podcast today, this is my words not ChatGPTs. Thank you Lilian, thank you Danni and thank you Peter and thank you James for being there just in case, just appreciate your time.
James: Thank you it’s been a pleasure, thank you.
Lilian: Thank you.
Sean: If you want to get in touch with us here at the Living with AI podcast you can visit the TAS website at www.TAS.ac.uk where you can also find out more about the Trustworthy Autonomous Systems Hub. The Living with AI podcast is a production of the Trustworthy Autonomous Systems Hub. Audio engineering was by Boardie Limited. Our theme music is Weekend in Tatooine by Unicorn Heads and it was presented by me, Sean Riley.
[00:56:11]