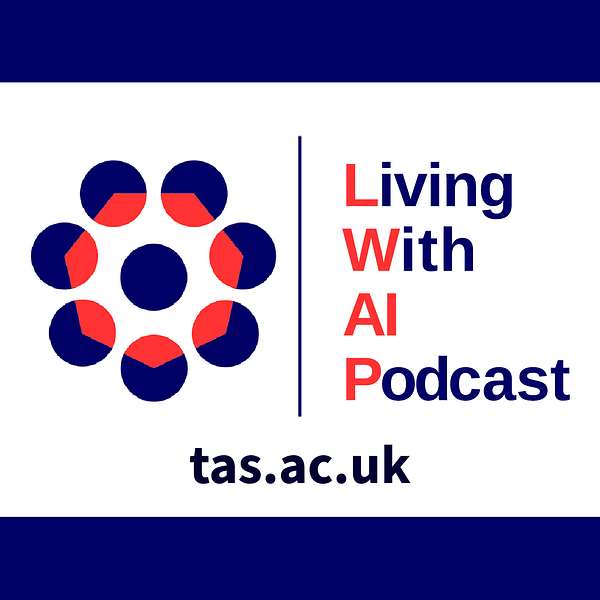
Living With AI Podcast: Challenges of Living with Artificial Intelligence
Living With AI Podcast: Challenges of Living with Artificial Intelligence
Why Do We Use Virtual Assistants Like Siri, Alexa and the Like?
0:30 - Christine Evers
0:38 - Joel Fischer
0:43 - Paurav Shukla
1:20 - Sean Riley
1:42 - Alexa Ruins Christmas (Heart)
11:50 Stuart Reeves
18:10 Computerphile on how Alexa Works
25:40 Eliza - Wikipedia
46:37 Amazon's Ring Security Drone (Cnet)
If you want to get in touch with us here at the Living with AI Podcast, you can visit the TASHub website at www.tas.ac.uk where you can also find out more about the Trustworthy Autonomous Systems Hub
The Living with AI Podcast is a production of the Trustworthy Autonomous Systems Hub
Podcast Host: Sean Riley
The UKRI Trustworthy Autonomous Systems (TAS) Hub Website
Living With AI Podcast: Challenges of Living with Artificial Intelligence
This podcast digs into key issues that arise when building, operating, and using machines and apps that are powered by artificial intelligence. We look at industry, homes and cities. AI is increasingly being used to help optimise our lives, making software and machines faster, more precise, and generally easier to use. However, they also raise concerns when they fail, misuse our data, or are too complex for the users to understand their implications. Set up by the UKRI Trustworthy Autonomous Systems Hub this podcast brings in experts in the field from Industry & Academia to discuss Robots in Space, Driverless Cars, Autonomous Ships, Drones, Covid-19 Track & Trace and much more.
Season: 1, Episode: 5
Why Do We Use Virtual Assistants Like Siri, Alexa and the Like?
0:30 - Christine Evers
0:38 - Joel Fischer
0:43 - Paurav Shukla
1:20 - Sean Riley
1:42 - Alexa Ruins Christmas (Heart)
11:50 Stuart Reeves
18:10 Computerphile on how Alexa Works
25:40 Eliza - Wikipedia
46:37 Amazon's Ring Security Drone (Cnet)
If you want to get in touch with us here at the Living with AI Podcast, you can visit the TAS Hub website at www.tas.ac.uk where you can also find out more about the Trustworthy Autonomous Systems Hub
The Living with AI Podcast is a production of the Trustworthy Autonomous Systems Hub
Podcast Host: Sean Riley
Producer Louise Male
Episode Transcript:
Simon: Welcome to Living With AI, a podcast where we get together to look at how artificial intelligence is changing our lives. Today we're looking at virtual assistants and smart speakers. In a few minutes we'll hear from Stuart Reeves, who's an assistant professor at the University of Nottingham. He specialises in human-computer interaction and we'll be talking about those assistants in a bit of detail. But first let's introduce our panel. Getting animated over artificial intelligence today we have the regulars Christine, Joel and Paurav.
Christine is a computer science lecturer at the University of Southampton, she specialises in machine listening and likes cycling. Joel is an associate professor at the University of Nottingham who looks at human-computer interaction. He's a runner and a climber and Paurav is professor of marketing at Southampton Business School. Now last time we discovered that Paurav has injured himself and I noticed you've been using speech-to-text to send emails, which did cause a small amount of confusion until I spotted that in an email a couple of days ago. How's it working out for you then, Paurav?
Paurav: With greater accuracy but at the same time, Sean, I can tell you that the kind of mistakes, my accent and the speech recognition system, God bless. There are times wherein I feel like, oh my God, I thank Lord I did not click send button here.
Sean: Well, attempting to steer a path through all of this, it's me, video maker and technophile, Sean Riley. We're recording this on the 19th of November 2020. So as ever, if you're a historian from the future listening to this, I really hope everything's worked out okay. Anyway, should we, without further ado, let's have a chat about what's been going on this week then. Anyone got anything exciting? Paurav, you've started talking, let's hear from you.
Paurav: Yeah, it was quite an interesting news I read today morning only, Sean, was that a mum has just sent a warning to people regarding these smart devices, a device which should not be named but starts with an A and it's a wonderful woman's name. And that device, particularly what happened was that the seven, eight-year-old girl of this lady asked this device very sweetly, you know, “What did I order yesterday?” And the device told this little girl that this is a particular item you ordered yesterday and it will be delivered for as your Christmas gift by this time. And that was it. You know, all the surprise was gone and so the mother has particularly written this social media, you know, outcry that be very careful. Such wonderful things are happening.
Sean: Absolutely. We can see all sorts of ways that could go wrong. I'm not going to go into the detail in case anyone with small children are listening. Anyone else had something like that happen to them with AI? Secrets that have gone wrong?
Joel: I'm not sure about secrets, but there are certainly plenty of other things that go wrong all the time when using these smart speakers.
Sean: We have a different kind of, we don't have a female name to call for our smart speaker. And again, I won't say, in fact, I can say it's a Google because that shouldn't trigger it, but might well do. But yeah, it doesn't always do exactly what you expect it to do. The volume seems to have a life of its own. So sometimes you can't hear it and sometimes it blasts you out. But, you know, these are just, again, back to that same old thing, this is implementation, right? Why are they not listening to us properly, Christine? I'm going to hand that over to you.
Christine: I was just going to go into that. I think the problem is that we as humans, we have such an advanced system for auditory perception, so for the ability to actually listen, that we don't realise how difficult many of the scenarios are that we're in. There's a huge amount of uncertainty. I mean, you've got background noise, say the humming of your fridge. There's other people in the room, different voices. Some people may be talking to it, maybe some people aren't. And for us, that all naturally makes sense because we've been in this world for God knows how many decades. But a machine is learning all of that from scratch. So it's the uncertainty in the system that basically provides quite a huge challenge that machines need to address.
Paurav: I have a different question in regards to this, what Christine just said, and I'm just fascinated by it in terms of, Christine rightly pointed out that these are very new devices. They are learning. They are like little babies. But we are expecting so much out of them, I feel. We want a perfect answer every time and we get really annoyed when it doesn't do that. And that annoyance is also quite interesting to see. In my house, being an Indian family, we would ask for sometimes Bollywood songs or Bollywood singers and Hindi names and different kinds of names. And the kind of mistakes that are being made, that have become now jokes for us. In reality, we are not expecting the device to really answer that. So, it's part and parcel of the whole joke.
So sometimes in the Friday family time, we would be sitting together and watching a movie and we would ask a question, you know, tell us about this particular actor's movies, and this device will come up with the kind of things which are just at times horrific. But hey, so is it our expectation, is one of my things I feel about them.
Joel: I think it's really a great point you're raising. And yes, expectations I think is one of the key things here. So I think with these devices, they sound so perfect, don't they? When they speak, they have such a crisp, clear voice that it sounds quite human, although eerily not so at the same time. But you know, why are we doing that then? Why are we setting the user's expectations so high by providing this kind of perfect sounding voice? And also another thing around this is, why is it always a female voice in most cases? Why does it have to be a gendered voice? Why is it not a neutral voice? Why doesn't it sound like a robot?
I think there are some concerns people raise all the time around how people as a result talk to these devices. And it's not just how they talk to the device. I mean, the device doesn't care, it's a computer, but it's talking to the device around other people, which might set a bad example and so on. And I think that's interesting. So the decisions that designers make in how they make these devices sound are actually really important.
Sean: They become almost kind of the butt of a joke in some cases as well. As Paurav just mentioned in his house, you know, there are running jokes from mistakes presumably it's made. And in our house, we'll have one of the children start to try to address the device and the other one will start making all sorts of noises to make sure that their address goes wrong and all sorts of things like this happen and it becomes almost like a running joke. Christine, is this is this normal?
Christine: The jokes? Well, yeah.
Sean: Well, using these devices, but working like they are, I mean, you know, is this, is it also employed by these massive corporations to try and make us see a friendly face of their corporation?
Christine: Well, it depends on what they're trying to achieve, isn't it? So if you look at the products that are deployed in our home, I suspect that the companies that supply those products have the feeling that they need to embed an artificial intelligence in our lives, and as such that artificial intelligence somehow needs to fit with us. And I assume that they feel that the sound of another human would perhaps be more acceptable within our own home, than perhaps some artificial voice that we don't associate with something that is biological.
The interesting bit about that is, if you actually look at robotic platforms, I've got one robot, for example, that sounds very much like a machine and not in the sort of rhythm of the language, the language is still very much human, but the voice itself is A, neutral, and B, it's almost childlike. It's a very small robot as well. So it's something that naturally would be used for teaching or for interaction with, say, children, and they're very, very popular for education in that sense. But it's interesting to see that with that completely different application, that probably normal end users wouldn't even consider purchasing a robot, that there, suddenly the voice is something that we very clearly recognise as not biological, not human, and clearly artificial.
Sean: Is there an expectation that a childlike voice might be treated more childlike by us, the kind of users?
Christine: Yes, I think that's very much the case there. I think if you deal with a robot, for example, I think as a human, we have a fairly good understanding that robotic systems are advanced for the stage that they're in, but they're nowhere near a natural or intuitive level of interaction with humans yet. And I think having that childlike voice helps us to reinforce that it's something that you can sort of experiment with, it's a case study, it's showing the potential, but it's not actually something that you expect to work straight out of the box. Whereas the voice assistants that we as end users can purchase at the moment, we actually pay money for it. And the question there is, should we actually expect that it actually performs up to standards that are being promised to us? Should we as end users and as customers be in a position where we can say this is not actually interacting, you're selling this as an interactive device, and actually it's not intuitive just yet?
[00:10:01]
Sean: One thing that we found in our house using the smart assistant is that it sort of falls into this rut of only being able to do two things, even though it's potentially capable of much, much more. We use it as a timer in the kitchen because when you're, say, chopping something and you haven't got a spare hand to press a button, then it's handy to be able to ask for a timer to be set. And we ask it to play music and very, very little else, unless one of the kids decides to try something different. Paurav, is that your experience as well?
Paurav: Very much so. I'm very fascinated by this. One of the main reasons is that have this become almost, instead of what they are called to be smart speakers, become glorified music devices for us. I still remember the old example of microwave ovens. When they were launched, they were told to us as these are your next generation of cooker and they have really become heating devices, reheating devices for most homes. And so are these smart speakers that should not be named predominantly becoming our, you know, just music speakers largely?
Christine: Yeah, I do agree. And I think there's also a tendency in the market by now, looking at sort of recently launched products from large companies, where I think potentially even the large corporations are acknowledging what we're really using these devices for and are sort of repurposing or changing the branding to adapt it to what it's truly used for.
Sean: Yeah, I mean, I think the times that we've used it for maybe making a quiz or occasionally asking for the weather are very, very seldom these days. You know, it's a bit like anything, you know, you have a flush of the novelty and then after a while it settles into, as you said, with the microwave, it's a heating device, even though in the beginning it could do this and it could do that and it could do everything. Yeah, not anymore. Well, time now to hear from Stuart Reeves. Welcome to Living with AI, Stuart.
Stuart: Hello.
Sean: Stuart is Assistant Professor at the University of Nottingham, specialising in HCI, Human Computer Interaction. Recent papers he's worked on include Conversation Considered Harmful, talking about interaction and how UX practitioners produce findings in usability testing, and UX stands for user experience. Now, I've interviewed Stuart before on the Computerphile YouTube channel and we discussed how a certain popular digital smart assistant with a female name that begins with an A works. You'll notice I'm tiptoeing around the name itself. It's not for legal reasons, but because lots of people get very upset when you start saying the words that make their smart speakers wake up. It's a problem, isn't it, Stuart?
Stuart: It certainly is. Am I allowed to say the word now?
Sean: Well, I think so. I mean, I think there's a set of audio frequencies you can remove to disable the, I'm going to say, the Alexa watch word, but I don't know if it works for all smart assistants?
Stuart: Yeah, I mean, you can kind of, you can hack it, I guess, and there is a kind of adversarial machine learning aspect to it where you can, you know, people have been experimenting with creating things that don't sound like Alexa or whatever it is, but actually do have the right kind of signal and triggers, the model to activate or whatever, and therefore activate your smart speaker. So you can hack them in that way.
Sean: I think I read somewhere it's because they needed to be able to use the word in advertisements, etc. and commercials, but they didn't want it to be activating everybody and driving everybody nuts because, you know, they wanted to keep them happy because they were their customers. But anyway, what are the mechanics of this? I mean, assuming that we do say that word, what happens then? What actually happens from that point onwards?
Stuart: Yeah, so I think I should, I should caveat some of it with, obviously, I'm not an AI academic, so some of this is sort of kind of guesswork based on things I've read. So some of the listeners might decide that I’m not quite right.
Sean: But I know that, just to interrupt there, but I do know that you've done a lot of research on this and that you've got various, yeah, you've certainly done a lot of working with these bits of technology. So you've got a certain amount of information anyway.
Stuart: Yeah, I mean, certainly kind of the wake up word tends to be detected on the device, on these sorts of devices. So, you know, if you've got an Amazon Echo or Google Home, that wake word is triggered on the device. I mean, I guess there's a bunch of reasons why you do that, it's a lot quicker, you don't have to send data up to the cloud to get processed and so on. Also there's element of privacy, I guess, so that other bits of audio that, you know, are not kind of sent away. Anyway, so you say a wake word of some kind, although actually increasingly, I think some of them, certainly the Echo and others have modes where you don't need to say the wake word subsequently, so you can continuous queries.
But after that, the bit after the wake word then gets captured. And it's not done incrementally, it's done in kind of a big, you know, one package essentially, and then sent up to the cloud for processing to the back end of these systems. Then some decisions are made around how to actually produce a response. Also, you know, there's things like error correction and stuff going on so maybe the transcription didn't work so well, or there's some uncertainty, lack of confidence in what the kind of next turn should be, and what should be produced.
So you might get an error back from the device, or you might get, you know, the thing you actually wanted to get in the first place. And that gets generated. But the stuff that gets sent up to the cloud gets turned into or transcribed using automatic speech recognition, gets transcribed into text. The text gets put in some kind of parser, so it has to work out which bits mean what, so extracting quote-unquote meaning from that text. Then there's some kind of dialogue management system, some way of deciding, well, we've got this question about, I don't know, what the time is, or what's on my shopping list, or something along those lines. You know, I want to kind of add something to my calendar or whatever it might be, what's the weather.
And then the system has to, the dialogue system has got to decide where to go from there, what kind of response to produce, whether there's going to be further questions or whatever it might be, essentially. Then that gets sent back and turned into speech, and that's what you then hear out of the device. So the important thing to note with those interactions is that they are very much, at the moment at least, they're not incremental at all. By that I mean that they're not detecting things as you talk. Now, in kind of everyday conversation, we do it, in terms of how we're chatting, we're kind of ongoingly monitoring what one another is saying, and perhaps spotting a moment to interject, whatever it might be. That's not what these systems do.
Sean: Sorry, for those of you, yeah, sorry, on the webcam then, I just pretended I was about to start speaking just to throw Stuart. It's because visual cues are also important.
Stuart: It works, it works.
Sean: Yeah, visual cues are important, which don't obviously translate on a podcast that well. But I mean, without anthropomorphising too much, we think of these things as listening to us, right? So the device is recording in some capability, in some manner, and waiting for what we call, as you mentioned, a wake word. So this is like a signal that the stuff that follows that wait word is to be recorded and sent off for processing. Is that right?
Stuart: Yeah, I mean, that's essentially what's happening. And yeah, so it is essentially sitting there waiting, listening, but what it's actually listening to is another matter, I guess or subjective.
Sean: Okay. I think, and we discussed this, as I mentioned in the video that we made, but context is king here, isn't it? You know, we have a real big problem with ambiguity. And there was an example you gave me concerning shopping lists, which it might be worth us explaining for the listeners. We asked the Amazon device about adding something to a shopping list, and it proceeded to explain to us what a shopping list was. It was quite an interesting example of that ambiguity that is a real problem in these devices.
Stuart: Yeah, so in one case, there's a difference between understanding what a shopping list is, I think that was the distinction that we were making, right? So you ask it, you know, what's on my shopping list? Or that's kind of quite different question to what is a shopping list in the first place, although those two things might be jumbled up. So even though we're talking about what is a list or what's on my list, they're actually very, very different things to be doing. I think that's kind of what we discussed.
Sean: It is. And I think, you know, I was just, I was calling to mind things like the old saying, time flies like an arrow and fruit flies like a banana. You know, these are problems for human listeners to decode, and therefore, definitely must be problems for the computers to decode. How do they go about doing that? You mentioned they don't work incrementally. So they're not listening to each word and trying to understand what the branches might be after that. They are taking a chunk of text and trying to interpret it, right?
Stuart: Yeah, I mean, I think this also is about a bigger problem in AI in general. I mean natural language processing is essentially what they're using or sometimes I think it's called or elements of it called, natural language understanding. So they're trying to extract meaning from language that's, in this case, transcribed to text. Now, that's a very, very complicated thing and it's perhaps a bit of a misnomer to call it understanding or meaning that's being extracted.
[00:20:11]
I think really, it's more like, you know, we're matching patterns, essentially, which is a very different thing to be doing than what we are doing when we're actually understanding the meaning of what it is that one is saying or what it is that you're saying. You can say the same word in many different contexts and it means radically different things. I mean, I think that's one of the big issues, you know, as you mentioned, sort of context, what's happening around us. Situatedness of meaning and by that, I mean that things are said in real places at real times and, you know, at real moments. And they are not kind of some abstract set of, you know, language is not an abstract thing. It's always embedded into what we're doing, where we're doing it, when we're doing it, and so on. And these devices, really, at the moment, can't cope with much of that context.
Now, obviously, you can start to kind of develop some elements of them that do deal with context, but it's still super fragile. It's really, really tricky and super fragile. And I think I'd argue, you know, this is kind of a wider problem for, I guess, the AI project in general, which has always been around, you know, the problem has always been around context and what's going on and how much you actually need to know to, in this case, say, produce a response from a device that is appropriate for that context and take into account some of the, you know, elements of the situated meaning, I guess we would say, about that particular moment in time.
You know, I mean, you could sort of think of some examples where saying one thing, you know, well, we've all experienced it, right, where you say one thing in one context, and you feel that other people have taken it out of context, and they misunderstood what it was you were saying, right? And that's just one illustration of kind of how meaning is embedded into those sort of situations. And there's a real problem with how you actually do get a device to even make sense of that or whatever it might be. And the basic problem is to do with language and what we're really doing is designing systems that are doing kind of statistical pattern matching around language. And that has very little to do with the way that we might make sense of what one another is saying, or the meaning of specific kind of words in certain kind of contexts. So it’s a real problem, you know.
Sean: Yeah, I mean, you know, obviously, even tone, never mind dialect, accent, the way we use different words in, you know, in different parts of the globe. The other thing I always thought would be interesting would be how these devices learn how you operate. So for instance, I expected, I have a smart assistant, again, full disclosure, it's not an Amazon powered one, it doesn't really matter. But I have a smart assistant in the kitchen, it's incredibly useful for timers and listening to music. Not very much else in my humble usage. But the point I was going to make is, I have to say the watchword followed by a certain string of words to get it to turn the radio on to the channel that I like. I assumed after a few times of repeatedly doing exactly all of this perfectly, I might be able to slim this down a bit, because maybe the device would learn about what I like. And instead, it's, you know, a couple of years on, and I still have to say all those same words in exactly that same way, or it doesn't work.
Stuart: I think, so I think some people are trying to, I've maybe seen some examples of people trying to develop personalised models. So in the sense, they learn more about you specifically, and how the way you talk. But again, I think you've been misled by the idea of learning and what's being learned. And it's not, again, the sort of sense in which you or I might learn at all. It has no relationship, it's training a model, which is essentially doing a bunch of maths and changing weights, and so on. That's actually happening. And I think, again, the language here is a bit confusing, a bit like the idea of conversing with a device.
So you set up a whole bunch of expectations about how you're going to interact with these sorts of things and the reality is extremely different. You know, we find this in our research that people tend to treat these devices more as kind of, if you think about them more like as a resource for action in the kind of place you're in at the moment. So you can get, you know, you can make it part of a joke with someone else in the room, as well as using it in a very functional way to say, you know, ask what the weather is or whatever it might be. You know, you could use it to make a point in the conversation. There's lots of different things you can do with this.
And equally, you have to design the ways in which you talk to the device. So if you imagine it's a bit like, more like creating input to the device rather than talking with it, if that makes sense. It's a really, it's just like qualitatively different activity to be going on with. And I think a lot of the language around these sorts of devices, and also kind of AI in general, I mean, learning is a good one, it's quite misleading and people misunderstand what is meant by those things. Partly because, again, the nature of language is that you can use the same word to mean different things. So we've got kind of language going on in the discourse of AI itself, confusing things. So we've got a lot going on too. So it's a very complex sort of picture.
Sean: As I understand it, there were some trials in the 1960s, you know, a very long time ago, over 50 years ago, with speech recognition and conversation computing with, was it called Eliza? It was supposed to be a psychologist or something.
Stuart: Yeah.
Sean: Well, tell me about that?
Stuart: It was on a teletype. It was on a teletype.
Sean: Ah, it was on a teletype, right.
Stuart: People would type stuff in. It was a basic programme, I actually forget the person who made it, but you know, it was, yeah, it was supposed to be a psychologist. Eliza was supposed to be a psychologist. It repeats a lot of stuff back to you, asks you questions about how you're feeling and so on and people found this kind of quite disturbing. And then, you know, now we might go, we might look at it and go, oh, those, you know, silly people, they didn't understand, you know, it was all new to them, so they didn't really understand it. But I think actually, it shows a really interesting aspect of how people potentially imagine what these systems will be like, but also equally how they work to make meaning all the time.
In that particular example, the assumption obviously was of some people potentially using it, was a system that was way more complex behind the scenes that really understood, and again, I'm using inverted commas there, understood what was going on and really was parsing what was happening. I mean, this connects with a whole bunch of kind of more academic stuff around Searle's Chinese room arguments, and also kind of this idea about what we'd call the documentary method of interpretation. But I won't go into that in too much detail. But what I will say is in those cases, those people were looking at the responses from the device, or sorry, from the programme, and working out how to fit the meaning of those, sorry, how to create, construct meaning into those things, into the context of their actual responses and the way they were talking to it, you know, in a similar way.
Again, hopefully this doesn't upset anyone who reads horoscopes and is into astrology, but in a similar way to kind of seeing, sort of taking stuff that you read and sort of fitting it to your life and your experiences and thinking, “Well, that's really talking about me, in particular.” It's not a very similar, it's not a dissimilar thing to be doing and of course, we do all this kind of meaning making all the time actively, and you kind of see meaning everywhere. The thing I was referencing in particular with documentary method of interpretation is actually a very easy to understand thing that happens.
So in the kind of 60s, a sociologist called Harold Garfinkel, got some of his students to engage in what he called kind of experimental therapy of some kind. And there was, they had a kind of board where the patient couldn't see the therapist, and the patient would talk to the therapist. And the kind of the twist on this was that the therapist was only able to give yes or no answers, right? I mean, obviously, there are all sorts of ethical minefields going on here, but we'll ignore that for the moment.
So students would, or the subjects, the patients would come in and talk about their problems with this therapist. Now, the key thing, and they couldn't see them, obviously, but they could hear them. The key thing that they did, that Garfinkel did was to ask or give the therapist a list of yes or no answers. And essentially, the patients knew that they could ask, you know, the therapist would only answer yes or no. So they adapted their questions to, or adapted what they were saying to fit, you know, yes or no answers. But the responses that were given by the by the therapist were random, essentially.
And what he found was, and this is what people tend to do is the patients assumed that the answers were meaningful. There's a kind of again, this goes to this idea of trust, actually, about what we mean by trustworthiness and trust in society in general, and you know, you get kind of sensible answers from people and they're meaningful at all times in some way, or people are trying to work towards meaning. So anyway, so in this case, the patients, the thing he found that the patients would work really hard to make sense of answers, sometimes, you know, sometimes they were contradictory.
[00:30:00]
So someone would ask the same kind of question twice, and get a yes, and then a no. Right. So and so the patients were working actively construct meaning around the responses they were they were getting from the, you know, the experimental therapy, as you know, as they were calling it. So a similar kind of thing is happening with the Eliza programme. So it tells you a lot about how people make meaning, it doesn't really tell you much about kind of what people conventionally think of AI as such. So I think the interesting thing about AI and these, you know, voice agents, and whatever it might be, we're using, face recognition, whatever, is that they tell us a lot about people, and how people make meaning in the world.
But equally how systems that we develop, which we attempt to inscribe, sorry, attempt to develop systems that actually understand human meaning, and the construction of human meaning, tend to collapse that meaning into certain kinds, into a kind of set of measurements, right, metrics. So face recognition is a really easy one to think about where a face is converted into a series of measurements. Now, obviously, that's not really what a face is, but that's what the system sees. And equally with voice recognition systems, and, you know, Alexa, or whatever it might be, we're collapsing the way that we make sense of meaning and language or whatever it might be into a set of measurements.
And that core, that's a different thing that we're doing, we're switching from one thing to the other. And if we don't quite realise that things can go awry. And, you know, and obviously, these sorts of systems are used in in the real world, and they do have real world effects. I mean, you know, I'm sure you'll be covering this, but things like bias, and so on, which we should be pretty concerned with, obviously. But the source, the generation, sorry, the origin of those things, those problems is in this kind of conversion to measurement. So meaning is no longer meaning is turned into measurement, essentially.
Sean: So it is a good way of thinking of that, like it's almost turned into, well turned into a symbol in the computer. So all the nuance is thrown away, is that what you mean by the measurement? So you said, I would like to listen to music. So it's right, listen, music, done. Not, you know, I'd like to or-
Stuart: Yeah, I mean, I should, I should make that a bit more sophisticated, I think, for the following reasons, because there's two big strands of AI, which are driving in the case of voice interfaces, quite interestingly, driving two aspects of it. So there's the machine learning, or typically, people tend to really mean deep learning based systems, neural net based systems, that are doing this kind of statistical pattern matching, where and that's kind of on the front end, where we're converting from voice to, you know, a voice signal to actual text. That's where that pattern matching work is going.
Sean: So that's basically, to simplify it, hopefully not too much. It's seen a load of possible options and it's trying to find the one that most likely fits what you've just asked for?
Stuart: Yeah.
Sean: It’s been trained.
Stuart: Yeah, so like other sort of technologies of recognition, other recognition systems, like face recognition, the same kind of thing, you get a tonne of data, you train your model and essentially, what you're doing is then matching the input and saying, how similar is it to the stuff I've already seen? And, you know, and is it this? Is it one category or this other category? And that's what's happening. So in the case of the voice stuff, you know, it's like, is it this word that it matches? Or is it this other word? What's the probability of it being a ‘the’ rather than an ‘a’ or whatever it might be? You know, what's the probability of it being Sean versus Stuart as being the correct output? And so the model is really just working through those probabilities and matching one or the other with a certain level of confidence. And then we get into errors and those sorts of things.
So that's one aspect. That's kind of one, you know, that's the that's what people tend to talk about. Now, when they talk about AI, they mean they tend to mean that stuff. But there's all this other stuff, which is symbolic based AI. So, whereas the pattern matching kind of AI, which the front end of these systems is based on, has no sense of meaning at all, by definition, it's statistical, it's probabilistic. The symbolic side has meaning embedded in it. So this is the dialogue side.
So if you think about a kind of simple example is like a tree, a conversational, you know, conversation tree, a dialogue tree, where we have different options going down, as we have a conversation, there are different kind of branches, you know, this is the way that people think about it. As we have a conversation, there's different branches and ways that we can go through that conversation that unfold and appear as we proceed in the course of our conversation. And if you're designing an agent, a chatbot, or, you know, one a kind of back end for something like Alexa, you need to deal with the user's input. And you might do that through a tree. And there's different, all sorts of different platforms you can use to do this now, it's very easy to go and build your own chatbot, detect sort of intents, and then apply and then kind of work through a kind of a tree of dialogue effectively.
And that's actually not how conversation works at all. But for the purpose of the system, you have to have some kind of, some kind of way of actually engineering this stuff. Now that is symbolic, because it's actually embedding meaning, you know, we understand, we believe we understand what it means when someone says this particular thing, again, that's also problematic for different reasons. But you know, if we say, you know, I want to know what the weather is, we're kind of then mapping those particular symbols to certain other kinds of outcomes that then lead to the response. So really, these systems blend those two major strands of AI together.
And those two major strands of AI is I think, is an active area that a lot of people want to, who work in AI, want to try and fix because they see the value of the stochastic side, the statistical pattern matching side, is that you get tremendous amounts of accuracy compared to previous ways of doing it. Again, computer vision is a good example where you see huge increases in capabilities, the system to do things like image tagging, or whatever it might be, to determine whether you know, what's in an image or what's not in an image. So you get huge increases in that.
Whereas the sort of symbolic side, you can actually control things. So with the statistical side, you can't control a chat. If you have a, if you had an agent, voice agent that was purely based on this kind of statistical pattern matching, I can't say statistical pattern matching, bit like anthropomorphism, statistical pattern matching, if you have an agent that's based on that side of things, you engage in an aimless chat that has no goals, it has no purposes.
Now, obviously, when we talk, we have a goal and a purpose. Again, that's a simplification, I guess, but we might be doing certain actions in our conversation, you know, whether it's kind of greeting people, checking how they are, or trying to kind of negotiate a contract or whatever it might be we're doing, we have certain things we want to achieve.
Sean: Well, we've got agency, actually.
Stuart: Yeah, yeah, yeah. So it is, by definition, what you mean by agency. You can't do that in that using a statistical systems, they're engaging in a kind of a synthesised output, which is kind of synthesised only from the data you get in. So there's this idea of like garbage in garbage out, again, you get like system machine bias, whatever it might be, you know, training on certain images, you know, all that kind of stuff, the discourse at the moment.
Sean: So basically, what you're saying is, well, from a statistical point of view, if you try and make a chatbot based on statistics, it's going to just pick the most likely response to any question, it's not going to pick something that moves the conversation on, or is new information, it will basically say, right, you live in Manchester, you're asking what the weather's like, it's probably raining?
Stuart: Yeah, it's a bit like the Google search where, you know, it's predictive. It's basically based on what other people have put in, and it determines, well, the chances are you're doing this. I mean, you can see the same sort of thing with GPT3 is the latest thing that lots of people lost their minds over. But again, it's like, it's generating a synthesis of text from all the text that it has, right? That's, you seed it, and then it just generates text based on that. There's nothing new in there at all, because all the stuff that's in there is in the model that you've generated from the source data. So it's a similar kind of thing with the voice recognition stuff.
Now, there could be some good applications for a chatbot or some kind of chat system that's based on that statistical side of things. An example I would think of immediately, which is quite famous, I think, is like a help desk scenario where people are asking the same kind of questions, you engage with the chatbot or whatever it is and it gives you kind of, you know, similar responses to the people who are engaging with it before, because you have the same kind of problem. That makes sort of sense.
Now, if you're then taking that from that help desk idea where you're talking about, say, technical problems to a medical context, it's completely different, even though you might end up with the same kind of system. There are lots of different issues obviously there around responsibility, whatever. But anyway, so it's a synthesis of that knowledge. It's a bit like an expert system, but a twist on that. But then, you know, there's this dialogue side, which is more symbolic side, which is goal-directed.
So you can then say, well, I want to achieve this thing. I want to get and enable someone to do a booking of a, I don't know, whatever it is, like a flight or something like that. You can't do that with this kind of other side, this other kind of statistical side. And those two sides are, as far as I know, they're not really, it's difficult to see how you would bridge them very easily.
[00:40:14]
Stuart: So it does pose a bit of an impasse, I guess, for AI, which is, you know, the kind of AI techniques that voice agents bring them together, really.
Sean: I know this is kind of an often trotted out thing, but thinking about those two different parts, why is it that we, do we know that those things are not inside the device in our homes, and that it's listening and making sense of what we're doing? How do we know that's not listening all the time to anthropomorphise once more?
Stuart: So that is a complicated question, because I'm now going to now going to kind of disagree with some of the things I potentially said earlier. So the device we have, because we’ll need to start talking about the back end of these systems and how things are actually detected in the first place. So I don't know if you've seen, but, you know, not too long ago, there was quite a few news articles about things like, you know, people's recordings, audio clips from their device being shared with other people accidentally. Or there were also some articles about how the back end of these sorts of devices, on the back end of these sorts of devices, like Alexa, or I think it was, I think it's Google's voice assistant or voice transcription services, there's a whole load of people who are listening to clips that the machine learning system, the deep learning system can't actually do the transcription of, and they have to do the transcription to train the machines.
There's a whole army of workers, potentially. Again, I know I saw some articles where there are questions about the kind of labour issues around those workers. And then there's kind of issues around responsibility and building the systems and so on. But it's not simply the case that these, the devices don't quote unquote, listen to you, in the sense that you may well have something that's un-transcribable by the system, by the current system as it sounds, actually being listened to and transcribed by a transcriber, so that the machine learning model can actually learn successfully how to deal with that kind of query in future.
And it's not, again, that's a real simplification, because it's more about how the model is shaped generally. So this idea of people, these devices not listening to us is kind of complex, it's a bit vexed, I would say, because it may well be that people are listening to what you just said, because the system, the current model couldn't work it out, and some transcriber, it got sent to some transcriber who had to transcribe it, who understands language successfully, and writes down, well, this is what was said, and then it goes back into the system and helps train the model. So that's a more likely scenario. Obviously, you can put your tinfoil hat on and think about security services, etc. But that's not my expertise.
Sean: Well, I was going to say, that's the sort of thing that brings, I suppose, the, if you like, must have said this in every podcast so far, the clickbait towards the kind of trustworthy, autonomous systems come together, which is the people saying, “Oh, it's a spy in your home.” You know, how does AI like this escape those negative connotations? Is it possible that it can get away from that? Or is that just baked in? People just have to say that these things might be doing that, get on with it.
Stuart: Yeah, I don't know. I don't have a very good answer to that. I mean, it's clear that more and more devices that we have in our home have the increasing potential for being invasive. I mean, think about video cameras, as well as audio recordings. So that's clearly kind of concerning. I think, yeah, it depends on what we think the purposes of this capture is for. There are obviously, there are, you know, examples of where data that's collected is being used for things we might not want it to be used for. So do we know what's actually happening to our data?
Do we really want the data that was collected from, I don't know, our Facebook profile, whatever, used in a way that is related to law enforcement? Who knows? Similarly, do we want voice, which is captured and stored in, essentially stored in models in some way or other, to be used to essentially make money for, say, transcription services, which could be used for all sorts of things. There's so much complexity to that question and how we think about how we regulate and manage these sorts of systems and where our data is going and so on. So I think it’s a really tricky and complicated thing.
Sean: Would it be simpler to think about the motivations of the companies that make these devices? I mean, is that something that's straightforward to fathom?
Stuart: Not really, not necessarily.
Sean: Maybe that's an unfair question.
Stuart: Yeah, well, I mean, I've got my own views, okay, about what's actually happening, but that's, I'm not sure they're necessarily relevant. I mean, I think one thing that’s obvious is that, to me at least anyway, is that it's clearly useful. I think with all these voice interface devices, probably what's more useful than the device itself is the transcription service, because the transcription service and having the best kind of most effective transcription service-
Sean: Do you mean for the businesses that are making them rather than for us?
Stuart: Yeah, yeah. So the most effective transcription service opens up a whole load of doors in terms of reselling those services to lots and lots of companies that want to write, understandably, in many cases, want to use automatic transcription all the time. So I think that's really what part of the game actually is. As to what goes beyond that, I don't quite know.
Sean: Well, with Amazon turning their latest home security into a flying ring security drone, if people haven't seen this, they should check it out. It's quite crazy. That's an interesting kind of future. What do you think the future holds for these devices? Are they going to keep just getting better at understanding or?
Stuart: I don't know. I think two, I guess I'm in two different minds, well, in more than two minds, multiple minds about it. I guess I can imagine a future where they don't really get, they get better but no more useful. I think there are big questions over how you actually interact with them. If they're going to be another kind of input device, which they are essentially, they need to be, to find an actual use, a kind of killer app or whatever it might be and I don't think that's really been found yet at all. On the other hand, there are potential communities of users who could benefit from them and there might be more interest in them. I mean, I'm thinking particularly about people who have, say, have visual impairments. I've got a PhD student who's investigating stuff to do with this. There are some potential benefits there for some certain groups of users. But again, I'm not sure whether those benefits for those people actually align with the interests of the companies making the systems in the first place.
Sean: We're probably still at the stage, so, I remember when the smartphone, kind of iPhone revolution happened 10 or 12 or whatever years ago and everybody's walking around with these apps that mimicked a pint of beer being drunk or a Zippo lighter being lit or, it was all the novelty things. The smartphone hadn't found its kind of proper use yet and it wasn't ubiquitous. Perhaps that's the stage we're at with these. I don't know. What do you think?
Stuart: Yeah, I think it's, so I think the smartphone is probably one of the, in hindsight is one of the biggest developments, really the biggest development. I think everything and probably since the I guess since, the internet, since the PC, it's one of the biggest developments. And with any new technology, people always say the same thing, which is they always do what you've done, not that I'm wishing to knock you, which is compare it to those key technologies, which really have changed quite a lot of stuff in terms of, and by change, I don't mean- by change, I mean actually change the way people do things in life, not just a tweak. Something like VR is a good example of something that looks a bit like a tweak to how things are. It's just another kind of way of kind of being able to view and interact with things.
But it's not paradigm shifting. Whereas I think the smartphone has been, and it's clear that that's the case, enabling new kinds of practices. For voice, I think at the moment, at least, it seems just like another kind of form of interaction, a bit like a fancy mouse but maybe it'll go beyond that. I mean, you know, you could argue the mouse would be very important.
Sean: Absolutely. But also just to knock that right back at you, you might argue that the smartphone thing was just the touchscreen, actually, because there were smartphones before the iPhone. What changed there was the input device of being a touchscreen and swipe and that ability to do that. And as we gain the ability to tell our smartphones what to do, perhaps, you know, maybe I'll be vindicated and voice is going to be, you know, the new black.
[00:50:00]
Stuart: Yeah, it could be. I can see how it could definitely get a lot more useful and find more uses. And maybe it's a case that you need another wave of it. I mean, you see this with certain kinds of technology. I guess the smartphone is a good example where you did have smartphones before the iPhone and other more modern smartphones.
Sean: Clunky as they were.
Stuart: So there was a wave of them. There was certainly an existence, as a wave of them, but someone needs to look at them and start from scratch again. So maybe you find that with voice as well. I mean, I can think of a load of uses for it, but where you really want to be hands-free or, as I said, uses that are for people who have, say, visual impairments or whatever it might be that might find specific. But again, it's like, I think, again, I think it depends on the way that these companies that are producing these systems actually think about those things and turn them into markets, whatever it might be. I don't think that's necessarily how things always work in the world.
So yeah, so I guess I feel a bit ambiguous about the future. I don't think it's completely hyped up without some reason, but at the same time, it doesn't feel, well, I'm hesitant to make any predictions.
Sean: Could be a quiet revolution rather than the next 3D holographic TV, which will just quietly die into the background.
Stuart: Yeah, not quite that level. The potential for these devices is not really in the front end from the user facing point of view. It's probably more in the transcription service point of view, because there's so many, so many ways that so many people would be interested in or want to do things like transcription for all sorts of purposes. That, to me, is the bigger thing where more and more audio, more and more content is kind of turned into text based media. So that seems like a big deal to me, much more. But that's quite hidden, again, sort of relies on this kind of behind the scenes stuff.
Sean: Yeah, because I mean, things like YouTube captioning is getting better. They can imagine, you know, even from a kind of, you know, tinfoil hat back on but from a spying point of view, if you could turn all the world's conversations into a text that's searchable, you know, your job is going to be a lot easier, isn't it? To be, you know- right, tinfoil hat resolutely taken off now. Stuart, I'd like to say thank you for joining us on the Living with AI podcast and yeah, we'll speak again another time.
Stuart: It was great speaking with you. Thanks.
Sean: Stuart and I, I think, did quite a good job there of not mentioning the A word too often. I don't know, maybe we did. What do you think, Paurav, any hints for us about how to not mention that word again?
Paurav: How I wish, but one of the ways it comes to my mind is that the amazing device that should not be named would be a very, very wonderful way to actually shoot it out to the world. We should be pioneers who are saying that this is the way how we should mention it.
Sean: And I don't know the exact numbers, but I did read somewhere that the name, I'm going to say it now, Alexa has really dropped out of popularity for babies. People are not calling their child by that name. And it shouldn't be that hard, though, because there might be quite a lot of people with that name existing and they are probably really quite cross with Amazon at this moment. You know, I mean, are wake words, the only way to do this, maybe Christine could answer that. Is there a better way to do this than wake words from a machine listening point of view? How would you sort of target that your robot needs to listen to what you're saying?
Christine: Well, the way that I see it, largely, you've got two options. You can either have a machine consistently listening to you, or you can make the machine aware that you're about to insert a command by having wake words. And the question about that really is the question of how much of your personal information are you willing to share with a machine, where it's not entirely transparent how that data is being processed. So that's, in my opinion, why wake words are extremely helpful.
On the other hand, of course, then requires that you need to find a wake word. And that actually in human dialogue, it's not very natural to use wake words. I mean, if I, if I perceive you as not, not actually paying attention, I will probably say, “Sean, what do you think?” But actually, in a very natural dialogue, I wouldn't say in every sentence. “So Sean, what's your thoughts?” “So Sean, how would you like to proceed?” So it's not a very natural way of communication for us.
Sean: And I suppose then it comes down to choosing the right wake word, right? One with more syllables is going to be less common to come up in normal conversation. Yet at the same time, you don't have to say a lot of syllables to activate a machine?
Joel: Yeah, so it's quite an interesting challenge and one that's being looked at in sort of voice interface community. So what one of the interesting ideas is to replace the wake word with glances. So to be able to detect if I'm glancing at the device, because glancing is a very natural way for humans to initiate conversations, and to address other humans. Of course, then, though, to be able to pick up glances, this would mean equipping the device with probably a camera, or some kind of other visual and optical device that is able to detect the glance, which raises all sorts of other potential privacy concerns of course, you can imagine the kinds of nightmares that could ensue from that.
Sean: Perhaps we need to get towards Star Trek where you press a button or flip open your flip phone. Christine?
Christine: Yeah, and I think picking up from what Joel said about the glances, A, as humans, we seem to perceive video as more invasive than audio, which is an interesting phenomenon in the first place. But B, there's also the problem of power and energy consumption of the device. So if you want to detect glances, which are very, very short lived gestures, you need a camera of extremely high frame rate, or extremely high rate, but sufficiently high frame rate, which also means that you need to process that data at that frame rate in order to actually have real time interaction. So you suddenly venture into a whole different depth of AI than simply having something that can sit there and listen, and just look out for that keyword. It becomes very complex at that stage.
Sean: I know one thing that we discussed before we heard from Stuart, which was the type of voice that the assistants have and I know Joel mentioned that, you know, they're predominantly female voices and we discussed the idea of perhaps having a childlike voice before we heard from Stuart. One thing that we talked about, Stuart and I briefly was the idea of Jarvis, this kind of like fictional AI in the Iron Man films, which is a very, obviously a male voice. And I think the idea is it's modelled on the kind of quintessential British Butler who does everything perfectly, accomplishes things, he'll or he again, he would handle it. There's no problem there. I mean, I wonder why the tech companies haven't gone down that route?
Paurav: I think it's possibly because it's easier for them, I think. It's just simple as that. You know, the herd mentality remains in the technology world also, if you see that one company, you know, it's something others follow suit very, very quickly. Once a female voice took shape, it's just been largely that and possibly it is the human way of creating a little softer version of AI. Psychologically, we would find that a little more appealing compared to a male voice, which may be sounding more authoritative rather than friendly in some cases. And so to make this family friendly devices, probably they have possibly chosen this type of a tone and voice.
Sean: I know that when a few years ago, sat-nav devices specific kind of like turnkey sat-nav devices such as TomTom were very big, and you could buy or certainly download different voices. And you could have comedic voices or famous voices and all sorts of different things. I wonder if that's next for the AI world or whether the corporations want to try and use these voices as a bit of branding, a branding exercise?
Paurav: Yeah, I think that is something also, that in terms of we need to, these are such a new experiences for millions of people so consistency is a very important thing. And I think a voice which sounds neutral enough, friendly enough, that makes people feel that this is a helpful voice would make it a far stronger impact in terms of usage patterns. So that's why we saw TomTom again. Most people bought, I have bought some of those voices also in past. And including the Queen Mother's voice and Star Trek voice and Spock’ voice and whatnot. And most of them did not last for more than two days of their fancy and novelty. And then after we came back to the same default voice because we were used to hearing that. And so that consistency, I think is a very powerful part of this branding experience of any of these big corporations.
[00:59:57]
I would give you a possibly different example, if you look at newspaper websites, what you tend to see is each newspaper has its own fonts. And we are so used to them that's why when we try to read another newspaper, we don't find it very comfortable. So if you go to New York Times website, and if you go to Guardian's website, you will like one font more than the other and then you're stuck with it.
Christine: I think what I would be interested about is how the companies are actually choosing the voices and how much research or market research is going into the perceived acceptance by human listeners into the selected choices. Because from a technical perspective, the models that are used to actually generate these voices could generate in principle any voice, they could take your voice and regenerate your voice with different words in principle. So, it'd be interesting to see how the voices are exactly selected and what sort of personal data, well not personal data but what data is used in order to make that decision.
Joel: Yeah, just on that, so I am aware of some earlier research that found that people tend to prefer female voices because they find them potentially more agreeable and more pleasant. And I think that's also been confirmed by some of the market research that has been cited by some of the companies designing these voices. But the other thing that I want to mention is that this sort of gendering that you're doing with the voice is also then embedding gender stereotypes. And there was an interesting report that came out last year by UNESCO. It was entitled, “I'd blush if I could.” Maybe you remember it? It was sort of in the news at the time when it came out. And “I'd blush if I could” was a response that Siri gave when you said something rude to it.
What the report does is it criticizes this female servant approach to gendering voices and this sort of idea that a personal assistant and often a personal assistant is associated, again, as a stereotype, that that's an occupation associated more with female. So, yeah, I mean, you've got to think about the choices you make. I mean, on the one hand side, maybe people prefer the voice. But on the other hand side, what are the responses you are inviting by doing that? And there's some perhaps more anecdotal evidence that it does invite some rather nasty language by users to it. And again, it's sort of what this might set a bad example to some people around it.
And just a final point, there's also been some initiatives to create gender neutral voices, artificial voices, synthetic voices that are gender neutral, like the Voice Cube by an initiative called Equal AI.
Sean: So leaving aside the idea of the voice and its gendering for a moment, one of the things that is clearly a problem with these devices is ambiguity and how do you get these devices to know what state emotionally you're in or what you intend? I mean, intent is coded into tone of voice, right, in a lot of cases. Christine, is there a way of getting these devices to guess your emotion, at least?
Christine: Yes, there is. And before I give an answer to that, I think my question would be, is that really what you want to do? And are we fully aware of the implication of what it means when a machine can gauge your emotion and your psychological state? In response to your answer, there's, of course, a lot of information about your emotion in the choice of words that you use and in the intonation that you use in order to express those words. So you can use both to actually do emotion recognition and get a fairly accurate gauge of whether a person is angry, happy, or sad.
But as I said, the problem that is actually associated with that is where we go from here, if we deploy emotion recognition on automated systems? And I think that's a very dangerous route to go down on, especially if you deploy it on, say, surveillance systems, where in a supermarket or in a large crowd, people are being observed, perhaps in a manner that they are not even aware of. And there's almost this sort of God-like being that is sat in the background observing the situation and gauging what emotion we're expressing and potentially even making decisions based on our emotion on how to act next.
Sean: Be careful what you wish for then. Paurav?
Paurav: But this is very interesting, Christine, from what you just said. In one sense, we are all concerned about this privacy. Very recently, I teach a very large course right now, and I put in a question to some of my undergraduate first-year students saying that, “Are you worried about these cookies? And are you worried about this privacy?” And they said, “Who cares? If I get the answer, I'm fine.” And when I think about it from a perspective, right now I'm wearing a smartwatch, and that smartwatch is measuring some very intimate data about me in terms of my heart rate, my breathing cycles, and this, that, and everything.
When I think about it, that as a fairly privacy-conscious person, I'm still ready to give away so much of that information. You know, isn't this possibly the next logical step? And especially with how these young people are thinking, you know, while we are asking that question, they possibly don't care. So that is something. But at the same time, when we think about these moods and emotions, could it not be that these devices right now, which are fundamentally, as we talked earlier, are becoming more of music speakers for us, could actually become far more important devices?
For example, for older consumers, you know, by understanding their voice patterns and possibly understanding their anxiety, the device could connect with some sort of relative or somebody who can then possibly answer the query or can understand something's going wrong. So, that is, there is some fantastic scope but I think you're absolutely right in terms of that, that privacy issue, that how much are we ready to give up to get more?
Sean: And it sometimes comes down to who's in control. Christian, I'll come to you in a sec. I think Joel put his hand up before, you got something to say, Joel?
Joel: Yeah, I just, I think I wanted to speak a little bit about emotion recognition. And I think one of the things that always sticks in my head about emotion is one of the first lessons in psychology I've had, is that impression isn't the same as expression. And that when you are, you know, seemingly detecting someone's emotion, that is an impression of someone's emotion, that is not their expression. And I think that's really quite important to distinguish those. And you might, you might be able to try and guess whether someone is angry or sad but again, it's just an impression that you have and it's not necessarily the truth. And I think that's an important lesson. And it's true for any kind of recognition that you are trying to do with machines. You know, it is a classification and classifications can have errors and can be wrong.
Sean: And of course, you don't know the motivation, they may be angry, but not necessarily with you. They may just have had an argument in the parking lot about getting a space and you just happen to be on the tail end of that.
Christine: Yeah, and actually picking up on that, the ambiguity isn't just down to only the impression versus expression part, which is, of course, a very, very important part. But again, there's that level of uncertainty. What if someone is speaking with an accent that that machine has never listened to before? Their accent might be because naturally, a particular language has a different toneation to it than perhaps language that it's listening to. And that might actually introduce another level of ambiguity that may lead to decisions that are simply not adequate for that particular scenario.
So ultimately, I think that the problem really comes down to how can we actually regulate this? How can we make sure that perhaps applications such as emotion recognition are actually deployed or can be deployed in scenarios like power of mentioned and healthcare, where it might be ultimately helpful to actually gauge how a person is feeling and if there's a certain stress level involved, so that help can be called for. But for decisions that may have legal implications, or may actually infringe on our personal privacy, that we regulate that data appropriately.
Sean: I think all of this ambiguity and the emotions and everything, all has to sort of sit to one side when we think that the they're not capable of this at the moment and yet they still are in our homes and listening to most of the things that we say, albeit with wake words or whatever. Just what are the implications of this kind of from a privacy point of view of having a speaker with a microphone on it sitting in your home, potentially recording anything?
Joel: Yeah, so I'm sure many of us have read the sort of newspaper articles and reports about issues around privacy intrusion, stemming from the recording of voice snippets from our homes, and then contractors who are working for the big companies, actually listening to those snippets of voice. Actually, it's interesting how this is sort of portrayed often as a breach of practice. But actually, I would say that it's part and parcel of the practice of creating voice interfaces, is that you are training the device and retraining it and making it sort of better by using more and more data over time.
[01:10:15]
And what's missing, though, is the transparency behind this practice and actually informing the users, informing us, the users of these smart speakers, that this is part of the practice, that in fact, some of the recordings, some of the interactions with these devices are being listened to by employees and contractors of the makers of these smart speakers, to improve the service and to improve the quality. That's actually how it works. I think part of the problem is that these devices are marketed as these magical devices that just always work. I think connecting to what we said earlier about this sort of, the voice that seems so perfect, and it should be so capable of understanding everything, but really, it's not and it's learning and there's a lot of human labour behind how it's actually working and how it's learning. And I think that's part of the problem is that we haven't got enough transparency and public dialogue around how these things actually work.
Sean: Is this because it's big tech doing this and not a governmental agency? I mean, is this you know-
Joel: I think so.
Sean: They’re beholden to no one, they are, as you say, making commercial products, we're buying them, they're embedding them in phones. I mean, my phone now has the ability to listen to what I'm saying, wait for a wake word and try and do something even if I don't want it to, unless I switch it off.
Paurav: It is, it is certainly a big tech issue, no doubt about it, Sean. But at the same time, I am also fascinated by the whole idea of the trust in this brands. If a particularly not so known brand was trying to sell you the same device, would you buy that? And possibly so, in some sense, when you think about it, some of these big brands starting with A's and G's and whatnot, you know, when they are selling us the smart speakers or the smart devices, as they are called, which are largely mostly listening devices, not really as smart in as their own capacity. But when you think about them, it's the brand's trust being transferred onto this device and that's why we are trusting it to do the right thing. However, how far right thinks it is doing remains a big question.
Joel: I think what's interesting, though, is that that trust can then be undermined by these stories coming out about privacy intrusions. I also think big tech is also learning lessons as time goes by, you would hope so at least, and it's seemingly is trying to address some of the shortcomings. And actually, you know, the question, I think it's interesting to think about, well, how might this sort of damaged trust be restored, or improved in terms of the practices and transparency that these companies might actually, yeah, provide their customers with.
Sean: You may have one of these devices in your in your home, I'm going to say all the names now, Alexa, Siri, Google Home, whatever. Hopefully, you know a little bit more about how it works now, and can perhaps think about the privacy implications. Anyway, Joel, Christine and Paurav, thank you for joining us again on another episode of Living with AI and hopefully we'll see you again very soon.
Joel: Thanks for having us, Sean.
Paurav: Goodbye.
Christine: Thank you very much.
Sean: If you want to get in touch with us here at the Living with AI podcast, you can visit the TAS website at www.tas.ac.uk, where you can also find out more about the Trustworthy Autonomous Systems Hub. The Living with AI podcast is a production of the Trustworthy Autonomous Systems Hub. Audio engineering was by Boardie Limited, and it was presented by me, Sean Riley. Subscribe to us wherever you get your podcasts from, and we hope to see you again soon.
[01:14:10]