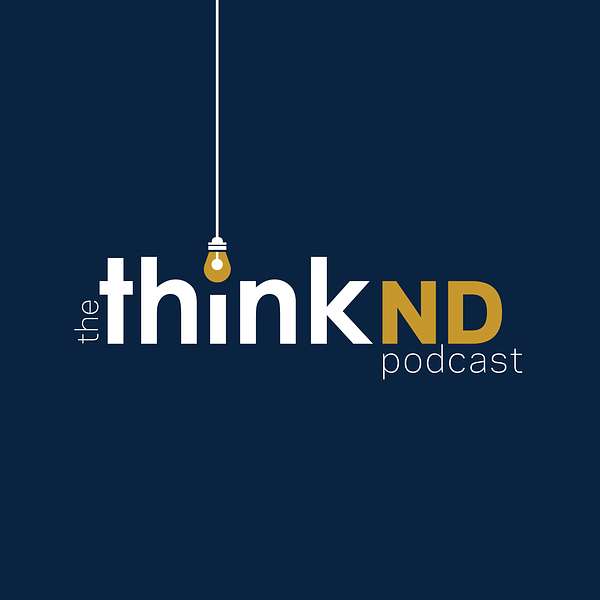
The ThinkND Podcast
The ThinkND Podcast
Ten Years Hence Artificial Intelligence Promise and Peril, Part 2: Autonomous Vehicles for Micro-Mobility
Join us for an insightful virtual event, "Autonomous Vehicles for Micro-Mobility," featuring renowned speaker Henrik I. Christensen, Qualcomm Chancellor’s Professor of Robot Systems and Distinguished Professor of Computer Science and Engineering at UC San Diego. Professor Christensen will explore the transformative potential of autonomous vehicles in the realm of micro-mobility, addressing advancements, challenges, and implications for urban transportation. Gain valuable insights into the future of mobility, including innovations in autonomous technology and their impact on accessibility, sustainability, and urban planning. Don't miss this opportunity to delve into the exciting possibilities of autonomous vehicles in shaping the future of micro-mobility.
Thanks for listening! The ThinkND Podcast is brought to you by ThinkND, the University of Notre Dame's online learning community. We connect you with videos, podcasts, articles, courses, and other resources to inspire minds and spark conversations on topics that matter to you — everything from faith and politics, to science, technology, and your career.
- Learn more about ThinkND and register for upcoming live events at think.nd.edu.
- Join our LinkedIn community for updates, episode clips, and more.
All right, why don't we get started. For those of you I don't know, my name is Brian Levy. I'm on the faculty here in Mendoza along with my colleague, Jim O'Rourke, who sadly could not be with us today, but we're all here and this is 10 years hence. Our focus moves this morning from AI for social good. to Autonomous Vehicles for Micromobility. Our speaker is Henrik Christensen, the Qualcomm Professor of Robotic Systems and Distinguished Professor of Computer Science and Engineering at the University of California, San Diego. He's also the Director of Contextual Robotics Institute at UCSD. His research is focused on robotics, computer vision, and AI. Professor Christensen has published more than 40 400 contributions across these fields. That's a lot. Okay, he's also an entrepreneur and has founded, wait for it, six companies. in the investment world he co founded RoboGlobal that manages more than 3 billion in investments across robotics, AI, and healthcare. Professor Christensen is a fellow of the American Association for the Advancement of Science, the Institute of Electrical and Electronic Engineers, and has served on many editorial boards. He is the, and we were just chatting about this offline, he is the principal editor of the U. S. National Robotics Roadmap that is presented periodically to Congress. we're grateful to Professor Christensen for sharing, all of this experience with us today. But we are especially grateful that he has traveled from San Diego to South Bend in February to be with us here today. Ladies and gentlemen, please join me in welcoming Professor Christensen.
Henrik I. Christensen:Hey, thank you very much for the introduction. thank you for inviting me. to come and talk about, things that I'm very passionate about. I'm always sort of trying to figure out where's the next technology going and what can we do? but I should say, you know, even though I'm going to talk sort of about a broad set of topics here, The real credit should really go to these people. you know, I'm just their ambassador. and they do all the cool stuff. so these are the students that I work with, at UCSD on building sort of smart mobility and a number of other things. I'm gonna try and start out talking a little bit about sort of megatrends. given that this is a business school, I'm gonna try and give you sort of my view of where do I see the world going, And what are some of the things that are sort of impacting? Why are we talking about micro mobility in the first place? So the megatrends, if we sort of go back, it used to be that, you know, it was all about owning the best resources. It was sort of about, so if you look at it, you know, the You know, the steel factories were sort of, they owned the resource, if you don't talk to me, you're not going to be able to do anything in manufacturing because I own this. GM, you know, was sort of the competitive advantage of, they had the biggest factories, we were doing all of this. And so it was very much about, if you own the resources, you were in control. That's not true anymore. So now, you know, we have a new economy where it's the Google and the Amazons that are sort of, it's about orchestrating resources. So if you look at Uber, Uber doesn't own a car, but it owns a way of organizing your resources in such a way that you can do it. So there's a lot of these companies today that are very powerful that can do this. And at the same time, if you're really smart about this, you can sort of become a very important player. So if you look at it Amazon, today, actually, they still make money on the warehouses, but most of the money they actually make on AWS, the computing resources. And they're very devious about it. They basically give away their interfaces, the APIs, so Application Programming Interfaces, for free. So if you're a startup, you go into, oh cool, I can get, I don't have to pay a license up front for my software, anything like that. You get in, you develop your system, and then you start selling it, and Amazon will jump in. You are aware, you're gonna have to pay us, you know, 10 cents on the dollar to be able to use our resources to be able to do this. So we, you get, you got started for free, you got into the garden, you started doing this, and then they kick in. Today. Sort of, if you look at sort of enterprise APIs, Amazon owns 40%. So this is more, you know, I would have guessed Siemens or Microsoft or Google even. no, it's Amazon. So what we're seeing is this is a new economy. We're going away, so can we do orchestration? So it's about how do you build these sort of very large networks and use it in a way that's sort of different from this. The other thing we're getting to is sort of mass customization. you know, everybody wants a pair of sneakers. They should be orange with yellow writing on it. With blue, sort of, you know, ties and all of this. so we want to be able to go in on the website, customize it in such a way that it's exactly mine. And then the next thing is, of course, I want them now. Don't say, yeah, you can have them in three weeks. People are like, no. I want my sneakers, and preferably I want them now. The same thing, you look at some of that BMW X Series cars, they're all made in Spartanburg, South Carolina. It's available in four million different configurations. Different seats, different audio systems, different color seatbelts, different sort of, all of this. We all want this sort of highly customized. I don't want to drive a car and say, oh, that's the same as, you know, Kevin's. Or, no, I want my car. And still, not so long ago I sold one of my companies and wanted to go and buy a really nice car. And I went into a car dealership and said, yeah, six months from now, I'm like, no. I need to drive out of here tomorrow in this car. And this is the new economy. And in some sense, if we think about it, it's almost where in an ideal world, you would buy something like a customized pair of sneakers. It would be driven around in an autonomous driving UPS truck with a 3D printer in the back. So you order it and it gets 3D printed on its way to your front door and, you know, in an hour you would have what you need. That's sort of an economy where, you know, it's on demand, manufactured on the spot for what you need. That's what people want today. We're not sort of, you know, when I order something on Amazon, I'm like, I need it on Prime, and I'm like, am I going to get it today? Wait a minute, is it coming now? This is the new economy. That's what we have to sort of think about. How do we build systems that can do this? The bad news is, post COVID We're sort of getting to a point where, so the orange curve is, This is upside. The orange curve is opening. It's sort of, the. it's labeled incorrectly here. The orange curve is em is openings and the blue curve is unemployment. So if you look at it during covid, a lot of people got laid off sort of short term and then they came back, but it never came back to the same. There's a lot of people that sort of say, yeah, you know, I don't really wanna do this. So if you look at the job pressure today for every 10 openings. There are only seven people on the work market that are ready to take up new jobs. So there's 30 percent of jobs we cannot fill. so that poses a huge challenge for us to sort of figure out. We have to figure out if we want to grow the economy. It's great that people in D. C. says, We will grow the economy. We don't have the people. So you can scream and shout as much as you like. It's not happening in the short term. and we're also seeing a change that people are much more in sort of the geek economy. We want to do a little bit of Uber. We want to do a little bit of, you know, Starbucks. We don't want to do when we feel like it. And that's a different economy and that makes sort of life very hard. The other thing is that we're still doing urbanization. Even though, you know, people said after COVID people left the big cities. Yeah, for 12 months, and then they came back. Because, you know, there's not a Starbucks nearby if you're in the middle of Montana or something like that. So people want all of these other things. So today, 85 percent of Americans live in urban environments, and we expect they will be 10 years out, above 90. you know, 10 percent will live out in the middle of nowhere, but 90 percent will do, which is good news and bad news. The bad news is that transportation is a pain. And the other one is, if you want something delivered quickly, it's not that easy to do. Amazon in Atlanta, used to live in Atlanta, would sort of guarantee that you can get one hour delivery services. You can't drive from East Atlanta to West Atlanta in an hour. It's just not possible. So you need to put out warehouses and all of this very close to the customers. It's the only way you can do it. so we're seeing this with, and we're seeing sort of public transportation. People are saying you can do public transportation, in Chicago. It's a pretty good system. In Atlanta, there are two public transportation systems. Sort of trolley lines. There's one north south and there's one going east west. For three million people, that's not quite going to cut it. and they've sort of, if you go and look at the bus schedule and the trolley schedules, it seems that they've tried to set them up to have minimum correlation. You know, it's maximally difficult to go from A to B just because, you know, why would we want to make it easy on people? so it's great that people say, what if we could do sort of public transportation and you don't need a car anymore? You better have patience and bring along an iPad or something like that because it's going to take a while. but the other thing that's happening is that because we're moving out these warehouses, and because we're, we have this sort of now economy, we're now getting to a point where from a logistics point of view, you do have these warehouses all the way out, and they will do deliveries. So if you look at something like, let's say that you have a Dell laptop and you want it repaired, it doesn't go to Dell anymore. It goes to a DHL warehouse nearby and somebody will repair it and get it back to you. So you can say, man, Dell was really good. I handed in my laptop and five hours later I had my laptop back. Dell was not involved. it was DHL. The same, you know, if you go, we just did sort of a test for, For beauty products. So if you buy Lancome or something like that, it doesn't come from Lancome, it comes from DHL. It comes from a local warehouse. You order your products on the website, it goes to DHL, they put it in a box, and they deliver it to you, and you can say, Lancome is amazing at doing shipping. No, they're not. UBS, DHL, and these sort of big companies will do all of this for you. But that implies that we now need to be able to do very good at doing sort of prediction of who needs what. And in some sense, we need to be very good at doing analytics on what do you want when. And just to give you an example, Coca Cola changes their appearance of their product 18 times a year. Super Bowl, Thanksgiving, Easter, all of this. So 18 times a year we change it. So that implies we have to be good enough at producing them just in the need. Because you know. Five days after Superbowl. You're not going to buy Superbowl, sort of commercial Coca Cola. You're going to look at it and say, what's wrong with these people? for that reason, we need to be incredibly good at doing diagnostics all the time so that we can predict exactly what do you need now. The same, you know, we sort of know from logistics, what do you buy on Mondays? Toilet paper, kitchen towels, those kinds of things. What do you buy on Fridays? And that, you know, oh, we're going to have a party this weekend, so it's a different set of products. We need to be able to all the way down to, maybe not in hours, but at least in days, we can predict what are you going to need when. so that's the new economy. We have to be this extremely agile. And even for warehouses, there is 250, 000 jobs we cannot fill. We just don't have the people for it. In warehousing, one of the challenges we have is that most people only stick around for four months. You know, you don't want to do this. if you're in warehousing, the expected weight of things that you have to move around is 55 pounds. And most of these people are supposed to do sort of at least one lift a minute. How many of you have lifted 55 pounds, 60 times an hour for 8 hours a day? That's a serious workout. You know, that, you know, most of us would say, Yeah, okay, I'm gonna go and do something else. it will wear down your elbows, your, shoulders, your, You're gonna do, most of these people also walk 10 miles a day. That's more than 10, 000 steps. That's a serious workout. So people will say, Yeah, I don't want to do this. So you go and you do this for for, you know, for four months. And then they say, no, I want to go and do something else. So it's a challenge for us. We need to figure out how can we use technology to make this accessible. And have sort of a much more robust workforce for this. my title said something about micro mobility. so what is micro mobility? Micro, any suggestions? UAVs? Okay. no. It's a part of it. Doing this, yeah. so in general we would sort of say, it's things like this. Where, you know, Amazon has built about 50, 000 of these. That will sort of go the last mile to go this. If we try to formalize it a little bit, we would sort of say 0 to 5 miles is micromobility. So it's going around. Can we build sort of things where short distances, this is 60 percent of traffic. So Micron Builds is sometimes when you go from one class to another class. It will sort of do this transportation back and forth. 5 to 15 miles is ride hailing. This is where you get sort of the Lyft, the Ubers, the, these kinds of cars. And then long distance is more than 15 miles, which is only 15 percent of sort of transportation in the U. S. Most of the time we just want to go from A to B. Micron Builds is also UAVs, you know, can we do sort of flying of this? And how can we build in these kinds of transportation? So we've done, so Amazon has tested as an example drones for flying short distances. a few years ago I was at a meeting with Jeff Bezos and we'd forgotten our sort of sunscreen. And a drone delivered a sunscreen to us in the middle of freaking nowhere. so why would you have somebody go back if you can just have a drone drop it off in the middle of the forest? so we're getting there, that we will actually get this kind of sort of mobility. If, if you go to San Diego, if you go to San Diego, just south of San Diego, sort of between San Diego and the Mexican border, is a small city called Tula Vista. In Tula Vista, if you call 9 1 1, they're gonna send a drone. So they know where you called from. They're going to send a drone, and then they're going to look down on this and say, What is an appropriate response? Is it a police cruiser? Is it a fire truck? Is it an ambulance? Rather than sort of sending the gamut. Then, you know, somebody says, Oh, it's really bad. It's fire everywhere. let's go look at it. So they do. Sort of between 25 and 50 drones mission every day, where they fly sort of very short distances, look at it, and based on this tell the 911 operator what is an appropriate response, which is pretty cool. That, you know, it's sort of a way of using your resources the right way. No, they send it there on top of the of the police headquarter. and so the testing in Tula Vista is, that you can do beyond line of sight. Normally, if you deploy a drone, you can only do a drone line of sight. There has to be an operator that can have a look at it and saying, is it doing something unexpected? And being, so Tula Vista is a relatively small community, but if you look at it, if you ever, If you're sort of into flying, if you bring up the FAA map for San Diego, you're gonna be, this is the worst possible place. Because it has, you know, the, they've decided to put the airport in the middle of the city, which is sort of not the best place to have an airport. But they also have the largest US military presence in the world. so there is the Navy SEALs, there is the, the old Top Gun school. There's all of these places. Yep? Neat road operation. they, San Diego and snow doesn't exist. so that's what makes it simpler for us. For rain, they can. but we are, you know, San Diego has 300 days of sunshine. And if we're unlucky, 10 days of rain. so it's sort of ideal from a weather perspective. You don't have, you know, I used to live in Stockholm. In Stockholm, this would be a no go. Because it would snow a lot, it would rain a lot, it would be gray, it would be, much more windy. So we are, it's sort of being tested in the best possible conditions, and then we'll scale from there. but yeah, good question. so I'm, as UCSD. We're a campus of about 80, 000 people. 40, 000 students and 40, 000 faculty and staff. so it's a small city. The advantage is that most universities is a separate police district. I can do stuff on campus that I can't do anywhere else, because I just go and talk to the police chief and say, Eh, can we bend the rules a little bit here? And it's actually possible to do. so that's sort of a nice way of sort of doing this, where we go in, do testing, And we wanted to do this of sort of testing some of these technologies on campus. It's like a small city. And at the same time, what's interesting is that I have 40, 000 students. And you can probably identify, you know, students are like, Oh, wait a minute. Oh, I should go to class over here. And that makes it incredibly hard to actually predict behavior of pedestrians. So if we can Not run into students, then we can operate just about anywhere. and that's sort of a test, and that's why companies like Nissan and BMW and others come to us and work with us, because I can do testing that, you know, most of them are driving sort of autonomous driving cars on the highway. That's easy. First of all, it's a one dimensional problem. There's only traffic in one direction and you don't have to worry about this. We don't have scooters and all these other things that sort of makes it hard. So this is sort of an interesting place and at the same time, I can go to the local police chief and say, I might have to, I might need your help bending a few rules to actually make it possible. And he's let me know when and we'll do it. so that's actually very helpful for doing this. So we, set out. And said if we can do testing here, we would be able to sort of scale it elsewhere. so we bought a bunch of golf carts. and people have since offered me very nice cars. I don't want a nice car. This I can plug on a camera, I can put a clamp on the roof, and it works wonders. If I had sort of a nice car, I couldn't have it sort of look like a Christmas tree of all sorts of computing. So for that reason, this is actually an ideal car for this. And on campus, I can only drive sort of 20 miles an hour, so I don't have to worry so much about it. So this is, and as you know, we have very little rain, we don't even put doors on the car, it's just We drive and it sort of actually works wonders for this. so the idea when you build some of these systems is that you have to sort of do localization. You have to figure out where is the car? GPS, a number of things. You can't rely just on GPS because it's just not reliable enough. There's gonna be sort of regions. So you have to sort of figure out how can I use a map to sort of figure out how do I go around? Then you have to come up with a global plan. I am delivering. Mail from one location to another. How do I come up with, you know, if I go from here to the Morris Inn, what is sort of the route I have to take? You can't just go the straight line. The, Facilities people will give you a hard time. and then you have to come up with sort of a strategy. You have to go straight, turn left, go a little bit more, don't run into people, a few other things, and then eventually you come up with sort of a strategy and then you try and implement it. So this is what we're after. Here's a map of a part of the UCSD campus. you'll see more of these that we sort of do. So we basically go in, build sort of a model of what this campus looked like. Use this to sort of come up with an overall strategy. On top of this we have sort of a, a, what is called a vectorized map, which will basically say where are the lanes, where does it go. and it's based on something called OpenStreetMaps. OpenStreetMaps is sort of an open source model for the equivalent of a simplified version of Google Maps. The advantage is that it's maintained in open source by volunteers. OpenStreetMaps. com It's much more updated than Google Maps. There are clearly people that are sitting at home, bored, and say, hey, you know, there's a new road here. I should go and add this to the map. you know, within an hour, it's sort of fully updated. And you're like, you haven't thought about doing something else with your life? But apparently people haven't. So people want to contribute to this, which is the new economy that you're going in and you're contributing to this. It's incredibly high quality. And it's up to date all the time. Whereas, you know, if you go to certain areas, Google Map doesn't exist. I had the problem that when I moved to San Diego, I moved to sort of a relatively new neighborhood. The first two years, my car will insist that I was driving off road. Because it didn't exist in the car map, and I'm like, I'm clearly here, and you know. It's not a, an off road vehicle. These are being maintained all the time. there's sort of a lesson here. There's a number of these tools that we can use to actually do this. So what we do is that we now have a, basically a graph, and you go through this graph and say, I want to go from A to B, and then you'll do graph search. This is something we've done for 50 years or longer. We know very well to do this, and it will come up with sort of an overall strategy for how do we go from A to B. So it goes in, the blue lines here are sort of saying, how do I get from this? What is the sequence of steps that you go through if you actually want to be able to do this? Come up with sort of a strategy for, what if there's a car parked on the side of the road, blah blah blah. And then eventually we get to sort of a very nice sort of software architecture today. What, if you go to sort of Kevin in the computer science department, they will talk about containers. How do you build sort of small, I'm from Denmark, so think about it as Lego bricks. So you build sort of a set of small Lego bricks. We can easily replace the Lego bricks and actually make it sort of composable and build systems. And if one of the bricks fails, I can sort of take the brick out and I can put it back in. I don't have to take down the entire car. So it's actually a very nice way of sort of building this. And this is the way. Modern software engineering today does it. We build these small Lego bricks, we try to make them as independent as we can of each other. And then, and now you can buy these sort of Lego bricks from other people. So we came up with this. And then, we had to sort of deploy it. And, you know, most professors are sort of, I'm just going to go out on campus and test it. And everybody's going to hate you. so I said, no, I'm going to try and do mail delivery on campus. If I can deliver mail delivery, I can do mail delivery on campus. First of all, now it has submission. It's not like people can say, yeah, we only went to so far today. Did you deliver mail? Did you not deliver mail? and the other sort of is that when you do this, you're doing a favor to somebody on campus. The number of doors that opens when you're doing a favor to somebody on campus. We got free parking, we got all of these things that are sort of impossible to get on a university campus. Because we were helping all the students, we're like, cool, free parking, you know, now we're talking. So we did a six month test of doing mail delivery on campus every day. and the, sort of the, Objective function here was really, normally mail would be delivered at 10 and at 2. And you know, if a big check arrived at 2. 15, they would say, you'll get it tomorrow. There was no sort of flexibility, but it's a big check, doesn't mean it's still going to be tomorrow. so we were saying, cool, now we can actually do ad hoc, we can almost set up sort of an Uber function to do this. you will see in the video that I'm going to play in a minute, that we were driving around on campus, There was a safety driver, inside the driver's seat to make sure that, if I run into a student, I would probably lose my job, so I was like, let's set the bar a little bit higher, than that. And then, there's a guy in the driver's seat, so riding shotgun, and he's basically taking notes. So we're annotating a data set to sort of figure out, what's going on. It's not gonna be perfect, but how far can we get on actually doing this in such a way that, that we can get a reasonable level of functionality. I'm gonna do a quiz afterwards, so if you look at the safety driver, there's something you should notice during this movie. I'll ask afterwards what's special about the safety driver. Hi, I am Henry Christensen. I'm the director of the Contextual Robotics Institute here at UCSD, where we do autonomy smart robots for all sorts of applications. Right here, you'll see our automated mail delivery system on campus. So the experiment we're trying to do here is to understand how can we use these vehicles for doing micros of the last Mile logistics. And it's interesting to try and test these in a campus environment where we have regular traffic, we have pedestrians, we have scooters. So this opens up a number of new challenges for autonomous vehicles. We bought a vehicle, it's retrofitted so that we can do autonomous driving. It has cameras, it has a lidar, and it has various other sensors on there. And we've then programmed it so that it will automatically know where it is, it will detect other things around it like other vehicles. Calls and pedestrians. And then we've sort of developed the logic to enable it to participate in traffic so it can stay on the road. We stay behind another vehicle. When we get up to an intersection, it will read what other vehicles are at this intersection who turn it, and then it will use this to drive through. So
Brian:it will drive just like a regular driver through traffic,
Henrik I. Christensen:except it's all done by a computer. So the technology we're using, this is relatively straightforward. We are using, lidar, which. Pattern detection to be able to fit into cars, to be able to detect. And we are using some of the combination of neural networks and traditional programming to be able to do the detection of all the things around us. Then we use sort of traditional programming and planning to figure out, given the current situation, how do we determine what should we do next? So we started this about a year ago. Anything special about the driver? No, he wasn't driving, right? but he embodied some, an assumption I had not anticipated. Mail can only be handled by a unionized member. you can't deliver mail on the UCSC campus unless there's a union member present. so he has, you know, very clearly on his t shirt, 9 2, whatever it is. So we were not allowed to do this. And if you sort of see it, when he was delivering mail, the graduate student, David, was standing next to him, not allowed to help him, because mail can only be handled by unionized members. So some of these, when you do sort of business processes, that there are some underlying assumptions where you're just like, oh, not allowed to do this. you know, we could, so we still do sort of mail delivery on campus. Otherwise, we cannot do it unless there's a person in the car. Because it's unionized. So it's something that you have to sort of take, keep in mind, there's certain things or sort of assumptions that, that you have to do for this. So we built this car. We deployed it on campus, and drove it for sort of six months. it's programmed to follow the traffic law. So it's very important that, you know, you have to sort of, I have good relationship with the police chief, but, so can anyone of you guess what a challenge was for me? So
Brian:it's sort of, you the carpets are on campus,
Henrik I. Christensen:but then how do you get the
Brian:mail? Where's the
Henrik I. Christensen:point of delivery? so that we could so here's the problem, what is the point of delivery? Actually today it's sort of possible to have a, Almost, you drive up and park next to a door in the building, and you just push out the mailbox into the building, if we could solve the rest of it, that would sort of be, that would be possible. right, so I'll talk a little bit about this later on. So basically it's, right now it's actually reading where are the different cars, and it will sort of take into account somebody was here before me, they have right away, we will take into account are they turning left, are they turning right, are they going straight for an intersection, so we're doing sort of intent estimation to understand what is the intent of this. Yep. Sorry? The safety of the car? We have a fleet of 100 golf carts on campus, just like I was taken from the Morrison over here in a golf cart. so it's very, that's, I share your concern about the sort of the safety of the week. but we'll come back and talk more about passengers or about pedestrians and stuff like that. But, so it's very important for us to sort of figure out how do we do this, and that's sort of one of the challenges we're still working on, good question, but that was not sort of one of the primary things. The primary challenge was actually we programmed it to follow the law. you know, and the law in California requires you to stop at a stop line for two seconds. Nobody in California stops at a stop line for two seconds, you know. You sort of slow down, and then you go through. you know, so the number of bodily gestures we got from people was like, Oh, it's this idiot that stops at all the stop signs. What the hell is wrong with him? And then we were like, okay, so what do you do? We put a small streamer on the back of our car that said, Experimental vehicle, expect erratic driving. And the problem went away. But it's one of those, we just, I couldn't tell. The students would say, you can program it to just, Notice stop signs, but don't really. That would not be. we're getting into this where most people actually don't drive according to the law. It's an advice for how we drive, so it's not. Kevin, you had a comment? Do you recognize pedestrian, a pedestrian pushing a baby cart right in my social, that's a totally different thing. Yeah, we don't care. We care about pedestrians. And we stop for pedestrians. And it's a big challenge for us to sort of make sure but we don't take into account is it an elderly woman or is it a newborn or is it, we, the people. to us. we do take into account, the average person takes up to 12 seconds to walk through an intersection. So we have to be able to predict 12 seconds into the future what's the reality gonna look like for us to actually take that into account. That's extremely hard. Still one of our own problems. but if you go here and sort of look at it, the, where we had the challenges, so we had six months of data, Where you sort of see the pink areas, so you see at the Mail Center, you see at Warren College, you see sort of a, here, this is mainly left terms. and one of the challenges we had is that people would just assume they had right away. It's a golf cart. I'm in a, I'm, you know, I'm in a rush. I'm just gonna cut in front of them and continue on. It was our turn. But they're like, yeah, but you know, you're in a golf cart, you drive slowly, so I'm more important than you. So there's sort of an implicit hierarchy, you know, if you drive in a fancy car, you can overtake golf carts. which was sort of one of the lessons here. the, down here, sort of at the bottom, you see a lot of, this was an area. So it was mainly a dorm area, where people parked on both sides of the road, and there was only sort of one lane. You could only have cars in one direction going through. And our car was not able to predict all the way from one end to the other, so somebody drove into that. Is really a critical resource that they now own and you have to, we have to sort of intervene and say, you know, somebody felt they were more important than you. so it's very important up where you see sort of Warren College, a lot of it, this is a pedestrian walkway. And trying to sort of drive autonomously on a pedestrian walkway where people walk to and from class. so a lesson for the six months was that we could actually do this and, So some of the challenges sort of came away was, you know, how can we generate sort of a dynamic model of the world based on the data? we had to be able to handle the fact that people occlude each other. you can't sort of make the assumption you can see them continuously. the lighting, you have to be able to drive, you'll see later on. You know, lighting, you have to be able to drive day and night, some areas on campus are very dark, but also you have to be able to drive where, you know, the sun goes down at 5 p. m., you're driving straight into the sun. It's not like you can say, oh, I didn't see you because the sun was in my eyes. If you run into somebody, you're in trouble. so we have to be able to build these. And then, of course, you know, rain, fog, snow. We don't see really snow and fog, fog we see, but rain and snow are rare in San Diego so we can sort of test on the ideal conditions. So the question is how can we build systems for this that will sort of be deployed in a much wider world. So one of the things you do for these systems is that you can look at, there's a number of different sensors available, you can buy radars, they cost nothing today. A camera costs even less. I, you know, San Diego is the home of Qualcomm. Qualcomm, among other things, makes cameras. If you look at this, they will say the free cameras in my Apple phone represents a cost of less than 30. A camera is less than this, and in many cases, in volume, we can get a camera down to 1 a piece. And then the computing power is about 9. So I can build this for 10, you can get a camera and the computing power to actually process it. put on, you know, if you're in doubt, put on two more. It's not a big deal. and then, LIDAR is sort of, it's, LASAR radar, where you send out sort of a light pulse, and you measure how long does it take before it comes back. And you can basically combine them. We've seen people like, Elon Musk says that you can do all of this with cameras, you don't need the rest. he should come to Stockholm. I will show him, sort of snowy weather where everything is white. you're not gonna get very far that way, But there are assumptions. There are different companies that are trying to do this. the advantage is that you can sort of go in and look at the different sensor characteristics for all of these sensors, and if you sort of combine them, you will see they will be very complementary. and by combining them, you can get to a system that's very robust. And you just need to get there. at least in the short term. Eventually, I still think LIDARs will go away. They're not long enough range. They're not good enough in snowy conditions. But cameras and radars seem sort of very, predominantly in sort of a 10 year perspective. We can build them in. We can make them reliable enough that we can do it. So we need to be able to do this well enough that we can. And it's more, You know, in a lot of sort of, in computer science, it's very common that you go to a conference and people will sort of say, I have 98 percent reliability. And that 98 percent sounds like a high number, 99, so it's a boo, this is really good. If you have 99 percent reliability, your system fails 15 minutes every day. and, you know, you can't really have a card that fails 15 minutes every day. That's just not reliable. So we need extreme levels of reliability, which is very hard to do. So you sort of build in systems where you combine all of these different things to, into sort of a combined model. You could do it at the low level, you could do it at sort of the medium level, or you can sort of do where you're tracking people over time. It might be different submodels over time, but it would sort of work in some way. And we work with this. One of the things that we're after is that we need to be able to do dynamic mapping. as I said before, OpenStreetMaps, if you sort of look at, this is the map, if you sort of see, the map that you're seeing here is what the autonomous driving companies use today. It has sort of a very detailed model of what does the stuff look like that's sort of on the side of the road. It has a detailed definition of where are all the lanes and it will specify what are all the possible ways you can go through an intersection. These are called HD maps. So there's a couple of companies in the world that make these. they, one of them is in Carlsbad, between LA and San Diego. So when we started to do mail delivery, I called them up and I said, I would like to buy this map for UCSD. And they said, good luck. and I said, but And they said, no, it doesn't exist. I said, okay, when can I have somebody come to campus and do a model for this? And they said, in five, six years from now. And I'm like, okay, that's clearly not gonna work. It took Cruz six months to build a map like this for lower Manhattan. Six months. So it's really hard to do. And the problem is that after six months, that map is already outdated. It's outdated. Our chancellor is very proud of saying we have eight cranes on campus. you know, so the world changes literally every day. so for that reason, we wanted to say this is not a viable solution. If you wanna go, somebody tried to drive from Italy to, Hong Kong, so they would have to go Italy through, up through sort of Eastern Europe, pass through Russia, Mongolia, come back through China, and eventually get to Hong Kong. 80 percent of the time they didn't have a map. It didn't exist, nobody had constructed this. So sometimes we need to be able to build cars that can operate dynamically without doing this. so the challenge here is, can I do this on the fly? Can I do this in such a way that we don't have to worry about this? Can we build? And the good news is that today you can get sort of a pretty good app on your phone that will actually build sort of, I could build a 3D model of this auditorium in 10 minutes. With my cell phone, and it would be accurate to within a couple of centimeters, sort of less than an inch. So in some sense, you know, we started out summer 2019, just pre COVID. This was what campus looked like. And then the chancellor said, go home. And then we came back, you know, a year later and said, Oh, wait a minute. There was a lot of construction crews on campus. So the map we had sort of two years earlier was completely useless, because, you know, one of them was this freeway intersection is now a roundabout, and, you know, it has weird stop signs on it, and, So we learned during this exercise there are 350 stop signs on campus. They move around, but they are actually amazing sort of things that we can sort of use. So we wanted to sort of say, can I go in, And they'll use a variety of different sensors, combine them in such a way that I can sort of go in, I have my car, and I have an RGB image, standard color camera image. I have a LiDAR on there, so one of those LiDAR lasers. I can combine these, and sort of what you see in the middle, that sort of multicolored is what we would call a semantic segmentation map, where we've labeled what are road surfaces, what are traffic marking, what are sidewalks, what are sort of vegetation, and we can use this to drive through the environment. So now, you know, cars, please stay on the purple area of the road. Don't start, try sort of running around on the sidewalks and do this. And today we can generate this on the fly. using some standard modern AI deep learning techniques, you don't want to know. But it's possible and it's sort of lean enough that we can run it on the equivalent of a cell phone processor. so here's It should be a movie. There we go. so basically going in, building sort of a pipeline, where we do this on the fly. So here we drive through something called the village. Here is sort of the equivalent of on the fly. The red are the cars. The blue are the pedestrians. the green is the vegetation. The white is the traffic markings. This can all be computed on the fly today on something that's less computing power than a cell phone. So it's actually pretty easy to build these kinds of models and use this to do navigation. So we don't need to be able to do this. Here's sort of a fully automatic map of the area around our sports arena. so it's actually, it's actually pretty good. So we can do this sort of navigation without having access to these full maps. It's, it becomes dynamic so that you can easily do this sort of Over time, we can, I'm going to go fast through some of these, in the interest of time. we can basically come out, initially what you see here is sort of a global plan. If I was going to go back to the Morrison. It would sort of say, you have to get out of here, you have to go a little bit to the right, and then you have to go to the left, and you more or less have a straight run at the Morrison. and then the problem is that's great, but you can't really implement this. You need accuracy, you need this. So here, you sort of come up with a global plan, and then you repair it on the fly. Now I see a pedestrian, so oh, I should stop for the pedestrian. Or, I come up to an intersection and I have to be able to sort of take into account, there's already a car here. basically we have a global plan, we use this, we use some modern AI, to be able to come in with a model that would allow us to go through this, based on a lot of math, you don't care. And then we'll come up with a, a new sort of technique that allows us to basically adopt. So when I go out here and say, get outside the building and you have to turn right, and then you have to go a little bit down and then you have to turn left. Now the question is, if you go straight out of the building and you turn right, you're going to be in a flowerbed. So instead we have to sort of now contextualize and say when he says right, what does he mean? He means go ten steps forward, go down this, and when he has to go left, it's at the intersection. So all of this is sort of being developed by modern AI techniques to be able to do this so that we can actually drive around. And basically here what you're seeing is that we have sort of a data set that we recorded. We only recorded this during COVID. It was wonderful to be on campus. There was nobody else there. So we didn't have all these pedestrians and all the traffic that would sort of interfere with us while we were doing this. it was also a little bit scary to come to campus and sort of realize that it was like a deserted town. But it helped us build a lot of this data, go in and actually do some, some pretty cool things. So we built maps that are sort of allowing us to drive very reliably. Within, I would say, four inches, you would sort of stay at something that's very optimal compared to what a driver would do. so that was pretty good. Now, with this, we can detect all the road users. So the A is a pedestrian, B is another car. so as we drove, as we were talking about before, now I can take into account all of the different, what you do when you sort of drive at an, you come up to an intersection, you look at all of these. An interesting sort of side fact is that many of you will claim that you look in the eyes of the other drivers to determine if they're paying attention. We cannot confirm that. so we did an experiment on campus where we dressed up students as car seats. So they sit in the car, their appearance is a car seat, you're looking out through the netting that sort of is the headrest, and then you're driving down here so nobody can see they disappear. And we drove around on campus for three months. With these sort of car seats. so we come, oh yeah, there's nobody in that car. And then people went on, sort of, wait a minute, you know, there's nobody in that car. And people, yeah, that's perfectly normal. but we could not confirm. It didn't change the traffic statistics at all. Whether there's a visible driver in the car, or there's just an empty car seat. So even though we would sort of claim that we're doing this, in reality we can't, there's no statistical significance that's actually true. But we use this to come up with a way of sort of doing this. But you have very complex interactions in some cases. So here's an example where there's a couple of bike riders, and they will come up to there and they'll say, You can go first. We don't know what to do with that. Wait a minute, you know, what do you mean you can go first? We, you know, we don't recognize gestures from people. And the traffic law would not allow us to actually go. So that poses a huge challenge to us. He says, go ahead. After a while he will disappear. We just can't do it. And if we went and we ran into him, I would be out of a job. but the other thing is that sometimes the world is very confusing. Here's a traffic worker standing, he said, Go slow, there's a stop sign. Who should I pay attention to? This is actually really hard to do. The other is that here where you see the sort of stop sign, this is where they're doing road work, and basically there's only one lane. You should never stop there in the middle of this. You know, somebody will yell at you or worse. being contextual is incredibly hard for this. I mentioned this before, Kevin and I met many years ago, we were both in Tennessee, and one night there was a new study that said, so they asked how many people actually has liability insurance in Tennessee. You're liable no matter what, but it's optional if you have a liability insurance. And it turns out that the more people that have a gun in their car, then they have a liability insurance. And I drove very nice afterwards. Because, you know, suddenly you realize, oh, this could be dangerous. But this is hard. So building these is still one of the areas where we have a big, sort of, a big challenge. The other is that we have to, as I mentioned, we have to be able to do robust detection of pedestrians. So all of the other cars today, when you see Tesla and all of this, are pretty good at detecting cars. They're pretty good at detecting sort of the layout of the road. They can drive on the highways. They can drive on major roads. You can't make the assumption that you can necessarily drive everywhere on the Notre Dame campus or something like that because they probably don't have a full model for the entire Notre Dame campus. But almost none of them can take good care of pedestrians. So it's a big challenge, and that's sort of one of the things we wanted to do, so we wanted to do, can I go in, can I detect and track these? so here is, you know, can you detect all of these people reliably enough? Turns out that there are people like Kevin that's done a lot of work on sort of doing biometrics, detection of people and all of this tracking, that can sort of be used for doing this, but can you do it reliably enough over time? And can you predict their intent? so here is an example of a video to just give you an example of how hard this is. Here you can see we're sort of doing skeleton tracking of people. Oh, by the way, you have to be able to do it at 4 in the afternoon when the sun goes straight into your cameras. And you can't sort of forget about people just because the sun is in this. So you can see there's a person here, actually relatively close, that's very hard to drive. So this is one of our challenges, can we do this reliably all the time and guarantee that we, So this is in the middle of the, this is the equivalent of next to the student center at UCSD. So during breaks and lunch, you know, if you can drive here, you can drive anywhere. but you can see this is actually quite reliable. we then, and we have sort of probabilities on this. If we're low probability, we would drive slower. but of course, you should also be thinking about Big Brother watching you. I can track the students on campus and tell who came to class and who didn't come to class. So you should also take into account how, you know, in your 10 years hence sort of theme, how far can we get on tracking people on this? So there's some very important sort of, privacy concerns that we have to sort of take into account to be able to do this. So we, go in, we detect people, both in terms of people detectors you can get. They cost nothing today. Simple enough to do the standard techniques. Whether you use sort of traditional image recognition, or you use sort of modern deep learning, there are end methods. and sort of relatively cheap. So what we do is that we go in and we detect this, but we also detect faces. And then we do, for our system, we do anonymization. We never store an image segment with a face on it. Because that immediately opens up all sorts of questions about, are we doing sort of monitoring? So you have to think about in your ten years hence, what can we do to build this so that if you video film in public areas, how do you make sure that you have privacy? which actually becomes very important. So we can go in, we can do this, we're using a number of different techniques, we're using sort of sensor fusion, put it all in. Do your best sort of statistics, methods for doing probabilistic modeling, doing fusion, and you can come up with techniques that are very reliable over time. So we do all of this, and then comes up and says, where are the head boxes, what are the fused sort of technology, and how can we use this to sort of go through the environment, and do, Very reliable things, So here's an example where we're just tracking the faces and they're all anonymized. so you can see, as we drive, we're driving very slowly here because there are people in front of us. This is recorded from our golf cart, where we can actually drive through campus. This is a nice scene, in terms of, you know, if you do this at lunch, there's just going to be, you know, thousands of people. And all you can do is just drive slowly enough. So now we have to do sort of crowd management for that. We're actually using methods from game theory to sort of figure out what's the right way of doing this. But you can see, you have to do it with the sun, you have to do all of this. This is still not really on cars today. On commercial cars, we're still waiting on a time. That's why you're seeing the I'm sure you've seen a lot of these sort of press stories about, Cruise and others. Mainly their tests are getting sort of Challenged because you need to be able to guarantee that you don't run into people, on this. So that's pretty cool. So a new project we've just started is doing another step on sort of micro mobility. I haven't been on campus long enough to really know how much you have a scooter problem here. At UCSD, we have a scooter problem. In the sense that, you know, we have a trolley station, we have two trolley stations on campus. And most people are between 0. 7 and 1 mile away from their final destination. So people then get on scooters, and they drive to a lecture hall, dump the scooter. So we have sort of heaps of scooters on campus. and you know, so they came to us and said, Great, you're doing all of this, what could we do? so one of the products we have right now, and we've just started to operate, is that we have a three wheeled scooter. I don't want to worry about balancing, this is a controls problem. I'm a computer scientist. so but it will sort of balance automatically. It will allow you to drive from the trolley station to your classroom. When you get off, the scooter becomes autonomous. It will then automatically drive back to the toilet station so that somebody else can use it. So we don't have heaps of scooters anymore. Instead, they go back and being used. And right now we then have a project where we're then looking at, can I do analytics on this to figure out where should the scooters be in the morning and where should they be in the evening? So in the evening, they should be close to the classrooms. In the morning, they should be close to the toilet station. And in between, we should monitor battery usage. So when I get loads of battery, it's stopped by a, charging station, uses wireless charging, so basically we now have, you know, this, and I'm trying to see if I can come up with a business model, where imagine you buy one of these scooters, and you, if you're at Notre Dame, You rent it out to Notre Dame from the time you arrive on campus until you have to go back. So you say, I need it back at four. What do you do in between? Make money for me. so that way it's like an Uber fleet of scooters that you have a high end. This, you charge for the number of rides on it. And that way, Notre Dame doesn't have to invest in a fleet of scooters. UCSD doesn't have to have sort of 10, 000 scooters. Instead, they can do this. Of course. The, the Ubers and the Lyfts are telling us we can do this. You don't need to interfere with our business. But, still, it's sort of, but I think you should see this in the future. We're going to get a number of these that will drive around. They will be, you know, you drive them wherever you want to go. When you step off, it gets autonomous. It doesn't have to drive 30 miles an hour. Five miles an hour is perfectly fine. It drives back and, you It puts itself into operation again. So we've just started this, and I'm not showing it, sort of, a video of it because we're still trying to sort of patent some of the technology. so I'm also an entrepreneur, so I want to make sure I actually get some money back. so we're doing, sort of, this, we're building autonomous vehicles for sort of robust operation. You've seen, to do this, we need to take away the HD maps. We need to sort of, it's not feasible in the long term. All the automotive companies have to sort of do this. We have to be able to do sort of, robustness, 99 percent is not getting you there. There has to be many more nines after the decimal point. you need to be able to be reliable enough to do it. When you come up to an intersection, you need to be able to predict 12 seconds into the future. You which is actually, today we can do six. So we're still halfway there, but we're not close enough that we can actually predict that car is going to stick at that stop line long enough that I can actually get into the intersection and get out of the intersection. and that's sort of where we get to, but at the same time, there's an enormous business potential here, both for the micro mobility to do deliveries, so that when you press the Amazon button and saying, you know, I want a new car, Whatever will it get to me within the next hour? Because I'm not a patient person. and then, at the same time, for autonomous driving cars, Buying a car is the worst decision of your life. You have to remember, you know, the biggest expense of your household is housing. The second largest is cars. In our house, it's almost the same. I have a really nice car, and, you know, but it's more, you know, when I bought it, and then we drove out of the lot on the way back from the dealership, my wife Looked up on Kelly's Blue Book the value of this car and said, We've just lost hundreds of thousands. And, you know, I was in the doghouse for a while because, you know, that was, That's what you do. If you look at it, I use my car 2 percent of the time. The rest of the time it's parked somewhere. It's completely useless to me. So car sharing is gonna be a big deal. Can we get to a point where you can actually do this? And then you can have different levels of car sharing. And eventually they will become autonomous. You just want to get from A to B. You don't really care. You know, in some sense, you would like to have different kinds of cars for different kinds of functions. You know, Tuesday night, you're trying to get to the grocery store to get some things, yeah. You know, any wreck will do, as long as it gets me from A to B. When I lived in Tennessee, I had a 50 Scirocco. it got me through six months of transportation in Tennessee. you know, that, that was real value for the money. that's nice. You know, Friday night, you want to go on a date, I want the fanciest car I can afford for money. and you know, Saturday I need to go to Ikea and buy some new furniture, and now I want a truck. I can't afford to own three different cars, but it would be nice if I could just have a car service where this would actually be possible. So we're starting to see a new sort of very much a service economy that will drive this, and that will very much sort of drive the economy going forward, which I think is going to be important, and for you that's going to be, don't make that big investment, it's not worth it, do sort of a car service instead. It was briefly mentioned in the introduction, the other thing I do is that I, I write the National Strategy for Robotics. every four years we publish sort of a strategy for where do we think robotics and automation is going five, ten, fifteen years down the road. and, we then go to D. C. and present it to Congress. I was told by somebody in the White House, Congress has no money, so you should come see us at the White House. It's a taxi ride away. so I went and talked to the White House. So we do this every four years. and so if you're sort of looking for where do we think the world is going, this is actually a pretty good sort of, place to start looking. the good news and the bad news is that it predicts 5, 10, 15 years into the future. So people can go back and read the 2009 and see how good were we actually at predicting. And I would say we are 70%. So we're above chance, we're not perfect. there's a number of things we didn't anticipate the new sort of deep learning If you invested in NVIDIA, you were all smiles yesterday, because some of us made 15%. but we didn't sort of anticipate that it would be that big a deal. We didn't anticipate some of the new camera technology. we didn't anticipate how quickly it would sort of get into Most of this is not driven by autonomous cars. It's driven by consumer electronics. It drives down the prices that makes it sort of accessible for us. So it's a good place to sort of go and look at some of this. It's our 2024 roadmap will be published in a week. so it's very close. we're doing sort of a meeting in San Diego next week, and that will be sort of the final editorial meeting. Here, of course, it's the intersection of robotics and AI. It's gonna get into a point where we can do this at a level we've never anticipated before. If you look at, If you haven't looked at it, you should go and look at a good example of where technology is going. There's a company in California called Embodied. They've developed this sort of small robot, this size. It's designed to sort of be the tutor for a child that are sort of early elementary school. Talk to the students, talk to them about, you know, algebra, Basic language understanding, geography, all of this. It's using sort of modern language technology. So it has a fluent dialogue with a kid. talking to them, saying, Hey, you know, maybe we should talk about this today. Or maybe we should do this. Or say, I have a mission. We should go and draw some beautiful pictures for your parents. And then it will actually go off and do it. a robot that sort of becomes your personal friend, it will understand your interests rather than other people's interests. And, if you look at it, it's pretty amazing, and it's 600. So you can get a robot for 600 that will have a fluent dialogue with you that will become your child's best friend. And that's pretty amazing. So now we can individualize training, tutoring, all of these things. So if you now look 10 years out, that's going to be pretty scary. I might not have a job anymore. You can individualize training anymore, which would be great. So we're sort of looking at this is an area that moves incredibly fast for this. As I said, you know, I'm just the ambassador. Most of the stuff is actually done by my students. having fun on campus and this is a typical day in San Diego, blue skies and no rain, so I can't complain. it's been published in a bunch of papers, so you can sort of look it up if you're interested. And of course, it's been sponsored by a number of different companies we work with. So for us, it's very important that I'm sort of, Adamant that we not only do the research, if it doesn't get translated into the real world, we're leaving money on the table. I'm a businessman, so I don't leave money on the table. So for us, it's important to work with the various kinds of, the Nissan's, the LG's, the Tucson's, the, to Amazon, we've done a fair amount of translation to Amazon and Qualcomm. so I think that's important for us. We need to make sure it actually gets out there and gets used in the real world. And with that I will say thank you and we still have 10 minutes or something like that for Q& A. Thank you.
Brian:I've got one, so the students will correct me, but, our scooters were banned recently, is that, yeah, okay, scooters were banned, from campus, somewhat selfishly, what, if the lecture is ten years hence, so looking into the future, ten years from now, will we have autonomous golf carts driving around campus to take, Faculty members to a meeting in the Dome. Do you see that as sort of a,
Henrik I. Christensen:Oh, I see it as, you know, your bus service will get replaced by a distributed transportation service. so that you're not going to have the heaps. This is going to happen very quickly. and also I think, you know, making sure you can get transportation all the time. we had, there was a period where I was at Penn where, We had drivers making sure people could get home from Friday night parties, not having to sort of worry about is this actually safe, is this not. so I think all of these will sort of become, because it drives, there's a need for these kinds of technologies. Thank you for the presentation. question about the commercialization of this technology taking things like interstate on the highways with big semi trucks versus just, you know, through schools. And where do you see that going in the next ten years? More personal use of automotive vehicles or commercial
Brian:use?
Henrik I. Christensen:The first place is commercial use. The commercial use will come first. The trucking, so we've seen the motion, there's quite a few companies that are doing this today. And it's partly a safety concern. We have people driving, you know, I 40 sort of coast to coast. It's half asleep. you know, so it's very hard, we will get there and some of this is also coming because a lot of the technology is slowly getting in there. So there's a company, there's a company in San Diego, Litix. They put in sensor monitoring for drivers today. Most commercial vehicles today have video monitoring of the driver. It will, Do gaze detection. It will determine are you looking at the road? Did you look at that pretty woman that passed by too long? or, you know, are you actually being, are you about to fall asleep? This is in 180, 000 vehicles in the U. S. today. But the next step is to actually make it fully autonomous so that we can actually drive much longer. I think for a long period of time, there will be a safety driver there that will be able to sort of say, I'm coming up on road work or something like that, I'm not sure I can do this. People will take over, but for the long stretches on the highways, this is going to get automated within the next 10 years. Getting off the road is much, much harder to get into sort of urban environments. But for the long stretches on the highways, this is going to get automated soon. A lot of these techniques are using, modern AI, sort of deep learning. And it's actually very simple to do sort of deception on these. Put small markers on stop signs or something like that, and rather than a stop sign, it looks like 30 miles an hour. And you blast through an intersection, and so I think one of the areas that we're going to see a tremendous amount of progress on is on certifiable AI. very much. We need to get to a point where we can certify these systems. So the, the truck I showed, at the very beginning, this one, 50, 000 vehicles, deployed by Amazon, is no longer on the road because Amazon couldn't explain to OSHA The safety requirements of when would it fail? And then OSHA said, you're out. And, when you paid for 50, 000 of these, you get pretty upset. so we had a project with Amazon on actually figuring out how can we do this with certifiable methods to guarantee that it will act, that we can get this on the road. So this is a huge challenge. that how do we provide guarantees? And today I would say Most of these systems, that was why Cruise was taken off the roads in California. Because they couldn't provide certifiable methods. Too Simple, one of the big commercial truck companies, has left the U. S. because they couldn't sort of certify their methods well enough. And eventually they went to China and then they did some, you know, if you are taking a management course on bad decisions, you should go and look at how Too Simple did sort of management of their company. but, Huge problem. Very important problem, but also a problem that is very active in sort of modern AI today. How do we provide guarantees for it? but, until we get there, we're not going to be able to deploy these things at scale. Autonomous vehicles, in November of 2019 in Las Vegas. And It was being tested by Uber or Lyft, and I was just an ordinary citizen that signed up. And, fascinating. I assume they've made progress since then. That's a city, obviously, with a lot of noise. are they still testing that with ordinary citizens in the cars? Do you have any idea? And also, it struck me, how do you build, when it gets to the point that it can be used, there was obviously an operator, watching it. Building public confidence in, Putting yourself in that kind of a vehicle. today we have, if I remember correctly, 12 states where you're allowed to test autonomous driving cars. California has withdrawn for most companies. Waymo still has a permit in California, but most other companies have lost their license because there's been too many accidents. You know, the, in California. And dumb, sort of, you know, I'm always saying that don't let engineers build systems, you know, you need to have other people involved in it, you know, so in, in, Cruz had an incident where they, ran through an intersection. They had a green light, but there was a emergency vehicle going across them, and they didn't know emergency vehicles had precedence. They'd forgotten to write that into the rules. and the same we've seen, they did the same thing. They ran into a person, and they reported it to the control center. We ran into a person, that was injured. Nobody called 911. And he's hello people, you know, don't let engineers run this. We need to have other people involved in making sure we actually do the right thing and the right protocols are in place. But they are trying to make us for CES and these shows trying to sort of get it out there and let people see that for the most part, it's, we're getting nine to nine percent there. If it's a good weather day, it's sort of reasonable traffic, you can start using it and then slowly it will get to a place where it's, where it's good enough. so it'll take time. I still think it'll, fully autonomous cars are 10 years away. because there's so much we still need to do, to get to it. So we're not gonna get it, In the short term, it's not realistic. There's just too many sort of edge cases where we're just not there. it is going to take time. But at the same time, the, you know, some people would feel much more comfortable putting their teenage daughter in an autonomous driving car than with an Uber driver, where you don't know what is the track record of this person. so there are drivers that will get it there, but it's not, At least 10 years out, I would say, before we get it sort of for everyday use. one last question. this is probably a question you get a lot, but I'm curious your take on, how the economy and just people will adopt their skill sets as autonomous vehicles take over different jobs, such as trucking and delivery, and, A variety of so many other things, so what's, what is your take on kind of how we will adapt? It's a good question. it's, I think we will, I think you will see multiple different approaches. So as I said in the beginning, today, For every 10 open jobs, we only have 7 people available in the workforce to take those jobs, so we have sort of a 30 percent shortage. So that's why we see automation in warehouses and in a number of these areas, because we just don't have the people. So if we want to grow the economy, it's great that in Washington D. C. says, we're going to grow the economy, okay? Please come into the real world and I'll show you that it's not just a matter of throwing money at it, you need to be able to do it. So I think there's a number of these. More importantly, I think we're in for a paradigm change, where you have to sign up for lifelong learning. You're not gonna, you know, so I, many years ago, when I got my college degree, the university president said, You're set for life. Was he lying? Damn, you know. so we need to get to a point where I think we need to think about that, the equivalent of four hours a week. ThinkND. Is spent on upgrading your skill set for the rest of your life. And it should be part of your job, it should not be something you have to do on, in your spare time. So if you're working 40 hours a week, 10 percent of that should be spent on updating your skills. And that's why I think the future universities will be a subscription model. It's not like you pay tuition for four years and then you get out and, no, you pay something and that's gonna be your fee for, sort of, doing this for the rest of your life and then, you We've seen dramatic changes in computing in, you know, anywhere from using, learning to use Word, to using Excel, to using all of these other things, social media for some things. So we need to think about this as upgrading your skills throughout, and we're in a world where we have exponential development. If we don't do this, we're going to have a lot of people, and we see China, we see a number of other nations that are very aggressively pursuing this. If we don't do this in the US, we're going to fall behind.
Brian:Professor Christenton, thanks so much.
Henrik I. Christensen:Thanks.