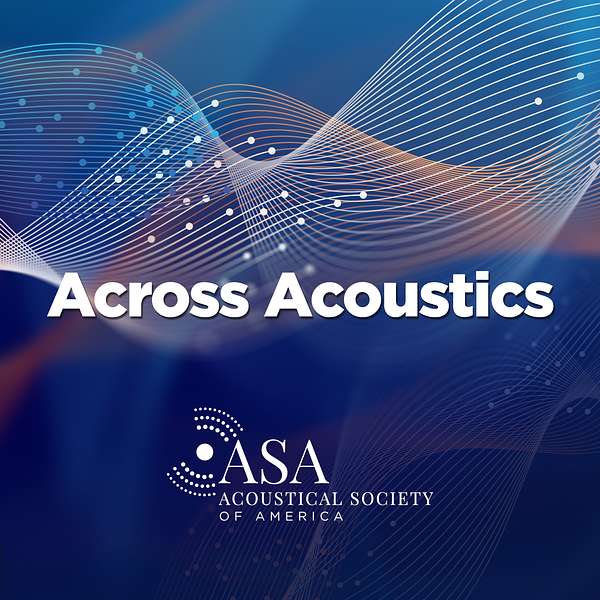
Across Acoustics
Across Acoustics
Lung Ultrasound
Deep learning applied to lung ultrasound videos for scoring COVID-19 patients: A multicenter study
The Journal of the Acoustical Society of America (JASA)
https://doi.org/10.1121/10.0004855
Authors: Federico Mento, Tiziano Perrone, Anna Fiengo, Andrea Smargiassi, Riccardo Inchingolo, Gino Soldati, and Libertario Demi
In this episode, we interview Federico Mento from the Department of Information Engineering and Computer Science at the University of Trento.
Read more from The Journal of the Acoustical Society of America (JASA).
Learn more about Acoustical Society of America Publications.
Music Credit: Min 2019 by minwbu from Pixabay. https://pixabay.com/?utm_source=link-attribution&utm_medium=referral&utm_campaign=music&utm_content=1022
Ambri Phillips 00:06
Welcome to Across Acoustics, the official podcast of the Acoustical Society of America’s Publications office. On this podcast, we will highlight research from our four publications, The Journal of the Acoustical Society of America, also known as JASA, JASA Express Letters, Proceedings of Meetings on Acoustics, also known as POMA, and Acoustics Today. I'm your host, Ambri Phillips, Business Administrator for the ASA.
Joining me today is Federico Mento of the Department of Information Engineering and Computer Science at the University of Trento. We will be discussing his article “Deep learning applied to lung ultrasound videos for scoring COVID-19 patients: A multicenter study,” which appeared in the May 2021 issue of JASA. Thank you for taking the time to speak with us today, Federico, how are you doing?
Federico Mento 01:02
I'm fine. Thank you. I'm pretty excited to join the podcast.
Ambri Phillips 01:08
Oh, great. We're happy to hear that. Thank you. Can you give us a little bit of a background on yourself?
Federico Mento 01:14
Well, I got my bachelor's degree in Electronic and Telecommunications Engineering and my master’s degree in Information and Communications Engineering at University of Trento, and I'm currently a PhD student at the Ultrasound Laboratory of Trento.
Ambri Phillips 01:31
Okay great. What are some of the complications associated with COVID-19?
Federico Mento 01:37
COVID-19 causes important complications to the respiratory system and the symptoms vary from patient to patient. Indeed, even though about 80% of patients show mild symptoms, the other 20% shows serious complications that need hospital treatments. And in this context, both the monitoring of patients and a timely and correct diagnosis are needed.
Ambri Phillips 02:02
What are the diagnostic tools that are used to test for COVID-19? How accurate are they?
Federico Mento 02:09
The most currently used diagnostic tool is the reverse transcription polymerase chain reaction, the RT-PCR test, which is essentially the swab test. Currently, this can be considered as the main tool to perform a diagnosis of COVID-19 and the only tool to diagnose COVID-19 in asymptomatic patients. However, these tests show high false negative rates. On the other hand, when COVID-19 patients present a lung involvement, other imaging tools can be exploited such as the chest computed tomography, which has reported sensitivity between 61% and 99% and specificity between 25% and 33%. However, the main problem related to chest CT is the need to move the patient within hospitals, thus increasing the contamination risk. And in this context, lung ultrasound has been proven to be an important ally for clinicians dealing with COVID-19 patients, thanks to its main characteristics, which are real time imaging, portability, and wide availability, lung ultrasound nowadays represents an important imaging solution that can be applied for triage of symptomatic patients and patients’ monitoring.
Ambri Phillips 03:28
How does lung ultrasound work?
Federico Mento 03:32
Standard ultrasound imaging is nowadays based on basic assumptions on the acoustic properties of human body, such as the quasi-homogeneous speed of sound in the volume of interest. However, lung is aerated organ, where these assumptions are strongly unmet. Specifically, the large difference in acoustic impedance of intercostal tissues and the air in lungs generates an acoustic interface, called the pleural line, having a high reflection coefficient, which is about 1. This does not allow the lungs to be penetrated by ultrasound waves when the organ is well aerated. Therefore, in well aerated lung, the lung can be approximated as a perfect reflector, and this reflection generates in the image the so-called horizontal artifacts. In contrast, when the lung becomes pathological, a part of the volume previously occupied by air is replaced with media acoustically similar to the intercostal tissues, for example, water and blood, and this replacement allows ultrasound waves to penetrate beyond the pleural line. This replacement essentially forms acoustic traps that can be entered by ultrasound waves, thus rendering the lungs partially permeable, and the result of complex phenomena generated by interaction of ultrasound with these traps can produce vertical artifacts in the image. Hence, lung ultrasound is currently mainly based on the interpretation of these artifacts, which are linked to small or large alteration of the lung surface.
Ambri Phillips 05:14
What is a deep learning-based algorithm?
Federico Mento 05:19
Deep learning-based algorithm can be defined as a specific type of machine learning algorithms that exploit multiple layers to progressively extract higher level features from the raw input. As an example, a lung ultrasound image classification task can be performed by a deep learning algorithm by exploiting labeled data. Specifically, given labeled images, the deep learning network can be trained to learn its task, which is in this case to classify the images according to the labels. Then, after the training, the network can be used in the prediction phase to classify the images.
Ambri Phillips 05:58
How was data collected for the study?
Federico Mento 06:01
The analyzed population consists of 82 patients with a diagnosis of COVID-19 positivity by RT-PCR swab test. A part of the patients was examined within the Gemelli Polyclinic in Rome, and the other part was examined within San Matteo Polyclinic in Pavia. The patients were examined by exploiting the protocol we developed in March 2020, which is based on the scan of 14 areas of the lungs. Therefore, an exam consists of the analysis of 14 lung ultrasound videos, each of which associated to a scanning area. As some patients were examined multiple times we obtain a total number of 114 exams, consisting of 1,488 videos that consist of more than 300,000 frames. The videos were classified by lung ultrasound expert clinicians according to a four-level scoring system, which is based on lung ultrasound patterns that are linked to the severity of lung involvement. Specifically, score 0 represent a healthy lung surface, whereas score 3 represents the worst condition of the lung. For this study, we fed all the frames to the trained deep learning algorithms we previously developed with two groups specialized in deep learning. As these algorithms provide the frame level scores and the clinicians, in their practice, evaluate lung ultrasound data at video level, we developed an aggregation technique to classify lung ultrasound data at video level.
Ambri Phillips 07:39
Okay and what were your findings?
Federico Mento 07:41
We found that the perfect agreement between deep learning algorithms and expert clinicians was more than 50% when looking at video level. However, a more significant result was obtained looking at the prognostic agreement. To clarify what the prognostic agreement is, a recent publication has demonstrated the prognostic value of our imaging protocol and scoring system once evaluating the cumulative score, that is obtained by adding the single scores on each of the 14 measurement points. Specifically, a cumulative score higher than 24 represents a patient at high risk of worsening, whereas a patient with cumulative score less than or equal to 24 is considered at low risk. This classification at exam level can be exploited to stratify the patients. And in this case, the results show a percentage of agreement between deep learning and lung ultrasound experts about 85% in the stratification between patients at high risk of clinical worsening, and patients at low risk.
Ambri Phillips 08:54
Okay, and what was the conclusion from the study?
Federico Mento 08:59
These encouraging results demonstrate the potential of deep learning models for the automatic scoring of lung ultrasound data when applied to high quality data acquired accordingly to a standardized imaging protocol.
Ambri Phillips 09:14
Do you have future plans to expand on the study?
Federico Mento 09:17
Yes, we have different plans for the future. As an example, we have recently published a similar analysis on the prognostic agreement between artificial intelligence and expert clinicians, but with an expanded database, including more than 200 patients, divided in COVID-19 positive patients and post-COVID-19 patients. And this analysis showed us how the prognostic agreement decreases when evaluating post-COVID-19 patients, suggesting the necessity to retrain the deep learning networks with post-COVID-19 data, as they can show different lung ultrasound patterns. Besides these semi-quantitative studies, we are also working on the development of lung ultrasound quantitative studies that are based on the analysis of radio frequency data. These studies, jointly with numerical simulations, could lead to a bigger understanding on vertical artifacts, allowing us to extract important physical information on the lung surface.
Ambri Phillips 10:25
What is the status of the proposal for the standardization of lung ultrasound?
Federico Mento 10:30
Well, currently the PI of my lab, Professor Demi, is working on an international consensus on lung ultrasound. And this consensus aims at including both the contribute from the clinical world, from expert clinicians, and from the technical world, from engineers and physicists. Indeed, we believe that the cooperation between clinical and technical worlds is fundamental to improve the capability of lung ultrasound by defining, for example, standardized protocols and imaging parameters.
Ambri Phillips 11:06
Great! Well, Federico, thank you for taking the time to talk to us about lung ultrasound and their relevance to COVID-19. This information was very useful given the current times. Thank you so much.
Thank you for tuning in to Across Acoustics. If you'd like to hear more interviews from authors about their research, hit Subscribe and find us on your preferred podcast platform.