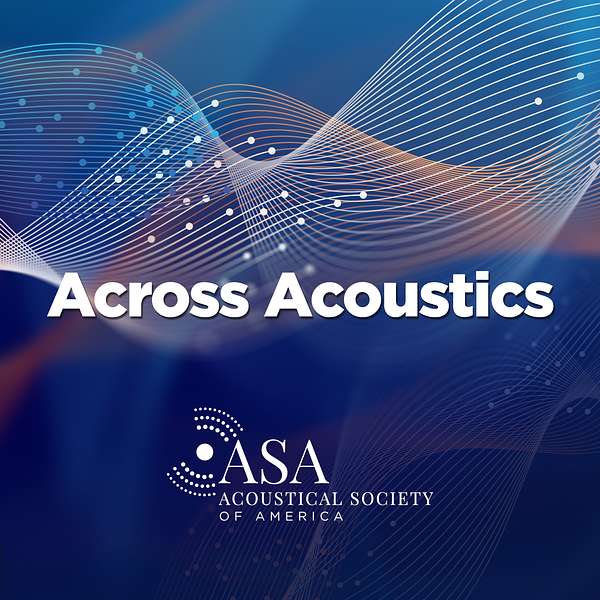
Across Acoustics
Across Acoustics
Student Paper Competition: Moth-Inspired Microphones, Rocket Noise Mitigation, and Deformable Microphone Arrays
Could moths’ hearing be the key to figuring out how to localize sound with tiny microphones? How do we prevent rocket launch noise from damaging the ship’s payload? Is it possible for algorithms to account for microphone arrays that don’t stay in a rigid structure? These are some questions considered by Acoustical Society students who won the latest round of the POMA Student Paper Competition from the 183nd meeting of the ASA. In this episode, we interview the three competition winners, Lara Díaz-García, Mara Salut Escarti-Guillem, and Kanad Sarkar, about their research.
Associated papers:
Lara Díaz-García, Andrew Reid, Joseph Jackson-Camargo, and James Windmill. “Directional passive acoustic structures inspired by the ear of Achroia grisella.” Proc. Mtgs. Acoust 50, 032001 (2022) doi: https://doi.org/10.1121/2.0001715
Mara Salut Escarti-Guillem, Luis M. Garcia-Raffi, Sergio Hoyas, and Oliver Gloth. “Assessment of Computational Fluid Dynamics acoustic prediction accuracy and deflector impact on launch aero-acoustic environment.” Proc. Mtgs. Acoust 50, 040001 (2022) doi: https://doi.org/10.1121/2.0001716
Kanad Sarkar, Manan Mittal, Ryan Corey, Andrew Singer. “Measuring and Exploiting the Locally Linear Mapping between Relative Transfer Functions and Array deformations.” Proc. Mtgs. Acoust 50, 055001 (2022) doi: https://doi.org/10.1121/2.0001707
Find out how to enter the Student Paper Competition for the latest meeting.
Read more from Proceedings of Meetings on Acoustics (POMA).
Learn more about Acoustical Society of America Publications.
Music Credit: Min 2019 by minwbu from Pixabay. https://pixabay.com/?utm_source=link-attribution&utm_medium=referral&utm_campaign=music&utm_content=1022
Kat Setzer 00:06
Welcome to Across Acoustics, the official podcast of the Acoustical Society of America's Publications Office. On this podcast, we will highlight research from our four publications. I'm your host, Kat Setzer, Editorial Associate for the ASA. We have another round of Student Paper Competition winners, this time from the 183rd meeting of the Acoustical Society of America, which took place this past December in Nashville, Tennessee. First we'll be talking to Lara Diaz-Garcia about her article, "Directional passive acoustic structures inspired by the ear of Achroia grisella." Lara, congratulations. Thank you for taking the time to speak with me today. How are you?
Lara Díaz-García 00:44
Oh, thank you so much for being interested in my research and for the opportunity to talk here. I'm fine. Thank you.
Kat Setzer 00:49
It's a lot of fun. I'm excited for our listeners to hear about it. So first, just tell us a bit about your research background.
Lara Díaz-García 00:55
Uh, sure. So I'm currently finishing up my PhD in the department of Electronic and Electrical Engineering at the University of Strathclyde, which is in Glasgow, Scotland. But my background is actually in physics. So I did my undergraduate degree in physics back home in Spain. Afterwards, when I was considering master's degrees, I decided to pursue acoustics. This comes mainly from my interest in music and my classical piano training. And I basically wanted to bring my two passions together, and decided to take a master's degree in Acoustics and Music Technology in the University of Edinburgh. And then when I finished, I actually had a broader interest in acoustics besides just music, and so bioacoustics sounded really appealing to me, which is basically anything related to life and sound. So think hearing aids or animal communication or biologically inspired design. And that's how I ended up applying to the PhD in my department doing what I do.
Kat Setzer 01:51
Awesome. Well, your area of research does sound super fascinating. And specifically with this article, you're using the hearing of moths to inspire microphones. So to get us started, can you tell us a bit about what's going on with microphones right now, and what the need is you're trying to fill with this research?
Lara Díaz-García 02:07
Yeah, so microphones and their working principle have remained relatively the same since their inception in the late 19th century. And in general, sound displaces a moving plate or element of some sort, that will consequently induce a change in, say, capacitance for condenser microphones, or a change in pressure for piezoelectric microphones, or induce an electric current when it is sitting within a magnetic field, like is the case for electrodynamic microphones. But in general, all of them have the same principle, which is sound moving an element, and that's translated somehow to an electrical signal. And we have generally gotten better at technology and material science, so that has allowed us to reduce microphone size greatly and improve their performance. And the first microphones had such a narrow dynamic and frequency range, that it was barely understandable, and nowadays you get quite high fidelity, but there's still a desire for even smaller microphones. So for smartphones or hearing aids, a reduction in size will improve the user experience by making them more inconspicuous and just in general easier to carry. And this general trend is reflected in the development of microelectromechanical systems, or MEMS. And that has been going on for the last 60 years or so. But for the small scale that we're talking about, there are specific size-related problems. So first of all, if the sound wavelength is larger than the microphone itself, as it might happen for human's speech frequencies, the microphone may not be able to pick up the signal; noise also becomes a problem for small microphones. And lastly directionality, which is being able to tell where a source is located, or like rejecting ambient noise, and that is also difficult when scale is reduced because the way it's usually achieved with MEMS is with microphone arrays of two or more elements, which need to be far apart at a certain distance. And that's going against the miniaturization you were looking for. So for all of these reasons, we try to look at how to improve the design in maybe not the most obvious ways.
Kat Setzer 04:13
Okay, yeah, that all makes sense. I don't think I ever realized how much of our current microphone technology is so size dependent. So why are you turning to insects to inspire the design of microphones?
Lara Díaz-García 04:23
Well, in the first place, anything that nature comes up with for evolution is generally speaking very simple and energy optimized, because of the principles of evolution itself. So if an animal evolves an ability, it will try to do it in a way that has the least energetic cost but still leads to good working capability it needs to perform. And in regard to hearing, insects are the perfect example for biodiversity because they have evolved hearing independently at least 17 times. Hearing organs can be found all over their bodies in shapes we might not necessarily recognize. So in comparison, ears in all mammals are in the head, usually recognizable enough having a pinna, which is the fleshy outer antenna. And that's just not the case for insects. In addition, insects are also generally small, which means some of the problems they encounter in hearing are the same ones faced by small-scale microphones, and some of the original solutions might be good inspiration for our designs.
Kat Setzer 05:25
Okay, yeah, totally. So you're specifically looking at the hearing of the lesser wax moth. And I'm gonna let you pronounce the scientific name from now on. Why did you decide to focus on the species?
Lara Díaz-García 05:34
Yeah, so Achroia grisella, which is at least that's the way I say it, is a small math that parasites beehives, with their larvae eating the wax, hence their common name, and they really don't look like much. So they have a small body size of an average of thirteen millimeters, or like roughly half an inch, if I'm not mistaken. And the interesting thing about the lesser wax moth, and this has been known since the 80s, because lots of experiments with these moths were conducted back then, mainly with agricultural interests. So we've known for a while that they use a mating call. So like birds who sing to attract their potential mates, the male Achroia specimens fan their wings, that produces a very high-frequency chirp that the females listen to and track to the origin of the sound. So this is directional hearing, like we were discussing earlier. And not all hearing organisms are capable of it. So it generally depends on having two ears at a certain distance, like mammals, and comparing the input received by each of these two ears, which will be slightly different and therefore allow the brain to estimate where sound is coming from. So if you're small, like the wax moth is, and your ears are too close, well, that trick, does just not work anymore. And to add to all of it, Achroia can also do this with just one ear because some nasty experiments that involved piercing one of their ear drums showed that they don't need it, they're not using binaural hearing at all, they can still hear with just one healthy ear. And something that we also additionally find very useful is that their hearing organ is pretty simple. So moths, in general, have some of the simplest ears in nature, and that's therefore easier for us to replicate. You would think that some complicated system is in place to allow them to have that directional hearing. So for example, it's well known that there's a parasitic fly called Ormia ochracea, that is known to have interconnected ears, so they're connected on the inside. But this is not the case for Achroia. So their ears set in their abdomen towards the front. And they just consist of a membrane that vibrates, so relatively similar to humans’ eardrums, elliptic in shape, with two halves of different thicknesses, and then it's directly connected to the auditory neurons that send information to the nervous system, which is unlike humans, because it's very simple. Humans have the cochlea and the ossicles, and a lot of things in the middle. So something else other than binaural hearing, or interconnected ears, purely depending on the shape and characteristics of their eardrums must be going on in the simple ears for them to achieve directional hearing. And that's what we're trying to test.
Kat Setzer 08:12
Yeah, that's so interesting that they have this directionality without the binaural hearing or interconnected ears. So what was the goal of this study?
Lara Díaz-García 08:20
So the goal of this study in particular, and my PhD more generally, is to try and replicate the structure of the moth eardrum in a synthetic material. So in our case, we use 3d printing with plastics, and see if we would observe a similar effect of monaural directionality, that it's passive. So it doesn't depend on anything that's going on in the moth; it's just because of the shape of the eardrums themselves.
Kat Setzer 08:46
Okay, so can you walk us through the process of developing and validating your model?
Lara Díaz-García 08:50
We started with a very simplified version of the model, basically a circular plate, and that behaves nothing like the moth year. So from there, we progressively increase complexity and similarity to the actual moth eardrum, until we reach the current model, which is an elliptical plate with two sections of different thicknesses, and then an added mass to account for the neural connection, and equations, computer simulations and measurements on real 3d printed samples were compared to each other. So the simulation was carried out with the multiphysics software COMSOL, and the software allows us to take into account things as varied as mechanical vibrations, thermoacoustic effects, diverse material properties, anything you can think of. And another strong point of the software is that it can be easily scaled up or down. So the model could be changed to match the bigger samples produced or the smaller actual moth ear without much trouble at all. So this way, we evaluated the natural resonances of the system in simulation. And once we check that the simulation results agreed with the simplest cases, which could be solved analytically through differential equations, we consider a model validated and look at how it agreed with the experimental results.
Kat Setzer 09:38
So how did the study turn out? Will we be seeing microphones based on moth ears in the future?
Lara Díaz-García 10:09
Well, we found out both in simulation and experimental measurements that the particular shape of the moth's eardrum does seem to grant it directionality. So passive structures were produced that provide diverse directionality patterns, which are key for directional microphone design. Now, these are still very far from being an actual microphone, like the ones you and I are using just now. But one day, eventually, in the future, they could lead to a moth-inspired design, or at least incorporate some element of its structure into another design.
Kat Setzer 10:42
Okay, I see. So was there anything about the study that you found particularly interesting or surprising or exciting?
Lara Díaz-García 10:47
Well, I think it's just fantastic to find out that this very small moth, which you wouldn't look at twice, has managed to circumvent a problem as important as directional sound detection of small scale, this is a big problem in acoustics. And it's just another really great example of how much there is to learn from nature, in particular, for innovative and efficient engineering design. So both in the use of resources and energetic cost.
Kat Setzer 11:13
Yeah, it is really cool how that nature has solved this problem like so elegantly, you know?
Lara Díaz-García 11:18
Yeah.
Kat Setzer 11:18
So what are your next plans for research?
Lara Díaz-García 11:20
Well, in the future, it would be great if we could use different materials for samples, so they are no longer passive and can instead produce an electrical output. So that can be done using piezoelectric materials, for example, and that would be a bit closer to an actual microphone, and also getting a lower working frequency range would be suitable for human speech applications, that would be ideal. Again, that can be done by switching materials or changing the size or manufacturing methods... But, yeah, just taking this a bit farther from the passive structures there are right now into something that actually produces an output.
Kat Setzer 11:56
That's so cool. I'm excited to see how your attempts to turn the ear of a moth into a functional microphone works out. It's so interesting, I know I already said this, that it could have that directionality without the multiple ears or listening points. Thank you again for talking to us about your research. And of course, once again, congratulations on winning the award from POMA.
Lara Díaz-García 12:14
Well, thank you so much.
Kat Setzer 12:15
Oh, of course! Our next interview is with POMA Student Paper Competition winner Mara Escarti-Guillem, about her article, Assessment of Computational Fluid Dynamics acoustic prediction accuracy and deflector impact on launch aero-acoustic environment." Congratulations on your award, Mara, and thanks for taking the time to speak with me today.
Mara Escarti-Guillem 12:32
Thank you so much, Kat, and thank you for welcoming me to the podcast.
Kat Setzer 12:36
Yeah, you're welcome. I think this will be a lot of fun for our listeners. So first, tell us a bit about yourself and your research background.
Mara Escarti-Guillem 12:43
Yes. So I was born in Spain, in Valencia, and I graduated from the Polytechnic University of Valencia in aerospace engineering, and also did my master's here in aeronautical engineering. And so about my research background, I first conducted research in during my master's degree because I did a research stay of eight months at the, well, I will say it in Spanish, at the Instituto Universitario de Mateàtica Pura y Aplicada, which is the Institute of Mathematics, basically, from the university. And so during this stay, I was doing my final master thesis. And this thesis is was part of a project for the European Space Agency, where we were doing some computational fluid dynamics models to study vibroacoustics during rocket and launchers takeoff. And so this project was being done by a consortium of different universities. But the leader of the consortium was the company COMET Ingeneria, which is a Spanish mechanical engineering company. And so they were interested in my profile and on what I was doing, so they propose me to do a contract and to do an industrial PhD with them. So I'm doing a PhD. And it's called industrial because instead of doing it only in academia, or university, it's inside of an R&D program. The company in this case is COMET Ingeneria. So right now, what I'm doing is doing both things, the PhD and also working at the company. And on what we're working right now is on both the prediction, so developing numerical models to predict and understand the noise and vibrations during launch of rockets, but also our final goal is to develop mitigation solutions that can reduce the acoustic loads during launch.
Kat Setzer 14:25
That's so cool. You get to have kind of that hands-on experience for the entire process and really get to see your research through the entire lifetime of the, you know, process of like how it gets applied and everything.
Mara Escarti-Guillem 14:36
Exactly, I think the industrial PhD is very interesting because you are inside of the industry. So you start to see how things work out, how to manage, also, which are the needs of the industry. And what is the most interesting for me is to do the transmission of knowledge from academia to industry. So you are doing a transfer of knowledge.
Kat Setzer 14:55
It's the application of it.
Mara Escarti-Guillem 14:57
Yeah.
Kat Setzer 14:57
So this study had to do with the vibroacoustic loading generated during the launch of space vehicles. Can you give us some background about space launches and the vibroacoustic loading that results?
Mara Escarti-Guillem 15:07
I think that the first thing that we have to explain here is what vibroacoustic loading is. And so vibroacoustic loading is the stresses and strains that appears on a structure due to vibration or sound waves. And so what happens during the launch of a space launcher? So the rocket engine is generating an intense pressure wave, which is reflected at the launch platform surfaces, and they are propagated towards the launcher. And so the noise propagates to the surface of the fairing, is transmitted through it, and then inside of the fairing of the rocket-- it's the upper part, like the nose of the rocket, usually we have a payload that can be a satellite or a telescope. And usually, this is why we send rockets to space, right, because we want to send or put something into orbit. And so this is the mission of our launcher. And so this vibroacoustic loading endangers the mission because it can break or damage the electronic and mechanical components of the payload. So due to this vibroacoustic loading, your mission is put in risk. And so, in fact, this vibroacoustic loading, it's a specification that you have to make sure that your payload has to be able to withstand, and there are other several dynamic environments. But the vibroacoustic is one of the most detrimental or more demanding in the design and manufacturing processes. So, this is why it's pretty important to predict and also mitigate these acoustic levels in order to enhance the reliability of the launcher, but also increasing the payload comfort.
Kat Setzer 16:34
Yeah, I can totally see why it would be so important to reduce that vibroacoustic loading and the impact on these very expensive, time-intensive missions.
Mara Escarti-Guillem 16:44
Yeah.
Kat Setzer 16:45
So what have noise mitigation techniques for space launch been like up until now?
Mara Escarti-Guillem 16:49
Yeah, so usually, the noise mitigation techniques can be separated or classified into two groups based on where they are applied: internally, so inside of the launcher, or externally, so for example, at the launch pad level. And so regarding the internal strategy, inside of the launcher, usually it involves using sound-absorbing or insulation materials inside of the structure of the fairing that, as I mentioned before, is the part where the payload or the satellite is placed. And so usually what is used is acoustic blankets manufactured with foams. And these are very effective at medium and high frequencies, but are less effective at low frequencies, that is where the payload has the structural dissonances. So it's where the coupling can be dangerous between acoustics and the structural behavior. And then regarding the external strategies to reduce noise, there are also different strategies. And the most common ones are, for example, increase the sound absorption because the absorption there is very low. So the most normal one in this category is the use of water injection, I think we all have in mind the typical video of a launcher taking off, and then we have seeing the water injection, the pressurized water being injected into the to the rocket plume, to the jet of the excess gases. So this is one very common, then another option is to avoid the ground deflections, because as I was saying, at the beginning, the rocket engine exhaust, the plume of the exhaust gases, and this is reflected at the launch pad surfaces. So if you design the launch pad in a way that your reflections are redirected away from your launcher, then acoustic loading that reaches the fairing, and at the end, the payload, is lower. So this is also another way. And the final way, or the final strategy is to decrease the noise that is emitted by the source. But usually this is very difficult or even impossible, because it's like you will say to the rocket engineer: "Okay, we have to use less propulsive power." And he will say, "No." So this is usually not an option.
Kat Setzer 18:50
Yeah, right. Understandably. So then your focus specifically is on the modeling of these launch acoustics to help develop better noise mitigation techniques, since it sounds like the ones that are in place aren't necessarily working 100%.
Mara Escarti-Guillem 19:02
Yeah.
Kat Setzer 19:03
So can you talk a bit about the models that are available right now?
Mara Escarti-Guillem 19:07
So the first point that we have to take into account is that experimental measurements near the jet, so near the rocket plume, during the launch are impossible because the environment is very hostile, it has high temperatures, pressure velocities, so it's very difficult, or I will say even impossible to measure in that environment. So there are a lot of different modeling techniques in order to do prediction. And this is why it's so important. And so usually, it's separated into semi-empirical models, sub-scale models, and finally numerical models. It's what I do. And so there is, there are some empirical models, there is a well established standard that was developed by NASA in the 60s, I think it was. So a lot of people use this standard, but the thing is that it has limitations, right. It has some accuracy and for the first design stages, I think it can be very useful, but at some point, when you need an increased accuracy, you need to give another step. So the next step will be to use a scale model experiment. So you can build a scaled up rocket and then measure it. But to understand and to study all the phenomenon that is occurring, it's complicated because the consistency between the scale model and the actual launch is challenging. So it's complicated. And then the final option is to use numerical analysis, which can provide detailed information on the flow behavior in the fluid domain. And what is most extended, what I use is computational fluid dynamics, which is also called CFD. And so what this is, is the mathematical computation of the fluid flow. And so what we compute is the governing equations by using computational power in order to predict the behavior of the fluid flow, such as, for example, velocity, and turbulence. And what we do with CFD is to resolve the Navier-Stokes equations, that are the governing equations. And so they describe the motion of the fluid and the conservation of mass, momentum, and energy. And so what is very important in numerical analysis is to compute the turbulence. So turbulence is characterized by irregular and chaotic fluctuations. And it's very important how you compute it. And so usually CFDs, it's separated in three levels based on the accuracy that you use to resolve turbulence. So the first one is direct numerical simulations, which is DNS, and this resolves everything, but it's pretty complicated, and also quite high cost. Then in the middle, we have Large Eddy Simulations, that it's also called LES. And this is in the middle of accuracy. So it's cheaper than direct numerical simulations. And you resolve a fairly good amount of turbulence, and you have quite accurate results. And then in the last part of the podium, we have Reynolds Average Navier-Stokes equations, that as the name says, what we are doing is averaging the Navier-Stokes equations. And so here, you don't resolve any turbulence or scale of turbulence, but you are modeling it. So you have some information, but the accuracy is not that good.
Kat Setzer 22:05
So you looked at the latter of these two types of models in this; you looked at Large Eddy Simulations and the Unsteady Reynolds Average Navier-Stokes. Can you tell us a bit about these models and why you chose to compare them?
Mara Escarti-Guillem 22:16
Yes. So first what we have to think is when you are going to decide which model you want to use is to analyze which is the problem that you want to solve and what is important in your problem in order to see which model you want to use. So for example, for launch vehicles, the acoustic loading is generated in every component due to the fluctuating component of the turbulence of the excess gases, so it's important to model turbulence. Then also there is a lot of physical phenomena that occur. So for example, the combustion side of the chamber, the supersonic flow compressibility, the lift of the vehicle, and so to model of all of this is unfeasible. So, we decided to do some simplifications. And this is why we started with the lowest level of accuracy, because this was the easiest way. But instead of using RANS that is, as I mentioned before, it's Reynolds Average Navier-Stokes equations, we decided to use the unsteady version of this model, because this model is an extension and solves the governing equations for the unstable average flow fields and also it retains a transient term. So the URANS model captures the very large fluctuations in the mean flow, meanwhile, RANS captures only the time average value. So it was not interesting for us because we could not track the evolution of the pressure in time. And so regarding the other model, that is LES. So this is an standard approach in the context of supersonic flows and supersonic jets. But the thing is that it demands a high computational cost to resolve the full scale at lunch, right? And so the main difference between both model is that LES can resolve the larger-scale turbulence motions, and so the small-scale turbulence is just modeled, and meanwhile, the URANS, so what it does is to model both the large- and small-scale turbulence by using a turbulence model. And as I mentioned our first models were based on URANS. And when we saw the limitations, so we first gained some knowledge and understanding of the problem. And then the next logical step was to develop an LES model. And the good part is that by using the code, DrNum, which has been designed it to make an efficient use of GPU computation, we were able to resolve this with smaller computational costs. So it was feasible to perform.
Kat Setzer 24:34
Okay, that's pretty cool. So the first part of your study was assessing the accuracy and performance of the two models with experimental data. So tell us a bit more about that. And what did you find?
Mara Escarti-Guillem 24:44
Yeah, so, well, I didn't mention it at the beginning. The thing is part of this work was done during a research stay that I did during the summer at the European Space Agency. And so this is why I was able to use the LES code that is based on GPUs, and I have to thank for this Oliver Gloth from enGits, who it's the owner of this code. And so at the beginning when we started to work, I was in contact with him and my supervisor from ESA. And in order to test the accuracy of both models, we were trying to obtain or to find some experimental data, but it's pretty complicated because usually this is, this belongs to the different space agencies or it's inside of industry. And it's not easy to have this information. So what we did was to look into the literature, and we were able to find an article that was showing some results of an a scale model. So we decided to use the study, or this information, to study the accuracy of our models. And so what we suspected was that the LES will have a better agreement with the turbulence as it can resolve the small turbulence scale that URANS is not able to do, so from the beginning, we were expecting this model to work better. And so the experimental tests that we reproduced was a scale model of ARIANE-V launcher that has two boosters, and they eject cold supersonic jet and the launch pad. And so with the URANS model, we were able to have some relevant information like how the mean flow is and also how the shock waves are and where they are placed. But it was very expensive. It took around two to three months to do a simulation, which is quite long.
Kat Setzer 26:20
Yeah.
Mara Escarti-Guillem 26:21
And then when we started using the LES, this model as it's on the article in the Proceedings of the Meeting, it has shown excellent agreement with the turbulence. And the most surprising part is that since it is very efficient, the simulations took for orders of magnitude lower than the URANS. So within a week, we could have very good results that were ready to be processed. So what this gives you is a tool that has good accuracy and also is fast because when you try to do a design process and your simulation time is three months, you cannot really do a lot of optimization with that. But with one week or even three to four days, then you can do some optimization besides.
Kat Setzer 27:02
That is very exciting. Yeah, just cutting it down by that much time.
Mara Escarti-Guillem 27:06
Yeah, yeah. By four times.
Kat Setzer 27:07
Yeah, it's amazing. Yeah. So in the second part of your study, you use an LES model to analyze the acoustic field of the VEGA launcher. How did you do that? And what did you find in this study?
Mara Escarti-Guillem 27:16
What we did was to study three different platform configurations, because as I mentioned at the beginning with the mitigation techniques for detecting the pressure waves, acoustic waves, is important to reduce, and also, it has been seen that it's an effective way to reduce acoustic loads right at the end the payload. And so these configurations were two different deflectors, one that was more simplified one and one that was optimized, and then also a platform without a deflector, so we had a flat floor. Because this is the worst case scenario, we wanted to see the improvement that the deflector gives. And so the study that we did was focused on both the noise sources, so the close field and also in the far field, that is around the launcher fairing, because this is the region where the payload is stored. So this is at the end, the main focus of all these studies is to try to increase the comfort of the payload. So to reduce the acoustic requirements. And what we found with our results and with our study is that the optimized deflector was able to effectively reduce the acoustic waves in a more efficient way, and this resulted in a lower overall sound pressure level in all the domains, but especially around the fairings. Also, what was interesting is that the deflector was effective to produce sound pressure levels that reach the fairing surface. So what this is showing is that, in fact, the deflector is a mitigation strategy that should be used for space launchers.
Kat Setzer 28:44
Okay. Very nice. So how did the two models end up performing? Did you learn anything about how to better mitigate launch noise with this?
Mara Escarti-Guillem 28:51
Yeah, so at the end of our two models, what we found out, as I mentioned before, is that the LES presents quite a good accuracy and also with low computational costs, which enables us to do different design stages or design processes to optimize the launch platform and different studies with also different configurations. And so regarding if we learned something to understand how to mitigate lunch noise, of course, these results have helped us to understand where and how noise is generated, and what we see is that the most important points are shockwaves and also the turbulence that is generated in the mixing layer, and the surface reflections. So these are the points to try to attack if we want to reduce noise. And in this study, it has been shown that the reduction of acoustic waves with deflectors is effective and that it mitigates acoustic loads. So I will recommend a launchpad optimization process for all the space launchers.
Kat Setzer 29:48
So it sounds like you've got a lot of information, just looking at these different models.
Mara Escarti-Guillem 29:52
Yeah.
Kat Setzer 29:53
And seeing how they work. So was there anything about this study that you found particularly interesting, surprising, or exciting?
Mara Escarti-Guillem 29:59
Yes. So I found very surprising at the beginning of the process, the limitation that we had with finding experimental information. This usually is a limitation of the work because at the end, when you have a numerical model, it is giving you some information, but you need to verify and validate your model. Because if not, you are only having numbers, and you don't really know if they are giving you the truth. So you really need to check the accuracy and verify if your results are correct. So without experimental information, this is quite difficult to do. So at the beginning, it was quite difficult to try to find information. But then also, it was very exciting when we were able to see the accuracy of the LES model and see also the results after some time. And it was especially interesting to see the pressure waves that were being generated by the turbulence and also the interaction with deflectors, because before, with our previous models, we were not able to see this interactions, so it was very exciting to finally see a more accurate result, a more realistic result.
Kat Setzer 31:02
And it sounds like that will be very, very helpful for your future research.
Mara Escarti-Guillem 31:06
Totally.
Kat Setzer 31:07
Which leads me to my next question, what are your future research goals?
Mara Escarti-Guillem 31:10
So our goals, as I was mentioning, at the beginning, it's based on both things, so do a prediction methodology and understand how noise is being generated because the final goal is to reduce the acoustic loading. So with the project that we're doing, with the R&D project were doing in my company, what we are doing now is to develop acoustic metamaterials for noise mitigation. And these metamaterials are based on Helmholtz resonators. And so a few months ago, we finished our second project with the consortium of scientifics that was funded by the European Space Agency. And we demonstrated the effectiveness of our metamaterials; we did the design, we manufacture the materials, and also were able to experimentally test the effectiveness. And so we designed two different metamaterials, and we were able to see and to measure an reduction of about 10 dBs in third octave bands. So with all this knowledge and expertise that we gained now, what we're starting to do is to do a new design and optimization process. So we expect to be able to provide an improved sound absorption solution. And yeah, I think I cannot really give more details about this right now. Because it's going on right now. But I think it's really exciting.
Kat Setzer 32:27
Yeah, that does sound very exciting, even with a little bit of detail you're allowed to give. Well, thank you again for taking the time to speak with me today, Mara, it will be exciting to see how these models can be used to help improve noise mitigation in the rocket launchers and to see how your current project with the sound absorption options pans out with the metamaterials. So good luck on your future projects. And congratulations again on winning this award.
Mara Escarti-Guillem 32:48
Thank you so much. And thank you for your time and the opportunity to speak to more people about this topic.
Kat Setzer 32:53
Of course. Our final winner of the POMA student paper competition from the 183rd ASA meeting is Kanad Sarkar. We'll be talking to him about his article, "Measuring and exploiting locally linear mapping between relative transfer functions and array deformations." Congrats on winning the award, and thank you for taking the time to speak with me today, Kanad. How are you?
Kanad Sarkar 33:12
I'm good. Thank you for having me.
Kat Setzer 33:14
Yeah. Very excited. So first, tell us a bit about yourself and your research background.
Kanad Sarkar 33:19
Yeah, I'm a second year graduate student at the University of Illinois, Urbana-Champaign. And I've been researching spatial audio for roughly four years now. I started as an undergrad doing research in these concepts. And now I'm continuing on as a grad student.
Kat Setzer 33:31
So your research has to do with microphone arrays. What specific uses of microphone arrays are you looking at? And can you give our listeners some background about what our understanding of these arrays' use has been like up until now?
Kanad Sarkar 33:43
Yeah, so microphone arrays are used for obtaining spatial information from an acoustic source, not just recording the audio, and the way they do so is they leverage the differences in how one source of sound reaches these multiple microphones. And using the differences in how the sound travels through space and hits each of these microphones, we can do a variety of tasks such as source localization, and source separation by applying filters, separate filters, on each of the microphones, a process known as beamforming. Most of these algorithms assume that our array has a rigid structure, maybe it's in a line, a uniform linear array, or a circular array, or even that our microphones are set in place during our recording, but it can have any structure to it. But there are a variety of scenarios where this assumption can lead to a decreased performance in our algorithms because you'll have scenarios where microphones are moving. Like there may be a chance where I want to incorporate phones as microphones in my spatial array processing devices that we can't assume to be stationary.
Kat Setzer 34:50
Okay, that makes sense. And I imagine since smartphones are so ubiquitous now, they might be used quite often in this type of setting. Okay.
Kanad Sarkar 34:59
Right.
Kat Setzer 34:59
So when we were preparing for this episode, you mentioned that there was a related paper that was important for the development of your current study. Can you talk about it and how it inspired this study?
Kanad Sarkar 35:08
So this paper was done by Ryan Corey and Andrew Singer. Ryan Corey was a postdoctorate when he first brought me in to my undergraduate research opportunity. He's now a professor at U Illinois, Chicago. He examined the performance of beamforming, which is applying the filter, separate filters, to each microphone to sort of steer the the array to listen at a certain region in space. He was looking at beamforming performance when microphones are also moving a little bit.
Kat Setzer 35:37
Okay.
Kanad Sarkar 35:38
And specifically, he's looking at adaptive beamforming, which is that our filters will converge to an ideal weight over time. And so as our microphones move, the adaptive filter will still be in this convergence process, trying to get the best weights for a desired signal that we know a priori.
Kat Setzer 35:57
Right.
Kanad Sarkar 35:58
So he did this wearable microphone case where he had microphones all throughout his body, and he was doing like dances or movements with the recording. And he found that, hey, our adaptive filtering methods work when I'm doing small deformations, but if I'm doing big, you know, dances like the Macarena, it would be nicer to have enough spatial information beforehand. So this paper sort of led to the need to search into how spatial information changes with the array geometry directly in a data-driven approach, sort of removed from this, like direct application of deformable arrays into our speech processing.
Kat Setzer 36:38
Okay, got it. That makes sense. So in this work, you're looking at microphone arrays, specifically arrays that can be deformed or moved rather than rigid structures, like you said.
Kanad Sarkar 36:46
Right.
Kat Setzer 36:47
So can you explain why arrays like this would be useful? You talked about it a little bit, but a little more information would be good.
Kanad Sarkar 36:53
Alright, so let's talk about conversation enhancement. Most algorithms for conversation enhancement, they assume that we have arrays that are set in place, or that we have arrays that move but locally stay in the same structure, like our air pods, right? But if we want to actually integrate things from multiple devices, we may not know things like array width, we may not know how these microphones are moving through a conversation, if one person decides to take their phone off and go on social media, and then put their phone back to the table to be integrated with the whatever conversation enhancement algorithm is going on. By placing a different way, there may be issues in how we calibrate our arrays.
Kat Setzer 37:37
Okay, yeah.
Kanad Sarkar 37:38
So viewing deformable arrays from that lens is important, especially in the age where a lot of people are looking towards spatial audio for these purposes of conversation enhancement. Another example would be a wearable devices for microphone arrays. Normally, we think, Oh, the smartwatch or our Bluetooth air pods. But if we think a bit bigger, and we have microphones distributed across our body, we have a lot of surface area on us to really pack in a ton of microphones and give us a lot of spatial control, just wherever we are. But the issue is that when we change our posture, or do things like breathing, those arrays will deform just a little bit, and that will have an effect on our processing if we don't account for it. So if we want to think about wearable microphones, even the integration of a smartwatch in these conversation enhancement scenarios, we're going to have a need to do some motion-tolerate processing.
Kat Setzer 38:32
Okay, yeah, that absolutely makes sense. So what was the goal of this study in particular?
Kanad Sarkar 38:37
So the goal of this study was to sort of take a step back from Ryan's work on motion-tolerant beamforming, and just look at a data-driven approach. So looking at manifold-based approaches, which means that we have a huge data set, essentially, and we're just examining this manifold or the shape of our data. And we base our work on this paper off of manifold-based approaches for source localization done by Laufer-Goldshtein, Talmon, and Gannot, and we can talk more about that later. But essentially, we wanted to see if we can apply the same principles found in source localization to array defamations, which makes sense why we can do that, but this is just to verify it for sure.
Kat Setzer 39:19
Got it. Okay, so you did a simulation on a binaural pair of microphones to look at the relative transfer functions. Can you explain the simulation and your goals with performing it?
Kanad Sarkar 39:29
So given two microphones, the relative transfer function is the filter that transforms the signal from one mic to the other mic, and it's neat, because we can estimate these relative transfer functions without knowing the signal and it directly has the magnitude and phase of this complex vector. The RTS is related to the interlevel and interphasw difference that we use as metrics for binaural spatial audio. So this complex vector can store information that directly relates to spatial information, without knowing the signal being played, the signal just has to play something within the bandwidth of the RTF factor that we have. And so we estimate, these RTFs at different sort of array configurations. And we hold the source position constant. And we do things like rotate the, the two microphones, stretch the two microphones. And that forms a mapping that we can then examine. And we didn't really look at more than two microphones under the assumption that all of our findings for this manifold will scale for when I have N microphones and a vector of RTFs, but then the data gets extremely large,
Kat Setzer 40:39
Right. And that's difficult to manipulate when you've got too much data.
Kanad Sarkar 40:42
Right.
Kat Setzer 40:43
Yeah. Okay, what is local linear mapping? And how does it relate to these deformable arrays that you're talking about?
Kanad Sarkar 40:49
You can look at any nonlinear mapping, a continuous nonlinear mapping, as a locally linear mappings. But what we mean by locally linear mapping is that our manifold can be closely approximated by a bunch of planes that are connected, low-dimensional planes that can be connected in our high-dimensional data set. So when I look at a continuous nonlinear mapping, there exists a region at every point of this mapping where I can approximate that region with a flat plane. But what we want is that region to be significant, that we can approximate without error.. And the way that we examine this for mapping is by examining different distance metrics on this manifold, seeing what distances relate. For example, if I stretch out my binaural array, stretch out the spacing between the two microphones, as I linearly stretch in Euclidean distance, my arrays, I want a distance metric that also scales linearly in my RTF that correspond to it. And the idea of a locally linear mapping means that when we trace along the manifold via approximations of the geodesic distance, only then we can find a metric that scales linearly with Euclidean distances on the space of deformations. And we wouldn't really find that using linear, like Euclidean, distance, or PCA-based distances. That's what we want to find. And this would corroborate Ryan's findings, which would mean that, like ,small deformations are linear, and can be approximated by a linear filter, but large deformations, you need to add some spatial information if you don't want errors.
Kat Setzer 42:29
You ended up applying a semi-supervised model to examine the problem of deformable arrays. How does this model work? And why did you choose it?
Kanad Sarkar 42:36
So semi-supervised model learns the mapping between RTFs and array configurations, using only a few of these array configurations actually labeled to RTFs. So essentially, I get a big file of complex vectors. And I only know a few of them in this big file that correspond to actual deformation parameters. And I say, definitely learn the labels I had but try to keep the structure the same from the RTFs to the array configurations. And in the act of doing this, they'll enforce the sort of local linearity and ensures that small changes in the RTF only relates to small changes in in our array configuration, and doesn't really do anything for large changes in the RTFs, all it does is enforce this small distance in RTF relates to small distance and poses, similar to how we would... that's just the staple to semi-supervised learning with manifold regularization. And it's just a different angle of showing that, hey, approaches that exploit, like, locally linear approximations with array configurations can be used. So one is examining the manifold using distance metrics. And the other is this model wouldn't have even worked as well, if we can't do these approximations.
Kat Setzer 43:59
Got it. Okay. So what did you end up finding?
Kanad Sarkar 44:02
So we ended up finding, for the first half of our paper we, where we examined the distances, we found that, hey, our mapping is very nonlinear, right? When we take like roughly geodesic distances, like diffusion distances, it does trace roughly monotone as we scale, our Euclidean distance of our array configurations. And you can view these deformations. For example, for spacing, if I have my spacing at 0.1 meters, I set that spacing as one parameter that's at the center of the two microphones and I scale by a distance and I scale from 0.1 to roughly one meter spacing. And I examined the manifold there. So the fact that diffusion distances are a valid metric means that we gain some benefit with having knowledge between positions that we're examining, right? The more data we have, the better here, because we can apply these locally linear approaches for that, right? At least for large deformations; for small deformations, we can make a linear approximation just fine. And what's interesting is that when the spacing is small, we look at rotations, that rotation for large range of rotation deformations, at a small array, it can be linearly approximated well, so this ball of deformations that we can approximate is related to the direct distances that each of the mics are traveling. Right? Because you don't see this with a widely spaced array. With a widely spaced array, we see something similar to just examining the spacing itself, where it's only linear for a small amount of rotations and a widely spaced array. So that leads us to the finding that, hey, you know, it doesn't matter what parameters we use to parameterize our deformations. If they're parameters that relate to large deformation in Euclidean space, it's only going to be linear for a ball. As a parameter changes, if it leads to small deformations in Euclidean space, it's going to lead to maybe a larger ball for which we can approximate linearly. We also found that the semi-supervised model worked really well.
Kat Setzer 46:19
So you don't need to do all of the work yourself. You could have the machine to some of it.
Kanad Sarkar 46:24
Yeah, yeah, exactly.
Kat Setzer 46:26
Yeah. Okay. So where do you see this research going in the future?
Kanad Sarkar 46:28
Doing this manifold analysis was cool and all, but I don't think that examining this further is the path for future work. I want to see with the knowledge that, hey, this is experimentally confirmed that we can use this, methods for how we smartly store information for adaptive filtering algorithms for beamforming.
Kat Setzer 46:49
Okay.
Kanad Sarkar 46:50
Right. And maybe there is potential as well to combine deformable arrays with stationary arrays for processing and examining how we do that. So maybe like having an array on the table, but I still use my phone, I can gain some insights that way as to how I initialize my data. But I think the goal in the future for future researchers interested in this field should also be looking at how you apply it in the actual conversation enhancement setting.
Kat Setzer 47:19
Yeah, totally. That makes sense.
Kanad Sarkar 47:21
Yeah, yeah.
Kat Setzer 47:22
It sounds like being able to account for the flexible microphone array structure can be really helpful... to be very vague about that. Thanks for taking the time to speak with me today. I wish you luck in your future research and your future studies.
Kanad Sarkar 47:35
Thank you.
Kat Setzer 47:35
And congratulations again.
Kanad Sarkar 47:37
Yeah, thank you.
Kat Setzer 47:38
So for any students or mentors listening around the time this episode is airing, we're actually holding another Student Paper Competition for the 184th ASA meeting in Chicago. So students if you're presenting or have presented, depending on when you're listening to this episode, now's the time to submit your POMA. We're accepting papers from all of the technical areas represented by the ASA. Not only will you get the respect of your peers, you'll win $300, and. perhaps the greatest reward of all, the opportunity to appear on this podcast. And if you don't win, this is a great opportunity to boost your CV or resume with an editor-reviewed proceedings paper. The deadline is June 11, 2023. We'll include a link to the submission information on the show notes for this episode.
Thank you for tuning into Across Acoustics. If you'd like to hear more interviews from our authors about their research, please subscribe and find us on your preferred podcast platform.