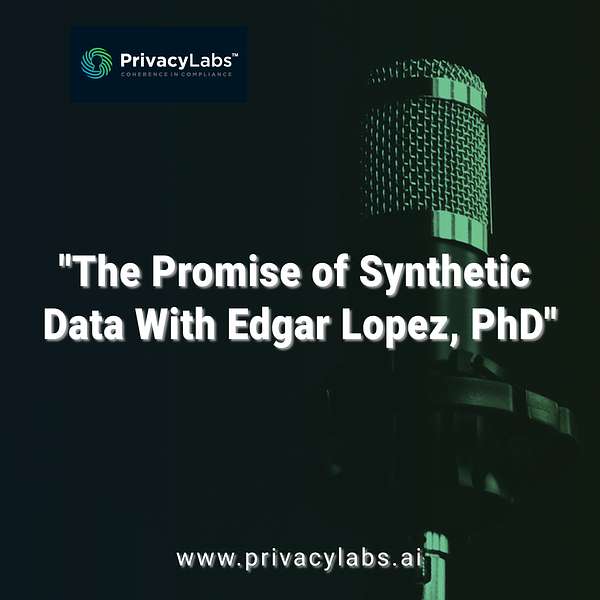
PrivacyLabs Compliance Technology Podcast
PrivacyLabs Compliance Technology Podcast
The Promise of Synthetic Data with Edgar Lopez, PhD
Hello and welcome to this podcast, I am going to be having a discussion with Edgar Lopez Rojas PhD, who is founder and CEO of Ealax, E A L A X. And Edgar, I'll ask you to explain that the audience here when we when you introduce yourself, but Edgar is a pioneer, and definitely at the top of the area of synthetic data, specifically, with regard to financial crime, anti money laundering and in similar areas. Again, this is for PrivacyLabs, is one word. And we specialize in compliance technology. And I'll go into that more at the end of the podcast. But with that said, Edgar, welcome. Thank you so much for your time. If you would tell us about yourself and about Ealax, and then we'll jump right into the questions.
Edgar Lopez:Hi, Paul, first, say thank you for the invitation to this podcast. guys say I mean, we we know each other. So I'm very pleased to actually be your guest in this podcast. This let me tell you about myself for the audience, a originally from Colombia. So I was born and raised in, in Medellin Colombia, the same time of the same famous guy, Pablo Escobar was running his business time, I mean, he made a quite a lot of a profit from the criminal drug traffic, and the dirty money actually came to my country and started to influence the society in terms of corruption violence. So just to make my story short, I Well, my background is in computer science, I did a bachelor in computer science. And when I had the opportunity to actually study abroad, I went to Sweden to do a master's degree and then a PhD. And the PhD I did was in topic of a money laundering detection, a and the first a the first frustration, that I had during my research, I mean, every PhD is to then have frustrations during the, during this research a but mine was data, I mean, the data sets one of the crushing things to actually tackle complex problems, like money laundering, and I was a I was having a background in machine learning. So I was eager to start applying random forests on whatever I could I could do for for the state of the art, but the data was just not there is basically confidentiality and and the privacy of the the customers actually is one of the topics probably that, that you're also interested in on this. But I'm happy to tell you more about it. I'll just let it go back to you.
Paul Starrett:Yes. Okay. And I, you've also been a pioneer in the area that you're talking about. You've been in it for close to 10 years, I think. So I think that's important for people to know that you have been in this area for a long time. And I've seen it grow up and develop. So is that an accurate statement?
Edgar Lopez:Yeah, so so just to continue the story about the my research, I started to have the frustration of not having data. So therefore, I started to look for alternatives. And one of the alternatives was the generation of synthetic data is more specifically synthetic financial data to tackle problems. So of financial crime. And in the beginning, I was just using synthetic data as a replacement for real data. But then later on, I started to understand a the interest of the community for a data sources to actually go deeper into this. So in 2017, I outsource one of the research projects I had this is called the PaySim simulator. And we say that the source was a positive in kaggle. And it became quite popular over there. So So I didn't know exaclty what was the value of synthetic data. But now I think after several years of trial and error, I have started to assert that there is an interest in the community and the market requires a solutions that only can be developed to synthetic data. And we can talk a lot about some of the projects that you and I have been participating like the Digital Sandbox in the FCA is one of the examples.
Paul Starrett:Yes, I think that's a great idea. And what we'll probably do is get into that towards the end of this so that people we can introduce people to the topic and learn more about how it works. I also want to just mention the PaySim is probably one of the most used synthetic data set in the banking industry and it's PaySim one word that you developed yourself. That's why you're considered one of the pioneers in banking and finance with regard to synthetic data set a fair statement?
Edgar Lopez:Yeah, thank you for that. Yeah, I would say I was one of the pioneers and also a not only the pioneer by have been one of the most consistent researchers in the area. Because sometimes people start a topic and forget about it. But I think the motivations I explained to you the very beginning, it led to me that this is something that I really, really want to create this as a mission statement for my life. And that's why I think the there's a lot of work that can be done with this. And I, I would like to see the results of my research has been implementing the real world and probably thats one of the reasons why Ealax is to actually to see this research implemented and to tackle complex, complex problems of a.
Paul Starrett:So it's not just a commercial venture for you, it's a passion and something that it's close to your heart, because I know that you started out with solving the issue, excuse me, of your country that you came from. So getting right into what is synthetic data. Now, I'm going to give a little definition here. Feel free to expand on that. And correct me if I'm wrong. I have learned a lot about this, by the way, in the last three months, and we will be planning a video. But synthetic data is essentially what you do is there's there's various components that we'll cover each one in a separate question. But the idea is to take real data that a bank or some institution has this generally in a highly regulated area. And because of that the data protection privacy laws restrict what can be done, as well. And we'll discuss this later, in machine learning. Oftentimes, even if you have access to the original data, synthetic data and approaches that are used, can be used to help grow out and simulate different scenarios to give you a broader set of data from which to learn and generate new intelligence from it. So starting out with the synthetic, just the basic idea is to take the original data, and basically create a new, not fake, well, it is fake, but it's synthetic, it's new data, that is basically go ahead,
Edgar Lopez:Let me help you out with the definitions in synthetic data okay, to the simplest as possible. So basically, synthetic data is data that is, is being generated by by the computer or by an algorithm. And this data resembles or we say, an abstraction of some real situation in the world. So basically, we use synthetic data as a way to a to make our model to represent a certain situations in the real world. So the generation of synthetic data is is a science that has been a it's been going on for for a while, I think several years of computer evolution have made a generation of synthetic data more popular nowadays. But there are so many ways to actually generate synthetic data. And one of those who say is the one that the approach that Ealax uses, and is a to use a real data as a reference for for, for generating the more realistic synthetic data. But some people might just experiment to generate that data without any real data. And what happens is that what you create is like what what I call a toy example, or a toy model. And these toy models can be a well it can be very inaccurate. So they'll say, because there's a difference of using a synthetic data with real data without really,
Paul Starrett:I'm sorry, I think she said,what type of data? And you mentioned something the word didn't come through?
Edgar Lopez:Yeah, so so what I mentioned is that you can use synthetic data, and we use it as a model, real datasets, or you can use it without a real data set. And when you use it without real datasets, and you create something like a toy, you model, right. So model helps you to have certain understanding, but those models are very inaccurate.
Paul Starrett:Got it. Got it. Thank you. So, so there's various values that come from synthetic data. One is what they call privacy preserving technology. So by the fact that one you are creating, whether it's toy data, certainly that wouldn't be private, because it's completely fresh, freshly. You know, it's it doesn't exist anywhere in the world. But if you're using real data, the abstraction you mentioned, gives you some obfuscation of the original data thus giving you some preservation of the privacy that was underlying the real data, the original data. And that gives you some freedom with regard to how you can use the synthetic data as compared to real data.
Edgar Lopez:That's true, Paul. So there is a trade off between a privacy concern so I'll say disclosure in real data using synthetic data and the analytic power of synthetic data. So, the more analytic power we have in the synthetic data will look closer to the real data and therefore, the disclosure will, will go up. So, so there is a there is some was like a balance point where we have enough analytic value in the synthetic data and at the same time we protect these patterns or these, let's say the anonymization techniques that will help us to actually identify who's the real subject behind.
Paul Starrett:I see. Great, that's great way to put it. Now, another area I think it's really important in, in machine learning, is explainability. And I think we've discussed how synthetic data can help with that process in exposing certain features or or other information. Is that a fair statement?
Edgar Lopez:Yeah, I think I think explainability is a very interesting areas, especially in the domain of financial crime, and is pretty related to the gathering of evidence, I mean, the you cannot explain why an algorithm has been a tagging someone for suspicious activity, it's very hard to actually tell the authorities that this person is doing something wrong. So therefor explainability is one of the key issues in in some of the domains like financial crime, I can, I can also tell that there are so many other domains that explainability is important to make the algorithms, fair, unbiased, to avoid these say, things like gender issues, and stuff like that. So So I, in my opinion, method explainability is something that there's still a lot of work to be done. But once you actually have the synthetic data assets, in you will have the possibility and capabilities to actually develop better this algorithm explainability. So So basically, synthetic data is a mean, for achieving a or getting these expandability levels, you call it.
Paul Starrett:Very good. Yes, well said. And then last, on this specific topic, is with regard to the ability to take the original data set, whether it's toy or real, and expand on that in to find new patterns. So in the example of Ealax, one of the things that you do well, is find hidden fraud, you find things that were not known before, that you're able to either find anomalous behavior, that is usually indicative of fraud, but you also can inject or what we call topologies. into the data set to help flesh out the data set and help increase the value of the model to find, you know, fraud accurately,
Edgar Lopez:Well said, Paul. Well, I think one of the beauties of using synthetic data is the flexibility that you that you can have, once you actually have a proper model always is robust enough that the validation gives you certain trust, then you can go to the next level, and you say this is something that the Ealax has been working on is to enhance the synthetic data to enrich it with a certain pattern, certain behaviors. Some of the some of these are driven by the expertise. So people that have been dealing with this issue before some of those of dealing with the historical data. But the capabilities of actually enriching the synthetic data sets. It's one of the advantages of actually of using synthetic data over real datasets.
Paul Starrett:I see. And I think we both know well that Gartner has predicted that it's something above 60% of all machine learning training will be based on synthetic data by 2024, which is right around the corner.
Edgar Lopez:That's fantastic. Paul, I totally I totally think that Gardner is right. He's in the right part. So in the in one of the latest report of Garner, in December 2020. It says that by 2024 60% of the AI will be trained using synthetic data. And you think about this, Paul, and I believe it's true, basically because generating synthetic data perhaps is not the easiest way in the very beginning. But in the long term is the the most cost efficient way. You have plenty data and you have it just in the tip of your hands when you need it. So it's been said that many of the data science projects, a 80% of the time it takes to get access to the data, and 20% of the time just just to use the data. So you want to achieve this, this product to, to actually make it a like you use simulation 20% of the time and 80% of the time, you just use the synthetic data assets to actually perform you, your machine learning algorithm. So testing and training.
Paul Starrett:That's interesting that it's cheaper, perhaps to generate synthetic data than to try and claw it out of wherever else it might come from, and to, and to include things that you've learned from other sources that you can then learn from that and include it into this synthetic data set. Great. So I think I think that kind of does it on this topic. But I did want to get down into specifically in financial crime and anti money laundering, and that you're, you're working in that area specifically, if there's any thoughts you want to
Edgar Lopez:One of the things that makes me realize that we are in we are doing the things right at the Ealax there is a big problem in financial crime. And the big problem is that it's very hard to understand if the financial controls are working properly or not. And one of the reasons is actually because of the hidden crime. So the hidden crime is basically the crime of the financial institutions a never never really get to see or to detect. And therefore they need to pay for that or they just say never know that existed until it's too late. And perhaps the authorities come and issue some sanctions and fines. And at that times too late because the criminals are enjoying the vacations or permanent vacation to Bahamas or enjoying or something. This is this one of the things that really makes me think, or maybe started to think how we can turn the things around. And one of the one of the the ideas I have is to use synthetic data in a very similar fashion as a as a vaccine. So So you think about for instance, the Coronavirus vaccine is the first thing that they do is that they isolate. They say they the malicious part of the virus and then they they put a harmless virus inside your body, for your body to understand what what can go wrong when it's inside. And you develop these these defenses before the virus hits you. And this the same thing we're trying to do with synthetic data. So we're we're trying to stress the financial system with harmless synthetic data before the financial criminals actually start to abuse the financial system. So this is the this is the concept that we have nothing is very sound. And we have we have reached a point that the technology is ready for doing these things. Because I think for this to be a possible there was an interest in funding, there was a lack of interest in the industry. And in the last two years a regulators like the FCA have been promoting the use of synthetic data for innovation, and specifically for innovation in the area of financial crime like Coronavirus. It changes a lot. And I associated these two terms because the way that Coronavirus affected the financial system, it changes the behavior of the people and therefore the controls that were made for the for the normal, normal don't work anymore in the new normal. So they need to evolve. And I think one way to evolve very fast is to actually stress the system before it's intended to date. And that's all valid purposes.
Paul Starrett:Got it in on that point you wanted to we didn't want to discuss the FCA project that is where we met and it was an early this year 2021. And do you want to tell our audience a little bit about that?
Edgar Lopez:Yeah. So just to mention a little bit in 2000. A little bit of background is in 2018 a many things went wrong in the financial system in the UK. Therefore the regulators it totally was was the right time to explore new opportunities to assess how to tackle financial crime for a project problems. And in 2019, I came to the UK as a consultant for the FCA. And I worked with them and teach them how to do a how to generate synthetic data. And in 2020, I was lucky enough to already have Ealax and we I participated in in one of the tech sprint the FCA runs every year. And this was it was called Data Sprint because the main goal was to generate synthetic data assets. And then so to my surprise, a big project was a was actually the Digital Sandbox, which is an initiative from the City of London and the FCA. And these two institutions together, are investing a lot of resources to actually make possible for the FinTech industry to test their solutions before. And one of the solutions that Ealax has been testing is the generation of synthetic data using real data.
Paul Starrett:Yes, and that was used in this tech sprint. And FCA is the is the financial crime authority is that
Edgar Lopez:Financial Conduct Authority is the Financial
Paul Starrett:Conduct Authority. That's the body that regulates in the United Kingdom. Right. And also that that sandbox was used to test new theories and to have the invited various invited various, you know, commercial entities like Ealax also to come in use it...
Edgar Lopez:its own context, a around 100 companies apply the for being a part of the first data sandbox pilots, only 30 companies made it only 12 companies of these 30 were working in the area of financial crime. And only one company was a specialized in the generation or synthetic date and our field
Paul Starrett:I see.
Edgar Lopez:That's one of the reasons why we are pioneers in this area.
Paul Starrett:Yeah, absolutely. No, and I don't think we can understate that at all. Well, great. And I think we're gonna see more sandbox like environments where synthetic data is used, where the government and industry can come together and help solve problems in various areas. So I think that does it. Is there anything else you'd like to tell the audience I would like to have you explain where they named me Ealax came from, but give you also the opportunity to, to say any parting words that we haven't discussed that you think the audience should know about, if anything, if we haven't covered anything.
Edgar Lopez:Yeah, well, first, thanks very much, Paul, for this space. I think one of the missions I have in my life is actually educate the general public about my research to try to try to put them into simple words, and they can stand it like with the analogy with the Coronavirus, but seeing something that people can relate, and I think these spaces are a wonderful opportunity for that. And I will make sure that the Ealax a promotes this podcast. And also well, the just to mention the the meaning of Ealax, it's an acronym in Spanish, that means 'E' at the power of 'X'. So so I want to represent the exponential growth. Hopefully, it's going to be the grow my company.
Paul Starrett:Right. And I think for some of the nerds out there, 'E' is a constant 2.7 something rather that's used very often.
Edgar Lopez:Yeah the Euler number,
Paul Starrett:Yes, right. And'ala' means 'at' and then 'x' is the exponential. Yes. Well, that's great. I think it's a neat, neat way to name your company. So Well, great, I think Edgar, then we are thank you so much for your time and for your efforts, not only making it a better world, but in the passion, you have to make the world a better place. And so with that said, I just very briefly, you know, PrivacyLabs will continue to work with Ealax to help make this available this this technology, we specialize in compliance technology, we can assist with bringing this to various projects and engagements. And we cover sort of the horizontal aspects of, of implementation. Because we are again, a compliance technology firm. So if people want to get in touch with you, Edgar, I guess they just Edgar@EALAX.com.
Edgar Lopez:Okay, yeah, that's correct. And you can also Google my name Edgar Lopez Rojas, and you're gonna find a lot of hits if you mix this with financial crime. Yes. So to say, Paul, thank you very much for the invitation. I'm really looking forward to to develop this partnership with PrivacyLabs. So so so we can conquer the US market together.
Paul Starrett:And that we will do so we've got the best in the business on both sides. If we do see ourselves. Alright. Thanks so much. And you have a great day. I know you're in the United Kingdom. So it's dinner time for you. So have a wonderful day. Thanks again. And thank you audience for listening.
Edgar Lopez:Same for you Bye.