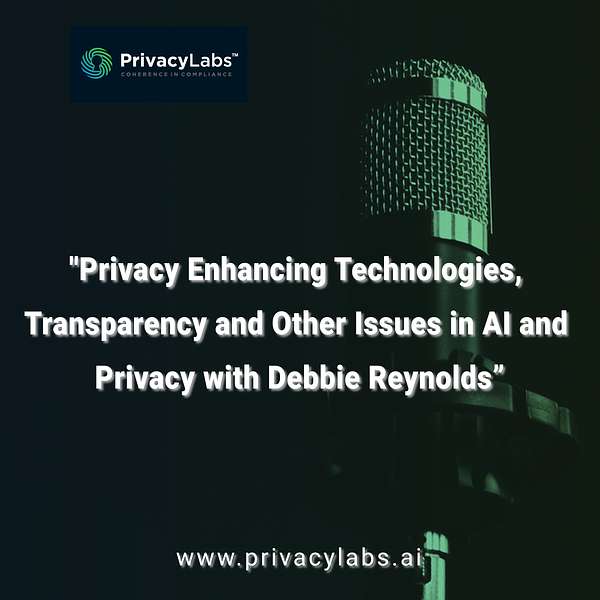
PrivacyLabs Compliance Technology Podcast
PrivacyLabs Compliance Technology Podcast
Privacy Enhancing Technologies, Transparency and Other Issues in AI and Privacy with Debbie Reynolds
Hello, welcome to this podcast. This is Paul Starrett founder PrivacyLabs. Remember, PrivacyLabs is one word. I will tell you more about what we do at the end of this podcast. Today I have the pleasure of having Debbie Reynolds of Debbie Reynolds consulting, often known as the data diva. She's one of the most prolific people that I know, in this area. And I don't think is anything that she doesn't know. And I also want to mention these probably one of the most selfless people that I know. So I've had a great pleasure of working with her in various capacities. And Debbie, go ahead and introduce yourself. Tell us about your who you are your your, your firm and some of your accolades.
Debbie Reynolds:Sure. Well, first of all, thank you for inviting me on your show. We've known each other for a number of years. And we always have very excellent conversations about privacy and data science. So and you're the person that I always call up and someone asked about data science, happy to be on your show. And your clients are very lucky to have you. So, yeah, I guess he did a pretty good introduction of me. My My name is Debbie Reynolds, they call me the data diva. I work at the intersection of privacy, technology and law. So I'm a technologist and I help companies navigate privacy issues as a relates to technology. Some of my people ask me about these accolades, it's hard for me to even think about, so I do a lot of writing a lot of speaking, I think I have like over 100 plus, publications, media mentions, speaking engagements for all types of companies related to privacy and technology. Two recent accolades that I received well, three is my podcast is voted the top five privacy podcasts in the world, and also voted top 20 by the threat technology magazine, for data privacy, right podcast. And then earlier this year, I was voted top 20 worldwide cyber risk communicator.
Paul Starrett:Oh, fantastic. And you were also featured in Fortune magazine in March of 2021. Yes, yes. Yes. And that's kind of what I what I meant by accolades, because I thought that was very impressive. And yes, you are very prolific. And one of the reasons reasons I mentioned that is on your website, people can find all kinds of dozens and dozens, maybe hundreds of videos, by the way, which I see in my feed on LinkedIn regularly, which are great is there you know, five minutes, and it's video so we get to see you there and it's always an interesting, progressive topic. So I just wanted to have our listeners know that that's what you do and there's all kinds of other things on your website. And just so people know it's fairly easy as DebbieReynoldsConsulting.com and it's just like the the actress the famous late actress, right?
Debbie Reynolds:Yes correct. Yeah. I was actually named after Oh, you were Yeah, very nice. My brother had a crush on her at that time when I was born. And then he convinced my parents to name me after her. So
Paul Starrett:yeah. That's awesome. That's awesome. Well, it you get to carry on the name, I suppose in some respect. So that's kind of why I wanted to mention that. And given that we both kind of live in the technology side of things I. And we're sort of like you said, a data science. I wanted to try and touch on some of those topics. We've had some recent podcasts on some very specific topics. But I definitely wanted to pick your brain. What do you think about synthetic data? And I know that that's fairly, I don't know, it's very new. I mean, it's been used a lot, but it's fairly new. But what are you seeing there? What is the promise of it that the challenges that you're seeing from your perspective?
Debbie Reynolds:Yeah, I'm going to favor any and all ways that people can help protect data. So so synthetic data is one of those newer ways that we're seeing, the companies are trying to protect data. So the idea behind synthetic data is that they take real data, and they replace some of the attributes or certainly information with fake information, especially in areas where that that individual data is not needed. So it sort of operates like real data. But But the key is when you're using synthetic data it's made to be used for a certain purpose. So if someone were to steal or breach synthetic data, once they took it out of the environment, it would no longer work. So it will give them people false information, or wouldn't work at all. So it's kind of a way to say, you know, as long as the data is in this environment, you can use it, right? Do whatever that you need to do with it. But once it leaves that environment, whether voluntarily or not, the person doesn't have, you know, if they're not in that environment, and don't have kind of the keys, quote, unquote, to be able to see that data, then they, they can't see it.
Paul Starrett:Right. I guess you touched on something that we really haven't covered in some of our previous podcasts. A couple that I want to get your thoughts on one other thing here in a minute, but is the data breach side of this is that if you wanted to retain some private or sensitive information, that you wanted to generate the, the, I guess, what they call the signal, the value of the underlying data, you could do that. And not worry about the breach because we we know how important or how ominous data breach cases can be from the standpoint of first you don't want to have your data breached just from a moral standpoint. But then you have the ensuing regulatory, class action lawsuit, some of the other reputational damage that comes with it. So that would be a great way of looking at the value of synthetic data.
Debbie Reynolds:Absolutely. And that's me, that's one of the biggest reasons why companies are looking at implementing, and then we're seeing a lot of businesses creating these types of technologies for that reason.
Paul Starrett:Mm hmm. From the standpoint of moving data from one jurisdiction to another, and I know this is an area that you are very, you have a lot of expertise in as far as the data movement and the very laws of the various countries and jurisdictions. One thing with this synthetic data does help with is movement across borders. So if somebody has machine learning effort, for example, in the EU, another one in Mexico, and other one here in the States, I mentioned those because they know Mexico's very tough on data transfer, that they can do that now is that they can share those insights. They know that they're no longer stuck with the insights they get from one data set in one country and another data set and other, do you see that as a promising area for this right? Because it's kind of obvious it would be but how promising. Do you think you see synthetic data and data protection data cross border movements?
Debbie Reynolds:Yeah, it is. It is significant. But I guess there are two things so almost any privacy law that you can think of has a exception related to data that has been anonymized in some way. So, for a business to be able to reach, it's kind of a low bar, in my opinion, in order for a business to reach the legal standard of anonymization is doable. Obviously, you know, there's work involved a lot of work involved in order to get it that way. But if you can anonymize that data, you can be exempted in some ways, against some of this, these data transfer issues that you run into. The flip side of that is that from a technological perspective, the legal standard for anonymization isn't strong enough. So I read a statistic recently that, you know, some data brokers have over 11,000 data points on individuals in the US. So just taking out just I'm just oversimplifying this, right. So just taking out maybe their people's names and certain things may not be sufficient from a technological perspective, to anonymize data. But you know, that's kind of that's the issue that we run into now. So you can do a anonymization project, using things like synthetic data for data moves. And that may may satisfy kind of the legal requirement. The problem is that companies that have more insights on people can re-identify them. So we're seeing some laws or some movement in places like Europe to try to make it illegal or bar companies from re-identification efforts.
Paul Starrett:I see. It's almost like making it illegal to reverse engineer copyrighted materials. Exactly. The DMCA, were Digital Millennium Copyright Act, I think. Interesting. Well, I guess that's, it's about time, right? Yeah.
Debbie Reynolds:Right.
Paul Starrett:So great. And, you know, another aspect of synthetic data being used as a, what I call, you know, privacy preserving data generation tool, or technology is this thing called the privacy budget. So they say that as you in the context of machine learning, or where perhaps we have a SQL based system, where you're querying something where machine learning isn't necessarily in the picture. But the idea is that the more you preserve the privacy, the less valuable the data becomes, the more it becomes its signals, if you will, with this, the insights you gain drops with an inverse relationship. There is the cost to make the data private. But there's also the expectation of the jurisdiction, where you are doing business or you plan to do business that has a certain strict or not level of privacy, data protection rules. So if it's if the, let's say that they're from one to 10, or six, let's just say it's, I don't know, France, or something, where they're very, very strict on their privacy rules. So if I have a project that I that involves artificial intelligence, or some other form of automation, that if the data has to be so constricted, because of the privacy preservation, that I wind up losing all value of the underlying data, then I just don't even do it at all. So you get this thing where if it was the other way around, you're in a more of a lax environment, but maybe in the United States, some state that doesn't have much in the way of a privacy law, or privacy preservation or data protection law. And I have a product that I know is going to be very useful, that that product then may be doable in the States, but not at another one, because of the amount of protection that you you have to put around the data to preserve the underlying sensitive information. Does that make sense?
Debbie Reynolds:Yes, it does actually. So as you make that a real example, so there is my can't remember, what the company, I don't know if it's Sony or whoever. But anyway, there's a big tech company that has a robot dog that they cannot sell decided not to sell in the state of Illinois, because Illinois has very strict Biometric Information, Privacy Act called BIPA. So and it has very high fines for every incident and which data is captured about individual like, their voice or their face, or, you know, fingerprints or anything like that. So that's that's kind of a direct example, I will say where, you know, and I think the we've seen over the years with GDPR, some companies saying that we think the cost of complying with GDPR may be too high. So let's not sell our, you know, our tool or our service or something in Europe. So I think it's definitely there. I think the thing that companies get wrong about this, in my opinion, is that I think Apple has proved this out with their recent iOS 14.5 update is that you can preserve privacy, and they can make you money. Because you are, you know, customers have appetite now for companies to help them preserve their privacy. So instead of seeing it as being a loss leader, maybe it can be an advantage to help you, you know, be a differentiator in your industry where you say, hey, we respect your privacy, we'll do X, Y, and Z, and then you'll get more customers. So it's not, you know, I think companies need to think of it more long term, and then the broader sense. And then, you know, transparency is the new black as far as I'm concerned. So, you know, if you're not being transparent, you're not gonna make money period. So if you're stuck in these old ways, or old data silo types of, you know, ways that you're dealing with data, you're not going to be successful because the future is about transparency with the user. And then I tell companies, you know, when you're handling data that belongs to someone, it's not your name is their data, and you're a steward of the data. So if you switch your mindset to that, then you'll be fine in the future, but if you're otherwise we're swimming upstream.
Paul Starrett:I see, that's a great extra sort of facet of this whole thing is that you can look at it from the standpoint of the privacy budget on the technical side of you just looking down that rabbit hole. But if you pull back and say, yes, you build trust with your, your clientele, and your businesses that you do business with, when they know that you are careful with their data. So that's, that's a positive, it's it is a commercially enabling kind of a thing to do. That's that's a great point. And think about it that way. With regard to automated human decision making, where are you seeing that going? I know that there are rules around the transparency there. So that if somebody is in again, I'm speaking from a place of general understanding of this, and I'm sure you have a much more refined and finely grained understanding. But the idea is that automated decision making, that if somebody wants to have an explanation, because they have been impacted by a decision that's been, it's been released or being decided upon by this automated process, they have right to have it explained. And so the machine learning, artificial intelligence has to be explainable and transparent to your point. So that's kind of what's leading me to think of that is because the transparency, going with that theme, there's another place in artificial intelligence, where I think, the law is. Where do you see that now? Where do you see it going?
Debbie Reynolds:Yeah, that's, I love your question. To me, this is one of the biggest, most important questions that is in front of us today, what you just talked about. And so the problem in my view is that we sometimes are, we're seeing technologies being implemented in ways that make people think that the computer is always right. And then the development of AI isn't transparent to the user. And then the harm to an individual can be catastrophic. But you may not see that because the harm may articulate itself in different ways. So I feel like sort of like the movies with the evil robot where, you know, I don't know, I don't know about you, but all these movies about the future is like the dystopian future where the robot take over and though the humans are helpless, and that Terminator, yeah, I think that that is an abdication of responsibility for humans, because we're told we rely entirely too much on computers. So I think that computers and AI and machine learning, you know, they need a human to be able to make those judgments to say, okay, this is right, or this is wrong, it'll do as much as you want it to do. But it may not apply the way that it should do in a human situation. So I think having humans be able to make those final judgments is really important. What one example that I give people about the harm of AI and and why it's different is, let's say, let's say, in a situation, let's say you go to the grocery store and buy lettuce. Okay, so you bought lettuce from the grocery store, and then you get a message saying, oh, well, you know, this batch of lettuce that you bought from grocery store, made, you know, 50 people sick and then we want to recall it. Okay, so that's something people will totally jump on. You're like, oh, my God, like this made 50 people sick, and then we need to do something about it. The problem of AI is that if someone is harmed, maybe maybe harm and 50 different ways, so it's hard to even quantify what the harm is. So let's say facial recognition camera picks up someone and thinks they look like someone who committed a crime. That person may be arrested, it may impact their life, maybe impact their livelihood. You know, someone may be put on a less aggressive track in their education as a result of, you know, AI decision making not run amok. And then, you know, things are medical, you know, there, there's an example of facial recognition, for example, being used in skin cancer detection for people and, you know, their situation where they found that this thing that this application of facial recognition was very accurate on people with lighter skin, and not accurate on people with darker skin. So they were giving someone a false result, and they will end up in a later stage or cancer because they trusted this machine, it wasn't, you know, calibrated for someone of that skin color. So I think, you know, you hit the nail on the head, I think it's, you know, we we know, the, you know, as a data person, know, we can't just let the machines take over, we can't abdicate our responsibility. And and, you know, humans have ethics. So machines don't, or AI doesn't. So I think we have to be the ones to bring the ethics bring the judgment there.
Paul Starrett:Yes, those that's very well put. And I'd love your examples to the broad, a series of examples and in ways that can impact people. And then in more than one way, so someone is recognized as being someone who is a thief and they were arrested. Sometimes you can't unring that bell to say, Yeah, you're arrested. I was innocent. Oh, sure. You're innocent. No, there's, you can't unpunch, you can't unpunch someone. So that's very interesting. You know, and just while we're on that subject, one of the areas that we look closely at, in fact, we have videos, PrivacyLabs, videos on this very topic on explainability. And the different types of technologies that we can use. There's a whole series of very promising technologies that help us understand how a models working, because, for example, simple models are generally easy to understand, but they're not very performant. The most complex models are very performant, but are blackbox. So you get this kind of, again, another inverse relationship. I think from the standpoint of AI and privacy preservation and transparency, that's a pretty good coverage. Is there is there anything else that you see that you'd like to talk about here, with regard to the technology side that some things you're seeing that are at an issue in the next year or two? Again, this is 2021. What are your thoughts there,
Debbie Reynolds:I think there's gonna be a lot of changes related to third party capture of data. Because we're already seeing companies like Apple and Google try to shift risk of third party data sharing. And this goes back to many privacy regulations. So almost any privacy regulation that you could think of now have a feature where it says, you know, if a customer gives you data, this is what you should do with it. And then if you have to transfer it to a third party, here is your responsibility. So it's no longer a situation where you know, the first party company, they can do whatever they want. And then once they transfer it to a third party, they can't this wash their hands and say, well, we didn't know what they did with it. So we're seeing companies limit the data that is going to third parties, unless the third party can get consent from the individual. So this is going to be really tough, right? So think of data brokers, the Data Broker business, they're basically changing that. So I think that will be maybe for some data brokers who can't adjust, just that'd be like an Extinction Level Event, you know, so let's say, let's say, you know, what I see is, you know, data brokers either going out of business either getting absorbed by other these big tech companies, so they can say, this data transfer isn't a third party transfer, because I'm with this other company, or we're going to see these data brokers try to create incentives for people to give them data, like, you know, instead of me making zero, let me give you part of what I would make, so then you can give me data or whatever. So I think it's gonna be really crazy, probably from next year or two years to see how all this you know, pans out because, you know, we're seeing people get more savvy, to say about their information, and we're seeing these regulations. You know, be pretty tough on businesses when they do these third party transfers. So they can't really wash their hands of it unless what they're doing right now saying, you know, I, as a business business have limited my third party data transferred to was the absolutely necessary, and then I didn't give them more than what they need.
Paul Starrett:Wow. I wasn't, I guess I didn't really see that coming. I mean, at some level, I could. But that's, that's interesting. That's that is pretty ominous, isn't it?
Debbie Reynolds:Yeah, it's gonna be crazy.
Paul Starrett:Like it's synthetic data, we kind of roll back to the first part of the conversation would be maybe something to pay attention to more. Yeah, I
Debbie Reynolds:think it'd be really popular. I think, in order to stay in business, these companies are going to have to do some drastic, different things they were doing before. So yes, maybe they'll buy one private jets that have to I don't know.
Paul Starrett:Yeah, there's big money in this stuff. Okay, well, I think, is there anything else you want to add or leave with our audience?
Debbie Reynolds:Oh, I don't know. Well, I've had a great time on the show. And we've all had you and I over the years, we had great conversation. So I'm happy to see you're doing this work. And so thrilled to see you know, your videos, we do a podcast, because I think it's just something that, you know, your voice really needs to be out there and be heard. So I'm really thrilled about that. And happy to support you.
Paul Starrett:Thank you. Well, you have actually, and you have inspired me, I can give you that credit right here. One of the reasons I started with the videos is because I saw you doing them, and I thought, Wow, that's pretty effective. a pretty good way to do it. So, and again, I do give you a shout out to being probably, I don't know, maybe one of the best resources out there for what you do. So. So anyway, I guess. Well, thank you so much, Debbie. I think we'll roll it up here. But I, I'm positive that we'll have another opportunity for another podcast here in the next, you know, near term here. So I would look forward to that. And yeah, and just as I finish up here, PrivacyLabs is privacy compliance technology company, we specialize in using tools we call unify, in order to bring the entire team in the entire effort together around tools like One Trust, BigID and so forth. We also specialize in automation, cybersecurity, and in audit, in particular audit, especially around AI and such. So I think we'll end it here. Thank you again, so much, Debbie. And look forward to the next podcast and please do look up Debbie, if you are interested in some, you know, some top level advice, and maybe we'll wind up working on a project together from this. I
Debbie Reynolds:don't know. Yeah, me wonderful.
Paul Starrett:Okay, thanks, Debbie.
Debbie Reynolds:You're welcome. Okay. All right.