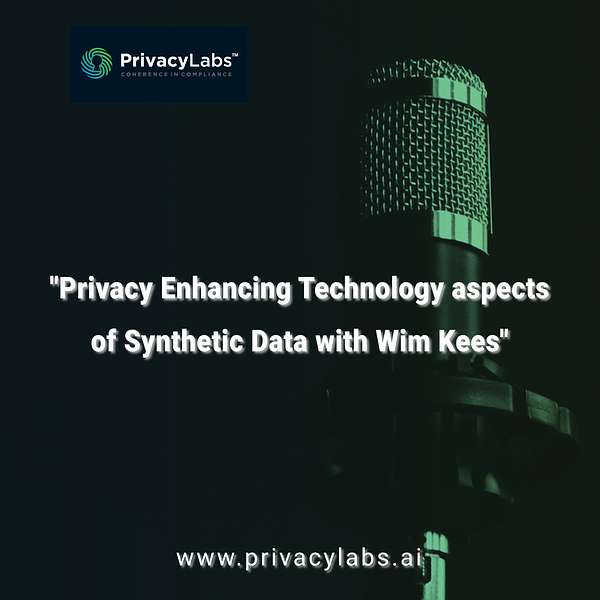
PrivacyLabs Compliance Technology Podcast
PrivacyLabs Compliance Technology Podcast
Privacy Enhancing Technology Aspects of Synthetic Data with Wim Kees
So welcome, everybody. This is Paul Starrett, founder of PrivacyLabs. Remember, again, PrivacyLabs is one word. And I'll discuss more about who we are and what we do at the end of the podcast. But I have the privilege of having Wim Kees on the phone on the call here. He is the founder and CEO of syntho.ai, a company that specializes in synthetic data. Specifically, I think around privacy related benefits, but I'll let him explain that here in a minute. Wim I met sort of inadvertently, I ran across his website, we've since had some nice discussions about his technology and, and how we can leverage it in the in the professional area. So with that said, Wim please tell us about yourself and your company and, and learn more about you and your company.
Wim Kees:Yeah, thanks, Paul. And it's always great to start a podcast with such an amazing introduction. Happy to be here. Yes, my name is Wim Kees Janssen, it's a typical Dutch name. from the Netherlands, in Leeds, founder of Syntho. We started Syntho, one and a hlaf years ago, really far from facing data privacy challenges in practice. So me myself working in, in software development, typically faced legal contracts, risk assessment, or really time consuming trajectories, typically also energy draining not only for myself, but typically from all involved parties. That's how we started Syntho. specialized in data, synthetic data. And yeah, we will deep dive on that probably during this podcast. So stay tuned on that.
Paul Starrett:Yes. You mentioned some things that were sort of pain points, like contracts and regulations. Can you expand on that your experience with that? And how that sort of, you know, resulted in your your company?
Wim Kees:Yeah, yeah, we typically see organizations really have higher ambitions to realize data driven innovation. So the opportunities are huge. We have AI, machine learning, IoT, we have strong computers now. So the opportunities are really, really, really, really huge. And at the ambition level is high. But on the other end, we really see our society. Yeah, worrying about their, what companies do with their data. So what they what are they doing with the data? Where do they store it? And what insights do they get from it? Yeah, and this as reaction, we see, really strong privacy regulation. So in the Europe, we have GDPR. And us we have CCPA. Really strong regulations, and those really conflict. So on the one on the one hand, we have, we see organizations really aiming to realize data driven innovation. And on the other hand, we really see regulations and privacy, really hindering that, that innovation. And what we see at the moment is that I would be need solution for those dilemmas, we need solutions to overcome and to innovate, both to realize those interfaces, but also to preserve privacy and to, to comply with regulations. And, and that was really the starting points I had really aiming to solve this practice global privacy, that dilemma and bridging this gap. So that's, that's really where we're coming from and why we started Syntho
Paul Starrett:on it. Yeah. And that makes perfect sense. It seems like one of the best practices now in order to leverage machine learning, because we know that machine learning helps you save money, reduce risk, and remain competitive. And so if you don't have some of these solutions, like what you have, it's more difficult, and even maybe not possible to get there. So we certainly see the value, I know is with a legal background, and I'm here in the States, of course, it may be a little different, but I think the legal legal systems are roughly the same, that you have contracts that may require a certain level of privacy preservation, you've got the regulations, as you've said. So with that said, I just want to make at the outset here, excuse me, that synthetic data is can be used for many things explainability for enriching models, you know, running simulations to build out some of the synthetic data. For example, one of the companies that I have worked with this company called Ealax. They are very focused on financial crime and anti money laundering. But I think in your case, and correct me if I'm wrong, you are. One of your strong points is that you focus on the privacy aspect of the data, rather than necessarily fleshing out other labels and features and so on. Is that a fair statement? And if you could then explain how what you do, roughly how it works?
Wim Kees:Yep. Yeah, that's correct. Indeed, we focus on that privacy aspect are really coming from that background on aiming and, and mission driven and solving the editing the global data privacy dilemma. And as an introduction to synthetic data, I think how we typically explain it is that original, ethically sensitive data is collected and generated in all your interactions with your clients, internal processes, are really generated by the source. And opposed to that we see synthetic data and synthetic data is generated by a computer algorithm. So that's the really key difference of synthetic data. And the benefit is that it's completely new generated data. So we synthetic data is completely new generated data points. And I think the key differences, what we do at Syntho is that we, we have a machine learning model, so we apply AI to capture the value statistics and patterns of the original data, so that we can generate synthetic data and reproduce those same statistics patterns and relations in the synthetic data. So that's what we do at Syntho. We have the idea to use synthetic data as alternative to to using the original sensitive data. So therefore, we always ask this question. So why use real data when you can use synthetic data. And typically, we always say, on one end, you can, of course, reduce the amount of original data that you that you use within your organization, and thereby reduce, for example, data breaches. But on the other end, you can lock a lot of personal data. Because we see a lot of personal data and data is restricted due to all kinds of regulations, and you're simply not allowed to touch that. So it also opens, really opens a lot of of data that you briefed you previously, would not be able to touch. So I think, at that, that's what we do at Syntho. Really, I generated data reproduces that this statistic, statistical value in the synthetic data so that you can use synthetic data as an alternative to using the original sensitive data.
Paul Starrett:Yes, and you just said quite a bit there. And I think we've I've got some questions now this is the nice thing about the pad podcast is that he had this sort of like mining for oil. And we we tripped over a vein here. I think one things you said that I that I really keyed in on was that, for example, data breaches, if you're able to take a synthetic version of the data, breach of that data is certainly much less risky, if risky at all, right. So you automatically are removing risk from your environment, if you if you use that data, there's the risk is much less because data breaches are so ominous, the laws are so draconian, in a class action lawsuits are horrible. But you also got into some issues, when issues that I think a lot of people kind of don't, can't quite quantify at the outset is what I've heard is a privacy budget. So when you do sort of obfuscate, or you put the noise in the data and the synthetic data, that that sort of removes the personal data, the personal aspects of the data, there's the value of the data can sometimes be lessened. You know, the the machine learning algorithm then loses some of its performance. How do you normally work with that? And typically, do you find is it always the case that it's a net gain, or the cases where it just the privacy budget, so to speak, just for the audience, the privacy budget says the more that you preserve the privacy, the underlying data, the the ideas that you also lose some of the value that you found in that data originally. So maybe you can explain the concept a bit and then what you're seeing out there as far as if you have 10 clients, it always works or it works for most, what are you seeing there?
Wim Kees:Yeah, I think an interesting definition, the privacy budget, I think we see it in typically we have more than a classical randomization techniques. So with classic anonymization techniques, what they have in common is that they all basically, I just manipulate original data in order to make it more difficult to trace back individuals. And examples of those techniques optimization, generalization, data masking, data suppression ability, basically, what you do there is you manipulate the data set in order to hinder tracing back individuals. And that is as of course, two disadvantages. So on the one hand, it always stays stays the original data. So you will always have privacy risks, and on the other end, you destroying the data data and thereby reducing the data quality With those classic anonymization techniques, you always have a sub optimal combination between both. Because if you, if you, if you apply those techniques a little you have really high data quality, but low data privacy protection. But yeah, the only solution is to apply those techniques more. And what happens then is that, that you get more privacy protection with that the data quality drops. That's really the trade off that we typically see with classic anonymization techniques. And that we want to overcome with synthetic data. So we have synthetic data, how we generate completely new data points. So individuals simply do not exist in synthetic data. And I typically explain that by we generate completely new data. So there's one, there's no one to one relationship anymore with the original data. So that's, that's basically, best explained by the fact that we with synthetic data, we can generate unlimited data. So we can generate more data than the original data, so that one to one relationship is not there anymore. And of course, it can be a disadvantage, because you cannot trace back individuals. But of course, that's one of our key selling points, combined with the fact that we apply AI to one of those in such a way that you can do analytics on synthetic data. So alternative
Paul Starrett:I see. Interesting, and I know there's different types of privacy, re identification attacks, where people can learn things that I don't think I really have time to get into that. But it sounds like based on the way in which you approach this. Do you find that? Is it typical? Is there ever a case where the amount of privacy protection you have to have basically doesn't didn't result in a net gain, but there's so much loss of value in the underlying data that it just doesn't make sense? Do you see that? Or is it fair to say that there's always a reason to use synthetic synthetic data for privacy preservation? Is that a fair statement? Or is an overstatement? What's your thoughts on that?
Wim Kees:Yeah, I think, yeah, we always, that's why we always ask why is real that when you can use synthetic data, but it is one of the disadvantages of synthetic data is that you cannot trace individuals back because they simply do not exist anymore there. And that means that all operational related use cases where you require at the original data cannot be cannot be performed on on synthetic data. So yeah, typically a typical a typical operational exercise, for example, as sending the invoices to your clients. Yeah, there's not really advised by us to send, send your invoices to synthetic addresses. Because Yeah, I can promise you that that you will not get paid, then those use cases will not be possible.
Paul Starrett:I never thought about it that way.
Wim Kees:But maybe this also, but this also explains a bit the the concept of synthetic synthetic data and generating artificial data points and artificial addresses.
Paul Starrett:Yes. But then you can glean like for customer retention, right, you can maybe help learn from the data to to to find out how customers are behaving at a high level, right? or other other high level? Yeah. So can you always make the case, though, for that in that situation? That synthetic data will always result in a net gain? I know Sure. If you see where I'm going with this, a lot of times people say for example, when we use artificial intelligence, there cases where you don't have enough data. It there's no real, but you know, the parameter parameterizations that's required. Our audience may not know what that means. But there's certain things that have to be there for a machine learning model to be a value. Sometimes there's not it's not there. Yeah, you just don't use it. So in the case of price of synthetic data is with regard to privacy preservation. Is it? Can we say that it's always going to result in a net gain? Or is there are there times where you look into it, and you just decide this? There's so much loss of value in the data? It's just not worth doing? Do you ever see that?
Wim Kees:Yeah, I think what we typically have as rules for rule of thumb to to generate high quality data is a minimum amount of data have because without if you have too less data, the AI model cannot get to this statistic and patterns. And as rule of thumb, we always say a minimal level of, of 100 data points per column is advised to make sure that that the value is captured properly by the model. So I think that that's a rule of thumb that we that we typically that we typically see and yeah, that's only a threshold basically, that we that we see with with with high quality synthetic data.
Paul Starrett:I see interesting From the standpoint of now, there's a Gartner article or Gartner will an article that says that 60% of all machine learning will be used will be using synthetic data by 2024, which is right around the corner. Do you agree with that, and I met when imagine that that is encouraging for your business plan? How do you feel about that statistic? Do you think that sounds right to you? It's Gartner. So we can assume it's probably credible. But this is curious, your thoughts on that?
Wim Kees:Yeah, still, it's not 2024. So we have some time. But I think a big step needs to be made before we are down because we see many companies are not familiar with the concept of synthetic data at all. So I think there are some some, some teaching and development and learnings need to be done before they actually start embracing synthetic data. But this is one of the use cases that that we go for, because what we see, especially with with machine learning models is that on the one hand, data, scientists typically face challenges in getting access to the data. So So typically, coming back to the risk assessment, legal contracts, and and data s data access procedures, they are typically long, and you do not want your data scientists to waste time on that. And especially since data scientists sometimes had our highest from an external party, as consultant they had, and then there are there are also a third party and then then that, that that challenge, where it will even become bigger. Yeah. So we aim to overcome that with synthetic data. So So our use cases to use synthetic data for for data, data science modeling, as so that so that data scientists can can start immediately with developing their models. And parallel, start with with setting up their data data access, procedure procedures. And then once once done with the model, they can either stay on the synthetic data because it's high data quality is high and actually can be machine learning models can actually be trained on synthetic data, where we see only a performance loss of 2%. But I think I think in the future, I foresee that there will always be value in highlighting those machine learning models work on the original data. And then we see that okay, after the model is developed, that the model will be run with with the original data. But then really value that that's added there is that the data scientists can start immediately. So there's no waste, there's no waste of time. And since most organizations, organizations work agile, it's kind of a value added to also have an agile data access infrastructure. So that's, that's the that's real value added agility, but also open innovation. Because your colleagues can now have access to representative data in any minute without any, any roadblock. So it's really also an and a starting point to realize open innovation. Yes, I think that's where we see the essence. So typically, yeah, no risk, more data, faster data access, and representative data. And that's that's basically the the foundation to realize data driven innovation, and especially with AI.
Paul Starrett:That's a great point. I don't hear that as often as I think I should, the Agile development process. And now you have, you know, serverless and containers and, you know, Docker and CI/CD, you know, continuous integration, continuous, continuous development. For those in our audience, things are moving to much more of a smaller packaged approach to delivering software, from the time it's developed, the time is actually pushed out into the enterprise environment where they call production. That's really fascinating I, that makes I did not come up before, but that is a great point. The other one is, I think the ability to, I'm using the word portability, I know that it's under GDPR. That's a different term, but the ability to share it with other to democratize the data so that you can then share insights with other people, other entities within your enterprise across borders. So these data protection laws to prevent movement of data across borders without you know, contracts and onerous hoops to jump through. So that's that's I think it's pretty promising. I it's hard to see a downside.
Wim Kees:Yeah, exactly. Yeah, I think I think the promise and indeed also with with data sharing, yeah, why share your original data when you can also share synthetic data, especially in proof of concept. Procedures also really, really valuable because if you have that synthetic data warehouse internally, you can also not only your colleagues will benefit from your static data warehouse, but also your external stakeholders, or maybe vendors or suppliers that need data from you can also benefit from it because you can maybe open your synthetic data warehouse with with those third parties as well.
Paul Starrett:Or you could even sell it. I suppose
Wim Kees:You can sell it, indeed. Yeah, that's, that's indeed also. Also one.
Paul Starrett:Use it for transfer, transfer learning.
Wim Kees:Yeah. Yeah. Yeah, yeah. So those are the interesting use case need. And we really see see the value of synthetic data indeed, in the fact that you have not risk, you have more data, representative data and the growth easy and fast. That's the foundation for data driven innovation.
Paul Starrett:Interesting. It sounds to me like I've kind of covered the basics. We discussed what it is how you do what you do, the benefits, we covered the challenges. So I think well, what I'd like to do is give you an opportunity to, is there anything that you think our audience hasn't heard yet that they should always like to give the people that I interview here, the opportunity to do that. So anything that you think we haven't discussed, or you'd like to emphasize, here's your opportunity.
Wim Kees:Yeah, I think I think we covered most of what we do, but I think, yeah, we are we are a Dutch organization. Founded in 2020, we won the Philips Innovation Award in the Netherlands. We are in the IBM hyper protective accelerator program. And we are funded by ten capital cybersecurity deck finance. So I think we also really backed by, by by, by, by, by a strong, strong, strong cohort of of experienced organization. So I think that's also something that, that that should be mentioned here as well.
Paul Starrett:I agree. And your background, maybe a little bit more about you your
Wim Kees:Yeah, yeah. So actually, my background is in economics and finance. So I started economics, accounting in a city in the Netherlands, and then to Rotterdam, its city and also city in the Netherlands. Yeah, and that's from that finance. I started my career in financial sector, but transformed internally, more towards software development in the innovation area, and there, yeah, faced a data privacy challenge challenge. In practice, from software development, and realizing data driven innovation, I saw the opportunity, really to bridge this gap. That's, that's how we how we started Syntho in it. So yeah, I came from from economics in the financial sector. And from that, from that move to the software development data, and now here and in privacy tech, so a great journey. So to say,
Paul Starrett:Yes, and I know that you are bet your, your Well, Your support is strong, and that you're, you've had many real awards and stuff people go on your website, as well as Syntho.ai. And if they want to get in touch with you What? What email address would be a good one.
Wim Kees:Yeah. So my email address is W.k.janssen@Syntho.ai. So that's my email address. And you can also visit our website at this Syntho.ai. There, you will find a really big blue button saying contact. If you press that, and then that form will end up in my email box. I see also work.
Paul Starrett:I see. That's probably the easiest way we just do people know the spelling of Janssen, is that right? That's correct. Yes. Okay. Yes. Just so people know, because in the States, when they hear Janssen they, it brings up various possible spellings. So So, so I think that will do it for us. Thank you so much. Just a little note about PrivacyLabs. We, it by the way, you gave me a little bit of a pitch unknowingly in there about the contracts. That's something that I can work with. I can help bring the the legal and the data science together as one of our values. But we did PrivacyLabs, we unify projects, we can work with automation. We also work with cybersecurity. And then with audit as well. You can see more about us atPrivacyLabs.ai as usual. Other than that, I think that'll do it for us today. Thank you so much, Wim. And I would imagine we could have another follow up on this podcast on a different topic or subtopic, but I really appreciate I'm sure this is very, very valuable information for our listeners. So, thank you again and have a nice weekend. I guess it's coming up here.
Wim Kees:Yeah, thanks. Thanks for having me. Really great, great podcast and definitely in for next next one. So, right.
Paul Starrett:All right.