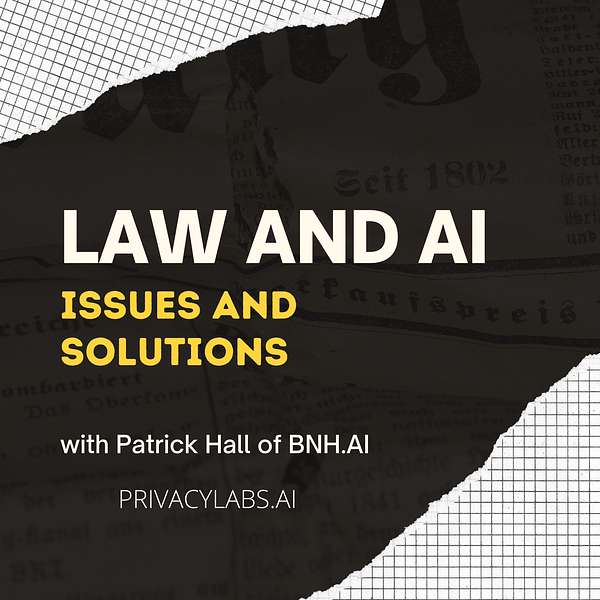
PrivacyLabs Compliance Technology Podcast
PrivacyLabs Compliance Technology Podcast
Law and AI: Challenges and Solutions with Patrick Hall
In this podcast, we interview Patrick Hall, a renowned data scientist, about the legal issues related to the use of artificial intelligence. Partick is co-founder of a law firm, BNH.AI, along with attorney-partner Andrew Burt. We discuss the ubiquity of AI and challenges related to balancing compliance with the commercial quest. Transcript included.
Hello, and welcome to this podcast today. Again, this is brought to you by PrivacyLabs. Remember, PrivacyLabs is one word, we're actually hoping to get our Registered Trademark here in the next month or so, which we're very proud of. We are a technology compliance technology firm, we do have strong capabilities in machine learning. And you can find out more about us on our website at privacylabs.ai. I'm very pleased and honored to have Patrick Hall here today, I had to book him out two months in advance, he's such a busy and sought after guy. And also, again, when I'm very delighted to have because for for a number of reasons. One is because PrivacyLabs really does focus on bringing compliance and the technology together. And much of that is built around machine learning and artificial intelligence. And Patrick's firm is a law firm bnh.ai, B for Burt and for and an H for Hall. And they do something very similar. So there was a we've had some great conversations and back and forth about that specifically, what we're going to try to do today is really sort of pull out the value that that brings in that. Really, artificial intelligence is something that it's not a corner case, it's not a piece of something, it's kind of like the heart and the body, you don't see it. But it's vital. In order for firms and organizations to be competitive. They are leveraging machine learning and AI more and more. And that brings with it compliance issues, legal issues, and other just basic is this stuff working like we want it to. So I think Patrick, what I'd like to do is give you a chance to explain what you who you are, what BNH is all about. And then we'll start into some open ended questions about how broad of an issue this is, and then maybe get into some specific things that you thought would be good to bring, which I agree later on in the podcast. So Patrick, welcome, thank you.
Patrick Hall:Oh, very, very glad to be here, I kind of dive in with where you left off. Previous to founding bnh with, with my co founder, Andrew Burt who deserves a lot of credit. I worked at the machine learning software firm H2O, and ran data science product there for for a number of years. And what I found was, every time we wanted to do something really impactful, and actually this goes back to my days with SAS, and I imagined that, you know, it's it's a fairly kind of universal problem. You know, every time we wanted to do something impactful about people, about customers about patients, um, you know, there's this phase of the development cycle was oftentimes the very last phase, the very end, very heartbreaking, you know, phase of the development cycle, where someone asks, oh, my God, is this legal? And, and very oftentimes, it it's not that it was illegal, but but just the questions were kind of unanswerable about legality and you know, that that sort of increased the risk for the whole product and made people nervous. And, and I just saw that happen again, and again and again, and talk to others in the community. And, you know, they were experiencing the same issue and so that we founded bnh, to help companies, you know, whether they're software vendors, whether they're, you know, retailers, whether they're insurers, whoever it is to, to help deal with these very nasty, tricky issues of when AI and machine learning systems collide with the law, which they often do.
Paul Starrett:Yes, I couldn't agree more. And I think this area's matured actually, pretty nicely, or fairly nicely, but I still think it's critical to have a hands on or eyes on, at the detail level at the finely grained level, like what your firm could do, and / or what PrivacyLabs could do that the devils often in the details.
Patrick Hall:Yes, it is.
Paul Starrett:Yes. So even though we have, you know, maybe the law is kind of maturing, kind of growing into this, you know, a, you know, GDPR touches on the law of artificial intelligence, we now have a EU directive, and so forth. But that that's still a requirement. I think synergy that you and Andrew have together, really kind of helps bring kind of coherent, holistic approach. That brings me to my really my first question is that is a fair statement that machine learning and this is based on your experience and what you've seen that machine learning is really, even in the mid middle market, besides market, let's say billion in revenue more is becoming the rule. It's really doing the heavy lifting. That was not possible before given that this becomes really a center focus of the enterprise quest. It's not just oh well AI is down over there. Or it's IT or legal? No, but it's a fair statement that this is, albeit maybe not, you know, on the agenda for the, you know, the board meeting. Is that a fair statement?
Patrick Hall:Yeah. And I think that caveat you brought up right at the end, really important. I will at least agree to this, I will at least agree to this, that all manner of companies and government agencies and nonprofit organizations, you know, I, I see them feeling a pressure to adopt machine learning and AI technologies, many of them have, and, you know, that caveat that that you brought up, you know, a lot of times boards have risk committees, right, a lot of times boards are where, you know, at least certain matters of risk kind of come to rest. And yeah, if if the AI or ml project is kind of buried down under some middle manager, which is just fairly typical, then these matters of risk may not be, you know, getting the right type of oversight. And, and they, you know, if we just take a step back and kind of peel, peel off the hype here, we're talking about systems that make decisions about millions of people very quickly. And it's just very obvious that those would have, you know, legal liabilities associated with them, if not any number of other risks, like, like you brought up I mean, just just does it, is it doing what we think it's doing would be another fundamental risk. So, so yeah, I can, I can agree with that, that that, you know, it's becoming much more common, and many companies are feeling this pressure to adopt.
Paul Starrett:Right. And I think it's also given that that's a conceptual approach. I think. It's also part of the guts of the enterprise, both the data flows, the infrastructure, the technological structure, the workflows, all of that. A few examples, one would be, you know, is the data private? Does it require that you have some sort of, right, and it really slows down the data acquisition process,y eah, we can talk about synthetic data in a second, because I think that's I've been seeing some interesting things, we talked about bringing that up. But I think that's a fair statement that when you have this model that you're building, just for the benefit of our listeners, when you build a model using software and data and hardware, you have to monitor it, you have to, you have to basically audit the process as you build it to show what you've done, how your decisions were made were privacy regulations considered in the process. But it's not just in that little environment, it touches it spreads out, does it not? To the way people
Patrick Hall:It certainly can.
Paul Starrett:Okay. I think that yes, I always I tend to round up. But I think on the only point I'm trying to make here is that it's not siloed it's doesn't have to be the center of everything, necessarily. But I think that's in your experience. That's a fair statement.
Patrick Hall:Yeah. And, and that the audit process that so lots to talk about, and what you just brought up, so So I mean, legality of data collection is all not all of a sudden, but say over the past few years. I mean, that's become a very difficult, almost universal question. And then, you know, the audit process that you mentioned, I think that many, I think, again, if we just kind of take a step back and try and try not to think about the hype, right? If there's other mission critical IT infrastructure at your company, it's likely audited, it's likely documented, there's likely some kind of legal oversight. And I just find that people in in the rush to adopt AI and machine learning sometimes, you know, the those steps get forgotten about the audit and about the oversight about the documentation. And again, that's why risk doesn't filter up to the levels that it that it should be inside a lot of large organizations. So So yeah, I, I can agree with your statement. I just want to caveat that, you know, I see outside of banking, right outside of banking and insurance, that a lot of times these audit processes aren't happening. And it seems to just be due to hype, right? People are just excited about this technology and kind of forgot about all that boring stuff that we have to do.
Paul Starrett:Yes. And I would actually tail off that that. A lot of times when you look at the audit frameworks, which we have, we actually have some podcasts on that very topic, whether they're ISO or NIST, or what have you. They the wording that they have already encompasses machine learning, because just because it's not a software, products or solutions you think of that way. It is in fact a software product. Yeah, that you have to audit no matter what so you don't have to say well, what are the standards for AI you still have to ask the same questions you would have about any IT resource or software piece of software. In fact, there's a um, I don't want to get too far off in this but there is somebody who has opined that the the gosh what was I gonna say here The process of auditing the the, you basically apply the same adjectives the same, is it reasonable? Is it cost effective? is it doing what it's doing? You might say that about SAP, but you're also saying that about a model that was put into production. Right? So you'd look at it through the same lens, which would then bring in the standards that we're seeing coming up through the EU and, and so forth.
Patrick Hall:And and again, you know, I don't want to take the conversation in a different direction here. But I mean, I challenged the audience to come what what is an implementation of machine learning that's not software. I mean, I'm not, I'm just not aware of one, it's it at its most fundamental level, it's software. And so yeah, just apply, just apply the governance processes that you do to all your other expensive high risk, important software, apply those to machine learning, and you'll be off to a running start.
Paul Starrett:Yes, and I'll just mention the name Andrew Clark is the guy who's been pushing this through, I think it's ISACA I forget. But in any event, that is, I thought is very, an interesting thing. The other aspect of this is that you worry about governance and privacy and so forth. And I think we touched on this, but the what, what I've heard called conceptual soundness in the auditing frameworks, which is what we just said, but it's part of the compliance process is is this Did you invest in software that is going to cause you risk? So just take those words and apply them into machine learning, now, there's a whole process that, that your firm could help address the technical and legal and how that interplays. And so I think that is another thing that the audience should be very clear on that this isn't something well, did you check to make sure that the data was private it? Was it private? Is it treated properly? Or some other such thing this has to do with the actual commercial purpose of the of the enterprise that in itself is compliance, related.
Patrick Hall:Oh, I, I totally agree. And I do I think a lot of people, aren't you, I think a lot of people are assuming that machine learning makes money. And yeah, and it likely does not, I'm not a total Debbie Downer here. But I think people were more clear about the the exact business use case and the exact business drivers of why they're investing in this technology, and what the business KPIs are, that they should be using to measure the performance of this technology, then I think people would see a lot more efficient return on investment and decreases in risk, right? When we're specific about what the system is doing, then we can be specific about governance and we can be, you know, we can we can more efficiently allocate our governance resources. So so I I fully agree that, you know, more businesses should should you know, instead of sort of having these grand, you know, we're going to adopt AI type type proclamations, right. Like, if I think back to all my experience in consumer finance, it's like, no, we're going to predict default in Northern California from mid 2019 to mid 2020. Using these inputs in the prime market only like that. Those are what successful deployments of machine learning look like highly specific with very specific business KPIs that can be measured. And just one more one more note, please. If if, you know for the listeners out there, if this sounds good to you, go Google SR 11-7, which is which is the the Federal Reserve and the Office of the Comptroller of Currencies, masterful guidance on predictive modeling risks that can really walk you through a lot of these steps. And in in a very nice way, it's a great paper, one of my favorite machine learning papers, written by a bunch of boring regulators. Very solid work, very, very solid work.
Paul Starrett:I'm very familiar with that, actually. Because we actually specialize in FinTech and banking AI. And I couldn't agree more. And I would also mention the Andrew Clark approach has been the CRISP-DM the cross, yeah, it's gonna skate me what that stands for cross representational something and data data modeling. You could also you wouldn't have to you could go to the SR. 11-7, but that kind of maps, the, CRISP-DM, if you Google that that's I believe, that's IBM, internal thing, I'm not entirely sure. But conceptually, it's very similar. And it's just common sense. You know, data science is math. And again, you can finish you can probably, you know, a guy like you is gonna know that better than I do. But I think that is I could not agree more to any to any approach.
Patrick Hall:On the math point very quickly. It's math with a lot of assumptions. And I think that, you know, a lot of these risk management frameworks get into this notion of just think about your assumptions, right? And just write down the assumptions of your system. And again, that gets into this notion of being specific, right? What is my specific application? How specifically do I measure success I'll leave it at that. I'll leave it at that and move on.
Paul Starrett:No, I think, again, the math, the math is really what it is data. And I get I wouldn't presume to continue this. But I think it's so important. The CRISP-DM I think does include, it includes that as part of what you used to consider. In my degree program. All my assignments had to follow that standard. In any event, no, I that's great. I think SR 11-7 is fantastic. I couldn't agree more. You know, I think you mentioned you sent to me an article, and I'll put the link in the podcast notes. But there was seven areas of legal liability that you that you address. And I don't know, we don't necessarily have to go over them one at a time. Although we could. But I think that's that really kind of helps show the sort of the how do I say this. Look at a diamond has facets, right? Yeah, the articles, the diamonds of the legal, it's kind of like you really have to see that, overall. Did you want to maybe could just peel back the layers there a bit?
Patrick Hall:Sure. And I think it goes back to one of you know, one of the comments you made earlier, if once once machine or AI starts getting used inside of business, it can start touching everything. And that's why that's why there's so many potential complications here. And, and so I'll go ahead and say, you know, I'm not an attorney, not an attorney just disclaimed. I'm not an attorney. And and also, you know, for for any attorneys listening in, right, there are some very basic legal issues that affect all technology that I'm not even going to address here. So things like anti trust, UDAB intellectual property import export, you know, of course, those things can come into play. But But we at BNH focus on a lot of our work is focused on algorithmic discrimination, because unfortunately, that that's one of the most common ways that companies and organizations can go wrong machine learning. So bias and fairness, which is I'm sure you're aware, and in consumer finance and employment, that that's highly regulated stuff. And you can really step in doo doo, with with machine learning. If if you're not careful when you're using machine learning and consumer financial o remployment, because not non discrimination regulations, they date back to the 1970s if not earlier, you know, if I'm not mistaken there. And and I think that's another important lesson for any data scientist tuning in. You can go download whatever new fairness Python package you want, but it may or may not align to pre existing regulatory standards. And if and if you find yourself in trouble, the regulators aren't gonna say, Oh, you use this cool new Python package, they're gonna say, Why didn't you do the test that the regulations stipulate. And so I think that that is another major part of our work and, and not to discredit you know, the marvelous work that, you know, researchers and open source developers are doing and algorithmic bias and algorithmic fairness. But there's, there's a lot of old laws because people have been doing, you know, what we might call data science for decades. And, and especially in banking, especially in banking, but but also in employment. And so these, these issues of algorithmic discrimination, and consumer finance and unemployment are probably where we spend most of our time. Of course, the second biggest place we spend time will be a surprise to you at all, that's data privacy. and machine learning can really complicate some already complicated data privacy risks and liabilities. But But I will say, I think just just the simplest problem is data scientists and their sort of direct managers not understanding that, you know, the need for some legal basis for data collection, right? That's the biggest thing, and that's what I tell my students, right? You know, I know you're not going to memorize all of GDPR, you're not going to know about, you know, the new California laws, the new you know, Virginia laws, new Colorado rules, just ask yourself before you start a data science project, you know, did someone consent for me to use this data? Or is this data you know, is there some other legal basis for me to be using this data there? And, and, of course, there's a million other things that can go wrong with data privacy, but I think that's the big one for data scientists just, you know, was this data scraped off the internet? You know, basically illegally these days or or, you know, was it collected with some legal basis and I think that a lot of our work is just dealing with machine learning systems that weren't unfortunately trained on on training data with no legal basis for its use, and and that, you know, that that causes a lot of problems. third biggest area is security. Machine learning has very specific attack surfaces. But what's what's more realistic is your training data is going to get hacked. And, and, you know, again, getting back to kind of how we opened up, right? A lot of the impactful work we want to do in machine learning involves collecting sensitive data about people that, you know, bad actors also want to get their hands on. And so you can't leave your thumb drive on the bus, you can't, you know, and I don't even use thumb drives or, or portable, hard disk anymore, or anything like that. But but there's some pretty, you know, when you when you collect all this high value data, bad actors also want to get their hands on it, and you have to be serious about security. You know, we can, I don't want to just read off the list, but but I will highlight, I think one risk that that will make a lot of sense to you. And a lot of sense to people listening is third party risk, right? When absolutely complex machine learning systems in large companies, they're never trained by just the people in the company, or just one person, you know, there's consultants, there's purchase data, there's data that's acquired by other means there's purchased expertise, there's software, you know, there's software that's made, there's software that's bought, there's open source software. And so tracking all those third party dependencies, oftentimes becomes very, very complex. And, and again, you know, it just takes one wrong license. It just takes, you know, one, you know, one Trojan in some open source package, you downloaded, something like that, to really go wrong. And so so, you know, I'll definitely call attention to third party risk as being another place where we, where our clients have a lot of questions and and rightly so rightly so.
Paul Starrett:Yes, and I would like to just harken back to something you said a little earlier. And then I would like to touch on some of what we do here to kind of help address what you're talking about this now is earlier, when you talked about the fairness and even some of the other legal liability issues. The determination of whether something is fair or not, given the risk, given the context of the data, and the requirements laws is a legal question, which should be made by lawyers. In fact, I'm a lawyer California, I know if you try to practice law, without a license, it's a crime.
Patrick Hall:Yeah. And that's why I said, I'm not a lawyer, I know,
Paul Starrett:<y license is inactive right now. But it's just that's a voluntary thing, I can go back anytime. But yeah, at least I have know enough to say that, you know, that's where BNH would be a really nice way of encapsulating that risk and make sure it's done properly. The other thing that I would mention is that, the way that you can really make sure that you're looking at all of the different areas as they come together, we partner with companies like One Trust, to get to help kind of manage and audit and guide the whole process into a direction. And so one of the things that we specialize on is the cybersecurity of the software development lifecycle, for example, which is every bit as much a part of machine learning as anything else. Yeah. yes, it'll move to serverless. And this whole new idea, and I'm just gonna sneak this in, because I see I hear it come up a lot, then move to agile development, which for those who don't know, I was part of the first part of the careers in software development, we have this thing called waterfall, where every quarter, you release something, right? And and you have this process now that has been chunked down into weekly, even daily sometimes releases. And that's called agile. And it's called continuous integration, \continuous deployment. And so the problem there, and I'm just going to get back to synthetic data is that getting data and feeding that that sort of high turnover process can be accommodated by synthetic data, which gives you the opportunity to generate data that is no longer has privacy risk in it, and therefore it can be kind of so any of these technologies all kind of feed into kind of help get get the whole process rolling. Great, I guess, and I didn't, I didn't expect that we would have to get through all seven of those. Got a 40 minute podcast here. The other thing I thought was interesting, you mentioned prior in our discussion about this, an example from Twitter that Yeah, you brought up if you wouldn't mind going into that as sort of a way of putting this into, you know, real world terms.
Patrick Hall:I'd love to I'd love to I think, you know, I think to at least two to two or three things you said are worth a real quick comment on that. Yeah, the fairness should be legal determination. That that's exactly right. And so if if there's any data scientists tuning in, or lawyers who are working with data scientists, generally speaking data scientists don't know that they're not educated that way, now you You said you teach a class where people are exposed to this. I do the same, but I think I think we're pretty rare. You know, I think a lot of data scientists don't know that that they have to work with lawyers in certain cases. And it's not easy. They're very different professions, very different philosophies, very different ways of communicating. But But more and more, I think we're going to have to see data scientists and attorneys working together, because again, systems that make impactful decisions about millions, millions of people have have a lot of risk and legal liability associated with them. And, you know, we can we can go ahead and move on to the Twitter thing, but but I just really wanted to highlight that because that that has just been so you know, like, when when attorneys bring us in, you know, when we get hired as outside counsel, they're just not aware that they're like, Why are the data scientists talking to us? Really, because they have no idea that they're supposed to be talking to you. They never they were never taught that in school. They've never you know, their managers don't tell them that. It's like a huge hole. It's a it's a risk hole that you could drive an 18 Wheeler truck.
Paul Starrett:Yes, it before me to Twitter, if you don't mind. A little idea. That's the whole point of the podcast is kind of freeform it. The class is a master's program in data science. And it's University of the Pacific, and a program that I built for them. And we've just recently overhauled it to include transparency and explainability. But I will tell you, the one thing that makes the data science students students sit up the most is negligence. When I tell them, you have a duty
Patrick Hall:Product liability.
Paul Starrett:Exactly. But even to them for malpractice, is if you always have a duty to behave reasonably, if you don't, you could be in the hopper, or in the hot seat to hear that you're always yes, even as you sit. So they always they sit up and they pay attention. And I think that's the one thing you know, and the other thing I would just leave you with, is that I think the the legal analysis that we were alluding to, is really a risk based analysis. Yeah. So the risk is that you have to look at the data, you have to get the model, you have to kind of go back and forth, flip, flop it down to its sweet spot. And that's where again, some you know that the partnership that you have with with Andrew Burt is really good. And of course, I would say that about what we do. But this is about you. So anyway, I'm sorry, let's Twitter example.
Patrick Hall:That's perfecty fine. I will I will very quickly because George Washington University has been very supportive of me over the past few years, and especially my department chair has really pushed for me to be able to teach these data ethics classes where where we do expose students to the same kind of ideas. And so just just a shout out to to them as well. So So thank you, George Washington University, of course, but yeah, yeah. So on this Twitter thing, I think you know, harkening back to when we were talking about SR 11-7, brilliant, masterful, you know, modeling risk guidance, but, you know, you know, we can critique everything, and I'd say, a major assumption SR 11-7 itself, I would argue is that it's mostly targeted at setting up this scenario where we're sort of like, highly paid experts train and test and oversee these models, right. And, and in consumer finance and big banks, that that is what happens, right? You know, it's it's experts, building models, experts, testing models, experts with decades of experience overseeing, you know, the models, testing, validating the models, auditing the models, and SR 11.-7 is sort of targeted towards that mindset. But I think as we all know, you know, that that's not how machine learning is happening in the broader environment like that the practice of machine learning, and consumer finance could not be more different from from the practice of machine learning, you know, just some company out there, brand. And sSR 11-7, I think, where we're it, it may fall short. And this is not a criticism of the authors or criticism of the guidance, but I think where it may fall short, though, is sort of this new set of technology. So things like robotic process automation, or chatbots, or self driving cars, where the system itself is allowed to operate in this very broad context, right? Because we said, you know, again, another maybe assumption SR 11-7 is that your model is targeted and being measured correctly, right, but but if you just have some chatbot, they interact with anybody on the internet. You know, it's hard to monitor it. It's like almost any kind of failure could happen. And if you don't believe me, email me, and I'll bury you in links. But I know another part of my academic research and work that we do at bnh is, is studying failures of AI systems and AI systems fail and all these very complex and unpredictable ways. And so to help govern those sort of broad context, you know, you know, I'm not gonna say true, but but you know, quote unquote, AI systems, you know, chatbots image recognition, self driving Cars robotic process automation, this newer generation of technology that's starting to filter through the economy that really operates in a much more broad context. I think you have to bring in, in addition to sort of the ideas from traditional model risk management, I think you have to bring in ideas from cybersecurity to govern, the system's want one because they can they can be attacked, I mean, you know, that that's one very obvious sort of on the nose issue. But then too, I think cybersecurity is better placed or better position to handle sort of, you know, failures that might be harder to predict. Like, we just know that someday something bad might happen, and we need to be ready for that. And and I think that the cybersecurity mindset really lends itself to that more clearly and more directly than than say, like traditional model risk management or CRISP-DM or something like that. And that is not a criticism of those of those approaches. It's it's just the reality of this brave new world that we're making for ourselves.
Paul Starrett:And really, I'm sorry, when
Patrick Hall:not well, I can get into what we're what we did with Twitter, which I think was was a really great idea but but I wanted to give you a chance to ask any questions or clarify anything and that sort of meetup?
Paul Starrett:Well, I think again, I would just sort of take that thought and maybe finish it off with and I think was implied of what you said but GDPR is a general data protection regulation. not, privacy. Really the biggest liability that a company has is when they have when they're hacked and they have you know, breach and now they have you know, private right of action that usually comes out with a class action lawsuit. That is where the real headaches are, in the the board as we were alluding to earlier, really sit up and pay attention so I couldn't agree more that cyber security and privacy are sides the same point in fact my last podcast was on that very point but in any way but that said a great as a great so glad we covered that and got both our thoughts on it. So yeah, the Twitter the
Patrick Hall:Yeah, the bias bug bounty so so and Rahman Chaudury at Twitter and and I believe she's also involved with a with a startup called parody that does not audit so really, she deserves a lot of the credit here and in setting this up. But you know, to kind of key off something you just said, not only are security and privacy you know, the two side of this, you know, two sides of the same point it's it's it's again, like a multi faceted diamond, like fairness and transparency are all they're all interrelated. And I would argue I'll borrow a term from human human rights law, apparently, I very new to this, in indivisibility. Um, who wants to totally secure but biased AI system? Who, who wants a fair but inaccurate AI system, you know, like, we want all these things we want it to be generally speaking, we want transparency, we want fairness, we want privacy, we want security, like, we just need the thing to work the right way, right? Just like an airplane, you know, we need to be able to assume that it does all the right stuff. And so so we can take cybersecurity notions from cybersecurity, like bug bounties, were essentially a well resourced company, you know, throw some dimes and nickels at the general public to to track down bugs in their software. And so you know, I shouldn't have said dimes nickels, but but to the corporation, it's dimes and nickels, but but it's not uncommon for these bug bounties to be 1000s, 10,000s of dollars, that would be very attracted to an individual. Um, but what the point I'm trying to make is it's cheap for the companies, it's, you're kind of crazy not to be doing this. So. So a bias bug bounty right is this idea where we provide rewards for people to find bugs in our software. And in particular, you know that the traditional mindset here would be security vulnerabilities. But Twitter had this brilliant idea and Rahman had this brilliant idea, applying the bug bounty to AI bias problems. And so they turned over this this kind of high profile, it already been written about image cropping algorithm that had been used on Twitter. That was, you know, Twitter, consumers were noticing weird behavior in this image Cropper. You know, it, it turns out that and we found out through the blog bias and through other people's research, it truly favored younger, wealthier, wider, more female images. And, and it really cut out veterans, people in wheelchairs, people in religious headdresses, non Latin scripts, You know, it had a very strong sort of pro Western pro northern bias to it. And even me, you know, I think the really important thing here is right, all I do all day long is think about failures of AI systems, I never would have thought that this image Cropper would have cropped out non Latin scripts, I may have picked up on some of the more obvious bias issues, but I would never would have caught like the non Latin scripts, I never would have caught the religious head dresses, you know, the, these are things that global companies need to know about. And very oftentimes, we know that engineers are sitting in California, or London or New York or Boston. And they're not thinking about people on religious headdresses. They're not thinking about non Latin scripts, right? And so you can't even find that bug. But your global customers are experiencing it. And, and so, again, this just seems so common sense to me. Because it's relatively cheap for the large organization, to to enable the public to find bugs in these broad context AI systems. Because no, you know, no highly educated developer sitting in San Francisco or DC like I am, is going to think about this stuff, even if they're trained to like I am, they're not going to think about it. And so, so I think that really, to me, proves out this notion of cyber, you know, model risk management, great, you know, CRISP-DM, great, um, but for these, you know, AI systems that are sort of operating on the broad internet without much oversight, very, you know, open context and in the way they're operating, you need a lot of different eyes on them, you just need a lot of different perspectives to catch bugs. You know, that may not be traditional software bugs, but they're, they're bias bugs, they're they're unfair to certain parts of your users and user population. And you won't even know that. That's fascinating. Fascinating, it was fascinating.
Paul Starrett:Well, I know you're getting going here. But we don't have time to get well, I think, let's put it this way. I think that, you know, when, when people are training, compliance models inside a company, they're tagging documents and records as this or that, and that becomes the way in which you can train a model and refine it and have a generalize more broadly. For the listeners, there's in machine learning is thing called overfitting, which means that a model is trained on specific data that can't capture unknown, or it's too constrained somehow. But this what you're doing with the crowdsourcing, which is the way I would put it is with all these people, they are helping you build a new training models. Yeah, I think if if, as a legal slash data science person of sorts, I might say, listen, isn't that reasonable? So even though you may have had this, this going off the rail with clipping out people with headdresses or Latin that that's okay, is that if you're going to have AI, this is the world we're in. And so you kind of say, Well, look, there's a certain amount of slack, you have to cut people. And then you grow as long as you're doing it, as long as you have a crowdsourcing. I think it's a fabulous example. And a fascinating idea. I'm, I've that's why I love these podcasts.
Patrick Hall:Well, I'll just throw out so other ideas to borrow from cybersecurity, if you're out there worried about your AI, I think another very obvious one is incident response and incident response plans. Right. And if you live in the cybersecurity world, it's highly developed in the cybersecurity world. Yeah, and up to the point where you have your choice of what kind of insurance policy you want to cover your cyber responders and that kind of stuff. But But I think, you know, AI systems have these possibilities to fail and, and, you know, depending on the type of failure, they can be very damaging to consumers, their reputations, they can have legal liabilities. And so a very simple idea is just get the failure under control quickly. Right, but before it's been out of control before it does more harm. And so I guarantee you, if you're sitting at a big company, you have all kinds of advanced incident response plans for all your important mission critical IT systems, except your AI systems, and it's just this it's just this kind of silly, hype driven oversight. Um, and, and, you know, if we take a step back, pull our heads out of the hype sphere and just think about if the system fails, who has the money to spend to fix it? Who has the technical expertise to turn it off or pause it? What happens when we pause it do downstream systems collapse you know, who's going to call the PR agency who's going to call the attorneys you know, the just these kinds of basic questions that most companies have a document that answers them all for all their other IT heart You know, all their other expensive IT systems. So I think incident response plans are just common sense thing to bring them from cyber. And then the last one is red teaming. And I don't, I don't think there's a whole lot of difference between red teaming and sort of this notion of model validation or model testing or even model auditing. I think the difference between red teaming and those things I just named, though, is the adversarial mindset. And I think that's something that data scientists are missing. You know, data scientists live in this world, like we're making cool new stuff where the good guys nothing bad can go wrong. That's just not right. And will abuse your technology, people will seek to abuse and gain your technology, people will find ways to attack your technology. And I think that it's that adversarial mindset that comes with red teaming, that makes red teaming so valuable. And so Facebook had an article on Wired a couple years back, I think now that you know, they were touting their ability to red team, their AI. And I, you know, I think that's a great idea, too.
Paul Starrett:Yeah, and we could again, there's a thing called adversarial machine learning, which I'm sure you're you're completely familiar with. For our listeners, if you want to learn more, there's a there's a site called cleverhans.io, I which is the name of the racehorse, or some dumb thing.io, where you get
Patrick Hall:a horse that people thought could talk. Okay, it's a horse that I can't remember if, if, if it was, if it was purposeful or not, but but people were deceived into thinking this horse could talk. And that's Clever Hans. And that's clever.
Paul Starrett:Got it? Got it? Yes, I think just again, for our for our listeners, the ideas that people are using machine learning to defeat machine learning protections that people have in place. And so I think, again, that's a rabbit hole, Patrick,
Patrick Hall:I'm sure we'd love to talk about that. We can Well, maybe,
Paul Starrett:Maybe we'll maybe we can have another one. But I think, yeah, that's actually probably good idea. There's probably whatever, I have that open idea with all my guests. But I think I think that kind of helps us end this here. We could go on for Gosh, I'm sure a long time, as I always do with people who I synergize and to have a passion or a similar passion with I can it kind of brings us full circle because really our The goal here was to discuss the the sort of holistic nature of this, it's an ecosystem, pull on one thing to tug on everything else, that you really want to have people who understand the risk from a lawyer perspective. And then from a data scientist perspective, we PrivactLabs, of course, have a philosophy and feel we can deliver. I think what you do, Patrick, and what, Andrew, you're both very well placed in your respective positions. And I think that's really the the new model, frankly, for for what happens. So thank you. What I do like to do with all my guests is give you a chance. Is there anything we didn't talk about that you would like to leave as a parting thought? Where's this all going? Or things you think we haven't discussed that you'd like our audience to? To know?
Patrick Hall:Yeah, I have a little tagline recently, we never got to talk about synthetic data. And so I'll just get in not not into synthetic data, even though I think it's a great resource. And companies should be looking into it, and at least investing in some some kind of proof of concept around it. But why, you know, why would we ever use synthetic data? And I think the reason is, and I wish I could go back and scream this at my young data scientist self is we're too caught up with with our training data. We're too caught up with this all this data we collect. We live in this fantasy world where we tell ourselves, oh, this data is accurate and objective, right? I think we've all been told we can make accurate objective decisions, data data is accurate and objective. But it's not guaranteed to be right. It's just simply not guaranteed to be either accurate or objective. And so I think that, you know, if you're an attorney, you should think about that, if you're a data scientist, you should, you should think about that a lot of the risks we've been discussing, go back to this assumption that the data that feeds into these systems is going to make the system do the right thing. And that's just not necessarily true for all kinds of reasons, right? The data could be attacked to data, if we poison the data could be wrong, the data could encode hundreds of years of historical sociological biases, like most of it does that. So I think, you know, just just, again, kind of applying common sense stepping back from the hype a little bit. data driven decisions are great, they're probably some of the best kinds of decisions we can make. But that doesn't mean we have to assume all the data we can get our hands on is accurate and objective, because that's just not true. That's just not true. And I think that underpins a lot of the risks we've been discussing.
Paul Starrett:You know, you'd have to say that, because we have several podcasts on this very topic. And just before we leave, I would like to just play a little bit of ground foundation there is if synthetic data is data that is inspired from the underlying training data. But by way of doing that, it's using probability distributions and correlations to mimic underlying data. And then there things called agent based modeling, which is a way of kind of simulating what could or should happen. And you lay that on top of the, the data I mentioned. And you can get this very nice sort of training data that fleshes out transparency, it fleshes out corner cases, and so forth. So I completely agree with you. It also helps hurry along the Agile programming process that I, that we mentioned, again, we do have podcasts on that. So that's a perfect way to end this. Patrick reallt. It's, it's a passion o mine, because for the reasons w stated, but I would encourag people to go that to go there We do have training videos o explainability machine learnin on our site privacylabs.ai under the media menu. So I think with that said, Sir, I, I guess we've we've pretty much done the damage we wanted to. And thank you again, so much, I would relish the opportunity to maybe to have another podcast on one of these different tentacles we've discussed. But I think with that said, what would be a good way for people to reach you if if if you want to provide that to them?
Patrick Hall:LinkedIn is probably the way you're likely to catch me the easiest. I'm also on on Twitter, of course. But But LinkedIn is where I might actually be able to get distracted and have a little chat with you so so I'll send people to LinkedIn, but all right, and you can find me out there on the internet.
Paul Starrett:Got it. But your your firm is BNH.ai.
Patrick Hall:person, a horrible salesperson,
Paul Starrett:Well, maybe that's part of the schtick, isn t it? So I will. Great, thank yo. And again, privacylabs.ai, and bnh.ai and please check out o r website for new podcasts like his one. And thank you all for l stening. And thank you again Patrick. It was a real pleas re
Patrick Hall:Glad to be here. T anks a