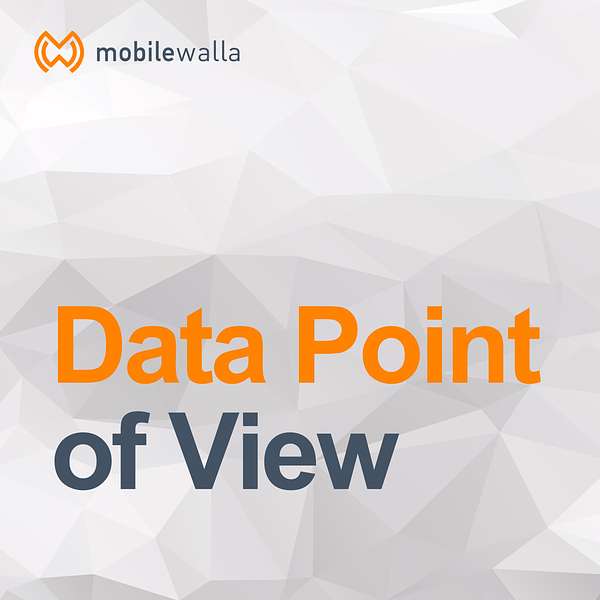
Data Point of View
Data Point of View
Understanding Data Drift and Stability to Build More Resilient Models
Predictive models help predict future behaviors, and they are powerful tools for supporting business decisions and improving operations.
But predictive models are only as accurate and reliable as the data that powers them.
In this episode of the Data Point of View podcast, our host Laurie Hood welcomes Anindya Datta, the CEO and Founder of Mobilewalla. They get into the problems of performance degradation in production, how to tackle data accuracy challenges, and the role of data stability in building resilient models.
D0E9EC4B_7 - Mobilewalla - Data Point of View - Anindya Datta
[00:00:00] Anindya Datta: In order to build resilient models though, we need to find data or we need to be able to identify the drift properties of data, so that we can preferentially build a model with data that drifts less.
[00:00:11] So we call it the stability, right? So stable data, data that drifts less and while drift is a point measure, stability is a longitudinal metric, right?
[00:00:20] Laurie Hood: Thank you for listening today. I'm Laurie Hood, CMO at Mobilewalla, and this is Data Point of View. Data Point of View is a podcast for anyone interested in using machine learning and consumer data to achieve business objectives. Joining me for another episode is Mobilewalla's CEO and Founder, Anindya Datta. Anindya recently published an article in "Towards Data Science" that discusses how you can use the drift and stability of data to build more resilient models, which we're going to dig into this podcast.
[00:01:17] So welcome, Anindya.
[00:01:19] Anindya Datta: Great to be here, Laurie.
[00:01:21] Laurie Hood: So, and with that, we're going to go ahead and jump right in. So when you're building predictive models, model accuracy has traditionally been the primary driver of model design and operalization. Well, this leads to high fidelity model construction at training and testing time, performance in production
[00:01:42] of in degrades, producing results far worse than expected. So Anindya, let's start by talking about some of the causes of performance degradation in production.
[00:01:54] Anindya Datta: Great question, Laurie. Well, there are many reasons that why models might perform poorly in production, but one of the main reasons, perhaps the dominant reason why models in production behave differently than, you know, when they're trained and tested, is changes in the properties that the data that anchors this models, right?
[00:02:15] The original data used to create the features on which the model was trained differs from those that power the modeling production. Right? Remember that usually this happens when some time has elapsed between when the model was deployed. And so in that time, the nature and properties of the data that are powering the features then are anchoring the model of change.
[00:02:37] So this phenomenon is called data drift, right? So data drift sort of which happenes when real-world environments contributing data change unexpectedly in very unplanned ways, is possibly the dominant reason that causes a problem sort of resiliency models. And interestingly, this phenomenon, which we understand much more than we did before,
[00:03:02] because a lot more models are in production now than let's say a few years ago when people would test models, develop models, but they were simply not that many models operationalized. So as machine learning sort of matures within organizations, more and more models get deployed to power sort of regular organizational processes. And an interesting phenomenon emerges
[00:03:26] because of what we just described, right, that models misbehaving production. What happens is that now resiliency often overrides raw predictive criteria. The resiliency often overrides raw predictive accuracy as the defining criteria for operationalizing models. Right? So increasingly, what the ops process owners care the most about is consistency, right? That payroll and supply chain and organizational processes work predictably and consistently, therefore the models that power these processes also must be predictable and consistent. Right? So increasingly ML practitioners are leaning towards operationalizing decently performing predictable production models
[00:04:14] rather than those that exhibit high-performance at desk time, but often misbehave in production. Right? And this preference for resilient models is now widely acknowledged sort of in the industry.
[00:04:25] Laurie Hood: So I want to go back a little bit to what you were talking about with data drift. So if the impact of drift on resiliency is pretty much acknowledged, but people want to build a resilient models, how do existing solutions then help them solve this challenge?
[00:04:44] Anindya Datta: Yeah. So yeah it is. Data drift is very well-recognized and it is recognized also widely that drift is probably likely the main cause of lack of resiliency. Right? So to compare this fortunately all existing machine learning software stacks built in mechanisms to identify drift, right?
[00:05:07] So effectively all software stacks have a utility into which you can compare the specific data distribution that is powering the model now, do the distribution of data on which the model was trained and did a utility then returns of a measure of how much the data has drifted. Right? So these are super useful tools, almost all of, sort of, it's a very key part of model monitoring and modern fixing.
[00:05:39] But the biggest drawback is that they're reactive, right? I mean, you apply them once you see that the productionized model is not behaving as you expected. So when a deployed model misbehaves, these tools are invoked to check drift, sort of revealing how the data fed into the underperforming model is different from the data that was used to train it.
[00:06:00] And it drifts the model is of course fixed by usually by retraining and redeployment.
[00:06:06] Laurie Hood: So it sounds like today correcting these issues is much more of a reactive approach, not a proactive approach. So wouldn't the modeler want to be able to better understand their data kind of prior to building features or using it in the model?
[00:06:25] Anindya Datta: Yeah. Great, great, great sort of thread. Yes, absolutely. We understand that drift impact resiliency and therefore we have pretty cool ways of measuring drift. But the real question, but these don't help the construction of resilient models from first principles.
[00:06:40] Right? So the real question is, "How do we build machine learning models that are resilient from scratch?" Right? So achieving resiliency, of course, as we talked about already, meaning models that have predictable behavior and underperformed seldom, right? I mean, they don't misbehave very often.
[00:06:58] So without resiliency to the operationalizing machine learning models would remain a major challenge, modelers will continue to build hundreds of models, and which will underperform in production, requiring frequent correction and the continual need to re-engineer these models will raise to the organizational doubt and questions over the operational utility of machine learning and predictive modeling.
[00:07:24] Laurie Hood: Which is a big issue for different organizations as they try to build out their data science teams and incorporate more machine learning and predictive modeling. So what should data scientists be starting to do in order to kind of take steps towards moving towards? I think you had talked a little bit about the concept of data stability.
[00:07:49] Anindya Datta: Yeah. You know, so it's sort of self-evident that in order to build models that are resilient, you got to anchor them with data that doesn't drift very often, right? I mean, if data drifts, models would misbehave. If data does not drift, the issue of course is understanding the future drift behavior of data.
[00:08:09] Right? So remember that data drift represents how a target data set is different from a source data set, right? For time sets data, right, for time varying data, which is of course the most common form of data powering machine learning models. Sort of drift is a measure of the distance of two instances of data.
[00:08:27] So drift sort of tells you, "Hey, at this point, this distribution is different than distribution, this distribution by this much." Right? So, in order to build resilient models though, we need to find data or we need to be able to identify the drift properties of data, so that we can preferentially build a model with data that drifts less.
[00:08:49] So we call it the stability, right? So stable data, data that drifts less and while drift is a point measure, stability is a longitudinal metric, right? So stability is the property specific data attribute doesn't drift a lot over time. So we believe resilient models should be powered by data that exhibits low drift over time. Such a models by definition would exhibit less drift and be it sort of misbehavior. To manifest this property drift over time, we have been using the notion of data stability, right?
[00:09:23] So stable data drifts little over time, whereas unstable data is the opposite. Data stability, if one can factor it in properly in the model building workflow and the model monitoring workflow, can serve as a powerful tool to build and maintain resilient models.
[00:09:41] Laurie Hood: So talk a little bit then about modeling at Mobilewalla and how we're incorporating this concept, this thinking around data stability and understanding that within the data that we're using.
[00:09:57] Anindya Datta: So at Mobilewalla, so there's two aspects of using stability. The first, the notion is easy to understand, the concept is easy to understand. "Hey, here is data that drifts less over time and here's data that does not."
[00:10:09] But measuring stability quite challenging, right? Because the distribution property of data is a very complex sort of artifact, right? So we had to properly quantify how are the property of data can be represented mathematically. And from that quantification, we had to develop the mathematics of the measurement off likelihood of drift.
[00:10:37] So, we've done that. And as a result of which, what the first part of the answer to your question is that for all the data attributes Mobilewalla ingest massive amounts of data, you know, hundreds of attributes. For each attribute that we ingest, we computed stability on a routine basis.
[00:10:55] Right? In fact, stability is sort of super simple, right? We have a categorical scale, right? Zero to four, zero means highly unstable, four means highly stable. So we are computing stability of data continuously, and then this values are made available to the modeler. Right? So when the modeler is building models, the modular knows how stable the data items are that he or she has at her disposal.
[00:11:22] So when they're building features, they try to build features that are good, that are predictive enough, but are composed of data items that are more stable rather than less stable. So, in the ideal case, a model is built with features only built with stable attributes, therefore the feature itself is stable.
[00:11:41] Therefore the feature properties don't drift much, and the model is resilient. In reality though, it is sometimes hard to build features only with stable attributes, right? Because certain features could be so highly predictive that you might want to use it anyway. But knowing, what you know, but what still, what this methodology allows you to do is to know, or is to predict the stability of features, which means that if you know that I've built this model with this four features, one of which is unstable,
[00:12:16] you know that you expect that model to, let's say, misbehave more than another model that is built with features by only stable attributes. Therefore you can devote, so scarce monitoring resources are very expensive monitoring resources, which typically have compute resources towards models that you would, that you expect to drift rather than towards model that you
[00:12:40] expect resiliency from, right? So not only does this help you build more resilient models, it also helps you allocate your monitoring resources much more optimally across your model portfolio.
[00:12:55] Laurie Hood: So now you're proactively monitoring the models where you know there's the potential for them to misbehave versus just wondering what's going to happen, seeing it, and then having to go back and retest and retrain?
[00:13:12] Anindya Datta: That's correct. That's correct.
[00:13:14] Laurie Hood: So, I mean, I know at Mobilewalla we have a large very sophisticated data science team, with what, like a hundred models in production.
[00:13:24] So we're
[00:13:25] running a big shop. And in working to address this challenge, I know that we have built some of our own technology that we've released as an open source project Anovos. So can you talk a little bit about what we're doing with the Anovos library and it's certainly available to anyone interested in using open source?
[00:13:47] So talk a little bit about that and some of the benefits that a data scientist or a data engineer can get from using that.
[00:13:55] Anindya Datta: Anovos, Laurie, is bigger than, or it's broader than just stability. Right? So, going back to our discussion that we had just now, we kept referring to the notion of features again and again, right? Stable data builds, stable features, unstable data. So one of the most important components of model building is making sure that you power the model with the right features or with the right predictive features. And this component of the machine learning workflow, where you build features from data, known as feature engineering.
[00:14:31] So Anovos is really a software library, a library of utilities that lets you do much more systematic feature engineering than what is possible sort of currently. Right? I mean, as you said, this is driven by our own experience at building and deploying large sets of models.
[00:14:51] We have found that in a feature engineering is a very, and it's well-recognized in the industry as well that feature engineering is a very ad hoc sort of messy, a lot of art, very little signs, sort of modular driven process. Right? I mean...
[00:15:05] Laurie Hood: Yeah. And it requires a high level of skill by the data scientist or the model, a deep understanding of their data. I mean, it's a very complex process and a very important one.
[00:15:18] Anindya Datta: Yeah, it's a complex process. It's not a linear. And it's one thing, if it's a complex process and there is a procedure you can follow, and if you follow that you end up in a good place, right? But this is not only is this a complex process. Not only is, does this require a lot of domain knowledge and a lot of sort of experience and skill,
[00:15:37] it's also extremely time consuming. I mean, feature engineering takes about 70% of the overall time for model building. And it is a huge impact of eventual model performance. I mean, even with all the skill, all the experience, you cannot ensure that your feature selection is sort of the best or even good, right?
[00:16:00] And optimal feature selections happens, there's longer a testing and you come back. So Anovos creates a framework where a modeler, or a group of our team of modelers, can go to a structured workflow and create features that are good and stable from the start. I mean, that's what Anovos is.
[00:16:22] Laurie Hood: Awesome. Well, great. Well, I encourage our listeners who are interested in using open source and improving the feature engineering process, they can find Anovos on GitHub and we'd love to have people use it and share their feedback. And so with that, Anindya, thank you for joining us today and for your insights,
[00:16:44] and just talking about ways that data scientists can approve their outcomes and to our listeners. I want to thank you for your time today, and please join us again for another episode of Data Point of View, brought to you by Mobilewalla.
[00:17:01] Anindya Datta: Thank you, Laurie. It's always fun to do this podcast with you.
[00:17:05] Laurie Hood: Well, thank you.