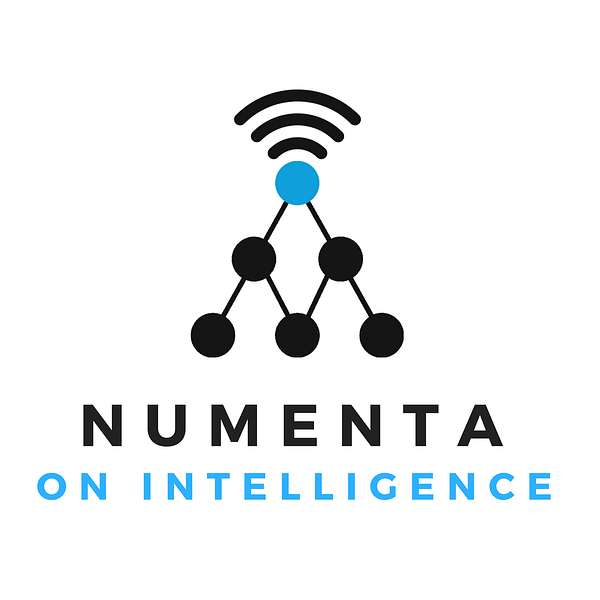
Numenta On Intelligence
Numenta On Intelligence
Episode 2: Research Update with Jeff Hawkins - Part 2
Welcome to Numenta On intelligence, a monthly podcast about how intelligence works in the brain and how to implement it in non biological systems. I'm Matt Taylor. Today, I'll be talking with Jeff Hawkins about the latest research Numenta has been doing with regards to grid cells and hierarchical temporal memory or HTM. While this is the first episode of the Numenta on Intelligence Podcast, Jeff and I will be going very deeply into the theory very quickly. Therefore, I prepared in the show notes a bunch of educational resources so you can learn at your own pace about HTM and grid cells. If you like this conversation and you don't know how HTM sequence memory works, I suggest you watch through the HTM School videos on YouTube. Just search for HTM School or see the show notes for links. This is part two of a two part interview with Numenta founder Jeff Hawkins. This recording continues where part one left off after an in depth discussion of how HTM sequence memory builds object representations in space through movement. A couple of things about grid cells.
Jeff:Yeah
Matt:There's head direction cells, there's border cells, there's stripe cells, there's speed cells. Do we have to pay attention to all these different types of cells and what, what did we learn from all these different things?
Jeff:Well, we're going to have to pay attention to some types, but maybe not all of them. Uh, now again, the basic theory we're working on here is that you have this old part of the brain that evolved for many, many eons to allow animals to navigate and map out environments and know where they are.
Matt:Hippocampus, entorhinal cortex...
Jeff:Yeah, hippocampus, entorhinal cortex are older structures. They've been under a lot of evolutionary pressure. They are designed to solve specific problems of navigation of an animal in an environment. What we think happened is that evolution co-opted those mechanisms and stuck them in the neocortex in a more regular, orthogonal way.
Matt:Which happens all the time in biology.
Jeff:It does, yes it does, but it doesn't mean all the mechanisms can't transfer it over and some of them are going to be very specific to the problem of the rat navigating in a maze or in some dark channel under your house or something like that. Um, and they may not work in the cortex. So I think what's happened in the, in this case, you have some very highly tuned specific mechanisms in the entorhinal cortex and the hippocampus, solving very specific problems, evolved over many, many eons so that they're fairly well tuned and then the evolution to say hey, I can take the best of those and rejigger them a bit so I have something very generic. So now I can apply it to recognize lots of stuff, how to use cell phones and how to use computers and tools and language and all these other things which wasn't originally evolved for. So how many of those things transfer over, we don't know. The idea of like for example, orientation, is clearly something with head direction cells. Head direction cells say, hey, which way am I facing in this room? You know? And as I, if I just sat in one spot and rotated in my chair, my head direction cells would change.
Matt:Right. You certainly need to know that when you're sensing objects.
Jeff:You need to know that. You can say where am I, but what you're going to sense is depending on not only where you are, but which way you're facing and where are you going to be when you move depends not only where you are, but where you are and which way you're facing. Some of those concepts are going to apply in the cortex because thinking about my finger, what do I sense on my finger? Well, not, it depends not only where my finger is touching an object, but it's orientation. Like I can rotate my finger around that.
Matt:Oh yeah, you can turn it on, on an axis.
Jeff:I'm touching the top of my Coffee Cup right now and I'm rotating, pivoting on the same location, but I'm sensing different things. So also where my finger is going to move if I flex my finger depends on where its current orientation is. So some of those concepts are going to come across.
Matt:So the idea of orientation could be represented in every cortical column based on some aspect of sensory input.
Jeff:Yeah. Yeah. And it will be, um, this is an area we don't understand as well as we'd like to understand. It's quite complex. Um, so at the moment the papers we're writing, we're not addressing specifically the mechanisms of orientation because the concepts you and I talked about earlier about composition of objects and so on really don't require we solve that problem. The concepts themselves withhold regardless of exactly how the orientation problem is solved. And they're really big concepts if you, if you are interested in intelligence and how brains work, um, that those concepts are, they stand on their own. And then the orientation component, it's more of a detail.
Matt:You certainly can't figure out everything at once.
Jeff:No, right. We need to do, as I always like to quote Francis Crick who wrote an essay nearly 40 years ago. We need a framework and we don't need detailed theories, right? We need a framework. We were not thinking even a basic framework for understanding how we perceive the world. And so the locations, um, uh, really give you... grid cells really provide that framework and then we can start filling in more of the details later about exactly, well for example, there was a, you mentioned these border cells and you know, when an animal, a rat is near an edge of a room, these border cells detect that. Are their border cells in the cortex? I don't know, maybe not. You know, is there an equivalent to border cells when I'm doing language? Probably not.
Matt:But I mean rats or specific organisms could develop specific cells that detect specific things that only exist in their environment.
Jeff:Exactly right. You know, a bat flying around, you know, he doesn't follow walls, right? Or she.
Matt:It might follow sound.
Jeff:Obviously, but you know, rats literally like to run along walls. They don't like to be out in space. They don't want to be away from the wall. So they have these cells attuned to knowing I'm near a wall. I want to be near walls all the time.
Matt:Less chance of death there. I know that's their modus operandi. That's how they get around. They follow walls and home owners know this. That's the problem. That's why we don't have, that's why you have rats in the walls and not walking around the floor of the kitchen. So, you know, again, that could be something very specific to rats and probably doesn't pertain to other animals in the entorhinal cortex and probably doesn't carry over to the cortex. But the basic idea of orientation is something that, or head direction cells in the, in the entohrinal cortex, they call them head direction cells. We just said that's an orientation. So that's the term we've developed, genericizing that term. That makes sense, um, about object representation. I have another question, but that is a misunderstanding that I had initially. It made a lot of sense to me to think about how we represent objects in the brain, to think of it as a map. But I know Marcus initially thought about this and we changed our thinking about it, but I want to walk through this because it's sort of an education for me and I want you to explain it for other people. So it made sense to think of, okay, I've got an object in my brain. It's essentially a map of locations in space using some type of grid codes to sensations that I felt at that location, that makes sense. And you can do some operations on that structure, but that's not actually the way it seems to be working, right? It's more about movement or displacements or changes, but going from point a to point b and what is sensed during that.
Jeff:Well first of all, let's talk about the word maps. We're not using it heavily right now and because there's multiple ways you can interpret the word map. And so at one point we were talking about the entire space of all points is one big map is like the universe. And um, and, but maybe the map is just a map of the phone or the map of a coffee cup.
Matt:I'm thinking of it that way, just the map of an object.
Jeff:So, uh, that's a fine term. We are not using that term right now in our language, in our description of this. Um, it's just a personal decision that we made. Now the second question you asked about is, we described it in the Columns paper, the October 2017 paper, we described how you learn an object by sensing at all different locations, and there's some truth to that, but it's more complicated than that. As we were talking about earlier, we really want to represent objects is composition of other objects, right? So when I learn a Coffee Cup, I don't really want to have to touch it everywhere to recognize every single feature I'm going to sense. Um, I can start feeling something that feels like a handle. And I say, oh, that's a handle. I don't need to touch every single spot on the handle to verify it's a handle.
Matt:You don't have to fill in.
Jeff:Well, I, I kind of say it's an object I felt before. When you're born, yes, you probably know nothing. So you have to learn everything, but you start building up this sort of language of components and objects. And so when I learn a new object, I don't really, you know what, I don't just move my finger over everything and sense every single point on the object.
Matt:No, you sense it enough to associate it with things you already know.
Jeff:That's right. So the idea that you are moving and sensing and moving and sensing is of course correct. But what you're really doing is you're moving in and inferring substructure that-- So I see, oh this is the, this is a circular rim and this is a handle and this is a cylinder. I don't. And so it's not like I have to disassociate the raw sensory input with the location. I'm using the location to infer a structure that I already know and then and say, oh I'm now touching a handle.
Matt:I know where the handle is and now I'm touching a cylinder and I know where it is. And I didn't have to touch all the points on this handle. I didn't have to hit all the points of the cylinder, but, but now I can associate the handle with a cylinder. So it's close to what we wrote about in the Columns paper. It's close to what you said. It's, we're moving and sensing and moving and sensing, but in reality what we're doing is we're moving and inferring structure more than what I can sense. It's not like I have to go around- It's like a, it's like if I want to learn a map of a room in my living room for example, I don't have to go and touch every part of every piece of furniture to say, where does this chair extend to? And then you know what's exactly... I can say, oh, that's a chair. And, and, and now I can just say, oh, given my point in the room, I know there's a chair here and given I know where the location of chair is, I can predict there's going to be an arm or back or something. So it's, it's a, it's a bit more subtle than just sense, location, sense, location. So you can operate upon it without really knowing what it is.
Jeff:Yeah you can operate. And this is what grid cells, the magic of them is. And so the only way to build your quote map of the world or map of an object is you have to say, start in one place, move and, and then say, Oh, here's my new location. Like, here's some random number of thing that hit my notification. What do I see it there? And then move again. And what do I see there and move again, what do I see here? So, uh, unlike high school math you'd say, oh, x, Y, and Z, that's my location and now I'm going to move, you know, increase x by two. You can't do that. You have some sort of, um, the only way these points are tied together is through movement.
Speaker 1:That's the only way they're tied together.
Matt:That's the tricky thing. And then trying to get to the center of here-
Jeff:it takes honestly, it took us a while to really get, get it.
Matt:Because I always felt like, oh, each grid cell code was a, like a coordinate or something, eh, not really.
Jeff:We should come up with a good analogy.
Matt:I wish. I like that people call them codes. I guess that's something. It's better than nothing.
Jeff:Yeah.
Matt:But the idea that objects are defined as, as sort of as a, as a transition between these two movements and a sensation.
Jeff:No, it's really the oobject is defined by different locations you're in and the only way to get between those locations is through movement. Um, and there's no other way. Like if I say what's over there, I can't say what's over there, I have to say, oh, I have to move.
Matt:Because that's the only way you were ever able to experience the object.
Jeff:There's that plus that's the way grid cells work. If grid cells worked like Cartesian coordinates we learned in high school, then you wouldn't have to do that. But they don't work that way. That's by the way, here's one way to think about it. People who do computer graphics or animation or 3D cad. So those programs all work on X, y's and z's. You know, I have a reference point and I say, okay, I'm going to make a cad model of my Coffee Cup and I'll say, here's the point, the zero point, and then I can define all the features that are related to that zero point. That is not what the brain does. The brain doesn't have a zero point. It just has a bunch of points that are tied together by movement.
Matt:But the interesting thing is you can take two points in cad and you can define a translation between them and you can't do that with grid codes too.
Jeff:You can, but in a very different way.
Matt:Yes, it's not a Cartesian distance formula at all.
Jeff:Yeah, Cartesian coordinates are pretty easy. You just do a little bit of math and you get your answer. Here it's not that way and this gets to this displacement transform that we've, we've discovered here that allows you to say, okay, given two points they look kind of random. How can I establish a vector that says where, where, how far apart they are and what direction are they are from each other and you can do that and you do that going back through the grid cells. It's Kinda like, it's, I'm not going to try to explain to here. It's complicated
Matt:Okay. Okay. I got to ask a question about this. So say you have an object and you have two points and you have a transition, something that moves from one point to another. Can you apply that to any point on the object and expect the same movement to occur in that space?
Jeff:Uh, we're, we're mixing a couple things up here, Matt. Essentially there's, the brain uses grid cells in two different ways. One is to build maps or structured defined objects, and the other is to tell me how to move my body. Okay? Okay. There's really two separate ways of doing that. So I can apply grid cells and say, Oh, my finger's at one location and I wanted to go to another location. How do I move my finger to get there? Then I could say that- it turns out the mechanism that does that- animals had to solve this a long time ago. Like, Oh, I'm, I'm in the woods. How do I get home? I may go a way I've never gone before, but I know which way to go. So then we think what has happened is the cortex has taken that same mechanism but applied it to a very different purpose.
Matt:Now you're talking about path integration, right?
Jeff:Path integration is when you physically move, what is your new location? So I'm saying,I wasn't really talking about path integration. I was talking about given, if I could tell you here's a point in space and here's another point in the same space, like here's where I am in the woods right now and here is my home is and I know those two representations of location and, and they're in the same space because as I wander through the woods to get to where I am now, I path integrated. They're in the same cloud of points, but. And so that's important. Yes, but now I can say I know I can calculate even though I can calculate the straight way from where I am home, even though that's not how I got here.
Matt:Right.
Jeff:I wandered around in the woods and now I'm saying, okay, I need to get home. I can calculate the straight path home. That's kind of clever, um, that you do this without using the Cartesian math.
Matt:Yeah it is.
Jeff:Okay. So there's a, there's a mechanism for doing that. Uh, we think we understand the basics of that mechanism and now that same mechanism is, is used to figure out how to move my finger from the Coffee Cup to my nose. Like it's the same problem. Like, Hey, my finger's over here, how do I get to my notes? So that's very analogous. I hope you can see the analogy, the analogy between like I'm in the woods, I need to get home and from I'm on the Coffee Cup and I want to touch my nose. I'm on a coffee cup, I want to touch the wall or something like that. Yes, that's going on in the cortex. But that's what we call the where pathway to the cortex. Now that same mechanism can use to be used to define object compositions. Right? This is a tricky idea, but if I have two different objects and they have their own spaces, right? If I move in one object, I don't actually move into the other space, I just don't do that. But imagine these two objects physically or in the same world together. So there's a point. Let's go back to the Coffee Cup of the logo. I have a space around the logo defining the logo, right. And I have a bunch of points around the cup, defining the cup. There are two different parts of the world where there's two separate things: there's a cup and there's a logo. Now, right now they're in some on my cup those two things have a fixed relationship to each other.
Matt:They're sort of on top of each other.
Jeff:It doesn't even have to be on top of each other. They have a fixed position. That's the important part. They're fixed. Now it turns out that the logo is on the cup, but that doesn't really matter. At this point, it's not moving relative to the cup. If I take a point on the logo is a location logo at any point in time there'll be an equivalent location on the cup. It happens to be the same physical location, but there are two different representations for it. One's in the space of the cup, one's in the space of the logo.
Matt:That makes sense.
Jeff:So now I have two points, two locations. They turn out to be physically the same spot in the world, but they're literally two different locations because one's relative of the Coffee Cup and one's relative to the logo.
Matt:Two different locations in two different reference frames.
Jeff:That's right. But right now, because I'm not moving the logo, the logo's fixed relative to the cup, there's a one to one correspondence between points in the logo space and points and the cup space. Doesn't matter, because they're fixed relative to each other. Yeah. And so now I have these two points and what I'm going to tell you is I can take the same mechanism that was used to navigate from the woods to home or from the cup to my nose, which is two points in the same space. How do I get from the point in, you know, in the woods to the point of where my home is, that's the same space, the same environment. Or now I'm gonna apply it to say how do I get from a point on the cup to a point on the logo?
Matt:Between spaces.
Jeff:Between spaces but physically actually not moving at all. Physically, it's the same point in the world.
Matt:Right. Right.
Jeff:But I'm going between one point in logo space and the equivalent point in cup space. So I'm converting between two points.
Matt:And you're not moving at all.
Jeff:I'm not physically moving at all but by-
Matt:This kind of trippy, like a wormhole or something.
Jeff:That's a good analogy. It's kind of like like a little wormhole. How do I get to another place by just not moving?
Matt:In your brain, it's easy apparently.
Jeff:Yes, it's a very clever idea. And Marcus came up with the underpinnings of it and um, um, we were working on this problem trying to figure how it does it. And he came up with some of the basic of the solutions that was really clever. But it was actually motivated by some work that was previously done about how animals can figure out their way home in the woods literally. How does an animal navigate from here to here? There was a proposed mechanism for that and we said, oh, that's kind of similar to the problem we want to solve instead of the question, would it solve that problem? So we think it does. And so, so it's the same mechanism, but now I'm not moving at all. I just like saying it's the movement of like an attentional shift. I'm saying attend to the logo, attend to the cup. Attend to the logo. Attend to the cup.
Matt:Oh yeah, yeah. Yeah.
Jeff:And as I do that, I switch spaces. And I can say, now I can say the following, I can say, well, my hand, my finger is touching the touching the logo right now. Where will it be on the cup? And I can figure that out by using this transform. I can say, oh, I know where it is on the cup. So now I move my finger relative to the cup. I can say where's my finger relative to the logo. Uh, in this case I can't really feel the logo, but in general that's the general idea. So a clever idea. It's an old mechanism that evolved a long time ago. We think it's been repurposed to do this thing and I realized some of these concepts are very kind of bizarre. They're actually very elegant and beautiful once you understand them.
Matt:Oh, it just takes so long to distill them into the simplest form.
Jeff:And, and part of our challenge is to pick out the right language to do this and what's the right metaphors with right examples. But I don't think it's any more difficult than other things um, a lot of the listeners will know. If you know how computers work or how deeply how software works. For example, like the first time I learned about a real time operating systems and reentrant code and how the stack point it works when you're doing, you're managing code. And these are details most programmers don't know, but some programmers do this. That stuff is mind blowing at first. It's like, oh my God, how does that really work? Is it really possible, all the stuff does it like that and can it do it fast enough? And, and you know, it took awhile for me to really internalize the nature of a real time operating system. And why does it look like it's doing all these things simultaneously when it's really not. Um, so it's kinda like that. It's not that hard. Anyone can learn it, if you know, but you got to work out a little bit, right? So the first time if you've never seen a computer before and somebody just dumped on you, oh there's this reentrant code and it works by moving the stack point around and we use temporary variables that are complicated and moved over here. And it's like, well what the hell are you talking about?
Matt:There's a lot of new concepts coming in neuroscience right now and people are trying to keep up with it.
Jeff:These concepts are, uh, are sort of similar difficulty I would say, but if you've spent a little time,we're trying to write these papers in a way that anyone could read them and get it. I'm not gonna sugar coat it. These are not obvious things, um, the nature of grid cells and how they work. It's kind of bizarre and even a lot of neuroscientists don't really understand it.
Matt:Nobody said the brain was easy, that's for sure.
Jeff:But it's not ridiculously complicated. It's more like the concepts are new.
Matt:When I first got what grid cells are doing, I was blown away to see that, that, that process was happening in your brain like almost just by itself, it just emerged. It's amazing.
Jeff:Yeah. It's a very clever idea, but it's no more clever than Cartesian math. You just said you learned Cartesian math when you were in high school and you were exposed to it for many years, now you get it. You know, if you'd never been exposed to any kind of mathematics like that and I tried to teach you, you know, it'd be hard, it'd take awhile. So grid cells are like that. They just work on completely different principles than anyone new until fairly recently and um, it takes a little while to sort of internalize it and get it and go, oh, now I know what's going on.
Matt:Now it's almost hard to keep up. There's so much literature out about it.
Jeff:Yeah, but the basic premises aren't changing. It's all these little details around the idea, I think what we've done is, is significantly extend it. We've significantly extended the theoretical basis for grid cells, uh, understanding what they do, how they can do object compositions, how they can represent behaviors. These are ideas that I don't think anyone's ever thought of before. I'm not aware of it. And even the idea that the cortex is using grid cells to build models of the world, uh, in the same way that the entorhinal cortex and hippocampus is using to build models of environments. The cortex is learning models of objects. Even that idea is new. I mean, there are people touching on the edge of it, but, um, it's a pretty big idea. So we're not aware of that existing out there in the literature right now. So we think those are contributions that we can help make.
Matt:So Jeff, I think we're running out of time. I have a couple more questions for you, but I think I'll just put that off until the next time.
Jeff:Alright, well that'd be great. I think we should check in every once in a while on this and see what's new.
Matt:Yeah. Um, well we've got a podcast now so we'll have you as a guest another time.
Jeff:Things are happening here rapidly here at Numenta. We are learning, you know, these sort of pieces are falling into place. I've said this before, it's so true. Um, so it's an exciting time and um, and that means it's going to be new things all the time.
Matt:A lot going on this fall. So stay tuned to Numenta, keep watching numenta.com and follow our YouTube channels and stuff. Thanks Jeff for joining me for this chat and it was a pleasure.
Jeff:Thanks Matt. It's always fun talking to you.
Matt:All right, take care and don't forget to subscribe to our podcast. Thanks Jeff Hawkins. This has been Numenta On Intelligence. If you like what you hear on the podcast and you want to discuss ideas like this with intelligent, friendly people, be sure to join HTM forum at discourse.numenta.org. Our online community was created around the Numenta open source project and continues to thrive on HTM forum. Hundreds of folks interested in HTM and related theories, share ideas, experiments, and open source code. If you are an HTM theorist, engineer or a programmer or just a hobbyist, HTM forum is a friendly place to keep up with the latest on HTM technologies. Thanks for listening to Numenta On Intelligence. Be sure to subscribe to our podcast on your favorite podcast service. To learn more about Numenta and the progress we're making on understanding how the brain works, go to numenta.com. You can also follow us on social media at Numenta and sign up for our newsletter.