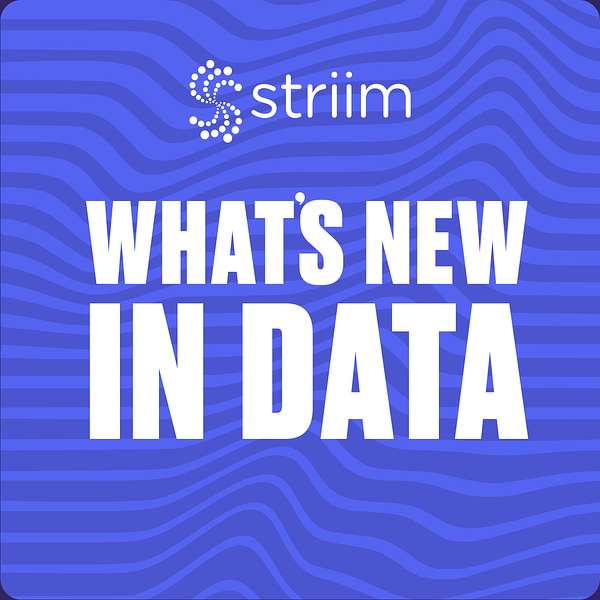
What's New In Data
A podcast by Striim (pronounced 'Stream') that covers the latest trends and news in data, cloud computing, data streaming, and analytics.
What's New In Data
AI Principles with Patrick Miller, Head of Data & AI at Newfront
We dive into AI principles with Patrick Miller, the head of data and AI at Newfront. Patrick, a seasoned expert in enterprise, AI, and machine learning, casts a unique lens on the role of AI at Newfront where they offer modern insurance, emphasizing the crucial role of "human in the loop" systems. Get ready to explore how data teams can leverage Large Language Models (LLMs) to create tangible value for companies, with insights drawn from Patrick's vast experience in AI roles at Google and other tech giants.
We also dive into the importance of transparency and explainability in AI product development and the impact of LinkedIn data trends on innovation. Patrick shares thought-provoking ideas on tying data initiatives to direct financial impact, providing valuable guidance for data leaders navigating a tightening cycle. This episode is a treasure-trove of insights for any professional interested in the practical applications of AI in business. Tune in to glean from Patrick's knowledge and experience, and understand how to navigate the intersection of AI and insurance.
What's New In Data is a data thought leadership series hosted by John Kutay who leads data and products at Striim. What's New In Data hosts industry practitioners to discuss latest trends, common patterns for real world data patterns, and analytics success stories.
Hello everyone, thank you for tuning in to today's episode of what's New in Data. Super excited for our guests, today we have Patrick Miller, who heads data and AI at New Front, which is a modern insurance brokerage company. Patrick, how are you doing today?
Speaker 2:Doing excellent. John, thanks for having me on the podcast. It's been a fun week, looking forward to chatting with you and talking about data and AI.
Speaker 1:Absolutely. For the second year in a row we got to meet face to face at DBT Coles. Last year it was in New Orleans. This year it was in a nice, sunny San Diego. It was a great time. Patrick, you have an amazing experience from working at Google on enterprise, ai and machine learning roles and data science roles at several companies. Now you're leading data and AI at New Front. Tell the listeners a bit about yourself and your background.
Speaker 2:Yeah, great, I'm Patrick. Everyone I lead data and AI at New Front, Been at New Front for two years. As John mentioned, New Front is a tech-enabled commercial insurance and benefits brokerage. It's mouthful. We basically help companies make better decisions about protecting their balance sheets and getting their employees the right set of benefits, whether that's healthcare, 401k plans. My team at New Front is focused on two fronts. We do the normal analytics thing helping to make New Front as a company more data-driven but a lot of our attention is focused outwards, towards our clients, our companies. How do we use data and AI to build great products that fundamentally help them make better decisions about their own businesses?
Speaker 2:Before New Front, I was at Google where for multiple years, I was running a machine learning team focused on Google's enterprise. So internal Google operations. My team built dozen plus ML solutions to support Google's legal team. There are people operations team, marketing team. We built solutions to help run Google's buildings more efficiently. Got the chance to work with the top research minds in the world and with some great applied machine learning engineers, learned a ton and always been a practical ML data science person. Before Google, I had a couple of different roles Wanted a hedge fund, Wanted a publishing company. Funny enough, Insurance and publishing both really old industries. I guess there's something about that that attracts me to this type of companies. My passion is around taking machine learning, data and actually changing how an industry fundamentally does its work.
Speaker 1:This show is called what's New in Data, but so many times we end up talking about what's old in data, because old is gold in terms of these principles that are carrying over from decade to decade. Even though there's new technology, it seems like we're implementing a lot of the same best practices and core foundations and principles. Speaking of that, you've created a set of AI principles for your company. They seem pretty practical in general for how a lot of teams can approach AI. I would love to hear about your AI principles.
Speaker 2:Yeah. So AI principles for folks who aren't aware, or just a set of guidelines that you can go to when you're building machine learning or AI solutions into your product or into your processes that help keep you in check, because there's a lot of things that can go wrong with deploying ML or AI solutions. I'm not talking wrong like Terminator wrong, but wrong in terms of doing things that are biased or leaking customer data. Making sure that you have a set of principles that you always fall back on before you deploy something to production. I find really important Newfront's AI principles I built together with our CTO, co-founder Gordon. The exercise itself was truly a highlight of my career. When I was running that ML team at Google I mentioned. I got to work with some of the best AI ethicists in the field as well. I got to learn from the best in terms of what matters, how to use technology to solve those ethical problems and being able to take what I learned there and turn it into something that's tailor fit and meaningful for Newfront as a company and for our clients. That has been quite the experience.
Speaker 2:We have five principles. We kept it short and sweet because we wanted to make sure that they all met something for Newfront. These aren't the only five principles that if you go out and build a set of principles yourself, you might come up with something different, but these are really what sort of tied to the ethos of the company. The first one, and the most important one in my opinion, which ties to Newfront's value statement, is that we favor human in the loop AI systems and leverage our insurance professionals expertise. We don't really build many systems where we're automating decisions. Insurance decisions for commercial entities are way too important to just automate. If you're trying to protect your balance sheet, where if you get a securities class action lawsuit and you are not protected and you had some system make that automated decision for you and it was the wrong one, you're probably going to feel pretty bad when you go bankrupt. We try to avoid automation and really have human in the loop expertise alongside AI-empowered advisory services.
Speaker 1:I want to drill into that one. That one's super important. There's always this boogie man argument that AI is going to just replace humans and automate everything we do. The point you're making here in your principles and this is from years of experience that you've had is that you actually still need a human in the loop, but you're empowering that human to do less manual work, less things that are error prone, and really just sort of like an airline pilot, you're monitoring the system, making sure that it's working properly.
Speaker 2:Yeah, 100% Not always the right thing to do, in my opinion. I think it's also how you drive the most value with AI, because going from that last 80% to 100% in terms of improving your accuracy, that fully automate something like advertising decisions of something that's at lower stakes that's pretty hard. That last 20% of performance is really hard to eke out. You see that as a problem with self-driving. You can't mess that up, and so you've seen self-driving companies struggle to roll out as quickly as they wanted to, because you have to be really close to perfect for things that you're automating completely.
Speaker 1:Absolutely, absolutely. This seems like a good set of principles for data teams that have plenty of experience doing data ingestion, pipelines, processing, materialized views and metric layers and things like that. Would you say this is adjacent to adopting AI?
Speaker 2:Setting up principles is adjacent to adopting AI.
Speaker 1:Just in general, is adopting AI adjacent to what the work that the data teams are doing now? A?
Speaker 2:traditional data team, absolutely. Folks talk about the data science hierarchy of needs all the time, and most data teams focus on the foundation and say they'll get to the top part of the pyramid, which usually is AI, sooner or later. I think what the revolution of large language models in the past year or so has done is given teams the opportunity to skip a lot of those foundations. No longer need to train those models in-house. You need to be careful about how you deploy the technology and you need to be mindful about what data you're feeding in.
Speaker 2:But the hardest part about building machine learning from scratch is collecting data and ensuring it's correct and building the infrastructure around that. You get that for free now. It gives you the opportunity to skip some steps. Data teams should be taking advantage of that. Product engineering teams can do this too, but AI has a fundamental data component to it, and data teams are positioned well for something that is experimental, for something that requires the ability to be quick at iterating. So I would certainly highly encourage data teams who aren't thinking about building AI products. Don't miss the book. Now is your opportunity to get into something that is tied to company value directly.
Speaker 1:Absolutely. That's such great high-level advice and totally confirms what a lot of the thought leaders in the market and in the data industry are saying, which is data teams. You have the leverage because you currently own the data, the storage, the prep, operationalizing it, and AI is right there. When you look at the actual AI stack, a core component of that is our vector storage. It's still a storage layer. It's a storage and a serving layer of data. So let's say you're a data leader hypothetically, and you historically have not done machine learning in AI, how would you go about trying to adopt it in your company?
Speaker 2:Yeah, so what I would say at Newfront, I haven't hired a bunch of ML engineers onto our data team. I've hired folks who are data savvy, who have an analytics mindset. I think the most important thing of an analyst is being quick to failure, quick to experimentation, being able to iterate on ideas, and so if you have folks like that, even if they don't have an ML background, you don't really need it. If you're not going to be building models in-house and so where the value is for your company is not in natural language, I think my answer would be different. You probably need to go out and find some folks who understand how to build a model for your domain-specific data set. So if you're using a lot of structured data for predicting structured data, you probably do need to get some expertise in-house. But if what you have is a bunch of unstructured natural language data, pdfs, web text if that's fundamentally your data and there's value prop in there, I think it's really easy to get started.
Speaker 2:Data professionals have experience with Python. That's really all you need in order to start working with GPT or other large language models out there. So, starting with pain points that are experimental in nature and just setting aside time to rapidly iterate on a prototype is how I would recommend getting started. That's how we got started at New Front is we weren't trying to do anything strategic with LLMs until we had a hackathon like 10 months ago or so nine months ago and we came out of it with three product ideas that we have now used in winning business with clients because they're so impactful and so quick to spin up, since you don't have to build that model yourself. So I'd recommend just getting out there and trying it. Start a hackathon, an internal hackathon where your team's focused on it. It really is easy to get into it now, which is the upside.
Speaker 1:I love the idea of a hackathon, which really makes a little bit of a competitive environment for light of fire under people and get them to start thinking and building with AI and then B at the same time.
Speaker 1:It shows the sort of the practicality of hey, your data people do have the leverage to start building AI applications because they know Python, they know SQL, they know the data layer. What we're seeing is so many of the. It's always this idea of a crawl, walk, run and you can crawl by starting to adopt the vector extensions of the data tools that you already have. I mean, there's so many great announcements here from Google, from Microsoft, from AWS, with just simple vector extensions and Postgres. Now that can be a foundation for LLM applications, internal chat GPT type experiences. I know the chat GPT thing has been. People have been talking about that over and over again but at the same time, if you don't have it yet, internally a lot of people are going to be asking where is it? It's becoming such a ubiquitous, popular AI experience now that everyone wants a piece of it.
Speaker 2:Yeah, 100% externally I mean for B2B companies out there. I'd encourage with an external mindset as well. What are your clients have, or what are your customers asking for? And so, yeah, building things that make your teams internally run more efficiently is it could be a good starting place. I think what we did was in the hackathon, we came up with only ideas that our clients wanted.
Speaker 2:So one of the flagship products that we've built right now that everyone is loving is this benefits assistant chat bot, where it's very simple. It uses the vector store technology you're talking about. So data professionals are great at being part of this project, where we just load up one of your benefits guides if you're a client of ours, and we deploy a Slack bot that has a GPT layer that does the retrieval augmented generation thing with it. It's a little more complicated than that. I think we'll write a blog post about how we did it successfully, but it's getting started. There is quite easy because there's lots of playbooks here. It is data focused and the best part is it can be client impacting. It can be a product that drives revenue directly.
Speaker 1:And that's the other great thing about this that it can actually be a customer facing data product, and this is one of the core ideas of bringing value to your company through data, which is productizing it either externally in a way that makes money or internally, through operational use cases, in a way that saves money and drives efficiency. But the AI driven data product is certainly something that data teams can have a lot more leverage to launch than ever before. So it's really exciting times and it's great to see folks like yourselves leading the charge here, starting with these AI principles that are just something that's broad and can be applied to a lot of data teams.
Speaker 2:Yeah, just to shout out a couple more of our principles that I think are really aligned with existing data teams. One of ours is incorporate transparency and explainability into those AI products, and that often means being able to link the data that is being explicitly referred to from the AI in the output. And so you know that's essentially either joining in structured data or, you know, doing some data operations on top of the AI output. That's very aligned to what data teams are good at. And then another one is, you know, being very thorough about testing performance before deployment. So teams that are really good at A-B testing things.
Speaker 2:I find this it's a little scary to me at how LLMs are moving so quickly that people are like let's just throw that into production, like let's just get it out there and like see what happens. That kind of scares me a little bit. I firmly believe you need to do some validation before you. You know you put it out into the world and data themes again, are very good at experimentation, a-b testing things and you know setting up your data sets that you hold out for evaluating how your end-to-end model is doing. You know the principles kind of led itself to data professionals getting really involved in this work instead of you know, at Google, my team was software engineers only. I didn't have a single like data scientist or engineer on my team. A lot of companies will just do this with software engineers. I think it's missing the mark a little bit, and data teams should be the ones being proactive about getting themselves involved.
Speaker 1:Well, you know, one of my favorite comments here in the market from Joe Reese, who's a best-selling author in the data space, author of Data Engineering Fundamentals is you know, if you want to look at the future where data is going, look at software engineering. You know all the same principles, all the same ways of you know doing sprints and test-driven development, domain-driven development. It's all coming right Agile. If you're not already using Agile, you know it's. Your data team might be a little behind. So you know it's such a good observation and call out from you that you know. Traditionally you had software engineers building AI and ML at Google, and now you see a future where data people have leveraged to do this with the right principles. Are there any other principles that you would want to shout out?
Speaker 2:So name most of them. The other two are around security and privacy of our clients' data. That's super important, right? You don't want to be, you know, hacking together or something where you throw it directly in the chat. Gbt, where you know, OpenAI clearly states that we're going to train on your data if you don't have an enterprise account. So you want to be, you know, very mindful about what use cases you pick in terms of from the security and privacy aspect as well as like how you store data and use it. And then the last principle is around making sure that we don't reinforce unfair biases. This is a really tough one, and but a very important one. You know, we fundamentally believe insurance and benefits should be accessible to everyone. If we don't want to be building AI products that don't make that a reality.
Speaker 1:That's such a good point because you know if there was historically inequality, you know, based on, you know, just hard cold data, right. Like you're saying, you don't want to reinforce it, right? You want to use data for good, right, and look at opportunities to improve things, and you know, I think that's a really beautiful principle and one that teams should be looking to improve. Just because its status quo and an AI model as learned from it doesn't mean that it has to be the future.
Speaker 2:Yeah, we ran into a test case where we were for our benefits assistance, we were kind of violating this principle a little bit and we had, like you know, five alarm, fire, go, fix.
Speaker 2:You know how our pipeline was working because we were returning you know what was in a benefits guide around cancer resources, but it was only about breast cancer and so it was only directed towards women, and you shouldn't be. You know, you should be making sure your product is inclusive in terms of what it's returning, in terms of its responses, and so we added another layer on top of the model to make sure that the response that it's giving, and its chatbot responses, is inclusive and not just focused on a single group. And it's tough, right, it's tough to do something. Principally, it's not the easy way. You have to build out the system and you have to make sure you test things effectively. But it is the right way, and I think you know especially where companies are going these days. They, they, they, more and more at least maybe I'm not in this, but they more and more want to do things the right way.
Speaker 1:Absolutely, absolutely. So, yeah, those are some amazing principles. If you want to just enumerate over them, you know, starting with human in the loop, you know you also mentioned security and privacy. Don't reinforce things from the past that aren't so great, right? We don't want to reinforce lack of equity and the other ones.
Speaker 2:If you want to go through them, Yep, so human in the loop, transparency and explainability. Build with that in mind to build trust with your users. Make sure your system is thoroughly tested, both from a performance perspective but also from a safety perspective. Ensure the security and privacy of your customer clients data. You don't want to be leaking that data, whether it's through your model or you know, straight up, just leaking it and then you know. Finally, avoid reinforcing those unfair biases that may exist in your training data or in the biases of, like an open-source model or a proprietary language model that you're accessing.
Speaker 1:Amazing, great principles that every team approaching AI can can think about as they start their journey or even if they want to make their existing AI practices much better. So thank you for sharing that, patrick, of course. So this year, or I should say this week, we caught up in person at coal less. This is dbt's marquee conference. It was a really great one down in San Diego Obviously beautiful, beautiful weather, beautiful setting. The dbt team did a great job setting that up and Making sure it was a really, really nice event that everyone had enjoyed. And there were so many great announcements there and I want to hear from you what were some of the announcements that really piqued your interest.
Speaker 2:Yeah, so coal last second year. This is only two years now that they've had it in person, but second year that you know, I got to go and have to say amazing conference. I'll definitely be going again next year. Just great, great energy, great hallway Conversations hanging out with leaders in the space.
Speaker 2:In terms of announcements and talks, you know dbt labs really hit on all the you know LinkedIn data fluencer buzzwords. I think you you mentioned, you mentioned some of that in terms of you know data contracts, data mesh, semantic layer. You know their new data catalog improved feature with dbt Explorer. I think they did a really good job this year of actually addressing folks, users pain points. These are things that, as a Data leader of a of you know a large team, you keep hearing from the folks on your team right, our data is broken, data quality is low. You know it's really hard to keep people in line with. You know our massive, our massive warehouse deployment at this point. You know semantic layer, like making sure people are using the right things when they're doing analytics. Like these are all things that users care about and so I think dbt labs did a good job of orienting around you know the zeitgeist of analytics problems that have been, you know, front of mind for the past year.
Speaker 2:You know, for us at new front, I think a lot of these are, you know, more third, third order effects, third order concerns, you know they're, they're a lot around.
Speaker 2:How do you make the analytics team is more efficient? So you know data contracts like how do you make it so I don't have to go up to a data producer upstream and get them to? You know, roll something back. It makes you more efficient at that. It reduces the stress on your team, ensures things don't break in production, it, you know, but at the end of the day it is really sort of an efficiency play of. You know, how do you make an analytics team more efficient, at least in my perspective and at new front, we have, we have 15 data professionals and other folks who contribute to our warehouse stack and, and so they're, you know they're features that sound interesting but not sort of top of mind for, I think, probably the smaller teams Likely makes sense for it for DBT labs, if you're running a 50 person Data team and you have, you know, 1500 data models in your warehouse, these things are becoming much bigger pain points than they are for, for for my team.
Speaker 1:Absolutely and Like, like you said and we were talking about this in person it seems like DBT Did look at you know some of the things they were hearing from the community. You know, and you can call it kind of LinkedIn data trends Meaning on the. You know a lot of them are discussed on the LinkedIn feed and comments and groups and and and so on. But it is great to see you know a company of DBT size really listening to the community, looking at things like data contracts, like you mentioned, actually operationalizing it in their product and it makes sense, right, because they're they own the, the transformation layer, and making it Efficient for data teams and analytics engineers. Put some of those in place. A lot of teams are already doing, you know, some form of that With tests and DBT. Right, my team, you know we also have a notion of data contracts here and the DBT pipelines are a part of that. We also use our streaming pipelines for that. So it's interesting to see you know the rate at which they're listening to the community and innovating.
Speaker 1:And, like you said, it's a great hallway Conference as well, meaning you know, if you just walk the hallways, you're gonna run into some of the the smartest people in the world and, you know, get their take on a lot of things. You and I both caught up with the obvious of asylum. You know, for example, and so many other great people who were out there. Sarah Krasnik was there as well and TJ Murphy can't shout out everyone, but there's a long list of people that were there Jacob Madsen and you know being able to run into them and catch up with people there. What were some of your favorite hallway conversations? Oh, if you can't share, yeah, because that's usually the record, but yeah yeah, there were.
Speaker 2:There were a lot. I mean, my probable favorite was needling Ben Stansel about the Braves losing the share. But actually for, like, data conversation, actually for data. You know data related conversations. You know I think we had a lot of conversations with some folks from Brooklyn data company, like Elon, and Caught up with Barry from hacks, a little bit around how, in a tightening cycle, like, how do you, how do you like, measure the impact of a data team? How do you, how do you, make the case for further investments?
Speaker 2:I think there are a lot of really good discussions or around Around that I talked with, is it true?
Speaker 2:I talked to a lot of different Vendors around vendors and you know thought leaders around how they are thinking about data contracts.
Speaker 2:I feel like everyone's coming up with a solution for, for, for contracts, and they all, they all make sense and, and so it's really cool to see different perspectives on how they're thinking about implementing it, why, and why their way is is the way to To do it. And then I think, finally, what if he excited me the most of the conference in terms of other talks and hallway conversations was the, the few folks who are talking about. You know how do we turn a data team into a growth engine, because that that hits really, really close to home. So Tejas had a talk with red ventures, which is a company, funnily enough, literally my backyard. Talked about how to turn data teams into into growth engines using, you know, data activation or whatever they call it these days. And I think, since this had one too, and and I think that's a hundred percent what data leaders should be thinking about, and In a tightening cycle like like we are in today, absolutely, absolutely.
Speaker 1:And you know, and you touch on so many great points and, I'm glad, those that's the subject of hallway conversations now which is, you know, turning data teams into a growth engine. And you know this does come back to the, the macro economic climate, which is, you know, we're, you know, in the for the past few years and it is very timely, you know. And then, of course, you know there are a lot of great people were impacted and you know it's just across the board. Companies are tightening and has nothing to do with skill level, but you know you see some, some great people having to move around from from company to company as a result, and it really comes back to data leaders thinking about this tightening cycle and, like, in your words, turning data into a growth engine where it's not just a cost center. So what are some of the? What's your key advice to data leaders on approaching this tightening cycle?
Speaker 2:Yeah, so 100%, you should be tying yourself as closely to financial impact as you can. I'm a I'm a full believer in the the berry argument. The very CEO of the CEO of Hacks who you know, essentially says that for internal analytics teams, the only way you really actually measure your impact is through stakeholders who go to bat for you. And so if you have stakeholders who really can't live without you, that that's another way to you know, survive or do.
Speaker 2:Well, during a tightening cycle of, if you're like your chief marketing officer is saying like I need to hire more, you know, data scientists to support my team, that's. You know that's one path, but you know, because that's really decision supported in a lot of ways, you're you're not seeing executives do that, and so you know, if, if my stakeholders aren't putting their money where their mouth is and saying like, hey, spike, ceo of New Front, we need more analytics resources, I'm not going to be the one going to spike and saying, hey, spike, we should hire more analysts. I'm going to be making proposals on how we can use data to drive direct financial impact, whether that's revenue or, you know, operations saving, like saving direct dollars by automating things. Those sort of first order effects is is where I focus on and where I would encourage other data leaders to really be figuring out ways where you can actually measure your impact with quantitative measures instead of just service.
Speaker 1:That's great. So tying yourself to financial value and you know to really put some data behind it. Obviously, if you're a data team, you need to be data driven. You know using surveys in the process. Does AI play a role here?
Speaker 2:100%. I mean, as we talked, sort of the beginning. You know, coming out of that hackathon, I'd say 75% of my teams are now focused on building, in some form or fashion, a revenue driving product that is has AI at its core. It's not just us developing that product. You need to be cross functional and work with, with you know software engineers to build the interface, for example. But AI products is a great place where you can have direct impact on the business, and so there's other places to you know making you going after the right leads, lead score and you know all that sort of stuff has closer impact revenue. But, like building product, now's the chance right. Now's the chance to get in there and really start building product, because a lot of the products that folks want are a driven.
Speaker 1:Absolutely, and you know there's this component of AI which ties to revenue generating, customer facing data products. Is there also a case for AI augmenting internal analytics and increasing productivity there and tying which is another way of tying it to savings internally?
Speaker 2:Yeah, to me it's more of a second, the second or third order of fact of making teams more efficient. So I think it's interesting. I know there's a lot of companies who are doing really cool things here, like Delphi or Delphi I forget what they call it. If it's Delphi or Delphi, you know, larry, non-pop.
Speaker 1:Yeah.
Speaker 2:David David.
Speaker 1:Gaiatella, this company. Yeah, great yeah former great podcast guests in the past here on what's new and data. We love having him on yes, ai augmentation for your data teams. Like the work that Delphi's team is doing super exciting.
Speaker 2:Yeah, I think it's interesting and it's a great product. Again, you know, at the end of the day, what's the final output. Is decision support right? You know you're helping your executive team, or whoever your stakeholders are, make better decisions. More data driven decisions, which is great, you know. I think it's important. I just think, in a tightening cycle, what I would be looking for is more like automation, where we can, instead of using like third party BPO resources in order to do data input, we do data extraction. We do it 10 times cheaper, like that. That is really direct sort of cost savings, whereas making better decisions and making more of them because we have an LLM layered on top of our semantic layer Great, important, but it is really a second order effect. It's like it's so hard to tie that to real value in a number of format, unless it's just unless it's your stakeholder really going to bat for you.
Speaker 2:I think of early in my career when I was at McMillan the publisher and we were. We were renegotiating a contract with Amazon. That was the biggest strategic decision we had to make for the year, and the you mean some another data scientist at McMillan were building this cost model and, of course, I put it on my resume that I saved McMillan X million dollars over the next 10 years because because of you know that decision. But was it really me who did that? Or was it the fact that you know our CEO was a really good negotiator? Or was it the fact that you know the strategic, you know the strategic positioning was was, you know, played the bigger factor? Like it's really hard to draw that direct impact and whereas it's not hard at all to draw the direct impact of we no longer have to outsource this, we have it completely automated via, you know, some extraction workflow that we we built out internally or like customers or clients are literally buying this product that we built. Like that, that's, you know, that's direct impact.
Speaker 1:Absolutely, absolutely. It's really having the impact and working backwards from that, you know, every time you approach your, your data, projects and initiatives, anything you're going to time. Spend time and people resources on Patrick. Where can people follow along with your work?
Speaker 2:So I've I've bored data Twitter, so you can. You can find me on our data X. I guess you call it data X now, I don't know. Gosh, yeah, folks can follow me on LinkedIn. I wouldn't call myself a data influencer, but I do post every so often on LinkedIn around what we're doing at new front with data or AI, and so LinkedIn, patrick Miller, search new front, that's where you'll find me.
Speaker 1:Amazing Patrick Miller, head of data and AI at new front. Thank you so much for joining today and thank you to everyone who tuned in.