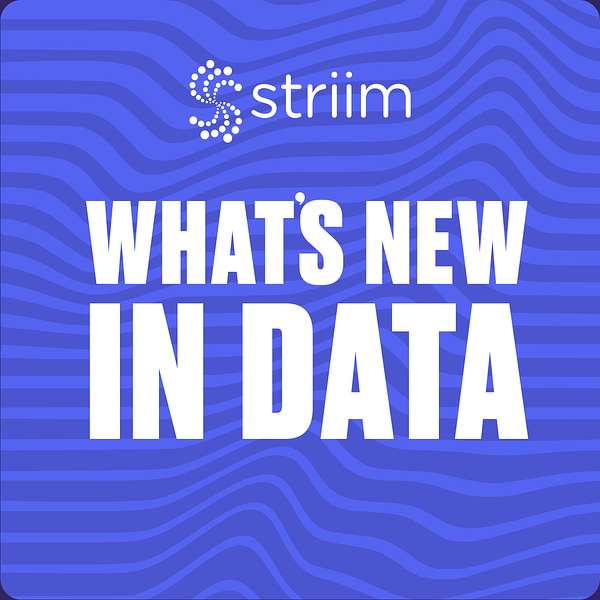
What's New In Data
A podcast by Striim (pronounced 'Stream') that covers the latest trends and news in data, cloud computing, data streaming, and analytics.
What's New In Data
Leveraging Data and AI in your Go-to-Market Strategy with Everett Berry from Clay
Everett Berry joins the show again to share fresh perspectives on applying data in your sales and go-to-market strategy. We talk about his journey to a key role at Clay, where he leads Go-To-Market Engineering to tackle the complexities of serving and enhancing data for sales. Everett discusses how Clay's tools improve data accuracy and reach, helping businesses streamline revenue operations with smarter data use.
In this episode, we also dive into AI's impact on sales operations and revenue processes. Everett and John explore how AI agents and human teams interact, the integration of customer data platforms with CRMs, and the merging of RevOps and data teams. Picture a future where AI autonomously handles data tasks, changing how teams collaborate and redefining organizational roles. For anyone following the fast-paced world of sales tech, this conversation offers a forward-looking view on autonomous data management and its potential to transform business practices.
What's New In Data is a data thought leadership series hosted by John Kutay who leads data and products at Striim. What's New In Data hosts industry practitioners to discuss latest trends, common patterns for real world data patterns, and analytics success stories.
I'm You Everybody. Thanks for joining us today on a special episode of what's new in data. I am a guest here at clay studios. Welcome with Everett Berry. Everett. Great to see you again. Last time you were on what's new in data, one of our most popular episodes, we were talking about, you know, cloud price optimization, using these big data infrastructure products, and you currently own, you know, one of the most popular open source price calculators for cloud instances, and many people follow you for that. Update us on what's going on with you now. Yes, well, it's great to be back, John. I think this might be the first podcast where I'm going to repeat guests, so thank you so much for that. Um, I, uh, was leading GrowthAdvantage, a cloud cost optimization company, um, the best cloud cost optimization company, and really had a lot of fun there working on ec2instances. info, which of course is all the, um, all the AWS prices, and actually now Azure prices as well. Hopefully, I would add that. We added that, uh, recently, but, um, but actually my primary focus advantage was not, um, technical cloud pricing, but actually growth. And of course, the reason that we operated that tool is because when you, when you operate a popular open source tool, many people know you for that and become aware of your brand, um, through that. So in the backend, what we were doing advantage was operationalizing a lot of that data to understand who are the people that were. Um, visiting us on EC2 instances, uh, or who are the companies I should say. Um, and using that as part of our, our go to market motion, which involved a tool called Clay. And so, um, yeah, for those not familiar, Clay is a data enrichment tool. I would say it's, it's, it's both a data enrichment tool, but also a largely a no code, go to market programming environment. Oh, very cool. Um, and so you can build almost anything that you want to accomplish as part of your go to market motion inside clay. So a lot of people use it to enrich their CRMs, but you can also use it to run outbound campaigns in Bali and qualification. We just launched round Robin, my personal baby feature, um, which I've been very excited about. So now you can do weed routing and clay. So it's, it's kind of the spreadsheet S tool where you can do all these different things. And so at Vantage, I was building go to market campaigns and motions of data enrichment in clay all the time. And, um, and, uh, I've, I've long been a fan of developer tools. Uh, my role before Vantage was also on developer tools, company leading growth. And so over time I became closer and closer with the clay team and now I'm here actually leading, um, go to market engineering, which, which we can talk about, uh, so kind of a, kind of a circuitous circuitous path, but, um, some of the same principles of delivering great technical products to technical end users kind of really applied with clay. Um, and actually a lot of the. A lot of the things I learned about, um, cloud pricing data, the complexities involved, the systems and how they interface, uh, those applied to clay and to revolve systems as well. Yeah. Super cool stuff. There is a huge segment of go to market teams that are trying to not only work harder, but work smarter, leverage their internal data, third party data, data from the web, get signals from, from customers. And leverage that in their sales and revenue operations, you know, outbound sales, marketing campaigns, what have you. And then you also see these data teams that are so business centric and they want to create their data as a product for the go to market teams and the leverage. So it'd be great if you could explain to us, you know, what is go to market data and how can we best leverage that? Yeah. Well, um, it's among the messiest data in the world is one way to talk about it. When you think about data enrichment, there's a couple different metrics that people use. They interchangeably use coverage, math rate, and fill rate. Match rate is largely, if I have some piece of data, let's say I have a signup, let's say I have a Gmail signup to my product. Do I actually know who that person is? Um, you know, if every PBA at, um, Yahoo. com signs up, For your product. Can you actually say, okay, every works at clay, clay has X employees. Clay has Y revenue has raised Z money from these investors. And, um, the reason you need that data is largely to start to segment the users of your product. Or if you're doing an outbound motion, the, the people that you're going after into kind of the appropriate messaging route them to the appropriate sales people and so forth. But actually in most cases, the, the coverage of, uh, data points that companies have on their own leads and on the leads they want to go after is very low, is very poor. And that's actually, it's, it's true. Uh, sales at companies, it's especially true of product led companies, um, where you're just getting thousands of signups a day and, um, they're activating on the product and they're using it a little bit. And there's different data points that you have about them, but stringing those together to kind of create a, a unified, consistent view of those users, and then to properly segment them and route them to sales to generate revenue from them is, is a, actually we have a very challenging problem. Um, and so, um, the couple of like primitives with go to market data, one is the domain that we could do a whole hour on domain. There's so many problems with them. In Clay, you have various functionality to, to just clean your domains. And what that means is, um, maybe you go to the domain website, actually it's, uh, parked on GoDaddy. So that website is not related to any kind of valid customer or company. So if you route that lead quote unquote to sales. Sales is like, well, I, this is just a waste of time. I don't know who this is or this company is probably not even legit. Um, you also have situations where the domain belongs to a company that's been acquired by another company. And so if you just are looking at that domain and you're maybe looking it up in some data enrichment tool, it might tell you like, Oh, okay. loom. com. You know, uh, 500 employees, you know, 500 million in revenue and, and, um, and various things. Actually Loom is part of Atlassian and they've been part of Atlassian for 18 months. So actually the team that is signing up with loom. com belongs to a 30, 000 person public company. Um, and, and so if you don't have, uh, systems and technology in place to even just do the most basic domain cleaning things, you quickly create a mess where you're missing out on opportunities. You're, you're routing sales opportunities to the wrong teams that aren't equipped to deal with it. You know, public company, or you might have like strategic reps who are, are equipped to deal with a public company, but they're getting sent startups for, you know, whatever reason. Um, this also happens with subsidiaries. It happens with companies that have global operations with different, with different, um, you know, websites that they have for like the UK or for France. And this is just domains. So you also have this exact same problem for every other data point that is relevant for, for go to market. And so basically it's helpful to have a situation where you have tooling and technology where teams can go in and kind of clean and properly and reg and manage this data. Um, and it's become a lot more of a top of mind problem for companies. as they have collected more and more of this data. And instead of creating a, a unified, amazing view of their customer, they've actually just ended up with a mess of conflicting information. Yes. And that is one of the challenges we generally see with, you know, third party enrichment tools, or just kind of brings in a bunch of stuff into Salesforce and you actually go check it and it's inaccurate. And, you know, there's Unclear ownership is okay. Who's, who's in charge of the quality and the accuracy of this data. And once that's kind of signed off on how does sales actually leverage this? Cause okay. The first thing, yeah, the ideal process is okay. We have all this amazing, uh, third party data, internal data, and we're going to automate our sales processes with this and increase efficiency, close deals faster when more, you know, get a higher conversion rate. The reality is that there's so much time spent, you know, Cleaning data from the data teams and the go to market teams. And then from the sellers themselves, having to go validate that stuff. And maybe you'll ask clients questions based on some wrong information, which kind of starts the deal on a, on a bad. Yeah. So how does go to market engineering play a role here? So the essence of go to market engineering is to take that whole problem set and abstract it away from sellers. What we try to do at Clay is present. A clean, accurate view of the accounts and contacts that sellers are working and their CRM, and then in one other tool, which is their sequencing tool. And that might be outreach or sales loft or, or LEM list, or there's, there's many tools that you can use for this, but basically, um, when I've been working with SDRs in the past, it is so true that in many cases, like 50 percent of their time was spent finding contacts. Finding different contacts, working on messaging, re ranking their accounts that RevOps gave them because their view is that actually the prioritization should be different. These things, I think, I think excellent go to market engineering just solves and abstracts that problem from sales reps. And you want a situation where sales reps are spending 80 to 90 percent of their time, either communicating with customers on some channel. I'm, I'm pretty channel agnostic. Could be LinkedIn, email, text. I love the text customers. Um, or they're like in calls with customers or they're doing sort of creative deal supporting activities, like building decks, finding information from technical teams and these things. And I think without strong go to market engineering, or that's sort of a, you know, the new term of without strong sales ops and rev ops and, and excellent tooling and automation. The avalanche of data that is possible to get about your, your prospects and your, your customers makes it so that sellers will inevitably spend a lot of their time not doing those like high value add selling activities and instead arguing about, um, employee counts and which bucket this certain company belongs in. Um, so I, I strongly actually believe that go to market engineering is, is. is the kind of key discipline that is going to help companies leverage all of the, um, new data points and the automation in the appropriate way. I think, I think basically if you don't have a go to market engineering function or kind of like strong technical ops people, you should also question whether you should, you should be trying to pull in more data and actually use it. Um, because, because, Uh, you need to actually have both. Yeah, absolutely. Because, you know, Sales Ops and Rev Ops and Marketing Ops, these are all disciplines that have been around for, for a while. Uh, and, you know, how, how is that different from what Go To Market Engineering is, is, uh, turning into? Yeah. Um, I think Go To Market Engineering is, is kind of solely focused on data and automation, quite frankly. Rev Ops is very strategic. Yeah. Rev Ops is in charge of what territories are, um, built for, you know, upcoming year, I've done a lot of fiscal year 2025 planning in the past few months with teams, sales office in charge of kind of process and, and, um, how do we, how are we kind of moving deals forward? Do people have enough deals? They Rev Ops to determine if I give a salesperson a 50 opportunities this quarter. More likely than not, will they hit their quota? This is very strategic thinking that involves understanding of, uh, the customer they sell to, of the sales team they have today, of the skill level of everyone involved. I think go to market engineering supports all that, but they're going to take those objectives and say, um, what are the automations and data that will allow people to work, maybe not 50 ops, maybe 60 ops. Can, you know, can I, can I take. An hour a day that people are spending searching through, um, contact databases and can I actually turn that into just a list of contacts that they can go work? So, so I think, um, I think actually all roles are present and I was asked this question at a, um, at a panel recently, like, what do you think the, the, you know, will AI sort of replace sales? And I think actually what will happen is that there will be more ops roles and fewer frontline sales roles. Yeah, that's super interesting. And right now, one of the areas where you see, uh, you know, a vocal sales influencer, someone that, uh, you probably cross paths with that dream force, you can't ignore Mark Benioff. He's talking about agents. He's talking about how that's going to disrupt sales. He's going after Microsoft. And co pilot and all this stuff. So there's obviously something there. How, how do you see agents sort of disrupting sales? Yeah. You know, I think what I haven't seen yet is like incredible. Agent to human interaction that is by those two way, even things like scheduling a meeting, surprisingly complex and nuanced. Um, and, and so what we have seen work well at Clay and what we focus on is kind of the first mile stuff. So, can the agent generate an email that is human quality and, um, and have that be sent off? And my answer to that question is emphatically yes. I, I actually think that a well, um, produced AI email is indistinguishable from a email that a person can send already today. And you can just imagine how that is going to the fidelity on those messages is going to increase. I think what, um, where this breaks down is when then the human responds and the agent responds. And I've seen a number of situations where it's just there's just an uncanny valley today with the technology. That people know immediately that they're talking to, talking to a robot. Um, and so, so basically, I think agent force can be divided into a few categories, and the category that I am really excited about is basically like, um, agents that help build sequences and automate, automate sort of like prospecting processes, and then research agents. Um, Claygents today can go out and find almost any piece of information about a person or a company that's available on the internet, deeply research, um, that, that piece of information. One of my favorite examples is like looking for mental health benefits for a company. Um, what in Clay, you'll see Claygents kind of like steps to obtain the information. And what you'll see is like. Let's say I want to find mental health benefits from Stripe. The agent is going to go to stripe. com. It's going to navigate through the website and find the careers page and benefits page and so forth. Then it's actually going to do Google searches and go to Glassdoor and levels. fyi and Indeed. And it's actually going to cross check the information from different internet sites. So that kind of functionality I consider quite advanced, and I think agents are amazing at that. And going back to my sort of original spiel on go to market data, It's not so important that the information is 100% accurate, 100% accurate. It can be 90%, maybe 85%. You're not gonna lose a customer typically because a research agent has, um, made some mistakes. Somewhere where you might lose a customer is if. interaction is happening where the completely wrong outcome occurs and it's, and there's an agent on the other end kind of being responsible for that. Um, I'll say one more thing on this, which is working in AI means that everything that you say is not quite working today may actually end up working in a year or three weeks, three weeks. So yeah, I think that it's important that, and I think I give a lot of credit to, um, our founders here at Clay. We, I think, are never trying to build ourselves into a corner where we're going to be completely upset by the fact that some previously unknown thing starts working. A good example of this would be, like, phone calls. I have heard a number of phone calls lately that an agent made that they have more or less solved the problem of responding quickly to humans. If you asked me six months ago if that was going to be solved so quickly, I would have said no. So I'm still prepared for the, um, The agent to human interaction thing to become quite seamless today, I would say it's, it's not the case. Yeah, absolutely. And things move incredibly fast with AI. I think that's the one thing that people have to understand is, yeah, everyone has some anecdote about, oh, you know, it's going to hallucinate in this scenario, or, you know, it breaks down in this scenario. And then by the time that paper is published, or that blog is out, or someone posts about on social media, you can test it again, and it's already fixed. There's several research papers where people were testing the You know, the, the, Oh, one model or, you know, uh, Claude or whatever. And they gave like very specific examples of where, you know, it doesn't work. And, you know, by the time you will actually start talking about it, you know, it's already evolved way past that. So that's one of the things that, you know, engineering teams in general just have to be aware of is that this thing, you know, AI is just moving incredibly fast and you do need to. work with, uh, products and teams that are able to quickly iterate and make that part of their, their offering. Yeah. I think one of the most interesting problems, um, is actually consistency with agents. So, you know, in clay, it's kind of this, the spreadsheet style tool where you, where you do the same operation across tens of thousands of rows. So actually it's quite challenging to get an agent to produce the same results. across, across 000 runs. Um, usually you will see some kind of variations on things. There's a lot of, there's a lot of things that help that structured outputs is a great innovation where the agent is kind of constrained to produce a more limited set of results. There's a lot of prompt engineering techniques to deal with this. Um, but, but still actually, uh, uh, using. Agents and and and AI tools for things like classification and segmentation and then and then Doing that consistently as part of some kind of data enrichment pipeline, um, is one of those areas where, um, where there's, I think, a lot of research actually happening. I've I've seen work on using agents to check the outputs of other agents. Um, the one of the best ways that we do this with. Um, that we prevent hallucinations with this in Clay is we ensure that the agent has internet access so it can kind of double check its sources. Um, and, um, but, but, but still, I think the, like, possibilities that have opened up for, um, for even just basic tasks, like creating custom industry segmentation are incredible. And then there's new challenges that happen when you start to really productionize, make some of that stuff work in production. One of those would be, like, Consistent outputs over many, many runs. Uh, that's a great point. The, the, the other part of this is, you know, let's say you do have, you know, 50 sales reps and rather than them going in manually doing research on their leads, right, they could deploy agents to do this work for them. And it just makes your existing reps so much more productive and work with better data. You know, the, the, but part of that is, yeah, the, the underlying products and tools they work with have to have good quality data. So what's the role of data teams that may own like the snoke Lake or big query the pipelines, you know, Databricks, you know, what have you, how do they work with go to market engineering teams? Yeah, it's, it's, yeah. It's super interesting because I was just in SF last week and I think Clay and CRM's are kind of a, you know, two peas in a pod. But actually most of my conversations with customers out there and prospects out there was not about the CRM, it was about the warehouse. So, Clay supports Snowflake today, we've added, um, we've added a lot of features to kind of, um, make that work well in the tool. We're also working very hard on BigQuery and Databricks, um, we're working hard on, like, ETL endpoints. Um, I've got customers that are actually using Clay. I've got one customer that is pushing data to Clay from a Lambda function and writing that data directly to S3 from within Clay. Thanks, Clay. So, so in this sense, the data engineering and, uh, data side of go to market is actually front and center. Um, and, and it's actually directly part of the, um, part of the. You know, main processing here. I think probably the biggest gap that I see is actually just a deep understanding of how data enrichment works and how it's measured. So this thing of match rate and fill rate as a basic example, you know, match rate is where you have a existing record about a contact or company and you have some data like you may only have one data point. You may just have employee count or revenue, or you may have a phone number, but not a work email. But you've matched. you've matched that lead or that company against your database. Fill rate is then within those matches, what data points do I have? So I might have a 90 percent match rate, but I might, and then I might have a 70 percent fill rate for employee count, which means that my, um, like across all of my data, I need to, you know, multiply 90 percent times 70 percent to understand how much of my data I have employee count for. So Uh, because data teams are are typically pretty involved in the evaluation of these data enrichment tools, it's important for them to understand kind of the theory of of data enrichment and and how it's measured and quantified because it's also quite easy for the right technical sales rep to sort of like fudge the results a little bit and to, and I think this is actually where teams end up with Data enrichment tools and stacks that they aren't happy with or that don't perform the way that they kind of thought they would because they probably had some gap in understanding of of the technical aspects of how data enrichment is measured and quantified. How these tests are run, things like what sample of data to provide to the data enrichment vendor. Should you provide a random sample? Should you provide data that only has not been enriched? Should you provide recent data or data from the past year? If you provide a random sample across your entire CRM, what does it mean if the vendor detects that someone has changed jobs? Should they then surface a new email and phone number for them? These types of details are actually super critical for for our data teams to. And it's probably the number one way they can support their sales and marketing functions because it really helps with situations where then reps kind of come back after the evaluation has been completed and the tool's been implemented and it's been in the field for three months. The worst nightmare of any go to market data team is the reps come back and they're like, actually, this is like worse than what we had before. Um, and so, and so by understanding. Uh, the, the, like, theory of data enrichment, and it's not that complicated, but there's some, there's some things. Data teams can be, uh, super helpful for their, their go to market orgs. Yeah, that's a great point, and it does seem like now with customer data platforms fusing with the data warehouse and with the CRM, you know, the lines are blurred a little bit, you know, from RevOps leadership owning the, the data that's used for things like enrichment and lead routing. Any of their, you know, intelligence sales processes to the data teams that are actually building the pipelines that are ingesting Either their internal product data, the external data from, uh, you know, third parties, and then, you know, data from the CRM. Because the warehouse is sort of the natural place to kind of collate and, you know, combine that data, model it, create a data product. For sales to work with. So, and I do see a lot of companies trying to figure out how to put structure around this. So what's your advice to teams doing that? Yeah. Um, a great example of this type of problem is what I call like TAM sourcing, or you could, you might call it market mapping. Basically, basically what companies are trying to do with this is they're trying to take the list of accounts that are in their CRM and, you know, customers, but also, uh, leads and they're trying to do, I always mildly screw this up. It's like, it's like a left outer join. With the overall, uh, database of companies that exist in that particular market segment. So like, let's say, um, you know, let's say you are, um, Vanta and you're selling to kind of like, um, growth stage, uh, mid market companies that are need, need compliance, uh, uh, offerings, largely software companies. And so you actually just kind of want to have an entire database of those companies. And then you want to have companies that you've never talked to, companies that are in your CRM, and companies that are customers. So, um, so the systems involved to create that are actually quite data warehouse intensive. It's basically a large analytical query. Where you're kind of excluding companies that are in your CRM, and then in Clay's case, you're including companies that are in our database with some filters applied. Um, and so, um, and so the way that you would ideally deliver that would not be through some kind of like UI where you're like searching for companies and you'd find like 50 companies in this segment and that segment and so forth. It's actually almost more of a SQL or, or like, um, search query style interface that allows you to kind of like do that. Do that join. Um, so, um, uh, But I think if you talk to most marketing and RevOps leaders and you describe this problem to them, they wouldn't probably describe it in that way. Um, and so, um, and so Data teams can really help their stakeholders by kind of saying like Look, actually the, the exercise of running this query is simple. If we have, um, If we have a well organized internal database of accounts and we have an external data source, which we can kind of compare against. Now, do you see a future where you can kind of unleash the, the sales AI agents on the warehouse to just find these insights, to find leads, to go do the joins themselves and come up with that, you know, that left outer join query? Yeah, yeah. And, and just figure that out and, you know, be both the data domain expert and the sales expert. Okay. Um, this is futuristic, but you know, I, I think, um, the agents seem very good at basically operating on a relatively smaller set of data right now. Um, I haven't seen a lot of instances where I would necessarily want the agent to be both writing and executing the query on the warehouse. Although I think that, um, People like get a lot of value out of having AIs write, write queries for them. I think, I think one area where, um, where the agents do work well is you kind of want to orchestrate. Individual agents on individual records. This is how clay operates. So I'm probably a little, a little biased here, but I want to really, it's working. I want to have, you know, I want to have not one agent, but like 30, 000 agents going out and doing research. Um, and I met, want to have another. Uh, 5, 000 agents checking the like low confidence results of those agents research. And so in that sense, what you kind of have is like, is like the opposite of a SQL query where you're operating over massive amounts of data. You actually have a sort of like function esque situation where you're asking, um, this, this, agency of agents or army of agents, or I think there's a interesting company called crew. ai, which has a lot of takes on the multi agent, um, setups, but you're asking this, this pantheon of agents to go out and do the work for you on an individual record basis. And you're not necessarily expecting the agents to kind of string together things across multiple records, if that makes sense. Yeah, it definitely makes sense. And you know, this is all, you know, a very new area and companies have to do a lot of testing in this process. And this is sort of something where an engineering team has the background to do that. Right. You know, not just go unleash a bunch of software. Right. to, to go do, go to market engineering. Yeah, there you go. Exactly. So. And, and, you know, I think traditionally, you know, like you said, you know, RevOps has a very, uh, important function, which is, you know, making sure that, you know, there's the territory alignment is correct. People are, uh, having the right compensations and quota objectives and things like that. And now that data and AI are becoming a core part of go to market efforts, you know, go to market engineering is the natural next step. Uh, to be part of the whole, you know, go to market and sales and marketing motion. So, and this is going to be interesting, right, because, you know, software engineering teams traditionally roll up into CTOs and, and then, you know, you, you'll have data teams at an enterprise level rolling up into CIOs and in some cases it's chief data officers. Uh, there's, you know, uh, CISOs now for, for information security and it's all sort of fusing together now. Uh, technology is not being used for a lot of go to market efforts. So seeing next generation products like clay being useful here, uh, is certainly exciting and something that both data teams and go to market teams really have to, uh, keep their eye on. Yeah. Yeah. Uh, the, I think go to market engineering should roll up into the CRO. Um, they're, they're kind of like an embedded team which leverages the information from those other parts of the organization. They leverage the, um, the sort of like clean and collated data from the, the CIO office. They leverage, uh, like product and event data from the CTO office. Um, but, but their end user and customer is definitely the, the CRO. And, um, I, I have a, I have a post on this. Uh, I have a, I have a post on lots of things, but I've met so many CROs recently who are quite technical. Uh, like they may not be technical in terms of, oh, they are going to go write Python scripts and stuff. But, but the way that you build a modern sales organization as certainly at a tech company is, is quite technical. Um, like, yes, you have kind of a theory of how you should sell and everything, but, but it comes down to basically a series of processes and playbooks and then data that informs whether those things are working or not, and a system of checks and balances that basically the CRO is trying to kind of operate. And then as things are working in one area or another area, the problem sets they work on are growing the business, or there'll be areas which just like fall out of line for whatever reason, and the CRO is then focused on fixing those. So, so the, um, technical, like partner or partner office for making those changes in a lot of cases is RevOps slash go to market engineering. Um, you know, because like, let's say you run a PLG business and for a long time, you've, you've sort of feasted on expansion. It's been super straightforward and suddenly like your expansion pipeline just dries up. And, and so you'll have a, some sort of dashboard in Salesforce, which will start to show that, but then going in and diagnosing that problem and fixing it. Um, it's, it's, you know, talking to sales reps and, and understanding things there. But, uh, but the fix for it in a lot of cases is like some more technical diligence and, and systematizing of things in order to, in order to, um, address that. And so, and so the, the best CROs that I've met are kind of like these debuggers in some ways. Um, and that's where I think actually go to market engineering is a perfect fit for their organizations because they're already in that mindset of like, Observing this complex system of people and customers and data and systems. And, um, and then when they see something wrong or they have an objective they're trying to achieve, they're really like leveraging the, uh, I think the technology in many cases, or the sort of like technology that supports the processes they manage in order to. Um, in order to, you know, to, to be effective and it's, it's kind of a, it's, it's honestly just the same theme of all of this, which is, I think just things are getting more technical. So, um, so, so yeah, CRO and go to market engineering feels like a natural fit from where I'm sitting. Definitely. If the go to market engineering team is mainly responsible for driving leads and revenue, it does make sense for them to roll up to the executive who owns revenue. And, you know, it's interesting because on one side of this whole AI, you know, pop culture, everyone's talking about, oh, AI is going to replace engineers. And then when it boots on the ground, it's like, oh, it turns out AI requires like more engineers. Yeah, yeah, yeah. Because they're going to use AI. And like, because I just think the practice of deploying, testing, Uh, scaling, any type of software process, you know, you do need that, that foundational background in, in, in software to, to really do it. Yeah. The, um, the thing I think about sometimes is just like a broad upscaling of, of people, you know, that the tasks that we're talking about automating are not tasks that I think many people would say that they enjoy or that, that they get a lot of personal development out of, you know, endlessly sourcing contacts. It's the same persona, the same set of titles, sending them the same messaging over and over again and, and, and jumping between seven different Chrome tabs in order to facilitate that process. You know, is that really like the limit of human potential, right? I actually think it's a better situation where, yeah, we're bringing in a lot of You know, complex new things. And we need in some cases, like you said, more people to, to facilitate all those, but then the jobs that people are doing are actually much, um, much more like challenging and I mean, creative is kind of the go, the going word at clay. Um, and that's a good thing. And I think companies would rather employ. Um, creative, motivated people that are challenged and, and what they're doing than, than drones, or at least, at least that's what I, what I hope for may not, may not be true everywhere, but I think that is like a positive vision for go to market teams as they're starting to leverage all this stuff. Absolutely. Yeah. It's certainly exciting time. Both data teams and go to market teams can. Really get a lot of value from AI and agents and all the things going on. Uh, Everett Barry, head of data engineering. I'm sorry. Everett Barry, head of go to market engineering here at Clay. Thanks so much for having me here and glad we were able to do this episode of what's new in data. The other thing, you know, that's a fun update since the last time we did an episode is you're a dad now. Yes, I'm a dad. Yes. Congratulations. Yes. I'm bringing the dad vibes to, uh, to Clay's office, which has been fantastic, But Yeah, I 11 month old daughter. She's great She now is like just stuffing food in her face every morning. Oh, and so yeah, she's the best and and it's it's been actually amazing to be at clay while this has been happening because Uh, there's a lot of other dads and moms here and, um, and it's, it's been a really supportive environment for that. So, uh, so yes, my, um, my, my journey into adulthood is kind of finally fully complete. I'd say. Yeah. Now, now you're unleashing AI on go to market teams and engineering and super exciting times all around and congratulations. Thanks to everyone for doing this episode today. Super fun stuff to talk about. Always love catching up with you. And thank you to the audience for tuning in. Thanks, John. I'm You