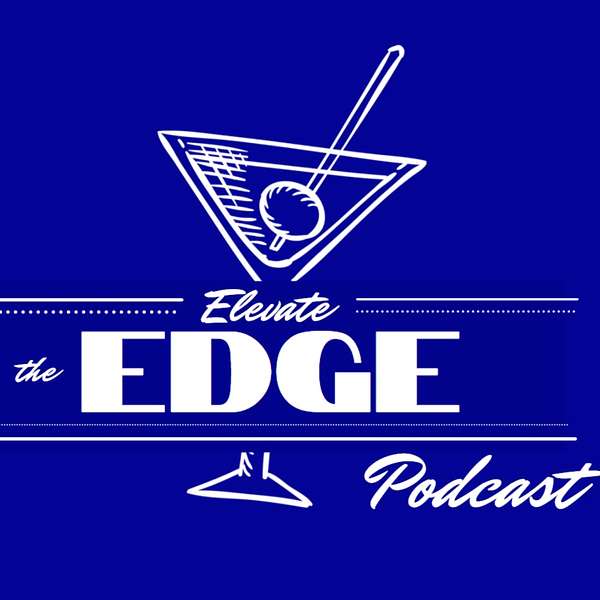
Elevate the Edge
Elevate the Edge
Edge AI & Distributed Application Management
Elevate the Edge hosts Jo and Maribel discuss Edge AI and Distributed App Management with Roshni Bhagalia, Vice President of Product Management — Editon AI & Patflorm for GE Healthcare.
Hello and welcome back to the podcast. I'm Maribel Lopez and I'm joined here today by my co host Jo Peterson, and this is elevate the edge. As always, we're trying to bring you new and interesting individuals to speak with. And today we're here with Roshni Bhagalia. She's the Vice President of Product Management for Edison AI and platform at GE Healthcare. Edison is GE Healthcare is intelligence platform designed to help the healthcare industry, improve efficiency, improve patient outcomes, and increase access to care. The one of the things that's interesting about it is it's, it tries to integrate with the other applications and assimilate data from disparate sources and apply analytics and dance algorithms to that to help provide insights. So some of the things we love about the edge are the ability to take lots of data from lots of different places and create new and interesting insights. So this sounds like a conversation that I'm very excited to have. Roshni, who is a experience technologist and product manager, and rashly and her team create infrastructure and services that power the next generation of GE Healthcare software applications. So with that, I'd like to welcome you to the program. Roshni, how are you today?
Roshni Bhagalia:Thank you Maribel. Thank you so much for having me. Yeah, I'm doing very well. I'm very excited to be on our way badge. And I'm looking forward to our discussion today.
Maribel Lopez:Well, today we're going to talk about edge AI and distributed application management. And in the past, you know, Joe and I have had several, many conversations actually, where we've discussed the many definitions of the edge, we've looked at several verticals, agriculture, retail, airline transportation, as matter of fact, today, we were just talking to someone in the seaweed industry. So the edge is everywhere, and all kinds of interesting spaces. We've done water, utility management, smart cities, but healthcare is clearly a place where we see tremendous opportunity for the edge. But perhaps we can start with the basics. From your perspective, what is edge AI in healthcare and how does it work?
Roshni Bhagalia:Yeah, so you know, the use of advanced technology, especially in AI, especially AI in healthcare, has the ability to deeply and directly improve patient care and outcomes. All of this is provided it can be harnessed in a clinical clinically viable manner. So for AI, this often means that we need close to real time performance with high clinical accuracy and reliability. And this is what can really be achieved by running AI at the edge. So you know, in sort of, it's it's in the terms, right? Edge AI is literally deploying at the edge. And in healthcare, the edge implies running AI on premises, closer to the data sources. And these data sources are offering medical devices or medical data aggregators. In several of, you know, the examples that we have in healthcare, AI is either embedded in the medical device itself, or sits in close proximity to it. And the big advantage of doing that is that you can act on that AI and get feedback from that AI, while both the patient and the clinician are still in the loop to act on the findings. And you know, with the recent advances of optimization, acceleration, and really compression of data models, it has now actually become feasible to do some very intricate and compute intensive tasks at the edge and closer to the devices where where they are more accessible to both clinicians and patients.
Jo Peterson:Roshni, you mentioned, clinically viable data, and the ability to keep the provider and the patient in the loop at the same time. Help the audience think about a couple use cases. And I know we're gonna get into use cases a little bit later. But help them frame that up, give me an example of somewhere where the data can come in, thanks to the work you're doing and the doctors there and the patients there and a decision can be made.
Roshni Bhagalia:Yes. So you know, a very, very simple and probably commonplace example is when you go to the doctor for either a preventive checkup, or a scan, you know, either either it's preventive, or it's acute or it's chronic, but essentially you think of going to a medical facility to get a scan of the body don't in in many scenarios, you get the scan done and then you go home, and then somebody else reads an X on it. Now while that works when it's not a latency intensive operation, you know, maybe it's just something you have minor leg pain or something and you're fine waiting for it. In, in many situations, it's an acute situation, meaning you are probably actually having a stroke, or you're probably actually having cardiac arrest. And in such situations, if you can get AI assisted feedback back to the clinician, before you actually leave the premises, it can actually impact your quality of care. So either, you know, there's a follow up scan that can help improve the diagnosis, or there is an urgent medical intervention that's needed, which is informed by AI acting on that imaging data in a timely fashion, before the patient leaves and sort of, you know, is lost to the system for a while. So those are sort of, you know, very simplistic and very general, widespread use cases where AI could really help.
Jo Peterson:Oh, that's, that's a great example, because that's literally life saving, or it could be, right. Absolutely. Yeah.
Maribel Lopez:Yeah, I think this really actually tees up some of the interesting differences in how we think about edge strategies as well, because some ad strategies have to take the data and analyze it in real time. And other ad strategies, you know, we can collect the data, do batch analytics at a different time, it's just a different level of intensity around insight. And not all, not all use cases are created equal. And so one of the things I wanted to ask you about is there, there are many ways to think about deploying AI models at the edge. But what are a few of the recent innovations that might be making this easier at the edge?
Roshni Bhagalia:You Yeah, so there are, you know, there are a number of innovations that have made AGI more accessible. And actually, the last, I'm gonna say five years have been particularly impactful. But I'm just going to call out a few here. So the first one, and probably the most obvious one is the advent of AI model optimization, acceleration and compression techniques. So things that you know, needed a lot of compute, either to run or even just to store the models have now some of those restrictions have gone away. And many of these techniques allow us to really compress the model, accelerate and run it with virtually no negative impact on performance. So that's, I think, the first really obvious one. But in parallel with that, we've also seen a really strong ramp up in the availability of cheap, faster, and in many cases, specialized compute with very low power consumption. And what this does is, you can now think of, you know, moving that large compute power, which used to be relegated to maybe the data centers, or the cloud providers, to really deployment at scale, especially because it can get to that lower power consumption, while still giving that specialized compute. The other two things that have really helped in sort of driving this field forward make it making it more accessible locally, is the growth of open source tools. And you know, it might seem tangential to VI. But really, the ability to manage and scale with cloud native technologies, like Kubernetes at the edge means that you can now deploy these workloads in a very seamless fashion, in a sort of distributed framework locally for both connected and disconnected scenarios. And then the last bit, you know, I really want to emphasize is, again, open source tools, but this time, specific to cross compute optimization. And so what I mean by that is, there's been a growth in tools and API's that allow us to build code that is portable, across multiple compute chipsets with almost minimal to zero rework. And so what this means is you can develop something using that sort of open source tool or API for one compute framework. But now you can very quickly and easily run it across disparate compute across many local sites. And that can be really powerful in sort of a edge based setting where you're no longer relying on a centralized cloud, or a central centralized set of resources to run your workloads.
Jo Peterson:And I love what you said cross compute optimization. You heard it here, folks. Roshni, you know, she she came up with a term that's that other folks are going to use. I know that. And you know, with that in mind, and how I'm thinking about it, and I'd love to get your insight here is, is it fair to say that edge computing is sort of a middleware for distributed We'd like a distributed application driven process. Can we think of it that way?
Roshni Bhagalia:Absolutely. Absolutely. So, you know, I and I think you alluded to this earlier, you're like, there are a number of scenarios where where data flow between cloud and on premise makes sense. You know, and there are some some straightforward examples where sort of you're doing offline computer delayed processing. So you have algorithms that assist, you know, the post scan radiologist, if you go back to our first example, where it's totally fine, to get the scan done, you come back the next day or three days later, and it's okay to get that delayed diagnosis. There are other situations where you can do longitudinal, population based studies on archival, or archive data. But when there is a need for real time performance in real time, compute where you know, in our example, it is an acute incident. And actually, the biggest bang for the buck, the biggest benefit is getting that feedback at the right point of time. In the care continuum, when both patient and provider can act on it, then you really need to make sure that you're not limited to network connectivity, you're not limited to throughput, you're not limited to bandwidth. And the only way that that's possible is by using edge compute as middleware to create those clinically meaningful solutions, and to make them very effective. And you know, I'll go back again to the plug I made about Kubernetes. Really, the having the ability to leverage those cloud native technologies and open source tools means that this middleware layer can now scale. And not only can it scale, if it's built, right, you can even manage it remotely, even in cases where there are intermittent connectivity. And you can possibly deploy it again with sort of that cross compute layer on top of it across various different workloads, right with zero rework. And that has this multiplier effect in the middleware, which really creates this continuum, closer to where the patient and the provider are.
Maribel Lopez:I think there's, there's so much in there in terms of the ability to not think of locking yourself in a technical Island. And I think that was one of the issues that we had with the early the early editions of AI, you know, people really being invested in a specific set of frameworks, the early days of cloud, you were investing in a specific set of framework, and there was no concepts of real real concepts of portability. And I also love some of the things that are happening in open source in terms of helping tools to help people understand and unpack some very sophisticated models from an explainability standpoint, which I could see might be very interesting in the healthcare field, where if a model comes up with a recommendation, you'd want to be able to explain why it had that recommendation, and what some of the data sources or other things were that led to that. So I think there's a lot of power in terms of both the open source tools, but also in terms of thinking about using multiple types of artificial intelligence, chipsets and stacks at the edge, the right tool for the right job and creating those right time experiences that you mentioned the right information, right person, right time, I think that's the, that's the wave of the future, what we're trying to get at with edge computing. So we've talked quite a bit about some of the innovations and the opportunity, but I thought maybe you could give us a few more examples of edge AI use cases that you're looking at?
Roshni Bhagalia:Yes, absolutely. You know, the healthcare industry in particular has seen a very strong growth in the use of AI and machine learning. In improving care. For example, the FDA recently published an updated list with about 187, new AI and ML enabled medical devices. I am going to make a quick plug for GE here because, you know, we were we were very pleased that GE sort of led that list with 42 Clear devices. And it just goes to show we have a very strong emphasis on on leveraging AI to really improve care across all our product lines, we really sort of believe that it can have a very strong impact on healthcare, but a couple of really concrete and sort of specific examples where where AI has improved things is first in in sort of the acute care situation with which is very prescient. So, you know, during the pandemic, there needed to be a very rapid scale up in skilled personnel dealing with pandemic related um, chronic and acute conditions, and GE and other meta companies were able to very quickly develop and deploy AI assisted clinical care, which eventually saved patients lives and really allowed people to improve bedside care at scale. A particular example is something that we call the critical care suite that's deployed on some of the GS X ray devices. And it essentially helps sort of placement and treatment plans for people who have pulmonary issues, and many of them linked directly to the pandemic. And this was particularly impactful in sort of underserved areas and in areas where we couldn't have the right set of resources or skills available simply because just the number of people that that we had to deal with. And so this AI assistance was was really impactful. I do want to mention, and emphasize something that that Maribel said early on, you know, explainability of AI is extremely important to healthcare, right, and so transparent, and Explainable AI is really valuable, but equally valuable, is, you know, clinicians want AI to be an invisible friend. And what I mean by that is, they don't really want to do anything differently. They need that AI assistance at the right time, and they wanted to just show up as friendly advice for them to either take or to ignore. And, you know, with with the critical care suite, GE and other vendors who came up with similar solutions with the pandemic, I think we were able to really do that, in the case of pulmonary care, and really move the needle there. So that's one example. Another example, in the not so acute care situation is, for decades now healthcare has has, you know, struggled with inverse problems, especially in medical imaging, where historically you have huge compute intensive tasks that historically used to require supercomputers, very high latencies, you sort of had to send it off, and it took minutes and hours to process. And it looked really good in a lab, but it made it clinically non viable. And one of the big benefits of, you know, if we can, if we could have gotten that to be to be locally deployed, is that you can now start reducing dose in things like CT scans, without affecting image quality, right. So really think about that. So you could now you know, reduce the radiation, that you are impacting people to especially, you know, maybe compromised people or younger people without impacting the diagnostic quality of the scan. So with the advent of AI, and you know, some of the advances we talked about earlier, we are now able to run deep learning based reconstruction algorithms, either in the device or very close to the device, and now makes them clinically viable. So you can actually, you know, lower the dose, lower the noise in the scans, and really get to that higher image quality, which really has an impact on on diagnostic value. And then the last bit I'll add is, because I think it's quite unique to healthcare, you know, healthcare, unlike almost any other industry has the very sort of disparate IT infrastructure. So you go all the way from community hospitals with very limited access to the internet or to it to sort of big ideas with Global Access and cloud like access. And being able to give the same quality of care in both those instances is only available when you have local edge compute. Because you can now take something that you could deploy in the cloud for a premier site and make it available to somebody locally because you can use all the three or four advances, especially blended together that we talked about earlier, and really get it closer to the point of care. So I know I may have rambled on a little bit there. But, you know, there's there's some really strong use cases for AGI and healthcare
Maribel Lopez:now, and I think the part that you brought up about the infrastructure as well is so important and so powerful the changes in the availability that we have today that we couldn't have done, say, three, five years ago. When I think of AI in general, for most organizations, whether they're in healthcare or not five years ago, it was much more challenging to do anything than it is today because of the availability of the tools just wasn't there. And now I think we're all the technology landscapes, making a concerted effort to make things more accessible for a broader range of skill sets to make things more affordable and to make it available in more places. So it can be on prem or in the cloud, your hybrid deployment choice. This room really quite good these days. But I love that fact that you brought out the real true challenges that we see in healthcare, which is around. Not every healthcare organization has had the same access to technology in the past and how do we level that playing field so that we can get equal care no matter where individual lives over to you, Jeff?
Jo Peterson:Well, I get the I get the fun thing to do, Roshni, of asking you a fun fact. So it doesn't have to be healthcare or tech related. But can you share a fun fact?
Roshni Bhagalia:Um, I think so. I'm a geek, so I might, it might be slightly tech related. A fun fact is the world's first reported computer software bug was actually a bug. So on nine September 1947, a team of computer scientists at Harvard University reported that one of the reasons their computer system was malfunctioning was because they had found the moth trapped inside their computer.
Maribel Lopez:Mobs destroying things since the dawn of time, including computing.
Jo Peterson:That is great. Well, you have been a pleasure to have with us today. Roshni, we loved your insights. We love what you guys are doing to help make health care more accessible for folks. And thank you so much for taking your time to visit.
Roshni Bhagalia:Likewise, and thank you very much for having me. I listened to some of your previous podcasts and it's a delightful series. So I'm looking forward to tuning in more often. And Happy Thanksgiving to you.
Jo Peterson:Happy Thanksgiving to you as well.
Maribel Lopez:Happy Thanksgiving to all the people here this after Thanksgiving. We hope that you have a joyous holiday season when ever the holidays happen for you. Take care everybody. Take care. Bye