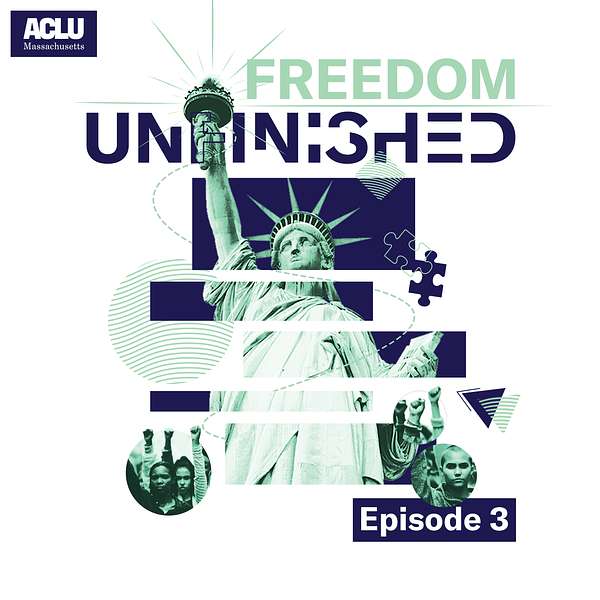
Freedom Unfinished
Freedom Unfinished
E3: Algorithmic Injustice
We've learned about the pressing nature of data control and the threats it poses — both in the private (where it's collected) and public (where it's enforced) sectors. Exactly how are the systems in place driving the decision-making in response to all of this data? Algorithms.
Listen to ACLUM executive director Carol Rose and Technology for Liberty program director Kade Crockford explore big data and artificial intelligence through the lens of power, democracy and the broken systems that will determine the future of our rights.
Join us this season wherever you get your podcasts and follow the ACLU of Massachusetts on social media @ACLU_Mass for the latest updates on Freedom Unfinished, Season 1: Decoding Oppression.
Thank you to Trent Toner and the Rian/Hunter Production team for sound mixing this episode.
Kade Crockford (00:04):
I wanna start this episode with a quick reflection on what we've talked about so far. We've interviewed lawyers, legislators, journalists, researchers, people who understand the impacts of the data economy on our communities, and they touched on actions we can be taking to protect ourselves. But some of these issues run a lot deeper than all of that. That's because technology isn't distinct from the human beings or societies that create technology. Technology is not an autonomous thing, it is not neutral. It doesn't exist in a vacuum according to some unbiased logic separate from the motivations of human beings or corporations or governments. At a very basic level, when we reflect on today's technologies, we have to ask why certain technologies are developed instead of others. And we will find when we ask that question that we can't separate these decisions from the dominant power structures that organize our society and pick winners and losers. Now, capitalism obviously plays a huge role here. Just imagine how different our internet and our technology ecosystem would look in a society that privilege, say human welfare, over profit maximization technology is tools, but it's tools whose purposes are defined by those who create and wield them.
Kade Crockford (01:36):
We can't separate our technology from the society in which it's created, and in addition to being capitalist, our society is afflicted by a history of racism, misogyny, and white supremacy. And all of that is reflected in the persistent structural inequality that dates back hundreds of years that we face today.
Kade Crockford (01:58):
As we'll see later in this episode from our smartphones to video games to surveillance technologies, the design and development of even seemingly innocuous technology, in addition to the more immediately pernicious examples we've talked about, to often reflect our inequalities and perpetuate problematic social and historical inequities, rather than challenge or undermine them, by no means has the United States closed the book on racism. So when we refer to white supremacy, we're talking about deeply ingrained institutional disenfranchisement and oppression of people of color. In the United States, white supremacy is redlining, it's mass incarceration, attacks on voting rights and the systematic exclusion of particularly black and native people from economic opportunity in the United States. Some people have described this as a cast system, but whatever you wanna call it, it's real and it did not end when the civil rights movement won important political victories in the 1960s.
Carol Rose (03:03):
I'm Carol Rose, executive director of the A C L U of Massachusetts, and in this episode I'm handing it over to Cade to investigate how our country's history with white supremacy impacts today's technologies.
Kade Crockford (03:15):
Thanks, Carol. This is c Crawford. And to everyone else, welcome to episode three of Freedom Unfinished Algorithmic Injustice.
Kade Crockford (03:35):
William Faulkner said, History isn't dead. It's not even really passed. Nowhere is that more true than in the world of machine learning where computers are taught to predict the future based on the past. To understand algorithmic bias, it's important to know what's going on behind the scenes at the companies that build the systems that we're talking about. And there's no real easy way to do that since these systems are so complex and proprietary and the companies are so large and frequently secretive, But periodically we do get a window into these systems and companies typically when something goes terribly wrong on the inside. For example, a few years ago and only after being pressured to think about the social and environmental impact of their artificial intelligence work, Google installed an internal team to review ethics at the company. Entered Dr. Timnit Gebru, a renowned computer scientist who was hired to co-lead Google's ethical AI team.
Kade Crockford (04:35):
Dr. Gebru had previously worked with friend of the ACLU, Dr. Joy Buolamwini on research showing facial recognition algorithms can exhibit very troubling racial and gender bias. And she continued that kind of work at Google. And after attempting to publish a paper about the risks associated with Google's use of large language models, the company forced her out. It's disappointing, but not exactly surprising when you hear stories like this. And the incident changed more than Dr. Gebru's life, a really tangible rift that had been building inside companies burst forth into the public like a dam breaking.
Kade Crockford (05:14):
People started to speak up about how troubling it was to have most of the work in AI being done by these massive companies, and they're well funded, secretive startup counterparts. Others flagged that the companies ties to law enforcement and state surveillance programs threatened racial justice and civil rights. For some, it was hard to take seriously any company's pledge to value human beings and basic rights when those very same companies seek to maximize profit seemingly at the expense of all of those other values. Out of this rift, Dr. GI built the distributed AI Research Institute or dare to independently research and document the harm that AI is doing to our collective rights, particularly those of already marginalized groups.
Alex Hanna (06:04):
We can think of a more of sort of a technological or data colonialism, and these aren't terms I've I've come up with, but others have used as reinforcing English dominance.
Kade Crockford (06:14):
That's Alex Hanna, the director of research at dare, where they focus on community driven research rather than data collection buying for the richest and most powerful corporations,
Alex Hanna (06:25):
Most of the big tech companies focus either on making their money via advertising and collecting of user data or capitalizing on buying hardware, right? Or if you're thinking about someplace like Microsoft tends to be much more of a traditional enterprise company. But the big emergent ones within the past 20 years or even the past 10 years have been very data hungry organizations, be that Google, Facebook, and also Baidu and Alibaba. They're very much oriented around collecting huge amounts of user data and being able to build prediction models based on that. But the idea being that you are collecting so much data on people, you know what they're looking at online, who they're talking to. So we can think a lot about how our civil rights are being impinged upon. This is pretty well documented if you're thinking about the harms that come from collecting on huge amounts of data, you're already thinking about people who are concerned with civil rights are Alarm Bell should be flashing because the way the model that is looked like before has been one of extraction has been one of really taking things from people in communities and groups without allowing them to reap in the huge profits and windfalls that come from collecting and using personal data.
Kade Crockford (07:52):
Alex had been on Dr. Gibb's ethical AI team at Google, but her background, which combines computer and social sciences, gives her unique perspective on big tech and data capitalism. It's sort of a cliche in the AI ethics world that machine learning is a great technology if you wanna make the future look like the past, Alex can help us understand how this is true.
Alex Hanna (08:14):
So as a sociologist, I start from thinking not just about the technology, I think about what kinds of things technology can enable and what types of things it can disrupt. And what we've seen a lot of is that technology seems to enable inequality and persistence of inequality. Inequality is very durable because of the structures and the institutions that support it. There is what is called kind of a Matthew effect that happens with institutions. The rich get richer than the poor port, but what does it mean? What is a way that necessarily that could be disrupted?
Kade Crockford (08:52):
Disruption is such a common theme in tech marketing, but when we see this so-called disruption playing out, most of the time it perpetuates the cast like system of privilege and white supremacy. So true disruption, if that's the actual goal, would actually be an approach that upends the status quo of inequality. And what do we lose as a society when a small group of companies makes decisions based on their unspoken biases,
Alex Hanna (09:21):
People will often point and say, Well, these tech companies are hiring many people from Asia and from South Asia, which is true, but at the same time, many of these people are very high cast. And there was this long form article and Wired that was a really fascinating interview with an activist who went nameless in the article talking about the cast discrimination that happens in Silicon Valley. I wrote an article when I quit, called on racialized organizations in complaint, a goodbye to Google, and I called out the white supremacy that really suffuses tech organizations, and I had a number of people respond on Twitter saying to me, What about Sun Pacha? He's the president of Google and the head of Microsoft is also an Indian man. I said, Yeah, true. At the same time, you're not acknowledging the way in which cast privilege works and much of the time, the way that cast privilege also reinforces white supremacy,
Kade Crockford (10:20):
Racist and white supremacist outcomes often manifest themselves unintentionally, even assuming that these business models are well intentioned, which I think is a stretch. We ignore implicit biases at our apparel, for example, the idea of amplifying our ability to connect with one another seems empowering, but in practice it doesn't always play out that way.
Alex Hanna (10:42):
If you've heard of people like Marcus Zuckerberg talk, they say, We just want to connect people and have the most fully connected network that we can. There's a kind of hyper connectedness ideology that many of the platform owners seem to think is the best kind of thing. It's this odd mix of libertarianism, but also hyper connectivity. And that is a unmitigated common good. But of course that's not an unmitigated common good. It doesn't pay to be as connected as possible as anyone who is a woman or black or queer on the internet knows it doesn't actually pay to be exposed all times. Being visible is really not the thing you want to do or be. There's a certain amount of visibility that lends people to harassment dosing. And so starting from a position of values that really thinking about what does it actually mean to be connected here? How can we think about a way in which a technology actually does support individuals and human flourishing? What does it actually mean to have community control over things? And there's kinds of frameworks of thinking through that.
Kade Crockford (11:53):
So recognizing how these biases can be amplified by hyper connectivity enables us to understand what impact they have on society. And while this can feel disheartening, organizations like dare aim to take that understanding and use it to shape an approach to artificial intelligence that accounts for the lived experiences of a diverse user base, the actual user base. Ultimately, this is a matter of approach, intention and mindset that for profit companies simply don't bring and can in fact be quite hostile to. And because these companies won't regulate themselves, nonprofits like dare are picking up the slack for a more ethical vision of technology.
Alex Hanna (12:39):
So I think that kind of experimenting, that kind of openness, thinking of what kind of framework it would be starting with people starting with their demands, their desires, their needs, I mean, I think that's what it's going to look like for a future that really is where to inclusive technology, inclusive AI is built.
Kade Crockford (13:02):
Talking to Alex like that, the solution to algorithmic bias seems so logical. Regulate for profit tech no matter what. It's purported intent, build more inclusive systems starting with people's needs and desires and not imposing a grand vision from above. The resulting world is fairer and more equitable with technology that works for more of us. That makes sense, right? So why is it so hard to make these changes? Well, probably the central reason is that the companies that have designed our technology systems this way find them enormously profitable. The status quo works for the rich guys, but it's also because our lives are complicated and technology we are told will simplify and streamline things often creates more complexity and we can see it right in front of us. But it's hard to consistently acknowledge the impacts of these things outside of how they impact us in discrete kind of micro ways.
Kade Crockford (14:01):
And it's even harder to take the impacts of algorithmic bias on marginalized communities and connect them to their root causes because so much of this is often hidden below the surface. It goes without saying that people are creatures of habit, particularly in the face of situations that seem outside their control or purview. But this is the value of fresh perspective of people and approaches that aren't stuck inside a single way of thinking. The ability to recognize a problem and say, Hey, I can do something about that. And it's something that at the ACLU we take very, very seriously. Our next guest, Dr. Crystal Grant technology fellow with the ACLU'S Speech Privacy and Technology Project brings that kind of energy and perspective to her work in part because she approaches it from so many different angles.
Crystal Grant (14:54):
So at the end of my PhD as I was writing my dissertation and I kind of treated it like this stream of consciousness, what have I learned in these last five years and ended up doing a section about bias in bioinformatics and in genetics and how a lot of genetic databases are primarily people of European descent and how this was leading to tools that work really well in European populations and do not work as well outside of them. And so yeah, I was just kind of talking about this bias and how it was unique to bioinformatics. So I thought only exist in bioinformatics and all these things that we need to do to try to counter it. And after I finished my PhD, I did a short tech policy fellowship dipping my toe into tech policy at the National Academy of Sciences and just found that I really liked it. And while I was there, I learned about this other opportunity for this fellowship, this nonprofit called Tech Congress, where their goal is to put technologists in Congress to kind of help them use their skills to mold policy.
Kade Crockford (15:53):
And that willingness to attack problems and approach solutions with a diverse set of skills and interests can lead down paths that others may not have even considered.
Crystal Grant (16:03):
It actually kind of all goes back to scrolling through Twitter one day.
Crystal Grant (16:09):
It was this talk of this woman who is a medical student and was talking about this calculator, this very simple algorithm used in kidney treatments and how it seemed kind of blatantly racist to her where there was this measure essentially of kidney function, but if your patient is black and only if they're black, you're supposed to adjust it to indicate that their kidney function is actually better than it appears. And she was explaining that it kind of all went back to this study that claimed that black people, because they are more muscular, actually have better kidney health than tests say and don't trust the tests. And yeah, it just started me digging into this history of clinical algorithms in medicine. The
Kade Crockford (16:48):
Healthcare system is something every single one of us has to interact with at some point in our lives. A system we look towards during our most vulnerable life moments. We look to professionals to take care of our basic human needs when we can no longer take care of them ourselves. Obviously we hope and expect that our race, class and sexual orientation won't play any factor in determining the quality of the care that we receive, but unfortunately that's simply not the case. And recent research shows some of these historic inequities in healthcare access and quality are actually being magnified through the use of new medical technologies involving artificial intelligence. One of the most famous examples of medical device bias became extremely relevant during the pandemic. Research has shown that pulse omes don't work as well on people with darker skin. And during the pandemic, this research was extensively cited in the mainstream press, but researchers have actually known about this problem since at least 1990 when the first academic article was published, raising concerns about pulse xometry's high rate of failure with black patients alarmingly this was still a problem in 2020 and 2021 as doctors were recommending pulse XOs to covid patients as a way of tracking the progression of their disease at home.
Kade Crockford (18:08):
Another example is the use of an algorithm widely used in hospitals to determine which patients should get extra care. This algorithm excluded race as a data input, but nonetheless still resulted in biased outcomes recommending that black patients had to be much sicker in order to receive the same level of care as white patients. Since joining the A C L U, among other things, Crystal has been researching bias in medical artificial intelligence algorithms and medical devices.
Crystal Grant (18:38):
It just comes down to should your innate ID or chosen identities affect your medical treatment? Should your health and medical care be based on the actual symptoms you present and the actual results of your medical tests, or should it be based on these other kind of sociological constructions in the case where those constructions maybe change your care for the worse? And it seems like this pattern has emerged throughout history of especially communities of color, black, indigenous, Latino communities kind of consistently getting worse care. And if that exists in our society, even before we start adding AI and other decision making tools into the mix, it will also be encoded in those tools and it'll become automatic. But
Kade Crockford (19:29):
AI can also be used to address existing disparities in the healthcare system.
Crystal Grant (19:35):
We know that doctors are human and doctors have biases and for example, how many women have spoken about their going in and talking to a doctor and talking about their pain and the doctor being like, Oh, it's fine. She's just being dramatic. If there was a popup when the doctor is going to dismiss this person who said they're in pain, it's like, Hey, just a reminder. A lot of doctors have biases against women. I think just reminding people sometimes of their biases can help them kind of take the blinders off a little bit.
Kade Crockford (20:02):
So what do we do to ensure that the civil liberties of black and brown people are being protected from not only big data and big tech, but from our healthcare system as well? Crystal thinks the department that controls medical AI systems could and should make a huge impact.
Crystal Grant (20:18):
It seems as though the fda, the regulatory body that is in charge of this medical AI has been putting out different frameworks around how to adjust the fact that these tools are able to distinguish things that trained radiologists for example, can't, which is a great step in the right direction. I think it needs to become sort of a required part of the approval or clearance of these tools to just assess can they distinguish these sociological buckets that we as humanity have put ourselves in? And if so, is it showing any kind of different treatment for different people in these groups Right now, this is guidance offered by the FDA to people who manufacture these tools. It is not a requirement and that is just a fundamental change that needs to happen. Crystal
Kade Crockford (21:06):
Also believes that certain groups within the medical field can make big shifts in the future of healthcare, addressing the prejudice that exist within the field currently thanks to outdated studies and test assessments as well as the new AI systems being used to treat people in their time of need.
Crystal Grant (21:22):
And also, I've just been really impressed by advocacy from medical students. A lot of times it's young medical students on their front lines who are the ones saying, Why are we using race and predictions of who will have adverse outcomes when in giving childbirth? Instead of using that more relevant information, do they have insurance? Why are we using race to change the outcome of a medical test? Just because the patient is black and kidney health and they are the ones that have really pushed their individual universities and health systems to change and to move towards more race concerts instead of race based medicine. And it's been really incredible like to see over the last couple of years, more and more of these race based tools now moving towards inclusion of more relevant like biological information instead of just using race as a shorthand. And a lot of that is because of the advocacy of medical students. I think that they have this tremendous amount of power that they maybe don't even realize that they have to change medicine for the better.
Carol Rose (22:24):
Hearing bbout the role that medical students play in fighting systemic racism is really inspiring. It's a great reminder that all of us have a role to play in getting this right in medicine and in data science. It's really easy for those of us who are not scientists to assume that science is by definition always accurate or correct, but in fact, bias pervades everything we do, including how we deploy math and science. And it's easy to assume that science has presented to patients in medical systems is rooted in fact when often we simply don't see the bias at the root level unless someone calls attention to the impact that the entire system is having on people and communities because the people who are impacted generally are not the ones making the decisions about how these systems function.
Sandra Susan Smith (23:14):
On some level. It's not surprising then that you would find both that black people and Latinx people are more likely to have future criminal justice system involvement if they live in communities where the level of police presence, it's much higher where their stop and frisk practices are in place. They're embedded in environments where those encounters are much more likely. And so you almost by definition create the outcome that you're looking for. And to the extent that the data that are used to determine what factors are important are relying on institutions that engaged in historical practices of discrimination. What you're getting as output is the result of that history of discrimination and bias.
Carol Rose (23:57):
That's Sandra, Susan Smith, the Daniel and Florence Guggenheim, Professor of Criminal Justice and faculty director of the program in criminal Justice Policy and management at the Harvard Kennedy School, as well as the director of the Malcolm Wiener Center for Social Policy. Dr. Smith's work focuses on recognizing the impact of racial bias in criminal policy and repairing the broken systems that perpetuate those outcomes
Sandra Susan Smith (24:23):
Over policing of black and brown communities, especially those that are low income increases the likelihood that those young people come into contact with law enforcement, even if they're not doing anything, but it also increases them the likelihood that they will be arrested if law enforcement was not such a presence in those communities, especially in a way that kind of over policed them but underprotected them. It strikes me that we would not likely see such gaps in terms of the ages of first arrest. So the system itself is helping to bring about the fact that they are arrested at earlier ages than our white folks. There are a series of other kinds of factors like that that play into how it is we assess risk that seem like it's about the individual.
Carol Rose (25:06):
Well, as Mark Twain once said, there are lies, Dan lies and statistics, and he had a point. The use of algorithms in the criminal system shows how unexamined reliance on data science can be misplaced. Too often, systems that are built on black box algorithms perpetuate and even exacerbate racial and other disparities in the criminal system. For example, a growing number of jurisdictions across the United States are implementing pre-trial risk assessment instruments that use data in an attempt to forecast the likelihood that a person will show up for trial or pose a risk to public safety. These so-called algorithmic risk assessment instruments are being used not only to determine pretrial release and bail determinations, but also for sentencing and parole supervision. Often these tools are presented as equitable alternatives to current systems of secure money bail in pretrial detention. But often automated predictions based on data are described as objective and neutral, when in fact they too often reflect and even magnify racial biases in the data itself. And they're potentially even more dangerous in the criminal system where people's fundamental liberty is at stake because they provide a misleading and deserved imprimatur of impartiality for institutions that desperately need fundamental change.
Sandra Susan Smith (26:26):
So in the earlier years of pretrial assessment tools, I don't think that the folks who were developing these algorithms did a good job of correcting for the kind of racial biases that are inherent in those tools. And so race would often be a major predictor, et cetera. And how could this be fair adjusted all? There have been attempts recently to address this to try to reduce as much as possible the amount of bias that still resides in these tools and still their concerns and rightfully so that what those tools are actually picking up are not really individuals propensity to engage or likelihood to engage in some new criminal act or to not show up for court. They're still picking up for the most part the ways that the system itself engages with individuals. And so it's really in some ways a measure of how systems act towards some relative to others. And I think we should take that seriously.
Sandra Susan Smith (27:24):
There's a growing body of research that indicates that mothers who have sons who've been stopped in frisk are much more likely to experience depression as a result of the fact that their children are being engaged in this way by the law. It also leads to depression and other mental health issues with the adolescents themselves and has a significant and negative effect on their educational attainment and academic achievement. These are not policies that have no impact and only do good for communities with the gang database. There's limited evidence of its advocacy and there's a great deal of evidence to suggest that it does a whole lot of harm.
Sandra Susan Smith (28:05):
And this is truly problematic in a context where we know based on evidence drawn from rigorous studies that there are other ways for us to address the youth violence issue without getting young people penalized for something related to how the system is engaging with them not from their own behavior. So we're constantly assessing the risks of blocks and assessing the risks of whites in situations like this to the extent that pretrial risk assessment tools rely on insights that emerge from data that have their own biases embedded in a system that historically speaking and continues to have biases embedded. All we're doing is perpetuating the harms from the past and making them very much present in the lives of the people who have been in standard in the system.
Carol Rose (28:55):
We've seen this in practice. The use of algorithms in the criminal system has not curtailed the over-incarceration of people of color pretrial people who should really be legally entitled to do process of law before being torn away from their family's homes and careers because of an algorithm. So we now see how systems that rely solely on algorithms can codify racial biases is a kind of shorthand to support the function of the system itself. And we've seen how coders and engineers perhaps unintentionally may reinforce these biases by building them into the algorithms when decisions in the criminal justice system are being justified by algorithms similar to the medical example above, it can be hard to recognize the biases, but they're there, there. And because the outcomes are so far removed from the origins of the biases, the justification can seem self-evident and the rationale can seem to play out statistically when in fact it's just a broken system that feeds on its own inaccurate and often racist assumptions about certain groups of people.
Kade Crockford (30:01):
The more you learn about technology and the technology systems that are impacting millions of people across this country and even the world, the more you realize that the historical injustices that run up into the present in the United States, white supremacy, systemic racism, gender discrimination, sex discrimination, all of those biases creep their way into the technologies that we use and the technologies that are used sometimes against us or to control us or to make decisions that impact us in really profound ways. And so the purpose of this conversation is to draw out some examples to show people that technology is not neutral and can be and enough and is infected with the biases that we see in the human systems all around us.
Carol Rose (30:54):
I think it's really important to understand how overreliant on algorithms can actually bake racial disparities into our medical system, into our criminal system, all under the guise of science, and that exacerbates the inequalities that we think we're trying to solve By using algorithms, it actually makes it worse.
Kade Crockford (31:13):
That's right. I remember having a Twitter debate with someone after the first studies came out from now Dr. Joy Buolamwini, who was then a graduate student at MIT showing that facial recognition software can and frequently does have really serious race and gender bias problems baked into the technology itself in the algorithms themselves. I was having this argument with someone on Twitter who've literally said, Technology cannot be racist, algorithms cannot be racist, math cannot be racist. And while math, pure math may not be racist, the conversations that we're having today I think illustrate that technology is not pure math and the way that we make decisions about what technologies to develop, the people who are at the table when those technologies are developed and are involved in the design and implementation of those technologies and the lack of any kind of regulatory system, meaningful regulatory system to prevent discrimination and abuse from harming people as a result of the use of these technologies all result in a situation where we are, in fact, seeing exactly what you said, Carol, the reification of historical injustice through technology.
Kade Crockford (32:31):
Sometimes we call it tech washing, that in many cases the risk assessments I think are a good example of this. Facial recognition certainly is as well technology that the people who are creating it hope will reduce harm and reduce injustice, can actually have the impact of magnifying it and kind of catapulting it into the future in these insidious ways through code that unfortunately most people simply don't understand because most people are not thinking about how artificial intelligence systems are developed or trained, or how risk assessment tools that are used in the criminal legal system are developed or tested.
Carol Rose (33:13):
That's right. And in the over reliance on algorithms in the criminal system is especially troubling when it involves youth. These systems actually label and then funnel kids into the criminal system instead of away from it. And that's why the A C L U has such deep concerns about this reliance on these gang databases here in Boston and around the country. In fact, in January of this year of 2022, the first circuit court of appeals here in Boston actually found that the Boston gang database is an erratic point system based on unsubstantiated inferences and it's shockingly wide ranging. And this critique by an appellate court just beneath the Supreme Court actually echoes the same critique that many of us have had for years regarding the inherent unreliability of things like gang databases and the harms that they cause to marginalized communities and to individual young people. And yet lawmakers continue to hang onto them with the notion that somehow because they're database, they're somehow neutral and that's simply not true.
Kade Crockford (34:12):
So yeah, Carol, the gang database is a really interesting example. Another one is the use of risk assessment instruments in the pretrial context. The backstory to all of this is the conversation around cash bail. There's been a movement across the United States to end cash bail. And here in Massachusetts actually we joined up with some researchers looking at whether or not these risk assessment tools actually produce the kinds of outcomes that governments hope that they will. And that's to say, reduce racial bias in terms of who gets locked up pre-trial. Obviously, it's not fair for someone to get locked up before they're convicted of a crime simply because they can't afford to pay bail, where a person with a little more money would be able to go home and a wait trial with their family in their community. Many studies have shown that people who go to trial from home have much better outcomes in court than people who show up to court dates from the county jail.
Kade Crockford (35:13):
And instead of going along with that and simply releasing people pre-trial if they are not found to be dangerous, pursuant to what's called a dangerousness hearing, governments are moving to use what are called risk assessments to determine kind of similarly to the gang database system on a point system essentially whether or not someone is a risk of flight and whether someone is likely to be arrested again, if they are released pretrial. The government is not supposed to make consequential decisions about someone's freedom, their liberty based on the actions of masses of other people. They're supposed to look at the facts stealing with the person right in front of them, right? That's kind of a fundamental promise of the American justice system that you will be treated as an individual pursuant to your individual circumstances, but the data that is fed into those systems is about the people themselves predicting whether or not those people will reoffend, whether they're, they'll show up to court, et cetera.
Kade Crockford (36:18):
Colleagues thought, what if we could design a risk assessment tool that instead of trying to predict the behavior of an individual actually predicted the behavior of the criminal legal system itself and tried to determine whether or not it was being unfair. And they found that in fact, they are able to predict whether or not someone has potentially been unfairly sentenced based on things like their race, the race of the judge who sentenced them as a result of data points essentially that are not legal, that are unlawful in terms of their applicability to decisions about sentencing. It's a cool example of a way that data scientists, including at the A C L U are using the science of something like risk assessments kind of flipped on its head to actually help people who are trapped in the criminal legal system locked up in prison as opposed to using a kind of tool like this in a way that raises a lot of very serious civil rights and civil liberties and racial justice issues like it does in the pretrial context.
Carol Rose (37:26):
Consider the case of Simon Glock In 2010, the ACLU on Mr. Glock's behalf sued three police officers and the city of Boston for violating his rights after police arrested him and charged him with legal wire tapping, aiding the escape of a prisoner and disturbing the peace all for merely holding up his cell phone and openly recording Boston police officers as they punched another man on the Boston Common. The case has been hailed and cited in multiple other cases around the country and was the first to create the legal protections that permit ordinary people to videotape the police and the performance of their official duties. And in so doing, it's enabled ordinary people to use technology to participate in the nationwide effort to raise public awareness of the epidemic of police violence against black and brown people. Of course, racial profiling by police and things like unwarranted risks, stop frisk beatings and killing of black people at the hands of law enforcement has been going on for years. But what's changed is that we've seen a surge in public awareness of the problem due in large part to the use of citizen videos as well as body cams and and dash cams because more incidents of police abuse are now being captured on camera, white Americans are finally waking up to systemic racism in police practices. Videotaped incidents make it harder for police to hide abusive behavior and make it easier for community groups to verify longstanding complaints about police misconduct.
Carol Rose (38:58):
The conviction of police officers for killing George Floyd stands out for another key reason. The jury was able to see exactly what police officer Derek Chauvin and the other officers did because there was a video tape of the entire incident. Darnell Fraser, a 17 year old high school student, had the presence of mind to record Mr. Floyd's last moments while she was walking by. All of which goes back to the importance of establishing the legal right of ordinary people to use technology to record the police, to use technology in the service of liberty.
Kade Crockford (39:33):
Until we fix issues with race and white supremacy in this country, it will remain difficult to address the issues with algorithm bias at a government level because it's all connected.
Carol Rose (39:45):
So join us next time on Freedom Unfinished for our final episode where we discuss what happens when you bring law and technology together. We'll also speak to an author who tells the story of a CIA agent who thought he'd figured out how the mind works and how it could be controlled. Until then, I'm Carol Rose,
Kade Crockford (40:03):
And I'm Crockford.
Carol Rose (40:05):
And this is season one of the A C L U of Massachusetts Freedom Unfinished Decoding Oppression.
Carol Rose (40:16):
Freedom Unfinished is a joint production of the ACLU of Massachusetts, and Gusto, a matter company, hosted by me, Carol Rose and my colleague at the ACLU of Massachusetts Technology for Liberty program, Kade Crockford. Our producer is Jeanette Harris-Courts, with support from David Riemer and Beth York. Shaw Flick helped us develop and write the podcast, while Mandy Lawson and Jeanette Harris-Courts put it all together. Art and audiograms by Kyle Faneuff. And our theme music was composed by Ivanna Cuesta Gonzalez, who came to us from the Institute for Jazz and Gender Justice at Berkeley College of Music. We couldn't have done this without the support of John Ward, Rose Aleman, Tim Bradley, Larry Carpman, Sam Spencer, and the board of directors here at the ACLU of Massachusetts, as well as our national and state ACLU affiliates. Find and follow all of season one of Freedom Unfinished Decoding Oppression, wherever you get your podcasts, and keep the conversation going with us on social. Thanks to all of our guests and contributors, and thanks to you for taking the time to listen. It's not too late to mobilize our collective willingness to act and to ensure that technology is used to enhance rather than diminish freedom. See the show notes to discover ways to get involved and always remember to vote, and not just nationally, but locally too. Together we can do this.