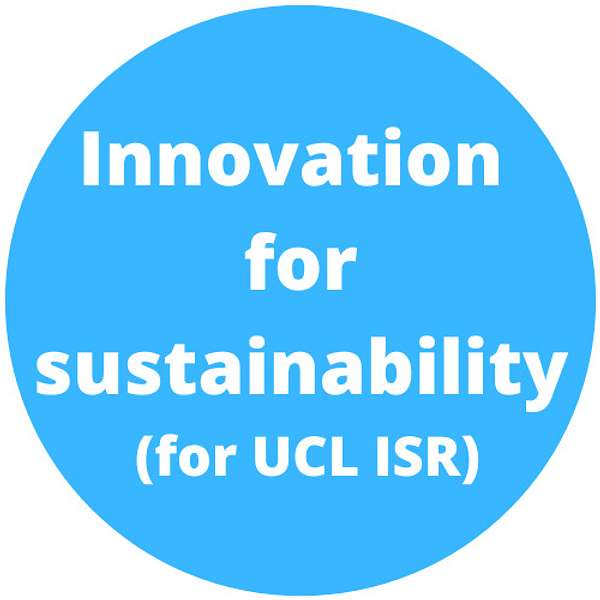
Innovation for sustainability (for UCL Institute for Sustainable Resources Masters)
Innovation for sustainability (for UCL Institute for Sustainable Resources Masters)
Sarah Goodenough
Sarah Goodenough is Head of Policy at Climate Policy Radar (CPR), a "startup using data science and AI to build tools that unlock global climate law and policy data. Open data, open source, not-for-profit."
CPR are right at the cutting edge of the application of AI to climate law and policy.
We cover:
-How CPR grew out of the Grantham Research institute on Climate Change and the Environment at LSE, building out from a project on the Climate Change Laws of the World.
-The approach of CPR to build tools that open up the messy black box of climate laws, policies and case law globally, helping decision-makers design more effective climate change strategies.
-How they are using AI tools carefully, so you can understand how the answer was arrived at (not a black box, like ChatGPT) and to avoid 'hallucinations' (where AIs make stuff up).
-CPR has created AI tools which do various things: extract text from documents; translate into English; train models carefully on this high quality input; and, perform semantic search, making it easy for people to find what they are looking for.
-How and why they work quickly with the data scientists, so the policy knowledge informs the AI development, and the AI capacity informs the requests on policy analysis.
-In the terms of the innovation taxonomy we use in the Masters' module, CPR is has several types:
(a) process innovation: the AI tools changing how someone can do research.
(b) organisational innovation: the way the data and policy teams work together, and having a mission and staff benefits, are organising CPR differently from other AI-led entities, in order to attract the motivated talent they would not otherwise be able to afford.
(c) political economy innovation: supporting others to lobby for climate policies which shift how the economy functions.
-The specific support CPR provided in last year's UN Climate Change Global Stocktake, which is designed to assess the global response to the climate crisis every five years. They launched Global Stocktake Explorer, a search engine designed to enable users to quickly and easily understand and navigate all inputs (over 1,600 dcuments) to the first Global Stocktake. (More here.)
-CPR is an antidote to Cory Doctorow here: “We're nowhere near the point where an AI can do your job, but we're well past the point where your boss can be suckered into firing you and replacing you with a bot that fails at doing your job”
Great to find a positive use of
This is part of a series of interviews about innovation for sustainability conducted for the UCL Institute for Sustainable Resources, as a contribution to a module in this Masters. You can find out more about these interviews, and the module, here.
Ah
David Bent-Hazelwood:Hello, this is one of several interviews on innovation, business and sustainability for the students studying for the MSc in sustainable resources at UCL. My name is David bent, and I'm an honorary lecturer at the UCL Institute for Sustainable resources. And I co lead a module on business innovation and sustainability. Much of the course gives people the latest academic theory and insight, these 30 minute interviews with practitioners to give some of the grit under the fingernails of innovating for sustainability today, and I am delighted to say today we're being joined by Sarah good enough, who is the head of policy, climate policy radar. Hello, Sarah.
Sarah Goodenough:David, lovely to join you.
David Bent-Hazelwood:Great to have you. So first off, what is your role and your organisation?
Sarah Goodenough:So as you said, I'm have a policy at climate policy radar, we are a not for profit organisation, working at the intersection of climate policy and artificial intelligence. And we were founded almost three years ago by my CEO Mikhail Nachman, we run one of the world's largest databases of climate law policy, and uns submissions. That's over 6000 different documents covering all jurisdictions. And we use a variety of different data science and machine learning tools to make these documents as accessible and easy to analyse as possible. We use a variety of different tools such that when you search through the database, it will give you all related terms to the search the use, instead of going Ctrl F through a horrible PDF, and then realising Oh, I was searching for electric vehicles, and in this document, they refer to them as electric automobiles. It we'll find all of those related terms for you and a range of other different techniques. It's quite a novel application of artificial intelligence. Very few people do this type of work generally, and especially in climate law and policy. It's worth saying that the original dataset, so the original group of national climate laws and policies. Most roots, which is where our organisation took its inspiration, our CEO, worked on that project to the Grantham Reese Search Institute, and realise that a small pamphlet or a large book was not actually the most effective way to enable people to keep track of the latest laws, policies, and all of the ever increasing speed updates to national legislation and indeed, un submissions as well. So that's a little bit about our origin story. And
David Bent-Hazelwood:we had a little bit of difficulty with the connection there. But I think we caused all of that. And it was LSE Grantham. So London's London School of Economics, because there's two Grantham institutes, one in Imperial and one at LSE. And that was the that was the sort of place where the first stage of peace was brought together. And before we get into some specifics on the stories and so on, just like what if you say is a relatively new use of AI in gathering and understanding this, these documents? Like, what what's a good outcome? Like in five years time in 10 years time, if what are the goals that climate policy radar set for itself?
Sarah Goodenough:Very good question, David. Our entire mission is to aggregate and help everyone analyse all of this information related to climate change, such that all of the relevant decision makers can make more sophisticated, actually evidence based decisions in order to best address climate change. But the moment you're an ice maker, and you want to understand what best practices in a particular area, say that have fossil fuel subsidy elimination, or whether it's different parts of transport infrastructure, it's actually very hard to do that research. The documents are buried in terrible government websites, they're in a language that you don't read. You don't know whether or not the documents actually relevant until you've read 120 pages. The whole process on understanding who's doing what to address climate change, and what's working is just ridiculous. So we're hoping that within the next five years, the sophistication of our tooling will enable people to answer those types of questions incredibly fast and genuinely evidence based. And that we will be able to overlay a lot of this previously unstructured data by which we mean just like lots and lots of text, being able to overlay this and combine this with a range of other datasets such that for example, you can look at all the different ways in which countries with coasts are talking about coastal erosion or other related extreme weather hazards in that policy and overlay that with a map of physical risks that these countries are exposed to, and then immediately see the gaps, where countries are talking about risks in a way that is insufficient, where they are not addressing something as rigorously as they should. And really starting to treat this as big data, which previously with climate law and policy, we really just don't do. Cool.
David Bent-Hazelwood:So there's quite a lot to unpack in all of that. So first is the role of climate policy radar in for one of a better phrase information architecture that or rather information infrastructure that you are bringing together in a way that is accessible to many different kinds of users, documents and data, which is otherwise buried or very hard to find, or very hard to get hold on. So there's an information aspect. But it's more than just turning data into information. It's also turning information into insight and accelerating the pace of learning what is good practice and what the next good practice might be, if I'm understanding you correctly, is that a good way of framing it?
Sarah Goodenough:Yes, that's a really good way of framing it. And I wonder whether it's now useful to re emphasise the point that we are a non profit. And we have, I think, through the white labelled climate change laws of the world, which is a database that we wrote with power for the London School of Economics, Grantham Research Institute, about half a million users every year already. So policymakers, researchers, students doing their master's theses, in academics, you name it already freely, being able to read through and analyse all of these documents. And our hope is that we will build even more sophisticated tools to support their use cases. And
David Bent-Hazelwood:that also just wanted to dive a little bit into those users. So presumably, users are civil servants who have to draft a climate law. And they're going How the hell did somebody else do this? It's think tanks going what is the best way of taking carbon out of hard to abate sectors, presumably, is also investors and investment analysts going which of these countries is making the best choices on addressing the physical risks of the climate risks that they may have? So there's a very wide range of different potential uses. And users? Absolutely.
Sarah Goodenough:And it's been quite complicated to explain, because the answer our users are everyone never really seems to be convincing. But it's also worth emphasising also that the way in which we use artificial intelligence in the tools that we are building is not in that scary black box style of the large language models that people are really familiar with such as you know, chat, GBT, you ask it a question, it gives you an answer, you've got no idea how it's arrived at that information. That is absolutely not the way in which we use artificial intelligence. It's in ensuring that we are finding and then presenting the data that is in the database to users in a sophisticated and speedy way as possible, so that the user is then presented with decision useful information as quick as possible, and can very easily read or analyse, and then make the decisions themselves. So there's no climate policy, radar bot, that is telling a certain country, this is how you do climate law or policy, it's very much making sure that we get the evidence into the hands of the decision makers as effectively as possible.
David Bent-Hazelwood:Yes, it's worth saying that in the master's module that will my my co lead and I run, people have to do essays. And we set a level of how much AI they're allowed to use, because it's inevitable that they are going to use it. And we say that if they use AI, they have to attribute it. And also to treat this as more of a chat GBT kind of AI and treat it like a knowledgeable friend down the pub, who also sometimes make stuff up. So there is what the whole hallucinating aspect of those chat GPT kinds of models. How do you? I mean, is there any risk of that hallucination from your AI? I mean, how do you control for that, that aspect? Or is it or is that not relevant to the way in which your AI is designed?
Sarah Goodenough:It is relevant, but I think it's worth just being very specific about the different types of artificial intelligence and different tools that we use in our process. And it's worth just, I guess, clarifying. I am the head of policy. It's the whole technical team. But please don't hold my feet to the fire of any of the extreme details of this although obviously, I am familiar with them. So in the first instance, we use a process called classing for which of the techie listeners will be interested as the Microsoft as your parser. What that does is transform PDF documents into machine readable text. So it's almost like kind of a vision recognition AI where it extracts the text from a PDF which is otherwise horrible to read both for humans and for computers, transforms it all into machine readable text, which then enables us to do all the really fun stuff that we do data. We Secondly, automatically translate all of that text into English, which is massive for accessibility. All of these documents soon, hopefully will translate into many other languages. But English is obviously a very good first start, we then structure this data in a range of different ways. So that's training machine learning models to automatically identify concepts within the documents. So that might be policy instruments, it might be sectors of the economy, it might be vulnerable groups, technologies, climate hazards. And that is the process of policy to our policy team, designing a methodology that is accurate for policy researchers, and then labelling a huge amount of data extracted only from our data set of climate laws and policies. So unlike some chart, GBT, which is trained on the whole internet, we train our models specifically on climate law and policy, and label a huge amount of data to teach a machine learning model how to identify these concepts itself. And we test that equitably and fairly across different subpopulations. So we make sure we sample documents originally written in English, and ones that were translated laws and policies and UN submissions from all different regions. So that's many of the ways in which we already use artificial intelligence, and also the semantic search that I mentioned earlier, the DVS electric vehicles example, using those embeddings is a really powerful tool as well, we are starting to experiment with large language models. And both there and with the machine learning models as where bias is a real challenge. So one of the ways in which we mitigate bias in the use of the machine learning models that we train to identify concepts is by that subpopulation work that I've indicated. So making sure that we get a representative sample of all these different types, because the last new one is to have a machine learning model, live on a product that half million people a year use, that says that there are no mentions of particular of a particular policy instrument being used by governments in the Global South, just because those policies actually use a slightly different term. And we did capture that. So we're very, very cognizant of that. So we work with a lot of different academics partners with from a range of different institutions. And so on the on the tech side, make sure that it is as balanced as possible with large language models, such as Jacques GPT. One of the big issues is that hallucination and bias that we all know from, you know, your students using it to write essays. Or there are some famous cases of lawyers fighting legal cases in court that literally have never happened. They've literally just been made up by chat GBT. And one of the really exciting developments in trying to address this is a process for retrieval augmented generation. Now, what not red, amber green. So rag, but it's retrieval augmented generation. And in short, the process there is, instead of asking a large language model a question and saying, draw on all of the information on the internet on which you were trained, which obviously then encounters a huge amount of risk. What you do is you say here is, for example, a specific document, I've asked you a question. And you can only look at the evidence within this document on which to base the answer that you generate for us. And then not only that, but you must provide the answer in bullet points, and you must provide references. So then you've got a limited data source that the large language model is drawing on, which makes it more likely that the answer is going to be accurate. And then it's all auditable? Because you can look at the passages that the large language model is citing and say, Yes, this has fairly represented Nigeria's agricultural policy from 2022. Or, oh, my gosh, this library model has just completely made something up. And that is a really interesting way of mitigating those hallucination of bias risks that we're experimenting with at the moment, we're literally launching a prototype I think next week to some users are staying on exactly that application. And
David Bent-Hazelwood:that that particular application sounds very much like a sort of a focus literature review, which as you say, whoever does it should come up with more or less the same results. So it should be repeatable, and therefore it should be auditable. And anyone who's had to do a literature review will know that they can be very dull, but they are very useful the outputs for not just for yourself, but for other people too. So that's part of this, accelerating the learning that we need, and which is an important part of addressing an urgent challenge if you're accelerating how fast good practice is tested and transmitted around the world and you're accelerating the pace at which we are responding to an urgent challenge. Nothing really in the world more urgent right now than climate change. As we move into the next set of things you started talking about you caveat it all of that with your only the head of policy, and not at one of the decades. So talk to me about your role. What is what does your What does health policy mean? What is the policy department get up to you?
Sarah Goodenough:Very good question. Davis. The climate policy radar is, you know, we're nearly 25 people now growing very quickly, when mostly software engineers, data scientists, data engineers, which is itself a fantastic innovation, because the world of climate change, advocacy research and NGOs is famously I think, still stuck in the 1980s, unfortunately. So the role for me and my team is to be the climate and the policy experts, which embed that understanding and that domain knowledge into everything that we do, the purpose of our organisation is to apply tech expertise to a specific problem. And you know, that is that is understanding of law and policy to accelerate action on climate change. So in that sense, we are there guides, and therefore, we're very influential on the strategy of the organisation because we're connecting our tech expertise to our purpose and our impact. And that guides, you know, what data we want in our database? Do we expand into nature based solutions, water, human rights? Do we look at sub national documents? Do we look at corporate disclosures? What is our main routes to impact? Why do these documents matter? Who can we go and partner with? It will be designing what machine learning models that we train to enable users to search for certain concepts in our database. And that includes designing what that priority list is actually designing the methodology of what counts as, say, a sector of the economy or a quantified target. And then designing and doing all the all of the manual labelling as well and partnering with our data science team, if we determine what projects we work on, and what partners we work with, so who's who's useful as a route to impact versus who's going to be not necessarily that valuable as a partner, and then all the product features, so is auto citation, something that is useful for users is also translation, something that's particularly impactful. It's worth saying that we're not necessarily expected to be actual policy experts. My my background, as you know, David is in strategic communications working at an international level with the UN. But it means I need to know who to bring in, who has that knowledge that we can bring in from from outside and connect to those relevant experts, and bring that learning, and also the tools that we build back out into the ecosystem as well. It's worth saying that, whilst I am head of policy, and we have a policy team, we work so closely with the data scientists that we actually also kind of function as an r&d team. Try coordinate with our head of data science, and that enables real innovation because we do, for example, we launched a prototype just before carp, which enabled users to explore quantified targets in law and policy documents that were which were identified through designing and training a machine learning model. And as part of that, we did a two week Design Sprint where you had one policy person or one data science in three teams of two, if you went away and quickly iterated different prototypes and different experiments, which we then combined together as part of this, which is a real, you know, the old world deployment policy meets the new all the data science. Yeah.
David Bent-Hazelwood:And the way I hear you talking about that is the climate policy radar is about enabling others to use that information. And so you yourself are not necessarily campaigning, you're not out meeting a client minister or anything like that you are making sure that the outputs and services provided by climate policy radar are relevant to that policy world, through all of that r&d and testing and through your own experience and checking with customers and so on. But you yourself, you're not like drafting policy documents, you're enabling many, many other people to be able to draft policy documents.
Sarah Goodenough:Absolutely, we are strictly policy agnostic as an organisation. All we want is for climate law and policy to be as effective and as evidence based as it can be. Whilst personally obviously we've Choni opinions over a bit as an organisational level advocacy here.
David Bent-Hazelwood:You heard the invitation here, first people so and then just the next question is about how is sustainability framed in your organisation? Do you have a particular definition? Do you have a particular desired end goal?
Sarah Goodenough:I don't think we know the definition of sustainability at CPR necessarily, but it's worth saying that it is just core to what we do. You know, our organisation was Set up, because we've identified a real pain point problem, issue with the way in which we were addressing climate change. And it's a core part of our mission. Sustainability has informed our focus areas. So, you know, we even though we initially are looking at climate law and policy because of the original climate change laws of the world project to the Grantham Research Institute and that dataset, a broader part of our work is hopefully to de silo domains, because this is a systemic issue and climate is one lens. But actually, water, oceans, nature, food systems, all of these things are, are fundamental. And so we will be looking at making sure that organisations and researchers have better access to that full suite of information that enables us to understand environmental issues at a much grander scale. But that's, that's a vision for several years time. I mean, it's worth also saying I think that sustainability for us, is also a core part of how we, we operate. So we're a nonprofit, and everything we do is open source. So literally, our code is open source, our data is open source, it means we're incredibly auditable, it means we can work with a large range of pioneering academics who would otherwise not want to touch us with a 10 foot bargepole if we were, you know, a for profit, corporate. But also, we're quite progressive in the way in which were structured such that we have a four day work week and unlimited holiday. And wellbeing is a core part of, of how we operate. So sustainability, also on the personal level, I think is quite important for us.
David Bent-Hazelwood:Cool. And of course, there's a lot of competition for talent in the AI world. So being able to offer those benefits is a useful way, like presumably not being able to offer the same kinds of salaries as a Google or an a Microsoft. But you can offer a sense of mission and positive benefits. And people who are motivated in my experience don't necessarily take all of the holiday that they're do so that just because they have unlimited holiday doesn't mean that people take it. So we
Sarah Goodenough:do we do force everybody to take the minimum risk with unlimited holiday that people actually on average, take less than the statutory minimum because of that feeling of peer pressure. But you're totally right. Like, we recently hired a director of engineering and a C, senior data engineer, who we just wouldn't have been able to afford otherwise. But they want to spend time with their kittens and their two year old son, and they're attracted to our mission. And as such, it means that we can be very competitive in an otherwise very expensive landscape. And
David Bent-Hazelwood:just to relate all of what we've been saying so far to one of the foundational pieces in the module, we make the claim that all sustainable businesses innovative in some way, and that there is different as we have a typology, which gives out different kinds of innovation. And already you've expressed at least three of those, one of which is process innovation. So how something gets made the the all of their AI, different AI is, in fact plural, about gathering that information, making sense of that information and making it easy to access all of that all of those have different process innovations. And you also described various organisational innovation. So firstly, how that r&d function works with the combination of the pairing up of the different expertise is of Data Science and Policy science. And then just in your last story there an organisational innovation to do with the nature of the staff benefits and how that matches with your mission in order to attract the talent and people that you need. And then finally, there is a an attempt at a political economy, what we call a political economy level of innovation about influencing the what governments get up to the the way in which economies function, the boundary between state and market, the roll all of these policy questions and political questions. And you're hoping that people will use your outputs in order to make differences in at that political economy level. So there's many different interlocked kinds of innovation underway here, which I want to acknowledge. And then move on to the next question, which is a chance for you to tell us a story. You've already told us about some of the new things you've been launching. But are there any stories which are a good example of your work, which you would like to be able to tell us?
Sarah Goodenough:So very fun question. Thank you, Davis. And I guess already you've identified that, yeah, there's a lot of different ways in which we innovate the CPR, but I guess a nice, a nice story to tap out is a project that we've launched in official partner, a ship with the UNF Triple C that's the UN Climate Change body to support an official part of the UN process last year. And I think it's an interesting story because it's the use of quite pioneering techniques. Knology as we've identified in the two famously cumbersome and bureaucratic multilateral space, where the negotiations it at the bottom internationals, the the SPS in June last year, I think there was a four hour negotiation specifically about whether the notes of that particular session were going to be captured in a Word document or in a in a Google sheet or something as ridiculous as that. So this is famously an area that is
David Bent-Hazelwood:just too so sorry to interrupt. But like, the reason one of the reasons why that the climate cop is a anyone can veto the result is because the rules were not agreed in the very first cop. And so it defaults to that. So it is even worse than the example you just gave, the first cop in Germany didn't manage to agree what the agreement rules were. And therefore we're left with the consensus, which means that no one can veto anything. But anyway, back to you. So you having you're playing with this incredibly bureaucratic and clunky world. And you've had an innovation, innovation.
Sarah Goodenough:Absolutely. So just to give a little bit of context, for listeners who may not be familiar with the UN process, there was the Paris Agreement, which was ratified by most countries in 2015, which is our only international agreement to address climate change introduced as part of its attempt to maintain ambition, and to not just keep what was agreed as 2015 as a static thing, but introduce future opportunities for programmers be delivered, included something called the Global stocktake, which was a process that's going to take pace every five years, which is why it was concluded, the first one was concluded last year, to do essentially what it says on the tin, which is to take stock of collective progress towards the goals of the Paris Agreement, which is incredibly important. It was the opportunity for all governments and also what we call non party stakeholders that could be universities. So you know, UCL could have submitted something, it could be different companies, different NGOs, other international organisations, all submitting materials that talk about where are we? Where do we need to get to one of the blockers, the challenges, but also the opportunities? What are the success stories and the lessons learned in addressing climate change in the last however many years specifically looking to what needs to be done in the next five years, unbelievably important information. And even though lots of NGOs do these kinds of state of climate action reports every year, this one was really important, because it's an official part of the UN process, which means that it has much greater weights in how you can then leverage those results or those outcomes to different governments. And it was part of the
David Bent-Hazelwood:sorry, just to build on that. Just sorry to interrupt again. For those the architects of the Paris Agreement, this was part of the ratchet. So the idea was a ratchet mechanism. This the Paris Agreement got us started. And they knew that what was agreed there would not be good enough. But by having a stocktake, the hope would be that that would constantly ratchet up the level of ambition. So it's absolutely crucial to delivery anyway.
Sarah Goodenough:Yeah, so the problem was, is that the way in which the global stocktake was actually written into the Paris Agreement, and the way in which they designed the process to be as kind of fair and equitable as possible, essentially said, Everybody stood met, whatever information you would like to submit, in whatever format you would like, to this terrible part of the UN IT system by a certain date, which as you can imagine, is just actively incredibly unhelpful, because that means you've got 1000s and 1000s of documents, just over 1800, and how everyone's going to have the time to read them, synthesise them, analyse them, and draw meaningful conclusions from them within a negotiation cycle of a very short amount of time, is just absolutely obscene. So without any additional funding at that point, and with with no, like end point, I see recognise this as happening. We knew the on our advisory board was a member from the UNF, Triple C who was responsible for the global stocktake process. And we said like, look, this is ridiculous, surely, surely we can help. And so we set about working with the UNF, Triple C UNF, Triple C to see whether or not there was an opportunity for us to design either bespoke or tooling that we already had in order to just enable just team members in the UNF, Triple C originally, to just read, analyse and understand all of these submissions, because they had to write this huge technical report that was going to be put to parties at some point like that. Late last year, and it was a key part of the negotiations and obviously incredibly important for our understanding of climate, and was very much in our wheelhouse of large amounts of documents, varying length and complexity and languages and needing to synthesise and read very quickly. So we sat about not only, you know, bringing all of these documents in and processing them from the UN portal, and designing a nice kind of interface so that you could just easily read because the navigating through the different documents on the UN portal itself was a pain. But actually, we went one step further, because there was a range of different questions that the documents were trying to answer. I think there was 64, or something into that 30 Something questions. And these are questions that had like 17 sub clauses in them, you know, at the sub national, national and international level, what are the barriers, challenges, success stories, lessons learned? You just absolutely chaotic. And so what we went about is trying to identify what is the information that people need to be able to understand from these documents? What are the things that they are looking for, that are common across all of these different questions, and that's where we prototyped some of the machine the our first attempt at these machine learning models, or classifiers, as we call them, and other linguistic rule based filters to enable people to get to this information within these documents that they're looking for. We worked with our data scientists to design these different solutions. And we went out to just a huge number of people in the climate space and asked as many questions as we could about, what's the information that you would need to look for? What does it need to look like? And I can't tell you how crazy that time was David because we like no one had ever done this UNF, Triple C didn't know what they were looking for. Because again, they'd never done this before. It was the first global stocktake the questions were very bureaucratic, that timeframe was very short. And so we really had to kind of MVP this. And then also you have the added complication that because we were working at that point, not officially with but very much supporting the UNF Triple C process, you had the added bureaucracy of making sure that all of the language was as sensitive to the UN as possible. So mitigation, including response measures, adaptation, including loss and damage in very specific terminology really mattered. But that's where it was very beneficial for us to be quite an agile startup, our head of product, absolute shout out to Alan he was a used to be head of delivery@gov.uk, Managing 40 engineers across a range of different programmes. He's absolutely fantastic at enabling us to do kind of agile work, sprint planning, working in short cycles, all the kind of innovations that we've seen in tech companies in the last couple of years that are able to build and deliver something that if we were a different scale, or a different structure would have just been absolutely impossible. And so we launched a prototype, the bond internationals called GST one.org, we very cleverly got that URL for anyone else did, which enables all people to read through all of the documents that were submitted to the global stocktake plus all the documents that weren't submitted, but are listed as being relevant in the Paris Agreement. So that's nationally determined contributions, which are government's commitments to address climate change submitted to the UN, and other relevant submissions, all translated into English. And we experimented with doing passage centric rather than document centric search, so that when you search through the system, it will give you the kind of paragraphs of text that you might be looking for. So you get to the evidence as quickly as you can. And we experimented again with these different machine learning filters. So you could look at where the countries mentioned fossil fuels and the movement of money, where our government's talking about a particular extreme weather event or a particular climate hazard in the context of a particular technology. So really enabling some of that nuance, to be identified within the documents. And that's, again, like the first time anyone's done this, as far as we're aware in the climate space. And we launched it at the negotiations in June last year. And the EU lead negotiator called for it to be an official outcome of the process, which was so much more than we had possibly imagined. And we got a huge amount of positive feedback for it. And then again, the UNF, Triple C then decided it could be an official partnership with them, which was fantastic. And that's just been brilliant for us, because we've just learned a huge amount about how people would interact with tools such as this, and how artificial intelligence and data innovation in this way can support previously incredibly bureaucratic processes. And so everything that we learned in that very short I think we delivered the project and maybe like 110 days which Thinking point is just mad. It we've learned so much and a lot of that is now being built upon more rigorously to then build into product such that our database will have a lot of that same functionality as well. Cool. Do
David Bent-Hazelwood:you know I mean, it's great that people love wanted it to be recognised as I mean, it sounds an amazing story. It's just astonishing. Do you know how it was used? Do you know if there's any outcomes you can point to from it existence.
Sarah Goodenough:There are no outcomes, specifically within the UN processes as a direct result of the tool, because UN process being what it is, unfortunately, there is
David Bent-Hazelwood:a difficulty however,
Sarah Goodenough:they may made their minds up. And the evidence is kind of irrelevant, right, which is one of the reasons why we're built in tools like this such that you can't say, whatever you want evidence be damned and actually know, the evidence is now very easy for you to read and understand. And so you've got no use about to actually take it seriously. But we spoke to a lot of negotiators when we were at the internationals, and also when I was at carp at the end of last year, who found it invaluable for understanding on the government's position on climate change, and especially for global south developing countries who have very little capacity. And, you know, very small staff in their, you know, Foreign Office, or the negotiating team tools such as that are invaluable. We also have a lot of tracking. So from a product user perspective, we know what people searched for, and we know how they interact with the filters. And that enables us to then understand, you know, what are people interested in? Why does somebody search for pineapple? It's fantastic.
David Bent-Hazelwood:And it's reminding me of, I think, is Arthur C. Clarke. And wonder if it could have been him who said it first. But that trying to get information from the internet before Google the search was like trying to get a glass of water from Niagara Falls. And I think there's something similar here about trying to get good information about what's going on in the climate space and going up. I mean, it's even narrower than that going on in the climate policy law space, was like trying to get a glass of water from Niagara Falls before you invented GST one.org which are sticking to the shownotes. So people can follow it there. And presumably there'll be GST to in five years time, but we'll see about that. There was one piece of jargon you used in the middle, all of all of that? MVP, minimum viable product? So just good enough? Yes. Sorry. No, no, it's great. It's just, it's so much part of the startup. Yeah, it's just part of such a part of that startup culture that it's become endemic, but I just wanted to unpack it. And then, even when we're gonna move on to the next question, which is about innovation management, and you've mentioned some key methods and practices, you mentioned, agile, for instance, are there any things which are? And you've also mentioned, sprints, and to some extent, hackathons, by pairing together different expertise? Like, what are the methods which are most in use within CPR?
Sarah Goodenough:And your answer is all of those Yeah. And we, we do short planning cycles. So within the r&d team, myself and our head of data science at ordinating, have three week cycles, because you can't plan on three weeks in advance, you know, our understanding of what we're doing changes almost every day, the technology changes, you know, new innovations. And so we have to plan in these codes, you know, got a six month view and a three week view, at some point, they'll fit together. We are actually incubated in a climate tech hub. Our offices are in the sustainable ventures office space and County Hall and Westminster, which is just exclusively climate tech startups, which is a fantastic area to work in. So that really supports us the way in which we work by having that r&d Team function. So combining the domain expertise, and the attack allows for that real innovation. And it's worth saying we do we're very user centred design. So our head of product does a lot of different user interviews. We work very closely with our partners in crime at the Grantham Research Institute, the climate change laws of the world team, who gave us a huge amount of advice and there are kind of first monkeys to test different products and tools that we're developing. But we also regularly do user testing on a range of different prototypes that the retrieval augmented generation that I mentioned earlier, we're going to be starting user testing I think today before launching it more widely. But I think it's that it's also the open source that enables us to learn from what everyone else is doing to work with academics we are or head of data science has started putting together a climate NLP community. So NLP meaning natural language processing, which is specifically a type of data science and AI application to the text rather than to numbers. So obviously very relevant to the work that we do. And now That's a kind of a slack and a monthly working call of everyone who's working on applying NLP to climate once a month. And so that kind of radical collaboration. And you know, we really do put our ego at the door and we prefer to collaborate rather than compete. I don't think that's helped you, David, he wanted to prioritise list.
David Bent-Hazelwood:I think what he tells us is that your your nonprofit, but you're one which is very much in that AI startup kind of culture and space. So you're using a lot of those methods, which had some incubation within Silicon Valley and relatedly. And one of our other interviews in this series is with Chris ganja, who is a specialist in Agile, so people who want to know more about that agile and the short delivery cycles, which have a rough goal that you're heading towards, but you don't necessarily know all of the pathway there should listen to that one. Last few questions. What's the biggest challenge you face? And how do you overcome it?
Sarah Goodenough:That's a really good question. I mean, there are several challenges. People don't understand what we do. Because how we work in the ecosystem is unusual trying to work is this fundamental enabling layer underneath the whole climate ecosystem to accelerate all of their work took a while to explain to funders who like to be able to point to the amount of money donated and amount of co2 taken out to the atmosphere. So that's definitely a challenge. People don't understand AI, which I've only worked for here just for just over a year. And there are still a lot of gaps in my knowledge. But the general public has a lot of distrust, rightly so. But there's also a lot of misinformation, which is a challenge, we want to play a role in, in educating and advocating for responsible use of AI. But I think the biggest challenge is that no one has done what we're doing before. We're at the cutting edge of the application of AI to climate law and policy. And that brings a range of different challenges, we have to be very forgiving on ourselves and keep a very open mind talk to many people as possible and tolerate a huge amount of uncertainty. We have to MVP, everything. So minimum viable products, and everything that we do perfection is absolutely the enemy of the good and the enemy of momentum. So Tom Carnac, and Christiana architects the Paris Agreement, and I used to work with them a global optimist, and they said, momentum is better than perfection as a mantra that I think is very important, both to me personally, but also for our work at CPR, and constantly come back to our direction of travel. Well, how much we've learned constantly reevaluate. We do something called retrospectives or retros. Very, very regularly, where we stick post it notes up on a virtual or an in person board and go, what's going well, what's not going so well? What do we need to change? And so our organisational structure and our ways of working are constantly being evaluated. We're constantly working out, you know, how to build new internal infrastructure to do the work that we're doing that we didn't even know we needed. So yeah, it's a, it's a lot of that plus a good amount of camaraderie. Yeah, well, I
David Bent-Hazelwood:just want to draw the link there between, if you're going to be at the frontier of a process innovation of AI, then you also need to have an organisational culture which matches with that, and hence the need for that sort of self compassion. And the other theme I'd like to just to draw out, and this is especially true of startup organisations, or organisations, which are in a frontier of highly dynamic area, the ability to learn quickly, so to reflect to say, what's going well, what's going badly, and then to implement the insights rather than just have the same post it's come up again and again, that that speed of learning is actually crucial. Lastly, questions if there's one thing policymakers could do, which would make your work significantly easier, what would that be?
Sarah Goodenough:It's a bit of a cheeky answer, but we want policymakers to continue using our tools and to engage with us and to look at what's been done, what works and rights genuinely evidence based climate law and policy. I mean, very specifically, we just want to talk to them as much as possible to understand what we can do to make their work easier, more evidence based, sophisticated, faster.
David Bent-Hazelwood:Answer and then the final question is your priorities for the future. What are they?
Sarah Goodenough:I mean, our priorities are never anything we want, we're very greedy. We want to eat as many documents as possible. That is as valuable. Think of us as a non for profit equivalent of a hungry hippo. And also massively increase the scale and sophistication of the tools that we build. I guess the best example is retrievable advantage generation. That's that's a major innovation priority for us, moving forwards, enabling people to be able to ask what does you know? What does this Nigerian agricultural policy, say? What are its three main priorities? Whatever it is, being able to get an accurate answer will save people so much time will be just such a leap forward in terms of our understanding and speed of analysis, liberal policy for so yeah, it's retrieval augmented generation for the next six months. Yes,
David Bent-Hazelwood:and as you say, the long term goal is clear. But the specific things will change on a day by day basis, because that's part of being an agile organisation innovating right at the frontier. I want to say a huge, huge thank you for all of that. I think it's so fantastic to hear. I suppose at a loss of scare stories in lots of different directions around artificial intelligence. And there's a writer called Corey DOCTOROFF who particularly says, well, the quality of AI isn't really good enough to replace your job, but the court the hype about it means you might get sacked anyway. It's great to hear an example of AI actually being put to use in a way which is making a significant difference. You keep on referring to climate policy, radar CPR, and hopefully you are providing some CPR on to the entire climate negotiations and climate policy space. So thank you very much to you, Sarah. 10 Fantastic.
Sarah Goodenough:Thanks for having me. David likes to chat. My pleasure.