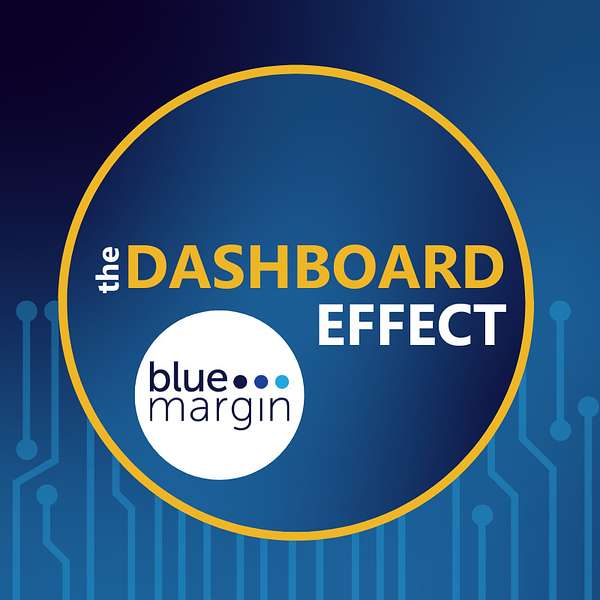
The Dashboard Effect
NEW EPISODES EVERY OTHER THURSDAY!!!
The Dashboard Effect Podcast: Simplifying Data for Smarter Business Decisions
Welcome to The Dashboard Effect, the go-to podcast for mid-market businesses and private equity-backed companies looking to harness the power of data.
Hosted by Brick Thompson from Blue Margin, we demystify data analytics and business intelligence, offering practical insights and actionable strategies that drive accountability, performance, and growth.
From breaking down complex data concepts to sharing real-world success stories, we cover topics like Power BI, data lakes, dashboards, automation, and the latest trends shaping the future of business intelligence. Whether you're a CEO, operator, or BI professional, we’ll help you unlock the potential of your data for smarter, faster decisions.
Tune in to The Dashboard Effect and discover how the right data, at the right time, can transform your business.
Subscribe now and take the first step toward becoming a data-driven organization!
Learn More: BlueMargin.com
The Dashboard Effect
Data is Gold in AI's Wild West: Insights from Salesforce's State of Data & Analytics Report
In this episode, hosts Brick Thompson and Caleb Ochs discuss the current state of data and AI, inspired by a recent report from Salesforce. They discuss the importance of a strong data foundation (and what constitutes one) for effective use of generative AI, emphasizing the need for high-quality, consolidated, and secure data. They also cover some of the challenges of advancing data initiatives (e.g. keeping data projects aligned to business goals, differing perceptions of data quality and usefulness across business departments, etc.)
Click here to watch this episode on our YouTube channel.
Blue Margin increases enterprise value for PE-backed, mid-market companies by serving as their fractional data team. We advise on, build, and manage data platforms. Our strategy, proven with over 300 companies to-date, expands multiples through data transformation, as presented in our book, The Dashboard Effect.
Subscribe here to get more episodes of The Dashboard Effect podcast on your favorite podcast app.
Visit Blue Margin's library of additional BI resources.
Welcome to The Dashboard Effect podcast. I'm Brick Thompson.
Caleb Ochs:I'm Caleb Ochs.
Brick Thompson:Caleb, we've been reading reports that have been coming out, specifically one from Salesforce and one from AWS around data and AI. There's a report out of Salesforce called State of Data and Analytics. Listeners can go out and get it. Just Google Salesforce State of Data and Analytics, and they'll send you to a page where you can give me your name and email address. They'll give you a copy of it. We thought it would be interesting, though, to talk through some of their conclusions in here.
Caleb Ochs:Yeah, some of the high points, our perspective on it, we're just gonna kind of wing it. So bear with us.
Brick Thompson:Yeah, so it may give you all you need. You don't need to go read, or you may want to go deeper on it. I think we started talking about Salesforce and AI because of an ad you saw with Matthew McConaughey that was really interesting, sort of that weird AI video. He's a cowboy.
Caleb Ochs:In an old Western town. Yeah.
Brick Thompson:And the tagline.
Caleb Ochs:He says, if "If AI is the Wild West, is data, the new gold?" That was kind of cool.
Brick Thompson:which I think it is. And acutally I love that play, because everybody's been saying, you know, "Data is the oil of business." But, I think that's right. AI is kind of the wild west right now. But as you go through these reports (and our actually our experience just working in the world), if you don't have your data in order, if you don't have your data consolidated, it's going to be hard to get value out of AI.
Caleb Ochs:Right. Right. And I think some of these reports really touch on some of those same thoughts, but it's interesting to see some of the statistics and the responses that they got in their survey that they sent out. So if we can touch on some of those things?
Brick Thompson:Yeah, well, well, let's do that. So let's just go through some of them. So I think they surveyed something like 10,000 data executives. It was kind of interesting everybody's viewm -- there's no question that there was very strong agreement that strong data foundation is what you need to be able to get good outputs from generative AI. And it's sort of that old maxim, you know, "garbage in, garbage out." So you have to first of all, collect all your data in one place, so the generative AI and analytics has stuff to work on, but it also needs to be high quality. Relationships need to be right.
Caleb Ochs:Yeah, that's kind of the theme. Right? I guess that does beg the question, (you kind of were just starting to touch on it), but like, what does a good data foundation mean? You know?
Brick Thompson:Yeah. Well, that's a good point, so what do you think it means?
Caleb Ochs:I think the easiest way to try and describe it is, in my opinion, is you have your your data is accessible, quickly. If you can trust it, if you rely on it. And it's proven to be accurate.
Brick Thompson:Right. Yeah. And I think that the survey here the Salesforce survey would agree with that. I found a stat here that IT leaders requirements for successful generative AI use 55% said you have to have accurate, complete and unified data, which is pieces of what you were talking about there. 54% said you have to have an enhanced security measures to avert new threats to the business. Obviously, with a generative AI you could have leaks, but also with your data. If you're really consolidating it, you need to make sure that you're doing a good job with that. It makes me think of something we've been talking about, which is, how do you make sure you have good cybersecurity around your data? The way we do it is we work in the Microsoft stack. So we work in Azure Synapse, now Fabric. We follow all of their best practices. We see companies that.. I don't want to use word kludge, well, I guess I will... that kludge together a stack for their data, using DVT, Fivetran, or various tools to get the data pipelines and data movement on the ETL and ELT is done and then land the data somewhere else. And as we were talking about it, we realize you've got so many potential holes there, you've got to plug, because these systems are able to talk to each other and who has access and do you really have it buttoned down. Having some kind of a system, maybe a snowflake system or, or an Azure system or, you know, some unified system where people are really watching that and thinking about how to do it well is super important.
Caleb Ochs:Yeah, I agree. I don't think that's something that people don't really think about when they think about their stack is all the potential different really holes that you've opened up. You just have a lot more to be worried about. If you're on Azure or you have everything in one stack one tool than you've just got to make sure that things locked down, has good policies and stuff like you're following best practices there. You have good practices around that tool. If you got six tools, now you've got six different ones, you've got to learn the security, you got to understand it, you've also got to have best practices around it, you've gotta monitor and make sure you're doing that. So there's a lot more overhead than then meets the eye with a stack like that.
Brick Thompson:I think that's right. So here's another page in the Salesforce report saying AI shines light on data management. So the generative AI is getting all the buzz, but the people that they interviewed, said, really, it all starts with data. It's amazing what percentage of companies think they're above average. I think something like 80% thought they were at industry standard or above. I think our experience has been that that's... Well, actually, it may be true that 80% are at standard or above, but standard is not great.
Caleb Ochs:Industry standard kind of sucks. I think that's one of the interesting things that we see a lot is that we start getting into doing some of these data projects. We start making some of these things more visible than just an Excel output from the source. And then the data issues really start to crop up. Because maybe you got an analyst that's like manually populating stuff just because they know that that needs to be over there. I remember one time when I was an analyst, and I would download these reports, these Excel sheets, and I would create an inventory report. And there was one set of SKUs that was under one business line. But every time I downloaded it, I had to move it into a different business line. Manually. I just had to do it. And I didn't one time, and everybody freaked out. Because they were like, "Why is our inventory so high?" Oh yeah, sorry, I forgot to move it. So you have little things like that that are may not be hurting you right now, but AI is not going to do that for you know, unless you tell it explicitly: This was not in the right spot. It's just going to look at the data at face value and take it as it is right?
Brick Thompson:Yeah. Well, you were probably an industry
Caleb Ochs:Yeah, right. standard there.
Brick Thompson:I found the stat it was only 5% who self reported they were below industry standard.
Caleb Ochs:5%. Yeah, alright.
Brick Thompson:So that's pretty good. That makes me think of that assessment you saw about how generative AI or AI is like, teenage sex. Do you have that?
Caleb Ochs:Oh, yeah, I can say it. I think this...
Brick Thompson:This is not in the Salesforce report - haha!
Caleb Ochs:Yeah, "AI is like teenage sex. Everyone talks about it. Nobody really knows how to do it. Everyone thinks everyone else is doing it. So everyone claims that they're doing it."
Brick Thompson:We're definitely seeing a lot of that right now. We do a lot of reviews on a monthly basis, or even weekly basis, of potential generative AI analytic systems. And there are a lot of people claiming they have it, and we're not seeing it yet.
Caleb Ochs:Yeah, right.
Brick Thompson:There's stuff that's sort of scratching at the edges of it, but not really doing it well, yet. That will come, though.
Caleb Ochs:Yeah, I think so. Yeah, I mean, this one isn't really new. But you know, data quality is still a top priority for people. Obviously, you need to get that cleaned up, I were just talking about that's really important. Just the amount of data is kind of exploding. And I think what's interesting to me about that, and what are they saying here? They say "surging data overwhelms users" is that when, when you do have a lot of data, and I think it's becoming more and more common just getting capturing data. We think of how many tools are out there that do that, and how easy it is just a sign up for something right. You might just sign up for monday.com to track your projects or something, and now all of a sudden, you've got all this new set of information inside of a new system. Now there's so many different places to get it from, you don't even know what an API is or how to get it out of there. It does become a big problem and barrier for especially for something like a generative AI because the first thing we're gonna see is it can connect to like a lake house or a data warehouse or something right and do some stuff there. Connectors to all these different systems and API access and stuff is going to be down the road later.
Brick Thompson:And we'll see that improving over time. I mean, there's already a lot of connectors. We find a lot of the sort of standard connectors that are out there, though, don't cover the edge cases that often crop up when we get into a client's data. I'm sure that the AIs will help with that. I'm sure the connectors will get better. I mean, everybody's seeing this same opportunity and problem. But right now, you need to get in there and make it happen yourself.
Caleb Ochs:Yeah. And then also, even if they can do that, you still have the data quality issue, right? I guess that does beg the question, you know,(we've touched on it in many episodes before this), how do you clean up your data quality?
Brick Thompson:Yeah, right. How do you?
Caleb Ochs:Well, I think some of the techniques that we take is you do start building reporting on it. Reporting is more than just analytics. It's also you can report on your data quality. Right? And that will help you clean up. You can get those reports into the hands of the people that are responsible for the data quality, and actually, some of the people on the front lines that are inputting the data, make that part of their KPIs is how good their data quality is, and it'll
Brick Thompson:Yeah, yeah, definitely. Yeah, that's a good clean up. point. You know, another thing that came up in this Salesforce report was that a large percentage of IT and data leaders say that their data strategies are disconnected from business goals. No one said(actually 1% said they're completely not aligned), but something like 40%, say they're only partially aligned. That is difficult. One of the challenges, for sure, of getting them aligned is to make sure you have access to good data, so that you can start figuring out how to use it well. It's not as simple as you might think. It's tempting. You get groups of business people together, start talking about what they're going to use their data for. There's a big wish list, usually. And there's often pretty low value stuff in there. And it can be tricky to get to what is the important stuff here to align reallyaligned with our strategy to drive our business?
Caleb Ochs:Yeah, we do that. Because right. I mean, that happens every time. There's a lot of ideas. And it might just be kind of flavor for the week, depending on when you're talking to these people. And you have to have a way to kind of sift through that and really get at the initiatives that are attached with business goals, and explicitly call that out. That will help your initiative succeed, and not lose steam, right. So the way that our teams do that (our customer success teams) is they'll have an exercise where they do, just get everything out, just get everything out. And then we come back around and start talking about priorities, start talking about what does this actually do for your business? Start asking those questions, and then we get a nice prioritized list of initiatives that we just start making progress on right away. So, it doesn't have to be overly complicated how you do that attaching to overall business goals, it could just be a matter of doing an exercise like that.
Brick Thompson:I think there's that. And then I think also (as we've talked about in the past), expecting to iterate. So do that, get your prioritized list, start knocking those off quickly. Don't do a waterfall project of, "okay, we're gonna produce all this great reporting in the next few months." Start producing reports in a week, start getting them into business leaders' hands, and then really start figuring out where they're delivering value and really aligning with their strategy. I found another interesting stat in here around data quality. It's interesting, in businesses 57% of data and analytics departments in the companies surveyed, say that they're completely confident in their data accuracy, so 57% of the data people are completely accurate. The Business People not quite so much. It falls off pretty quickly. 42% of the sales people, 40% of the service people, 45% of the marketing people are counted. So there's a there's a disconnect there. Why do you think that is?
Caleb Ochs:That's a really good point. If I had to guess, it's the difference between macro-level and micro-level. Like I bet you a salesperson or someone that actually has ownership over a smaller chunk of data is going to point out an issue that maybe the macro-level leaders like not gonna see it. Yeah, yeah, they might not see or think it's important. So they don't really think that's a data quality problem. When in reality, too many of those, it really is. It really does become a problem. Yeah. So I would think that the people that you mentioned there later are probably more on the money than, than the others.
Brick Thompson:Yeah, I think that's probably right. Data folks may be a little overconfident.
Caleb Ochs:Yeah, right.
Brick Thompson:At least as it relates to the business folks, and the business folks are their customers. That's who needs to be confident in it, or they're not going to use it. They're gonna go around, and they're gonna have shadow BI, and they're gonna have all those problems that we've talked about in the past.
Caleb Ochs:Or maybe it's that the data people are like,"That's the data. It is what it is. That is accurately reflecting what's in the system."
Brick Thompson:Oh, that's interesting.
Caleb Ochs:But the business users like, "Yeah, but it's not right for the real world. Like it doesn't...
Brick Thompson:because of process issues, or... Yeah, right. Interesting. All right. Anything else you wanted to add?
Caleb Ochs:I think that's good.
Brick Thompson:We'll wrap this one up. Thanks.