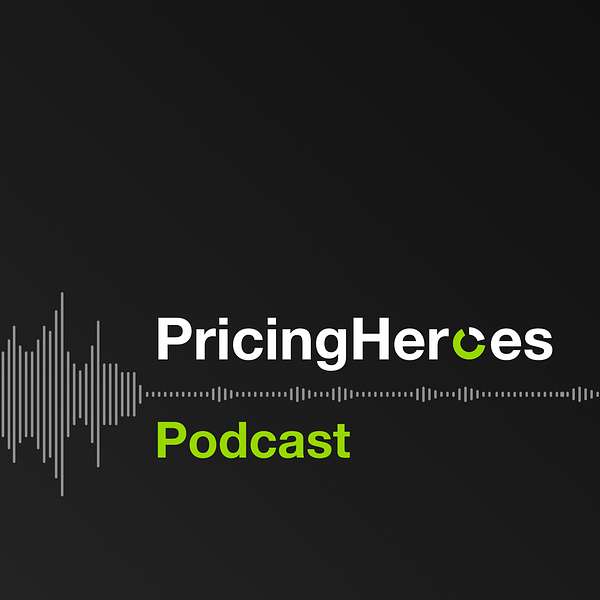
Pricing Heroes
Pricing Heroes: The Retail Pricing Podcast for Practitioners and Executives
Your go-to pricing podcast for transforming strategy, boosting margins, and leading with confidence.
Pricing Heroes is the leading retail pricing podcast for pricing practitioners and retail executives focused on building smarter strategies, protecting margins, and earning customer trust. Each month, we feature exclusive interviews, case studies, and practical insights to help you implement the most effective pricing strategies for today’s retail environment.
Whether you're managing promotional calendars, navigating price perception, or scaling AI-powered pricing systems, Pricing Heroes offers the expert guidance and real-world perspective you need to lead with confidence.
🎙 Each episode includes:
- Expert Interviews: Candid conversations with top pricing professionals and retail innovators
- Case Study Analysis: Behind-the-scenes strategy breakdowns from across the industry
- Actionable Takeaways: Practical insights you can apply immediately in your organization
We explore the full landscape of pricing in retail — spanning e-commerce and in-store, global brands and regional players, and categories from fashion and grocery to electronics and beyond. Topics include:
- Innovative pricing technologies and emerging trends, like AI-powered pricing platforms
- Data-driven consumer behavior analysis
- Strategic solutions to complex pricing challenges
- Tactics to boost profit margins and market share
- Pricing topics making headline news
- Building and transforming pricing functions
Join a growing community of pricing professionals and industry leaders who tune into Pricing Heroes — the trusted pricing podcast for anyone shaping the future of retail strategy.
🗓 New episodes drop the last week of each month.
📲 Listen on Spotify, Apple Podcasts, Google Podcasts, or your favorite platform.
Pricing Heroes
Data-Based Decision-Making in Retail & E-Commerce with Dr. Markus Husemann-Kopetzky
In this episode of Pricing Heroes, we sit down with Dr. Markus Husemann-Kopetzky, founder and managing director of the Price Management Institute, a boutique consultancy specializing in price management and price communication. As a senior research fellow, university lecturer, and advisor with more than a decade of experience in management and consulting roles, including over five years at Amazon as a senior manager of pricing and business analytics, Markus brings unique insights from both academic and practical perspectives. Some of you may know him as the author of the Handbook on Psychology of Pricing.
We invited him to discuss his latest report: “Data-based decision-making in retail & e-commerce,” which compiles actionable insights from a survey of more than 200 retail executives.
Recommended Resources
For pricing professionals looking to deepen their knowledge, Markus recommends:
----------
Get your free copy of Get Ready for the Future Of Pricing with our A-Z Guide.
For more information about AI pricing solutions, visit Competera.ai.
Aaron: Hello and welcome to Pricing Heroes, a podcast sponsored by Competera. This is a series of interviews with best-in-class retail pricing experts driving bottom-line metrics for major retail brands and the industry as a whole. Today's guest is Dr. Markus Husemann-Kopetzky. Markus is the founder and managing director of the Price Management Institute, a boutique consultancy for price management and price communication. He's also a university lecturer and advisor. Some of you may know him as the author of the Handbook of Psychology of Pricing. Markus has more than a decade of experience working in management and consulting roles in financial services and retail, including more than five years at Amazon as a senior manager of pricing and business analytics. We invited him on the show to discuss his latest report on data-based decision making in retail and e-commerce, which compiles actionable insights from a survey of more than 200 retail executives. Markus, welcome to the show.
Markus: Thank you so much for having me.
Aaron: Before we begin to discuss your recent report, can you please share a little bit about yourself and how you came to work in pricing?
Markus: Yes. If somebody has a topic they're passionate about, for me, it's pricing. Fifteen years ago, I started my doctoral research on pricing, published academically, wrote a couple of books, and worked in consulting. After a couple of years, I thought explaining how things work is nice, but maybe it would be better to actually do things.
Then I joined Amazon for five and a half years. At Amazon, you typically switch roles rather quickly, like every 18 to 24 months, but I never did. After three years, I was the most senior pricing manager in this role and was responsible for very interesting projects across Europe together with colleagues in Seattle. I had dotted line teams in India, and it was really an exciting time, but after five and a half years, it didn't get more exciting.
At one point, I had to decide: either keep tweaking the last algorithm at Amazon, or with a sense of patriotism, help the European retail space. Then I stepped out and started my own company. When you leave a company where all decisions are based on data and all data-based decisions are automated, you find yourself in the real world, and then it gets exciting again.
Aaron: Did you find the transition from academia and consulting to the actual practice of pricing challenging?
Markus: No, not at all. I was really excited about seeing how your concepts apply after you actually leave your consulting project. I have a very strong sense for data, and I found myself putting in the SQL code and getting the data and training your models. It was really fun. It's like building something, not just painting the blueprint of how it should look, but then you build it yourself and it actually works. That's really nice to see that your plans work out. That's really fulfilling.
Aaron: Yes, I think going from providing guidance to implementation in practice is where you actually get to see and own the results yourself.
Markus: Yes, indeed. And when I stepped out of Amazon, I found myself in a consulting role again, but trying to implement those concepts we know and develop isn't as easy as in more data-based companies. I always had the gut feeling that decisions are made on gut feeling in other companies. To get more data around this gut feeling of gut feeling-based decisions, I created a study that I'll probably share today with more than 200 executives about how they base their decisions and how data-based their decisions are. This was really revealing. So I practiced what I preach and got data on my gut feeling. It was really insightful, and now I try to get the message out there to do more data-based decisions.
Aaron: Since you mentioned the report, how about you tell us a little bit about it and what inspired you to conduct this research?
Markus: I had a couple of conversations about how to set prices with executives and senior executives. The decisions or the discussions that we had were partially disappointing. They doubted that data-based decisions are actually helpful beyond the expertise that their category managers had. Of course, I talked a lot to people who believe in data-based decisions, but a surprisingly large number of people just don't do this. And I said, okay, this is not the right time to convince with my social skills—we need to get more data around this and bring something to the table that is hard to reject because it's black on white. And yeah, I just had to do this report.
Aaron: You know, when you talk about people emphasizing the importance of data-based decision making, but you have this feeling that people are not actually implementing it, it makes me wonder how self-aware a lot of these teams are when it comes to whether or not they use data. Because I often hear someone say, "Oh, I leverage data to make these decisions." And at the same time, when you look at the outcomes for their processes, you're not entirely sure that they are using data. And so you ask them maybe to provide the insights: what is your source of this data? How are you analyzing it? How are you leveraging those insights and implementing it to improve the results? Have you found it challenging to bridge the gap between the perception of leveraging data and the actual implementation? And how have you been able to do that when you speak with these decision makers?
Markus: I think the discrepancy from this study and the conversations that probably both of us have when we talk to others is the following: If you talk to somebody and ask them about the way they make decisions, of course, they will claim they put some intelligence and objectiveness into the decision making and don't say "I apply rule of thumb or my intuition," because this feels like doing something wrong.
But if you sit in front of a market research survey and you are alone, you can actually admit—believing that what you put in there remains anonymous—and become a bit more honest because what you have in social interactions also has kind of a social bias. Nobody wants to admit it's not the way it should be, unless we have a strong, trusting relationship.
And I think that's the reason why we have this discrepancy. Otherwise, we wouldn't have it, right? If our companies would have the data-based decision making in place that they claim, they would give it into the report. Given that the report falls below what we learn in conversations, I think that's a bit more correct, honestly.
Aaron: That's really good. I think it emphasizes the need for doing internal audits around data readiness and decision making process. If you have this discrepancy, it's sort of a red flag to wait a second, we need to pay attention to what's going on here and really dive in and find where those gaps are in decision making. In the report, you identify what you call Data Kings versus Data Laggards. Could you explain these categories and the performance difference you find between them?
Markus: Yes. What we did is the following: We asked all 200-plus participants with a standardized scale to rate the degree to which they make data-based decisions, and we created a score. Then we took the top 25 percent and called them the Data Kings. So they are the more data-based decision makers. The bottom 25 are those companies that do the least data-based decision making.
Then we compared both groups—the Data Kings and the Data Laggards. And the question that guided us was: Do we do data-based decision making just for the sake of it, or does it actually have an impact? We asked all participants in the study to rate their company's performance relative to key competitors.
Previous studies revealed that the perception of executives on their relative performance to key competitors is strongly related to the actual performance. And as we don't have the financial statement data, we rely on this perception. And we found two really interesting insights.
First, Data Kings have a systematically better financial performance than Data Laggards. The financial performance we measured had four different dimensions: We measured revenue, revenue growth, profit, and profitability. And we found Data Kings are better than Data Laggards—not surprising. But what we also found is that Data Kings perceive they have a systematically better financial performance relative to key competitors. So this means Data Kings have a competitive advantage. And this is really insightful. So it's not nice to have, it's actually a must-have.
Aaron: Were there specific trends, frameworks, processes that you found that differentiated the Data Kings from the Data Laggards? What were they implementing specifically that differentiated them?
Markus: A key distinction between both was the degree to which senior management enforces data-based decision making. For the Data Kings, it was most of the time that senior management wants to have data-based decisions. Otherwise, they just wouldn't let them get through. And the Data Laggards had this kind of seldom in the ranking. So it all starts from senior management. You need to be a role model in doing so. And this was a key distinction between Data Kings and Data Laggards—the degree to which senior executives enforce data-based decisions.
Aaron: Then it was more about leadership than it was the roles or tools that they were using?
Markus: We did not measure specifically the composition of teams because this is a function of the data-based decision making per se. We didn't want to confuse cause and effect. So those who place more weight on data probably have different teams in place, and we don't want to show that you need to have these teams to have more data-based decision making. I believe it's the other way around.
If you believe in the power of data, you spend it on teams around this, but we included the degree to which senior management starts to enforce these data-based decisions because data-based decisions do not create senior management to enforce it, but only the other way around. And that's why we included this question in there. If you want to have a starting point somewhere, probably starting from senior management is the point where you have the highest leverage.
Aaron: That makes sense based on the conversations I've had, especially when it comes to change management and pricing. There tends to be an emphasis on enablement from the top. It's not necessarily a top-down decision, but it's definitely the beginning of the process from the top and enabling your teams to implement new technologies or change processes or focuses and values to even emphasize the importance of data-based decision making.
Aaron: Okay, so let's focus on pricing specifically. Your study revealed that 56 percent of companies consider data-based pricing crucial for their pricing success, but only 17 percent strongly agree that they have sufficient technical support to achieve it. What specific technical challenges are preventing retailers from implementing data-driven pricing?
Markus: My perception is that it's not the technical restrictions that prevent them, but it's the limited attention to get these technical roadblocks out of the way. The key problem is, from my perspective, that the internal teams cannot make the business case very clear that it is worth investing into pricing and into AI specifically, and to allocate the resources in this way.
And of course, there must be roadblocks. Otherwise, if it's easy to implement, they would just do it as a low-hanging fruit if they believe it makes sense. The key problems are usually the different places where you find the data that you actually need.
So you have a place where you have your transactional data, and you have a completely different place where you have your product data or your PIM data. The stock data are somewhere else, competitor pricing data, if you have them, are of course also somewhere else. They don't belong usually to the normal infrastructure because competitor pricing data are only used for pricing, the rest for other use cases.
Then the question is, okay, where do we have my promotional data, like the promotional calendar? Where do I find my seasonality indicators? Where do I find the stock data or the availability data of my competitors? And all these data are all over the place. Even for your internal use cases beyond pricing, you sometimes just don't get the data together.
And that's really a big problem. It's not so much a problem to implement, for example, external pricing software, because the business model of external pricing software is to be very adaptive, so you can usually plug in everywhere. But internally getting the data together is a huge problem. Yes, and then if you have a business case that everybody believes in, and you have a plan for how to get your data together, at one point you need to decide: make or buy.
And for some reason, retailers tend to overestimate their internal capabilities and look too deeply into the make decision. And when they start to work on the make decision, they usually find themselves in the rabbit hole because nobody on their team has ever implemented pricing software.
And then the first project on pricing becomes a bad reputation because it usually takes longer than expected and delivers less than expected. And so the second project probably has a bad start, and it makes companies reluctant to start a second round. But it all starts with making the case clear—pricing is worth investing in particularly.
Aaron: That makes a lot of sense. I've worked for companies like SAP EPM and some smaller services companies that specialize in either building custom software or implementation. And it's a constant struggle when you have an organization that believes they can actually build rather than buy at a cheaper cost when in reality, not only having the technical capacity and the capacity to build and then support, but also having the knowledge of implementation and integration across the organization—sometimes you just really need someone who's done this many times to guide you on that process. Otherwise, you throw good money after bad and it becomes this bloated project that doesn't deliver any value when bringing in the right people with business expertise can really have a significant impact on the project and guarantee the success of the implementation.
Markus: Yes, I totally agree. And from a commercial perspective, there are two cost components that usually get overlooked. The first one is development costs. If you work with a professional pricing company, their development costs spread over the whole customer base. Second is SDEs, software development resources. They are limited. So when you put in the hours or the FTEs into a pricing software project, you don't invest them in something else. And if you can buy kind of a standard out there that is proven, why shouldn't you do it? Nobody is programming Virtual Excel themselves because there are standards out there.
And it's usually more beneficial to put your SDEs on the project that gives you a competitive edge and not try to program software that's already existing because you think you can make it cheaper and better.
Aaron: In addition to the maintenance cost, right? Managing software is challenging in and of itself.
Markus: You're absolutely right.
Aaron: So, you found that transitioning to data-based decision making typically takes 12 to 24 months. What are the key milestones retailers should target in their pricing transformation journey?
Markus: From my point of view, they should go very small steps in a conscious way. I make it black or white—either they don't embark on any journey, but if they do, it somehow started with, "Oh, I met this guy from this company and they promised me a margin uplift of 200 basis points. We should look into this software and implement it."
Of course you can do it and probably sometimes that's successful, but you never know whether any other solution would be better. So I propose actually seven steps. The first one is, depending on how you frame your project, you start either with a long or already have a short list of options. I'm not talking about vendors, but usually it's vendors. I take personally, they make option always off the table.
Then you have a process that leads to the final candidate of one or two software solutions that you use for your MVP—that's your second milestone. So you consciously make a decision, let's go with them, and this is the reason why. Not gut feeling. The third milestone is usually the MVP phase was successful. So you have also designated phase and criteria for what determines whether it was successful or not, also consciously decided.
Then you usually have after the MVP, also a lighthouse rollout project for a pilot product segment. This lighthouse project is not 100% necessary content-wise, but to create a sense of excitement within the company. So whatever you learned during the MVP is put into the first part of your rollout plan and you start with a very successful rollout.
Then you have a longer period of up to 12 months where you actually roll everything out. So you have a rollout plan—that's a milestone and successful rollout. And the second and last milestone is your performance management and improvement is in place. How do you measure we are successful? It's not like we switch it on and now it's solved. The question is how do we ensure that we keep improving? And what is our mechanism here? So these are the seven larger milestones, I would say.
Aaron: I like that process because it's a slow implementation that allows you to be more agile in the development. And we all know that that is crucial. We don't have the time to implement something in full and try to make the adjustments afterwards. At Competera, we pride ourselves on doing the MVP and the pilot in order to make adjustments very quickly on a very short time scale. We're talking weeks, not months or years.
Markus: Yes, old school waterfall approaches. And that leads to what you said, throwing good money after bad. The agility is really key here.
Aaron: So of all the business functions studied, pricing showed the highest potential for AI implementation. Why do you think pricing stands out as such a promising area?
Markus: I was really surprised that my personal intuition that pricing might be a good starting point for AI projects was confirmed. So what did we do? We looked at pricing, but we also looked into forecasts and projections, assortment management, promotions, marketing campaigns, the annual purchase price negotiations, and product placement and presentation.
And we asked actually three questions for each of these: How important is AI for decision making or will be important for decision making? Are you using it today? And do you invest sufficiently into this? And we measured the gap between "we use it today" and "it's important" as an implementation gap. And we measured the difference between "we invest sufficiently" and "it's important" as the investment gap. And we found that both gaps are largest for pricing. And by the way, pricing was considered the most important use case across all seven decisions.
So when we asked the first question, how important is it, it received the highest ratings. So our 200 executives rated pricing to be most important and agreed that they don't use it sufficiently and do not invest sufficiently. And that makes it a very good starting point.
Why is that? Okay, if you look at all the other six decisions, pricing has the most immediate impact. So actually, you can change pricing from today to tomorrow and each transaction delivers you whatever you changed in pricing. So pricing has the most immediate result. And my personal experience is that AI-based pricing projects pay themselves within the first 12 months. So if you start early enough in your financial year, you end with incremental positive impact within this fiscal year. And that's why pricing should be a good use case because of the short-term effect and the large magnitude on bottom and top line.
Aaron: There are two reasons that we hear as obstacles to AI adoption. The first and foremost being a lack of trust. There's a misunderstanding of what is capable and what truly happens with AI pricing. Executives tend to think that there's this black box that you put data in and you have no idea what's coming out and whether or not that is actually going to be effective for retail X, Y, and Z's pricing strategy. And then the other issue that we see is a lack of data readiness, which is relevant given your report. So I'm wondering, are you hearing the same things and are there other key obstacles preventing retailers from bridging this gap between the need for implementing AI and the lack of investment in it?
Markus: Assuming they understand the need for better pricing, what are the obstacles in this kind of project? And they totally relate to your trust topic. Today, the category manager uses, for example, Excel to create sheets to set prices based on gut feeling. And if you take this away, they lose a sense of purpose.
And the projects that we are running are usually starting there. So instead of framing it as "a machine takes over," it is, "Hey, you have so much to do. Why should you waste two hours a week with Excel?" No, we do the following: We start with automating pricing decisions. First, whatever you have in your head, teach this to the machine in decision trees, for example, and you will always be able to recalculate the prices and check whether they are correct as if you would have done it yourself last week.
And once you develop the trust that you can give a machine the authority to make the decisions and you know they are right, then you move on to optimization where you only set the guardrails and make the machine or neural network sets the price in between at an optimal level. And you also teach the algorithm, "Okay, this week we focus more on profit and less on revenue growth" or a better trade-off between both. So you are always in the driver's seat.
But if you tell them, "Hey, tomorrow, a black box makes a decision—and by the way, you remain responsible for your P&L," it won't work. So you keep them in the driver's seat. The question is, why do we start on this endeavor? Because they probably connotate retail AI pricing projects with a black box.
Markus: But actually it starts with data-based decisions that become automated. And then you make the methodology a bit more sophisticated with AI. But the starting point is probably pricing automation. And turning discussion from "this price is wrong, it should not be 12.99 but 11.99" to the rule or the mechanism that led to the price. Teaching people to think in these mechanisms is probably more helpful. So probably AI-based pricing is the wrong term—too far ahead of where they are now. So you should probably talk about pricing automation or pricing processes or pricing mechanisms or something like this, which remains closer to the decision maker and not too far away.
Aaron: I think that's where something like A/B split testing can really come in handy because you're able to demonstrate the efficacy by having a test and control group running through the process to actually demonstrate, when we use contextual AI pricing for this product category between this period of time we can actually show the uplift in revenue and profits. And this is how the overall basket value increases—you get a lot of data. So we are in a time where we can measure these performances at a very precise level and actually adjust to see what effects these changes make. So perhaps going through the process of demonstrating the value, showing it with data so that they're not having to make a gut feel when it comes to purchasing a product itself that's supposed to help them become more data driven—even the decision making around the implementation should be data driven itself.
Markus: Yes. This is actually the first step that I would recommend—turning gut feeling decision on implementing AI-based pricing software into a data-based decision on data-based decision making in pricing. And the A/B test during the MVP phase is a very sexy way how to do it. It's objective. Nobody can argue.
And then the potential uplift is on the table, and then whatever reason you have to object to this project, they should be stronger than the financial impact that is on the table. So you show them what is the uplift that you would otherwise lose if you decide against it. And if you don't have this number, it becomes easier to be against AI or data-based pricing decisions. But once you see what is at stake, it becomes a better decision or a better data-based decision on how to implement it or whether to implement it.
Aaron: So I'm wondering, could you share some examples of companies that have successfully transformed their pricing from gut feel to data driven and what measurable improvements did they achieve?
Markus: Yes. I think about a market-leading non-food retailer with a very strong brand position. Over the past years, they managed their financials by raising their prices because they had a better brand position than competitors with a lower brand perception. But at one point you cannot do this anymore, right? Otherwise, you hurt your price image and then it becomes really a problem.
They thought, "Okay, maybe we need to implement some more intelligent and data-based pricing decisions." And they did. And what happened was quite counterintuitive. So for items for which a good price is very important from the perspective of the customers, the so-called KBIs, the retailer lowered prices and reduced the gap to competitor prices by about 30%.
So they lowered prices. But on the other hand, the overall margin increased by raising prices for other items where a good price is not so important for customers—they are happy that you have this product. And overall, they increased the margin by 1%. So they became more profitable, although they became also more competitive. And this is a very nice example of how to set up product prices in a very intelligent way. And just to call it out, pricing is all about de-averaging. And that's what they did—completely different price level, relative price level to competitors, depending on the product segments. And that was an interesting example here.
Aaron: Looking ahead, what developments in data-driven pricing do you expect to see in the next few years?
Markus: I think we will find a stronger individualization of price calculations. So we saw that for a large assortment, we have very different relative price levels and we calculate prices depending on the article role and the time of the year and what the interdependencies are to other items that go into the same cart, but probably this is also a function of customers.
Of course, we don't want to have differentiated prices for the same customer at the same time, depending on whether you use your Android or Apple phone—that's a problem. But probably you have regional differences. And in some areas, people are more affluent and in others, they are less. And at one point you should be able to set probably store-level prices that cater for these individual differences.
And what I see right now are either country-level prices or pricing clusters like, these 100 stores belong to one cluster and they received the same price because they are sufficiently similar. But probably if you have a network of three and a half thousand stores and the composition of people around there are completely different, it might make sense to have different prices for each store, depending on the buying behavior of the customers—not only their purchasing power, but also their product preferences.
If you have a store in a neighborhood where the average person living there is 60-plus, you probably don't have any school kids anymore that buy peanut butter. So peanut butter probably is less important, and those who want to have it because it turns on childhood memories, they are happy to buy it. And for others, it's kind of a commodity—they just need to have it probably every week, and you can offer peanut butter at different prices.
So a strong individualization, probably for example regionally. And second, talking about individualization, I think we'll see a better fine-tuned interplay between prices and promotions and coupons, for example. So coupons are a good example to individualize prices without making people feel they're being taken advantage of—they don't see they receive a higher price than others, they just get a lower price than others. And as everybody gets different coupons, nobody knows who is better off. Usually you have an affinity score, so that means each customer receives coupons that are best for them.
Markus: Turning to the interplay between price and coupons and fine-tuning this a bit better or balancing those will become one of the future developments. And lastly, in particular in e-commerce, you have a couple of decisions that you make simultaneously. For example, in performance marketing, you decide how much you bid to get traffic into your store. And the more you bid, the more traffic you get. On the other side, you have to make pricing decisions, and the lower your price, the higher your conversion rate.
So with your traffic coming in times conversion rate, you have a number of transactions. So you have to decide whether you bid one more or you lower your prices by one. And there is an optimal trade-off between both. Given the money at stake and the currently high cost for performance marketing, we will probably have a better interplay or balancing between these decisions in the future.
Aaron: I really like the personalization aspect. And I appreciate that you made a distinction between personalization from a regional perspective and personalization through coupons or special offers that target specific individuals. Because I do think that there is benefit to targeting individual shoppers with opportunities to purchase products that they don't typically purchase with you. And that is a great way to do it.
Typically, you know, coupons are presented broadly and someone can leverage this, whether or not they're buying the product. And that doesn't make a lot of sense because if they're going to be spending money on that product regardless, you may not want to provide them with a coupon. But at the same time, you don't want to be providing coupons for items that the person is not particularly interested in because then it's pointless. So understanding your shopper and then being able to target them, perhaps with an item that you know they would want to purchase at your store, but maybe they choose not to because they are purchasing it at a better price somewhere else. And so you can use this to pull them in, build that brand loyalty, and ensure that overall there's satisfaction and a greater willingness to shop with you.
Markus: I could not agree more.
Aaron: So final question, what books, podcasts, or other resources can you recommend to our community of pricing professionals?
Markus: Today I brought two books and they perfectly fit to what we're talking about today. The first one is "Getting Price Right" from Gerald Smith. This is about psychology behind pricing, but not how consumers perceive prices and odd numbers or so, but about the psychology of those who set price and manage prices. So we talk about data-based decision making—this is a good book to learn about your biases as a price setter, for example.
And once you decided, okay, let's go into data-based decision making and where can I find something that teaches me or to get my feedback beyond Excel-based pricing, I like this book, "The Expert Guide to Retail Pricing" by Kiran Gange. I don't know whether I pronounce it correctly, but this is also a good book to learn about retail pricing and how to use data to do it. That's a very good introduction.
Aaron: I will make sure to link those in the show notes as well as a link to your book and your recent reports. So Markus, thank you for being on the show and sharing your insights with us today.
Markus: Thank you so much for having me. It was a real pleasure talking to you, Aaron.