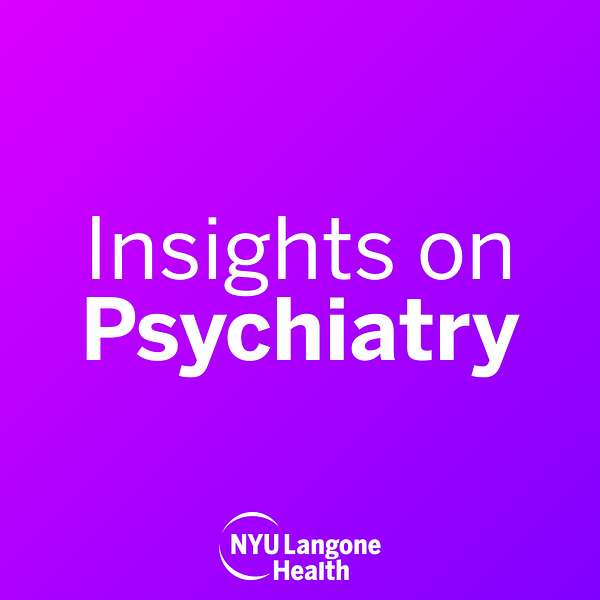
NYU Langone Insights on Psychiatry
A podcast for clinicians about the latest psychiatric research. Host Thea Gallagher, PsyD, of NYU Langone Health interviews world-leading researchers about advances in their respective fields, gaining insights that clinicians can apply today.
NYU Langone Insights on Psychiatry
Precision Psychiatry (with Ronald Kessler, PhD)
Dr. Ronald Kessler is the McNeil Family Professor of Health Care Policy at Harvard Medical School. His groundbreaking work on the social determinants of mental health, studied from an epidemiological perspective, has made him the most widely cited psychiatric researcher in the world. In this wide-ranging conversation, he talks about precision psychiatry's enormous potential and incremental development, delving into his own efforts to better identify at-risk patients and predict treatment efficacy. Dr. Kessler stresses the need for better data and bigger studies, and envisions a future of AI-supported clinicians.
00:00 Introduction
00:53 Dr. Kessler's Journey to Precision Psychiatry
02:45 The Importance of Data
04:14 Risk Factors and Treatment Optimization
10:42 Successes and Challenges
13:23 The Importance of Baseline Information
23:46 Machine Learning in Veterans Health
24:27 Determining Suicide Risk
25:35 Interventions and Cost-Effectiveness
26:16 Esketamine Trials and Response Prediction
27:07 Risk Models and Comparative Risk Models
27:15 Insomnia Treatment in Military Personnel
29:36 Cost-Benefit Analyses
35:45 AI in Medicine and Patient Response
42:21 Future of Precision Psychiatry
45:51 Closing Remarks
Visit our website for more Insights on Psychiatry.
Watch this episode on YouTube
Executive Producer: Jon Earle
NOTE: Transcripts of our episodes are made available as soon as possible and may contain errors. Please check the corresponding audio before quoting in print.
DR. THEA GALLAGHER (00:00):
Welcome to NYU Langone Insights on Psychiatry, a clinician's guide to the latest psychiatric research. I'm Dr. Thea Gallagher. Each episode, I interview a leading psychiatric researcher about how their work is shaping clinical practice. Today, it's my pleasure to welcome Dr. Ronald Kessler, professor of healthcare policy at Harvard Medical School. Dr. Kessler is a giant in the field of medicine. His groundbreaking work on the social determinants of mental health has made him the most widely cited researcher in all of psychiatry. Now, he's leading efforts to take psychiatry into the era of precision medicine. In our conversation, we talked about why precision psychiatry has been relatively slow to arrive, what it will mean for clinicians and how he's using machine learning to identify at-risk patients and craft individualized treatment plans. Well, thank you so much Dr. Kessler, for being with us today.
DR. RONALD KESSLER (00:52):
My pleasure.
DR. THEA GALLAGHER (00:53):
Can you give us an overview of your current research interests, especially as they relate to precision psychiatry?
DR. RONALD KESSLER (00:59):
I'm a psychiatric epidemiologist. For many years, my work was purely community descriptive epidemiology, estimating the prevalence and correlates of mental disorders. I did look at treatment, how many people got treatment and barriers to treatment and stuff like that. I never did clinical epidemiological stuff. Clinical epidemiological stuff is by and large looking at what are the predictors of whether treatment works. That kind of thing is nowadays called precision psychiatry because there are a lot of fancy statistical methods to look at that. In the past decade or so, I've gotten involved in doing more of this clinical epidemiological stuff initially through my involvement in something called the Army STARRS Study, which is an ongoing, very large initiative with many different studies inside of it, of suicide among people in the US military. We started in, I guess 2011, a few years after the rise in the suicide rate among military people was first observed and were asked by the Department of Army to come in and see if we could have an outsider's perspective to see what's going on and what could be done.
(02:27):
Typically, psychiatric epidemiologists are never asked those kinds of questions. We're usually on the outside saying, "Hey, look, mental disorders are a big problem. Somebody should do something about that someday." Nobody really comes to ask us what to do. This was a unique, actually, really unique opportunity. Unique in the sense that the military is a big organization and it has an unbelievable amount of data in stuff I've done subsequently and where I spend most of my time now working in commercial health plans and in the Veterans Administration. We have medical and pharmacy claims data and procedure codes and test results. Sometimes we bring in data about the neighborhood where the person lives so you can look at social determinants. In the active military, we have IQ tests, personality tests, blood samples, performance ratings, criminal justice data, because the military runs their own police force.
(03:31):
We have child and protective services data, because the military runs its own social welfare. We just have this unbelievable wealth of information. I, for many, years found myself being very jealous of people in Scandinavia because they had all these registry data. Actually, now in working in the active-duty military, I have much more than they have in those registries. Because as I said, we have like say, IQ, when somebody joins the military, they get an IQ test. They also get blood samples taken, so we have access to that. We have personality scales because the military uses personality tests to help figure out what's the right occupation for the person when they come in. We started looking there at risk factors for suicide. That's really the first part of precision medicine, trying to figure out who's at risk of something, who's going to have the heart attack. That's the person we should be going and doing the intervention with.
(04:37):
Then subsequently, the real core of precision medicine is when we have treatments that exist or better yet, multiple treatments that exist as we do in psychiatry, not just to predict who's at highest risk, but who is highest benefit among the people who get a treatment. Who's the person that this treatment is most likely to help? Then if we do that in parallel for multiple treatments, we can splice that data together to use a technical term and say, which of these treatment alternatives is the best one for person number one? That could be very different from the situation for person number two. It all starts in developing these risk models for how well will this particular treatment work for this particular person. Then we do that in parallel for many people and many treatments and can fold it all together to try to come up with an optimization scheme for how to get the right treatment to the right person at the right time.
DR. THEA GALLAGHER (05:48):
It sounds like this method or these methods are possible when you have all these data points, which like you're saying, we happen to have in the military. Is it possible to do this work with the general population or are we going to have to really move toward collecting more data about individuals to even begin this precision medicine process? Or do we already have enough that we could start making some sense of what we already have?
DR. RONALD KESSLER (06:14):
Well, we certainly have enough data already to start looking at things. It's also the case that the more data you have, the better a job you can do. There's a trade-off of course, between the costs of the data collections and the benefits. I think that one reason we went down a rabbit hole in precision psychiatry is that the natural inclination is to say psychiatric disorders are real illnesses and we're real doctors and this is real biological stuff, so let's go do these biomarkers and we'll discover subtypes where we find these natural cleavages. There's not just a thing called depression. There's five depressions.
(06:58):
It costs an enormous amount of money to do that kind of stuff. It hasn't really panned out. That doesn't mean it won't eventually, but up to now, we spent an incredible amount of money on largely underpowered studies. The thinking being that, well, I can't afford to do this with thousands and thousands and thousands of people, so we'll do it with just a few hundred and then we'll get the signal and then we'll see what's going on. It turns out statistically that if you only have a few hundred people as many of these biomarker studies have, the noise that you get in the data overwhelms the signal.
(07:39):
In fact, you have an extremely high probability of coming up with what you think of as significant results that when you then go try it on a separate data set, it totally disappears, which is overfitting the data. Spending incredible amounts of money on underpowered, expensive studies is not really getting us any place. I don't really know whether that realization has sunk in as much as it should in the field, but we've spent an awful lot of time in what turned out to be not a very productive line of investigation. This thing of getting better measures is great, but we can't afford to do $10,000 specs for everybody to figure out whether a $350 intervention is going to be better or not. Just give them the $350 intervention and see if it works.
DR. THEA GALLAGHER (08:36):
It sounds like you're saying it's also which pieces that you pick that you find are relevant, because if it's too much, like you said, you're overfitting. Is it part of your work to figure out which pieces of data are relevant and important to be looking at?
DR. RONALD KESSLER (08:51):
Well, there's two pieces. There's the overfitting. When you look at claims data, say, and we're trying to predict who's going to have a heart attack or who's going to drop out of treatment or whatever it is we're looking at, we have literally thousands and thousands of pieces of information. If we have strong priors about what's important, we can pick a subset and look at them. Very often, it's the case that there are signals there to be discovered that you wouldn't have thought of previously. Sometimes it makes sense to look at thousands of pieces of evidence. In genetic studies, for example, that's done 500,000 SNPs you start with.
(09:37):
If you start out with 500,000 things, the chances of you finding something that looks significant is a certainty. Very often, you have these immense, immense genetic samples and they say there's something significant at the 0.01 to the minus 10th power. That's what you look at. The problem there is that you only end up getting really big effects. I think it is useful to look at large samples of predictors, but you have to be thoughtful about how you do it. You've got to figure out ways of doing your analysis to minimize the chances of overfitting. You also have to test your models in ways that allow us to discover overfitting when it exists.
DR. THEA GALLAGHER (10:34):
It seems like for things like cancer and heart disease, maybe they've come a long way with precision medicine. Why do you think precision medicine has progressed more slowly in psychiatry than other areas of medicine?
DR. RONALD KESSLER (10:48):
Well, it hasn't progressed all that much in heart disease. Cancer has progressed dramatically. There are a couple of reasons for that. One big one is there's an enormous amount of money in cancer research. Also, they have ready access to a lot of variables that are the core variables they need to look at. The other thing is that virtually every other branch of medicine, not just cancer, but the ones that haven't progressed so far either, have many fewer treatments than we have. That's a blessing and a curse in psychiatry that what you're interested in doing in precision treatment is to say, here are these range of things I can do, and is there a way of figuring out what the right one is for any one individual? If I'm in the business of fixing people's broken arms, there's not really much value in precision medicine. There's only one thing you do when you break your arm. You go to the emergency room and you get your arm set.
(11:56):
If you have depression, you can go to a minister or a social worker or a psychologist. There's all these things. Each one of those people have a range of things they can do. That makes it more complicated. In psychiatry as well, there's this dizzying array of things that we can look at. In the long run, when and if we get organized to march through this stuff, the potential value of precision medicine, it's much greater in psychiatry than other areas. Because it helps us with those much more complicated decisions. Not just complicated decisions, but because the mechanism of action is so different in a lot of those things. The possibility of getting something that works for a person out of that many different things is much greater than in other areas of medicine. You can try five or six treatments for depression, they don't work and come up with a seventh and it just, boom, all of a sudden, it works. You just don't have that option in places. There's only two treatments.
DR. THEA GALLAGHER (13:00):
Is part of this also because most of the way that we measure psychiatric symptoms is through self-report? Do you think that adds to the complexity?
DR. RONALD KESSLER (13:09):
Yeah. Being unclear about what the phenotype is muddies things, certainly. Back to why are we not advancing more than we should advance? I don't think that's a major reason. I say that because even though it's the case that there are a whole bunch of different measures of any mental disorder, when you look at treatment effects, you find pretty consistent results in trials where you're sticking with depression. If you have the Beck scale or the Zung scale or the CES-D or whatever, if you find a treatment that works, it works. The fine grain of exactly what the effect size is going to differ. I think the signal is going to be there. The real challenge is you have to get good predictors. We have pretty abysmal predictor sets in most studies that are done of trials, precision treatment trials that have tried to look at does treatment A work better than treatment B? The simplest one.
(14:25):
There are many studies that have essentially looked at severity of depression, age, sex, three biomarkers that are pretty useless, and that's it. It's not all that surprising that we're not going to find much of anything. There's now enough studies, most of them done by psychologists because the specifiers that I think are most important at the moment are psychosocial ones. There are probably 75 different variables that have been found to be predictive of differential response. There's never been a trial. This measured all 75 of those variables. There's never been a trial that's measured half of those variables. The big problem is we don't have the right predictor set. The second big problem is that the sample sizes that we have are too small. We need big trials with rich sets of predictors. Once we have those two things, I think we're going to find that we can make progress in psychiatry equal to or greater than that in virtually any other area of medicine, despite the fuzzy phenotype.
DR. THEA GALLAGHER (15:52):
Is that on the horizon? Do you see that? Is that in the work you're doing or the work you hope to do?
DR. RONALD KESSLER (15:56):
Well, certainly in the work I hope to do. It's on the horizon, but it depends on how strong your binoculars are looking and how far you look out at the horizon. We are involved in several big studies in the Veterans Administration where we're looking at precision treatment for treatments for preventing suicide. One more, we're looking at treatments for depression. There's a big study that my colleague, Andy Nierenberg, is just starting with PCORI, looking at different treatments of depressive episodes among people with bipolar disorders. There's not that many medications approved for that. These are pretty massive undertakings. Andy's study, as I recall, is about $22 million. It's pretty much on the edge of being big enough. Anything less than that would not really work. It's a non-trivial thing to do serious studies of this sort.
(17:09):
If we start getting into the world of measurement-based care, which I think is on the horizon, things are going to be a lot easier. That's another, by the way, another reason why it's more difficult in psychiatry than in some other areas of medicine. You mentioned cardiology. Whenever you go to your doctor's office, they take your blood pressure, they take your temperature, but you don't get self-report scales of mental disorders, typically. Even people who go to psychotherapy or go and see a psychiatrist, it's not common for them to get administered scales on an ongoing basis to see how they're doing. It's harder for us to get objective measures of treatment response in routine data sets in ways that other areas of medicine have.
DR. THEA GALLAGHER (18:04):
What does that make you think about clinical judgment in clinical work?
DR. RONALD KESSLER (18:10):
Well, that doesn't make me... You mean other than the fact that why do clinicians have such poor judgment that they don't use measurement-based care? What do you mean? Say more about your question. I don't quite get what you're asking.
DR. THEA GALLAGHER (18:24):
I guess currently, there is a subjective element in psychiatry and psychology sometimes based on the clinicians assessing symptoms. Would you prefer that we move into a more objective way to measure symptoms? Then again, it sounds like some things that can't even be measured by a person.
DR. RONALD KESSLER (18:54):
Just focusing on the measurement-based care stuff, I think it is valuable to have objective data on symptoms that we chart over the course of time and look at, compare treatment trajectories of individual patients to norms to be able to say more quickly, we might, otherwise, whatever I'm doing is not working because the typical symptom course of a person who ends up remitting with the kind of treatment that I'm doing doesn't look like the course I'm seeing here. It does give us an opportunity to switch treatments more quickly than we would otherwise. There's standard protocol for trying something for X number of weeks, and if it doesn't work, we move to something else. That could be short-circuited. That's a piece of precision medicine too, since most people require multiple courses of treatment. I shouldn't say most, it depends on the disorder, but many people require multiple courses of treatment before they're helped.
(20:03):
The quicker we can, picking the right first treatment is really an important thing. Knowing how quickly to cut your losses when that first treatment is not working is an important thing. Figuring out what the right second treatment is an important thing, cutting your losses on the first treatment, that's where the measurement-based care comes in. If we can look at, here's this person, and there's quite a bit being done in trials now. Looking at interim kinds of measures that we get every one week, two weeks, three weeks, four weeks, to predict at eight weeks, this is pretty much doomed to a failure and I can see that at three weeks. Let's not torture this poor patient for another five weeks and move them to stage two right now. I think measurement-based care is useful there. I think the big thing is that we need to get better baseline information.
(21:02):
In trials, there's such a concern about making sure you can enroll enough people. The challenges of doing that, that very often, trialists will get a baseline measure of whatever the outcome is they're interested in. They're doing a trial of PTSD, so they give the PCL or whatever it is, and if they have that score and get some other very basic kinds of stuff and then do the trial. In the trials I'm involved with, we typically spend at least an hour assessing patients. Not just the symptoms, but I mean an hour of getting information about things that could influence treatment response before we start. That's a scary thing for people doing trials because the concern is are people going to sit through this hour and drop out? I guess my experience is that the people who don't sit through the hour are going to be people who are going to drop out of the trial anyway.
(22:06):
I'm involved in several big trials now where I've been always pushing for getting a richer, richer and richer baseline assessment. I'm involved in a trial now with Steve Holland and Vikram Patel in India and a cast of others, where we started out with, I think, around five hours' worth of data. We're boiling it down to probably two hours of a baseline assessment. Ultimately, we're not going to need two hours' worth of information. Probably once the precision treatment rules get developed, maybe it will be 20 minutes' worth of information we need to figure out what the right treatment is for that individual. The problem is, I don't know which 20 minutes out of my five hours is the right—you have to start out with something to begin with.
(22:55):
Then over the course of time, you can throw things away. I think that's a challenge. There's an understandable reluctance to do that. Then people have their own theories about things. There's lots of trials where people have their pet measure of cognitive something or other, and that's the only measure they have. Even though that measure could very well be useful, it is extremely unlikely that we're ever going to get to a point until we get some magic biological thing and measure of that particular kind of illness that we can do it. In the absence of that, it's very, very unlikely that there's going to be one measure we have that will be all we need to tell us which treatment to use. Big examples with rich baseline assessments is what we need.
DR. THEA GALLAGHER (23:49):
Speaking of a number of different measures, moving into machine learning, you've applied machine learning to veterans' health, including calculating risk for PTSD, suicide and even insomnia. Can you give us a little bit of an overview of that work and talk about what you've learned there?
DR. RONALD KESSLER (24:08):
Well, as I vaguely alluded to earlier, there are two kinds of models, basically. One is something called a risk model. We're interested in saying at the negative side, who is it among these? For example, we're doing some work right now among people who are inpatients, psychiatric inpatients, and we're interested in a suicide after discharge from the hospital. As you probably know, the suicide rate after discharge from a hospital is very high, and it's highest in the first week after discharge. It goes down over time. In the US population and in the Veterans Administration and in the Department of Defense, about 1 percent of the population is hospitalized a year. Those people count for between 12 to 15 percent of all suicides over the next year. Of those inpatients who subsequently go on to commit suicide, well over half the suicides occur in the 10 percent that are predicted to have high suicide risk by existing machine learning models.
(25:22):
We can say here are of the hundred patients who are in the hospital, these 10, that's where half of the suicides will be. The other half, we're not good at predicting. There are things that happen after they leave that we can't predict and so forth. There are interventions that exist, these intensive case management transitional services interventions that can be effective in reducing risk of suicide. We can't afford to give that to everybody. Just being able to target the high-risk people sometimes makes it possible for us to take something that we know has potential to be a useful intervention, but isn't something that we can cost-effectively give to everybody and target. A lot of what we're doing is that. There are lots of other areas in psychiatry where that kind of thing is being done. You're probably familiar with over the past decade since Esketamine came along, there's been a number of trials.
(26:21):
Who are the people who respond to that? Who are the refractive cases that do? It's probably 40, 50 percent of cases who respond to that. If we knew who those people were before we gave it to them, it's an expensive treatment, there are risk factors and so forth. Being able to do that is a useful thing. Those are the first kind of models. At the risk end, there's who's the person who's going to have the heart attack? That's the one I've got to do the intensive, or who's the person who's going to be at risk of suicide? He's the one I've got to do the special thing with. On the flip side, who's the person who's going to respond to this treatment? That's the one where that treatment is indicated. There are lots and lots of places where that comes up. That's the first kind of model, risk models. The second kind, as I mentioned earlier, is comparative risk models. I'm at the moment involved in some work in DOD and looking at, you mentioned insomnia, which is a big deal among military people.
(27:26):
They get up early in the morning, they have to do things in the middle of the night, stuff like that. The recommended treatment for insomnia is—there's a CBT for insomnia. There aren't a lot of people who know how to do that. It's relatively expensive. There's also all these Z drugs that are around which seem to be less effective, but they're a lot easier to deliver. We have been developing models to say, who are the patients that if they get a particular medication for insomnia, that's likely to be effective for them? We can actually do not a great job, but a lot better than chance. There's only about a third of people who do well, but we can say in this 50 percent of people, about half of them will do well. This other 50 percent, only 25 percent will do well. We double your probability of doing well.
(28:33):
For ICBT, there's more people who do well. It turns out it's a different profile of people. We've developed things for that. If you only have so many people who you can deliver this intervention to, here are the ones most likely to be effective. Then splicing those together to say, here's a person where medication is just as likely to work as the psychotherapy. It's a lot cheaper and it's a lot easier to get, so they should get the medication. Here's people where the medication is definitely not going to work, and the psychotherapy has a high probability of they're the ones who should get the psychotherapy. Those are the two kinds of models. First of all, when we don't have comparative treatments, we just have to do something versus doing nothing. Who are the people at risk? Then in cases where we have multiple treatments trying to figure out models to say, how do you get the right treatment to the right person?
DR. THEA GALLAGHER (29:36):
It sounds like you're talking about what works for, the profiles, what works for one person, what won't work for someone else, and trying to find what's the most cost-efficient manner to get them to treatment that they need.
DR. RONALD KESSLER (29:52):
Then cost comes in there as well. That's right. As I said, having a case manager, giving everybody a best friend that will come home and make supper for them is actually pretty, but we can't afford it for everybody. Figuring out how to juggle this thing. In the suicide world, that's a big thing. There've been a lot of concerns saying that these machine learning models are pretty useless because there's only 12 people out of a hundred thousand who will die by suicide. The high-risk people, maybe 200 out of a hundred thousand. Can you afford to give an intervention to a hundred thousand people? That kind of thing. We want to see if we can develop a model that has enough concentration of risk at the top that it's not just that, say the 5 percent of people at highest risk account for 40 percent of all suicides. That the risk of suicide in that population that they have 1.5 percent of people are going to die by suicide or 3.2 percent of people.
(31:00):
What is the intervention that if it had an effect of X and it had a cost of Y, I could afford to give to a group of people where only 1 percent of them are going to have the bad outcome anyway? It might be that the answer is nothing. There's just nothing that I could afford to do. If it's fluoride in the water, it costs a penny, I can do it. It turns out though that the answer to that question is not obvious. The critics, as I said, there was a whole exchange in JAMA Psychiatry and a couple of ripples and things in other journals about this critique of these suicide models because positive predictive value is so low. The proportion of people who are actually going to die by suicide is so low that it just isn't cost-effective. They were seat-of-the-pants ideas of saying, well, how can you afford to do that?
(32:03):
Well, in fact, if you sit down and think of it carefully, the proportion of patients in say these inpatient suicide prevention models that I mentioned, the proportion who end up dying by suicide over the next year at the high-risk level, it's a small number proportionally. It's not different, say from the proportion of people who would have a heart attack who are right now recommended to get statins. Seven per 50,000 or something like that. Why is that recommendation made for statins? Well, it was made literally sitting down by saying, here's how much it costs for a statin. Here's how many lives it's going to save per X. Something that we don't think of a lot, but you have to think about when you do this is how much is a life worth? How much are we willing to pay to save one year of life? The answer is $50,000.
(33:13):
The US government has a cost of a statistical life. In other words, they have values of here's what, and interventions, highway safety interventions of various sorts. If they are going to save X lives, they refer to those things. They say they save that many lives. Here's how many millions of dollars we can afford to spend. No, we can't afford to spend it. The question is, how much? A statistical life is worth $7 million, supposedly. I mean, if you just think of a hundred thousand dollars for every year of life, it turns out that the pensions that are the cost-effectiveness threshold, the optimal threshold is a lot wider than we think. This is a level of thinking about precision medicine that you see in the cancer world, for example, that you don't see yet in the psychiatry world.
(34:14):
There's something called decision analysis, net benefit analysis, where we build these things that we literally can say, if you could tell me all those things, how much does it cost? What's the benefit? What's the risk ratio? Blah, blah, blah. I can tell you exactly who to intervene with. When we have done those things, in a hypothetical way, so far, in our precision treatment models, it turns out that these things have a considerable value. When I say in a hypothetical way, we report these things. If you look at some of our stuff, we have net benefit curves across the range. The reason for the range is I don't have a particular treatment in mind all the time. That means I don't know how much it will cost because there's no “it” yet. I don't know how effective it will be because we haven't done it. I also don't want to prejudge what the value is of the intervention. How much is it worth to prevent a case of depression? How much are we willing to spend for that?
DR. THEA GALLAGHER (35:21):
It sounds like what these models are trying to look at, and maybe people might not want to hear that, but there has to be in society likely some sort of cost benefit. That's just the reality of the situation. It sounds like something that could make this really positive is if we could have some AI-supported medicine, so to minimize the cost. What's the response from patients to recent experiments with AI-supported medicine? What are your thoughts there?
DR. RONALD KESSLER (35:53):
When you say AI-supported medicine, you mean the patient can go and tell the computer their symptoms and the computer can spit back things to them?
DR. THEA GALLAGHER (36:02):
Yeah. Maybe both about their diagnosis and then, hey, here are some helpful treatment interventions. I know that CBT-i created a Somryst app. There are other things where they're saying, hey, this information is actually not terribly complex. It's accessible. A lot of CBT therapies are very accessible. What are your thoughts about ultimately using that?
DR. RONALD KESSLER (36:24):
Well, it's a brave new world, and we don't really know. There's a concern, but it's an exciting thing in psychiatry for a couple of reasons, because the reluctance of patients to ask or to tell doctors about these embarrassing things is a lot greater than in other areas of medicine. It's not the only place, people who have impotence or hemorrhoids. There are other embarrassing things. That's a good thing. There's a concern obviously, about medical misinformation, too. There's got to be a way of monitoring that. We've all heard about these, sometimes there are these crazy things that AI systems spit back at people. Those will become less and less common over time as these systems become refined. I think there's a lot of promise. The healthcare providers are freaked out by machine learning, which is much of it, AI in spades because it's threatening their hegemony.
(37:34):
Like any other kind of technology, I think in the long run, if we figure out how to work with it, it could be helpful. There was a paper a number of years ago in, I think the New England Journal of Medicine, about the resistance to AI in healthcare that talked about, it was written by a historian, a medical historian, and it was about the stethoscope. The idea that when the stethoscope was first invented, that there was this terrible resistance against it. Because it's like, I'm a doctor, I have trained ears. I listen to these things. I don't want this stupid thing. At the moment you think it was just a nutty kind of thing. That's very often the natural reaction to many, many things. You don't understand it. This idea that they're in these black box models. Somehow, it's a black box. I don't really understand what it's doing.
(38:31):
I kind of thought, well, an X-ray is a black box. I mean, how many people know about the physics of it? I know how to read an X-ray. Those kinds of things. There are growing pains, I think, to integrating new technologies whenever they come along. I think in the long run, AI has really incredible potential. I have a kid, I should say, who has a very rare autoimmune disorder. It took us 10 years to figure out what he had, and he's doing great now, which is wonderful. When ChatGPT first came along, my wife went to it and punched it and said, "What would you say if you had a patient who had this, this, this, this, this, this, this, this and not this, not this?" It immediately came back and said, "Oh, they have GA , blah, blah." It took us 10 years to get that.
DR. THEA GALLAGHER (39:28):
If we could find a way to have the algorithms work for us and still find a way to deliver compassionate care, but also, like you said, a lot of things that we use, an X-ray is impersonal in a way. It's, hey, if you're actually getting better and maybe we're using some impersonal ways to understand your symptoms and help you get better, I think people would prefer that over just therapeutic alliance, right?
DR. RONALD KESSLER (39:56):
Yeah. This is a little bit of askance, but as you probably know, during the COVID pandemic, the reluctance to have telepsychiatry fell by the wayside by fiat. Then seeing patients, seeing inside their homes, and so there's this visual stuff. Clinicians, all of a sudden, see the houses where their patients live, which they didn't see before. The kids running around. People all of a sudden discovered, gee, my no-show rate is going down. I thought the patients didn't like me. There's all these funny things that happened. The other thing that happened is that, which we don't really know a great deal about, but patients are willing to tell things to their mental health treatment provider over the internet that they don't tell them face-to-face. Because there's a certain psychological distance that will allow you to say something.
(41:07):
We know this from doing research that when you ask people about embarrassing things, the highest rates you get is when you go to a classroom and say, tell us about something rather, and fill out the form and don't write your name. Just drop it in a box over there. You hear about high rates of these embarrassing things. When you say and sign your name, the rate goes down. When you call them on the telephone to do the same, it goes down farther. When you show up at their house and look at them face-to-face, it goes down farther yet. There's a way in which psychological distance allows you to say things. Also, I can't reach out and touch you. There's a certain way in which the alliance you have with the patient, there's some things that you can have them say to you, but there's something missing as well. How do you get the good part without the bad part of that? There's that funny stuff. There are pluses and minuses of everything.
DR. THEA GALLAGHER (42:05):
It's worth the height of AI growing while we also have, according to the surgeon general, a loneliness epidemic.
DR. RONALD KESSLER (42:12):
Yes, that's right.
DR. THEA GALLAGHER (42:12):
That's how I think you navigate. Threading the needle of what we need is really fascinating and which I think you're trying to get at with precision medicine here.
DR. RONALD KESSLER (42:20):
Yeah, that's right.
DR. THEA GALLAGHER (42:22):
Well, for my final question, just what do you hope to see in the next five, 10 years with your research that clinicians could ultimately utilize too?
DR. RONALD KESSLER (42:33):
Well, we are, just my little group, but in general, this is happening, developing a number of clinical decision support tools that are starting to be put into place that we think are going to make the lives of clinicians easier, help in making decisions, maybe save lives. I'm right now in my next hour talking to somebody about, I think it just had come out, I think last month in JAMA Psychiatry where we had a precision treatment model of when somebody comes to an emergency department who's suicidal, what should you do? The standard of care is supposed to be that you hospitalize and you stabilize with the treatment plan, you discharge them. In fact, only about 50 percent of such people get hospitalized in America. Because not having enough beds and people resisting and so forth. We've developed a model which showed that there are about 20 percent of these patients in the Veterans Administration really need to be hospitalized.
(43:43):
If you don't hospitalize them, you're pretty significantly increasing their risk of being dead pretty soon. There's another group of people of almost equal-wise, which is interesting, where they really should not be hospitalized. This is sort of “DBT thinking” that the people need to learn how to, but the idea is that it hurts them to be hospitalized. It not only is characteristics of the patients themselves that predict that, but also characteristics of the environment. If they have good PACT programs or good intensive case management outpatient partial hospitalization programs in their community, hospitalization actually for those people is bad. Because there are these other alternatives. There's another 60 percent, makes no difference. It turns out that our simulation suggests that if what is currently done in VA was replaced with what we suggest, the suicide rate among people coming to emergency departments with suicidal would go down by close to 20 percent.
(44:49):
Interestingly, the number of people hospitalized would go down by 20 percent, which of the world we live in where there's a massive boarding crisis in many emergency departments, is pretty cool. I mean, you're sort of a win-win situation. We're in the midst of having conversations with the VA leadership in emergency medicine to put in place a demonstration project and some VAs where we try this out. If it works, then that would become something that's a protocol that we could do. Reducing the number of hospitalizations by 20 percent in that segment of the population and saving 20 percent more lives, not a bad thing. If we can get one or two or three or four of those things to become realities, I think that will jumpstart a realization that this world of precision medicine is something that has value for psychiatry, would sort of, I think, open the flood gates. That's my hope.
DR. THEA GALLAGHER (45:51):
Well, thank you so much for this conversation. Great information. We really appreciate you on the podcast.
DR. RONALD KESSLER (45:57):
My pleasure. Good talking to you. Bye-Bye.
DR. THEA GALLAGHER (46:00):
Thanks so much for that conversation, Dr. Kessler. If you enjoyed this episode, be sure to rate and subscribe to NYU Langone Insights on Psychiatry on your podcast app. For the Department of Psychiatry at NYU Langone, I'm Dr. Thea Gallagher. See you next time.