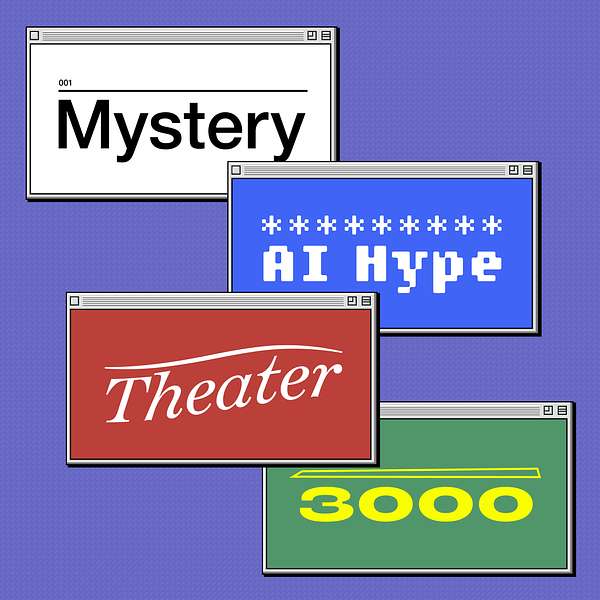
Mystery AI Hype Theater 3000
Mystery AI Hype Theater 3000
Episode 1: "Can Machines Learn To Behave?" Part 1, August 31, 2022
Technology researchers Emily M. Bender and Alex Hanna kick off the Mystery AI Hype Theater 3000 series by reading through, "Can machines learn how to behave?" by Blaise Aguera y Arcas, a Google VP who works on artificial intelligence.
This episode was recorded in August of 2022, and is the first of three about Aguera y Arcas' post.
Watch the video stream on PeerTube.
Check out future streams at on Twitch, Meanwhile, send us any AI Hell you see.
Our book, 'The AI Con,' comes out in May! Pre-order now.
Subscribe to our newsletter via Buttondown.
Follow us!
Emily
- Bluesky: emilymbender.bsky.social
- Mastodon: dair-community.social/@EmilyMBender
Alex
- Bluesky: alexhanna.bsky.social
- Mastodon: dair-community.social/@alex
- Twitter: @alexhanna
Music by Toby Menon.
Artwork by Naomi Pleasure-Park.
Production by Christie Taylor.
ALEX: Welcome everyone!...to Mystery AI Hype Theater 3000, where we seek catharsis in this age of AI hype! We find the worst of it and pop it with the sharpest needles we can find.
EMILY: Along the way, we learn to always read the footnotes. And each time we think we’ve reached peak AI hype -- the summit of bullshit mountain -- we discover there’s worse to come.
I’m Emily M. Bender, a professor of linguistics at the University of Washington.
ALEX: And I’m Alex Hanna, director of research for the Distributed AI Research Institute.
This is episode 1, which first recorded on August 31st of 2022. And it’s actually one of three looking at a long…long blog post from a Google vice president ostensibly about getting “AIs” to “behave”.
EMILY: We thought we’d get it all done in one hour. Then we did another hour (episode 2) and then we did a third (episode 3)...
And this being our first episode, we started off with some sound issues, so…it’s a bit awkward at first.
ALEX HANNA: Okay and we're back.
EMILY M. BENDER: Are we back?
ALEX HANNA: All right.
EMILY M. BENDER: Yes we're back.
ALEX HANNA: We're back. All right so sorry
EMILY M. BENDER: We're here. How's the sound folks?
ALEX HANNA: Yeah how's the sound? Let us let us know um if it's still trash, then maybe we'll reschedule this thing. But now I'm using OBS to stream.
EMILY M. BENDER: And it looks like it's working. Everyone's happy.
ALEX HANNA: Oh cool. All right why don't we get into the articles so while we have good luck?
EMILY M. BENDER: Okay, let's do the thing! So just to very briefly recap, we are concerned about AI hype. It seems worthwhile to dig into an AI hype artifact because there's not enough pushback in the world and this is a way to do pushback. The beautiful graphic that Alex created for this event suggests that we're actually talking about a New York Times article, but we are not.
We decided to keep the actual article secret until moments from now when I reveal it. um And the other important thing to know here is that I have read this blog post and Alex has not. And so the idea is something along the lines of reaction videos.
ALEX HANNA: It's like and yeah reaction, unboxing, things of that.
EMILY M. BENDER: But she does know who wrote it and what it is, right?
ALEX HANNA: I I did I I must confess I skimmed it last night and I was just like and then I was like you know and then I I think I was on the phone on a Zoom call with someone and I was like what the fresh hell is this? So let's.
EMILY M. BENDER: Let's do it.
ALEX HANNA: Let's do it into it.
EMILY M. BENDER: So here we are! Did my screen share work?
ALEX HANNA: Yeah I'm seeing it.
EMILY M. BENDER: Yeah.
ALEX HANNA: Oh perfect yeah.
EMILY M. BENDER: So this is a blog post written by a Google VP asking the question "Can machines learn how to behave?"
ALEX HANNA: Yes.
EMILY M. BENDER: Yeah.
ALEX HANNA: And I just and I just just want to say some background on this yes I was I was talking to Dylan last night that's who I was talking to. He's in the the chat. Hey Dylan what's up?
Dylan, our uh data engineer at DAIR.
And um so so Blaise is a VP at Google. He's been there for a while, has opined a lot on AI and large language technologies uh sort of um you know like known to be sort of this charming figure in a very uh uh uh I don't know I think fancies himself a little bit of a philosopher.
Uh I don't want to I don't want to go after him as an individual um because uh as a sociologist
I'm less interested in talking about individuals, more interested in thinking about what ideas
they're propagating and how that is sort of um kind of a discursive knot and sort of a set
of discourses and what that enables so yeah.
EMILY M. BENDER: Yeah um right and I should say I've never met Blaise. I've
interacted with him by reacting to some of his blog posts in the past with blog posts of my own
um and you know like Alex is saying I’m in it for the artifact and not for the person here. And this
artifact has a lot going on. So when I read it the first time it seemed like every paragraph or
so there was what felt like a howler and it felt like it'd be worthwhile to talk about it.
So it starts off saying "Beyond the current news cycle about whether or not they're sentient, there's this more practical and immediately consequential conversation about AI value alignment" and already that feels like we're down a strange path.
ALEX HANNA: Yeah yeah we're like we've we've kind of kicked this idea of value alignment and sort of this really weird terminology sort of evading kind of things about um about kind of AI and whether AI should exist or like or not. And rather than like yeah AI should exist but it should have our values. You know whatever that means because I don't really know what that means.
EMILY M. BENDER: And also that whole discussion is around, and this whole thing "can machines learn how to behave?" suggests autonomy and suggests that we don't have a choice
about whether or not we delegate authority and autonomy to machines.
And we absolutely do and this like idea of looking at it in terms of we have to get the AI aligned with our values; there's a whole problem whose values, right, but on top of that it's like well why? Right, why cede so much to automation? And it often feels like because if the values that the automation are aligning with are the values of people with power then we can sort of say oh well it's not us it's the machines. Yeah yeah so I'm super skeptical.
Um all right second paragraph "If as some researchers–” Hello! “--contend language models are mere babblers that randomly regurgitate their training data then real AI value alignment is at least for now out of reach." So as one of the people who contends that–
ALEX HANNA: Right.
EMILY M. BENDER: You know.
ALEX HANNA: This is like this is pointing at you directly Emily
EMILY M. BENDER: Yeah um in you know two peer-reviewed papers I should say not you know just Twitter and blog posts um it's not that I say therefore AI value alignment is out of reach. I have a totally different reaction as I was just saying right. It's just a category error to ask this question.
ALEX HANNA: That's right.
EMILY M. BENDER: So and then so, "There are some profound challenges here including governance, who gets to define what is toxic," um and so on. This is around the idea of trying to clean up data sets um and that always just feels like such a cop out. Like, well, who gets to say it's toxic?
ALEX HANNA: Yeah I mean well there's people who have been saying that it's toxic for some time. I mean people who are saying that are calling these out they're saying that these things are happening and I mean it is a dodge because one of the things I mean is that one you know like as you know one all these things are typically bound to only English language right so the quote unquote Bender Rule right?
That so people are but you know like we have this people toxicity that; I mean toxic is is such a bizarre term itself too because I mean when you talk about toxicity or hate speech or I mean toxicity is a term that sort of lives within computer science but when you're talking about things like discrimination or different kinds of frameworks you're not using the word toxic.
You're using you know language about rights or language about um disparaging or um dehumanization right? I mean those are different and more concrete kinds of things right? And so that kind of um and these things are happening all the time already you know.
EMILY M. BENDER: Yeah yeah exactly. And this question of who gets to decide sort of putting out there like that suggests that there's no power analysis to be done that everybody has um an equal ability to perceive what is disparaging and discriminatory. And you know what's really missing here is something like um you know understanding via the the matrix of domination.
And then you know there's there's a whole bunch of of really great work in Black feminist thought that sort of lays out systematic ways of thinking about this that are more sophisticated and more valuable than just like well we put everybody on evil playing field and then people. Equal playing field–evil playing field–and they have to just sort of fight it out as to who gets to decide, which is not the suggestion.
ALEX HANNA: Mm-hmm. Yeah um so labor uh I appreciate it naming that labor. So I mean is it humane to employ people to do toxic filtering? I mean I will give him credit there, sure I mean like that's something that like people a lot of people have written about. Sarah Roberts' work on on commercial content moderation.
And "Scale: can we realistically build larger language models under such constraints? This skeptical view also suggests a dubious payoff for the whole language model research program since the practical value of a mere babbler is unclear–” Agreed. “What meaningful task could a model do, a model of no understanding of concepts be entrusted to do? If the answer is none then why bother with them at all?"
Curious. I'm interested to see what the argument is Emily. Let's read the next paragraph.
EMILY M. BENDER: So I just you know I want to point out here that there's so many assumptions that of course we should be building large language models right? "How can we realistically build them under such constraints?" Well maybe the answer is don't build
them. Like that needs to be on the table um and yeah, "What meaningful tasks could a model with no understanding of concepts be entrusted to do?"
Um well, let's see, you can get pretty far with um you know speech recognition without understanding concepts and that's a meaningful and useful task. Not as good yet as you know the work of actual humans like the wonderful captioner that we were able to hire for today but um still beneficial. um but anything where um the outcome matters to people um you probably don't want to trust that model, especially if the people are looking at the language output and interpreting it. Because it's it's got no, there's no there there.
ALEX HANNA: Yeah yeah I'm curious what the premise here is on the turn here. "So on the other hand if as I'll argue here, large language models are able to understand concepts–" Okay you know I'm curious what he means by understanding a concept but let's continue.
EMILY M. BENDER: I think we'll get there.
ALEX HANNA: "--then they'll have far greater utility, though with this utility we must also consider wired landscape and potential harms and risks. Urgent social and policy questions arise too. When so many of us myself included make our living doing information work–"
Curious what that also means, information work, and what that who that encompasses.
"--what will it mean for the labor market, our economic model, and even our sense of purpose when so many of today's desk jobs can be automated?" Ooh that is quite a presumption.
EMILY M. BENDER: Yeah.
ALEX HANNA: Yeah.
EMILY M. BENDER: Oh man um so there's this are you following the sub stack by um uh Narayanan and Kapoor, if I'm getting their names right, um about AI snake oil? The first episode
of that just came out today.
ALEX HANNA: Oh yeah I I I yeah yeah I'm not familiar but yeah.
EMILY M. BENDER: They make wonderful points in there about how so much of this work in I mean they're going after the category of deep learning I think it's the same thing sort of looks at the artifacts that are output and says well, the work of producing those artifacts is the work and so if we can make that same form then we have automated the work and and that's where this like presumption of being able to um automate desk jobs. They say it really well in the substack. I recommend it.
ALEX HANNA: Yeah drop it in the in the in the chat or something and yeah let's go on. While you do that let's scroll down. I'm like I'm like okay we scheduled an hour. So: "This is no longer a remote hypothetical prospect but attention to it has waned as AI denialism–” Denialism, curious.
“--has regained has gained traction. Many AI ethicists have narrowed their focus to the subset of language model problems consistent with the assumption that they understand nothing.
Their failure to work for underrepresented–for digitally underrepresented populations, promulgation of bias, generation deepfakes, and outputs of words that might offend." That was what?
EMILY M. BENDER: AI denialism?
ALEX HANNA: Is it like is that like climate denialism, Emily?
EMILY M. BENDER: So I mean okay I'm always interested in looking at what's presupposed right, so the phrase like you say, ‘climate denialism.’ Someone utters that phrase, they're saying there's a important thing about the climate that I the speaker agree to be true, and if you by listening to me–if you don't challenge the presupposition you're in there with me.
ALEX HANNA: Yeah.
EMILY M. BENDER: And these other people are denial and denying it they are–they are denialists. And so he's saying the same thing. Like, “AI denialism has gained traction.”
Those those people out there who are putting out misinformation about how AI isn't what we claim, I guess.
ALEX HANNA: Right it's like it's like that it's what an interesting thing yeah.
EMILY M. BENDER: Yeah yeah um and then, "Many AI ethicists have narrowed their focus to the subset of language model problems consistent with the assumption that they understand nothing.”
ALEX HANNA: Their failure to work for–okay so this is sort of like it's sort of like the premise is that you have to say that they understand. There's no concept of under understanding. Okay but this is the argument. The argument is is his premise seems to be, we have to say that there's some notion of understanding that's happening under the hood and um there's sort of a coherent kind of thing that can be called sort of a concept or sort of a body of facts.
Because I'm even in this sort of loose kind of understanding of what is a concept is sort of like I'm sort of it evades me a bit. And there's some work that exists within machine learning on sort of like–so like TCAVs for instance, the um concept active you know concept activation vectors but I've always kind of wondered like what exists here?
What is a concept? What is that what is this, what is this ontologically? Like what does it actually mean? Like is race a concept uh you know it's like is gender a concept? Like and then you look at the papers and it's like well you know stripyness, this is a concept. Like stripe, like because they're like you know like how do you understand like the machine is understanding that this is like a zebra? It's like, well stripyness.
I'm like how's striping is like a concept that's like a visual. So it's kind of so I'm just like how is that different from from saying this is like an understanding of sort of um a pattern or as you've said Emily and like that these are pattern matchers you know?
EMILY M. BENDER: Right right yeah so the concepts–like a lot of the stuff in language is like well we've got we've got clusters of word vectors that relate. That are sort of they're clustered because they appear in similar contexts and so that is a concept. And it's like yeah
that might be the reflection of a concept in textual distribution, but that's not the same thing
as the concept itself and yeah.
But there's a I think there's a bigger problem. Well something that's bugging me about this paragraph is he says, “Many AI ethicists,” like who's, how much have you read, what's that qualifying over? But setting that aside, “have narrowed their focus to” these problems. As if we are um really not engaging with the important stuff which happens when the AI is actually understanding something and from my perspective is like no, actually the people who are looking at um propagation of bias, failure to work for digitally underrepresented populations.
That's probably a pointer to like Joy Buolamwini's work with Deb Raji and Timnit Gebru right um and um you know generation of deepfakes, output of words that might offend. All of these are what happens when the technology we have now is deployed and affects actual people living in the world now.
And I feel like the folks who are off, they’re thinking about, well let's make sure we get AI aligned with human values, they're the ones with the narrow focus because they're working in this fantasy world that's not connected to anything actual people's actual experience.
ALEX HANNA: Right, yeah yeah.
EMILY M. BENDER: So that's what's getting me worked up about this paragraph.
ALEX HANNA: Yeah.
EMILY M. BENDER: But the next paragraph, oh my god.
ALEX HANNA: The next paragraph is so this is like in the citation so yeah so. "There's serious issues however–These are serious issues. However AI models are becoming far more capable than this narrow focus implies.” Okay. “AI can engineer drugs or poisons, design proteins, write code, solve puzzles, model people's states of mind–" Okay I just want to stop at that because what the hell? "--control robots in human environments and plan strategies.” These things–
EMILY M. BENDER: Yeah.
ALEX HANNA: These are a lot and like I'd love to like pop and then you've got the second tab open because we like, we gotta go over to these citations. So like okay.
EMILY M. BENDER: I hit that one and I was like what does he mean, “model people's states of mind”? Because states of–modeling someone's states of mind, that's what allows you to say things like, oh I can tell that um Kim over there is looking for their keys right and that's because I am watching Kim's behavior and thinking about what would make me do those things, what can Kim see, what does Kim like? And and like that's modeling Kim's state of mind.
It's also really important for um communication and language right? We model each other’s states of mind. And I'm thinking what research would be showing that? Right today's AI today's AI models–that's large language models right, we're talking GPT-3, we're talking LaMDA, we're talking right? Really? What research is showing that?
ALEX HANNA: Right.
EMILY M. BENDER: So first of all this is on arXiv right?
ALEX HANNA: Well all of these are on arXiv. Like the last three, I mean. I think the only one was the protein um one the one and the one that was the poison one, um “competition level code gen–” like I'm not sure what competition-level code is. That's what's kind of curious given that most code is not competition level code um but they're all yeah these are all um yeah these are all on arXiv, not peer reviewed.
EMILY M. BENDER: And then is this there we go um so I went and looked. This is kind of small. Hopefully people can see it. What did they do? Well they took a collection of text–in in Korean by the way, exciting to see something that wasn't English here–and they had people annotate sentences of diaries for whether the writer was mentioning the presence of others without inferring their mental state.
So the example is, "I saw a man walking down the street" fails to take the
perspective of others. "I don't understand why they refuse to wear masks" successfully takes
perspective others. "It must have been hard for them to continue working." So some existing texts written by people annotated by other people for these three categories and then they trained a BERT type model to redo that classification right? That is not the same thing as the BERT model actually modeling anybody else's state of mind.
ALEX HANNA: Right.
EMILY M. BENDER: Right, it's BERT reproducing those classes and
it doesn't actually do that well.
ALEX HANNA: Yeah.
EMILY M. BENDER: When you click through the paper it's like yeah like you know better than chance kind of thing, right.
ALEX HANNA: Right.
EMILY M. BENDER: So this is quite a yeah I mean–it's quite a claim and I mean that's yeah I'm I I mean this is like I have a hard time sort of parsing this, because it's sort of thinking about this in kind of a communication you know like communication. lt's very curious how this is and I mean I haven't really come across this kind of idea of ‘theory of mind’ or or kind of a matter of empathy uh uh uh as a matter, but it's it's sort of like you're you're saying that you're inferring some kind of internal state, which is already like all my alarm bells are ringing on what this is you know?
I mean I think this is something that people do on a regular basis and it's embedded in our language. And I noticed in the chat that people are reminding us that this is also frequently weaponized against autistic people and that's a big problem um so thank you for pointing that out in the chat.
When I hear "theory of mind," I'm thinking of things along the lines of it's what allows us to say and understand sentences like you know, "Alex believes sociology is interesting." Where that sentence is talking about your what I think your beliefs are, and in order for a computer to do that the computer would have to like literally have representation of the person that it's talking to and the ability to reason about it and all the things that language model doesn't have.
ALEX HANNA: Yeah, yeah, it's not entering any internal states.
EMILY M. BENDER: Yeah but I think Blaise wants it to and so he makes this statement here right uh model people's states of mind and then you know cites that thing off arXiv which doesn't even support that.
ALEX HANNA: Yeah uh okay let's let's get into this. "These things are hard to dismiss as mere babble.” Okay. “They'll increasingly involve sensitive interactions with people in the outcomes in the world, whether for good or for ill. If AIs are highly capable but malicious or just clueless about right and wrong, then some of the dangerous outcomes could even resemble those popularized by the different community of philosophers and researchers who have written more both more sensationally and less groundedly about existential risk AI–existential risk."
Okay there's a lot.
The “AI existential risk people,” like I kind of like if we go off on them I kind of don't think we'll finish this, but anyway we could have a whole thing about the AI existential risk people.We could invite Timnit to talk about longtermism and effective altruism and like we could go off. We we could do a reading group and like a hate read of that but like I'm willing to bracket that for the sake of going reading the rest of this.
EMILY M. BENDER: All right let's keep going.
ALEX HANNA: Okay let's see um yeah–streaming series. This is definitely going to be a series uh it's– "It's becoming increasingly clear that these disconnected camps in the AI ethics debate are only seeing one part of the picture. Those who are deeply skeptical about what AI can do haven't acknowledged either the risk or the potential of the emerging generation of a general purpose AI.” Woof. “On the other hand–" I want to finish this the sentence and then like and then get into it.
Yeah yeah and Ben's in the chat saying "AGI makes an appearance. Drink!" Yeah. "On the other
hand while those in the existential risk camp have been expansive in their articulation of potential harms and benefits, they consider AGI to be so distant, mysterious, and inscrutable that it'll emerge spontaneously in an intelligence explosion decades from now."
Here's what the citation is there: "AGI might then proceed perhaps due to some Douglas Adams-ish programming oversight, to turn the whole universe into paperclips, or worse." What's the citation on this intelligence explosion? Oh, Future of Life okay. Like all right oh so we're
getting into the cites of “Superintelligence” and Nick Bostrom so this is definitely um stuff to stuff to be annoyed about.
EMILY M. BENDER: Yes.
ALEX HANNA: I'm like willing to bracket this because I'm just like yeah yeah but so okay, "Such doomsday scenarios may have seemed credible in 2014 but they're far less so now that we're starting to understand the landscape better. Language modeling has proven to be the key to making the leap from specialized machine learning applications of the 2010s to the general purpose purpose AI technology of the 2020s–"
EMILY M. BENDER: It's not general purpose AI technology.
ALEX HANNA: No it's not. We wrote a paper on this! On benchmarks. Yeah they're they're language models or they're visual models and kind of anything this kind of language of general purpose AI sort of says, you know you take a large language model and then you bolt on some kind of um specialized tuning to it. And this makes it sort of a general sort of model.
I'm really curious on the sort of like bolting on a sort of like a move, both technically and sort of as a uh you know it's because there's a certain kind of this idea of general purpose AI is something that is a slightly different argument than an AGI, because I feel like it is we're going to have sort of one computer and one thing that does stuff with language or we're going to do something that is stuff with multimodal data inputs.
There's been so much hype specifically on that that is you don't have to believe in AGI to sort of like go to general purpose AI–you know like you know I'm saying?
EMILY M. BENDER: Yeah yeah. And the problem I mean now I'm just repeating some of the stuff we said in that paper but, and I put the link to the paper in the chat, this general purpose thing is just a fallacy that we're all falling into because you put enough training data into a large language model, it can spit out text on any topic.
And so you can easily fool yourself into thinking that it can do something useful on those topics. But as soon as the outcome matters, as soon as like the sort of truthfulness and groundedness of that text matters to anybody, it's clear that it can't. And so no we don't have general purpose AI technology and I'm not sure we want general purpose AI technology. Like that's another–you know all this stuff about existential risk, it's like so don't build it?
ALEX HANNA: Right. Yeah I'm, yeah we're going to have to do like a like a hate read of “Superintelligence” or something because I just–
EMILY M. BENDER: If we could stomach it.
ALEX HANNA: If we could stomach it you know I feel like any but let's um yeah let's let's let's do it.
EMILY M. BENDER: Yeah well it looks like there's some enthusiasm for this to become a series.
ALEX HANNA: Yeah.
EMILY M. BENDER: We'll be faster and better with the setup next time right?
ALEX HANNA: Yeah. "Although anthropomorphism does pose its own risk, this familiarity is good news in that it may make human value alignment far more straightforward than–than the existential risk community has imagined." Woof, that was a sentence I didn't want to read.
"This is because, although our biology endows us with certain pre-linguistic moral sentiments such as care for offspring and in-group altruism, both of which we share with many other species, language generalizes these sentiments into ethical values, whether widely held or aspirational. Hence oral and written language models have mediated the field of ethics, moral philosophy, law, and religion for thousands of years." You're the linguist Emily, what?!
EMILY M. BENDER: Yeah so I mean okay I'm a linguist I'm not into like you know the sort of deep anthropological history and these questions about like how does language relate to our development as a species and whatnot. But I mean does language generalize sentiments into ethical values?
Language allows us to talk about these things with each other yeah and to um produce uh you know morality plays and other things where we pass them on to you know within a society to further generations.
"Oral and written languages have mediated the field of ethics–" Like well yes it's true that um any academic field takes place in language. Laws are famously written in language, religions famously have religious texts which is language. Yeah um but again it's I think it's important that these are texts that are produced and interpreted by people who like actually have full linguistic competence and–
ALEX HANNA: It's kind of a yeah it's kind of an interesting claim to sort of that these are language, “generalize these sentiments into ethical values” as if there's a way to sort of say that there's sort of ethical values that I like it's it's I yeah I'm I don't know how to evaluate this claim other than it's kind of bizarre.
EMILY M. BENDER: Right and as someone was pointing out in the chat, not a whole lot of citations in this part.
ALEX HANNA: No.
EMILY M. BENDER: Okay. "For an AI model to behave according to a given set of ethical values it has to be able to understand what those values are, just as we would.” Um no false. Right? I mean all designed artifacts have values designed into them.
ALEX HANNA: Right, yeah.
EMILY M. BENDER: If we're going to have a machine that's behaving according to a set of ethical values that could be because we have designed it well for its environment and the values that we want to represent, without it having any understanding of what they are. "By sharing language with AIs we can share norms and values with them too."
ALEX HANNA: What? So it's sort of so I think there's a claim here that because ethics are mediated by language, and these models–we're building these AI models and they have to understand our values, then we share our norms with them too.
And so that's sort of like that's either one claim, one of the more reductive claims which is sort of
these things have values embedded with them which is true, sure. Artifacts have values. Artifacts have politics. But then the other kind of I think the stronger claim which I think he's making here is that AI has some kind of internal representation of values, and that we're sharing language to them and they're sharing those norms as the sort of agentic agent and that is a bizarre claim.
EMILY M. BENDER: Right. And then that those norms are guiding their behavior.
ALEX HANNA: Yeah.
EMILY M. BENDER: No, these are machines.
ALEX HANNA: Yeah, yeah. Oh okay that that was a that was a knee slapper. There should like come up with like a thing for the series when something's a real zinger we like ring a bell or–
EMILY M. BENDER: Have some kind of have a little animation go across the screen.
ALEX HANNA: Yeah. If you people like know if there's some Twitchy like norm on that,
please share the chat.
EMILY M. BENDER: Well someone someone said Wittgenstein is rolling in his grave. We
could have a little animated Wittgenstein rolling in his grave.
ALEX HANNA: I know oh yeah well that's Wells. Wells, make an animated Wittgenstein and we can like–
EMILY M. BENDER: Oh man. All right, keep going through. "In itself, the ability to endow an AI" and can we please stop it with the you know an AI or the AIs as if they are like individuals in the world. Okay: "--the ability to endow (one of these things) with values isn't a panacea. It doesn't guarantee perfect judgment, an unrealistic goal for either human or machine. Nor does it address governance questions who gets to define an AI's values and how much scope will these have for personal or cultural variation?"
So now we're back to the just like, oh yeah well you know we're all different so how do we decide who decides?
ALEX HANNA: Right.
EMILY M. BENDER: You know, yeah.
ALEX HANNA: "Are some values better than others?” Oh dear. “How should AI–" How should these machines, let's say.
EMILY M. BENDER: Salami.
ALEX HANNA: Like let's say um let's replace like AIs or any kind of that with like um how does how does mathy–how does a mathy model.
"--their creators and their users be held morally accountable–” Sure. “--neither does it tackle the economic problem articulated by Keynes–” First shout out to Keynes! “--in 1930 how to ethically distribute the collective gains of increasing automation, soon to include much of intellectual labor." Oh, okay.
EMILY M. BENDER: Asserted like that.
ALEX HANNA: Yeah this is such a claim. I want to go to the next paragraph and see–we got like 10 minutes left and like but I'd love to get to the end of the first section at least. Maybe we can take out the rest of that in a following chat.
EMILY M. BENDER: This one blog post is gonna become a series.
ALEX HANNA: I know. What it does so like let's see–let's let's get to the end of this and discuss. So: "What it does offer is a clear route to imbuing [mathy um mathy math] with values that are transparent, legible and controllable by ordinary people.” Okay I'm not sure how that shakes out. “It also suggests mechanisms for addressing the narrow issue–narrower issues of bias and underrepresentation with the same frame–same framework."
EMILY M. BENDER: Because remember those issues are the narrow ones.
ALEX HANNA: Those are the narrow ones the–you know and I in some ways I agree that bias is a very narrow framing.
EMILY M. BENDER: Yes.
ALEX HANNA: An underrepresented framing, but the way that he is saying the more important
issue is quote unquote ‘value alignment,’ which is a I would say sort of a narrow also narrow
and fits very squarely into considering bias.
"My view is that AI values need to be and shouldn't be dictated by engineers, ethicists, lawyers or any narrow–other narrow constituency.” Yeah, I mean okay. “Neither should they remain bulleted lists of desiderata posted on the web pages of standards bodies, governments, or corporations, with no direct connection to running code. They should instead become the legible and auditable operating handbooks of tomorrow's [mathy maths]."
That's a lot there like what's yeah what is being what's being said here? Because I'm sort of like reading this and it's sort of saying that the way I'm reading it is saying we shouldn't have sort of first off you have to accept that value alignment is sort of a real thing. Which I'm already like hmm okay. I do think governance of AI and data should be more collectively gained, but value alignment is not where that governance comes from.
Value alignment already gives away the game. It says that AIs or mathy maths are these things that need to be need to be created. They are going to be publicly serving some kind of need and because they're going to be, we need to have some kind of um auditable something of this. And that has some connection to do with these internal states by these models, rather than like-- Which is a really weird it's a it's a bizarre claim because it's sort of saying like you're already giving some some inevitability to the modeling.
You're also sort of discarding standards bodies, governments, people that are ostensibly you
know part of existing governance structures.
So it's sort of like it's a real it's a real dodge here and I'm and I'm and I and I'm just having such a struggle even taking the premise of this you know very seriously.
EMILY M. BENDER: I want to take us back up to the top on top of what's on the screen
"How should AIs, their creators, and their users be held morally accountable?" So first I want to say there's a category error here. I'm talking about AIs being-- sorry mathy maths being held
morally accountable right? The moral accountability is something that properly sits with people.
ALEX HANNA: Yeah.
EMILY M. BENDER: And and it properly sits with people who have agency. So creators, yes, have agency here. Users will, depending–right? You know if if you're the person who has to deal with some voice recognition system to get to your bank account, you don't have a whole lot of agency right right. But if you're the person who decided to deploy that for the bank you're the person who created it, then there's an agency there.
And I think we already have things you know imperfect and still needs work for holding people accountable for their actions and the things they make and put in the world. And it's not like–
It's a dodge as you say, to say just because we now have mathy maths it's a whole new problem. And you know, oh dear what are we going to do, how do we hold these new mathy maths accountable? It's like well, you don't, because that's a category error.
There's people there who are responsible. People make the decisions to create them and to put them out in the world and have the power to turn 'em off.
ALEX HANNA: Exactly, Yeah. I mean you're like already kind of thinking about this I mean and we know I mean we already know who takes the fall for this stuff, right? I mean when AI you know when AI goes wrong or there's some kind of failure, failure in sort of a data collection process or some kind of a decision you know the people–corporations are not taking these falls. AI, if you can start to say a model is taking the fall right um when this happens you know the people who take this fall are data annotators, you know, people who are creating the data and you know.
Mila Miceli for instance is a DAIR fellow, just defended her PhD yesterday–awesome!
EMILY M. BENDER: Yay!
ALEX HANNA: –and she has documented that. Like the way that a lot of this in the AI field talks about data laborers is that they are um you know there are problems to solve. There's like bias and that's a problem you know. There's like when we need to sort of erase the subjectivity of these people. And you know who really whose subjectivity we really ought to prioritize is like these people mostly in the West who you know really believe in these kind of articulations of liberty and freedom and all these kinds of uh things typically based on kind of a U.S. constitutional right or sort of kind of the the the EU rights. And kind of like the EU human rights code.
And so it's you're already sort of saying like well we already say like you already these things are already done in practice right and I-- Blaise sounds like he's like saying well no no we're gonna unsettle this but in a way that is like you know is already assuming a lot, right?
EMILY M. BENDER: Yeah.
ALEX HANNA: And it's already assuming that these these sort of things have kind of internal
states.
EMILY M. BENDER: And concepts!
ALEX HANNA: And concepts right concepts. And this is kind of like fascinating. This idea of
like a concept that this kind of machine has this kind of internal state that has a a representation
of a concept. As if that was not overlaid with clear boundaries by an annotator but not
annotators but you know data set creators or requesters and people who are um asking you know thousands of people to you know annotate this according to very strict guidelines
or you know people at companies.
EMILY M. BENDER: Yeah.
ALEX HANNA: Yeah. So we're so we're like at the first how much left is this on this. Maybe we need to do this again like.
EMILY M. BENDER: Yeah, so I think we like look for–my my scroll bar is here.
ALEX HANNA: I know. Oh yeah gosh there's like maybe another you know like 500–there's another like ten thousand, twenty thousand words on this. We didn't even get to ENIAC which I would love to you know like and we didn't even get to where he calls out you know uh a parrot you know Stochastic Parrots so like–
EMILY M. BENDER: Yeah no. Okay this is yes so the scroll bar ends up down here.
ALEX HANNA: Yeah.
EMILY M. BENDER: And we were like up here.
ALEX HANNA: Yeah, yeah.
EMILY M. BENDER: Yeah.
ALEX HANNA: So like maybe okay so maybe yeah. Wells is asking, how did he have the time to
write this. Like I'm really curious how someone when–when we were at Google we spent a lot of time just putting out fires. I'm really curious how like a VP has you know has enough time to do things like and um it's it's what's like maybe maybe he is one of Andrew Ng's like famous, ‘I work 18 hours a day’ you know like.
And if he is like you know some–some people relax in different ways um and some people you know like some people love to write long Medium posts and you know like you know go for it. But you know I won't you know there's I don't want to you know criticize Blaise's time management techniques and I'd love to get into the rest of this. So maybe one thing we can do is like you know make this into a series we can sort of you know do you know do two or three I don't know however long it takes you know like–
EMILY M. BENDER: We might figure out how to be a little bit more efficient but yeah just we could turn a series on just this one post.
ALEX HANNA: Yeah we could we could do that and then I think maybe we do a group read of
of something by Nick Bostrom.
EMILY M. BENDER: Oh man.
ALEX HANNA: Because I do think I mean I might have to have my like bottle of Pepto Bismol right by me but–
EMILY M. BENDER: Nice and pink, here we go.
ALEX HANNA: Yeah well uh yeah and some people are in and they're saying they're like you know asking if this is a Google blog or personal one. But it is it is–it is a personal one but it does have to get approved by Google. So you know if you want to put anything on Medium you know and he has 4,000 followers then he gets shared, so you know there's there is a reach and a bit of a cult of personality involved here. So next time bring your bingo card. Yes Ben, bring your drink.
Uh you know it's we're doing this in the middle of the day and it's a Wednesday I mean.
And let me do it on a Friday and then we can just, you know you can have your drink next to you um of your choice. I'm gonna have my my tea.
And then you know maybe we can and–I'm glad we got the sound fixed so we'll actually do this so yeah. We'll also have um you know the recording we're gonna put I'm gonna post this on YouTube um for folks that missed it and yeah. But hey Emily this is a pleasure. I think I think the people say this this should be a series.
EMILY M. BENDER: Yes. I love the last comment here this was agony. We have to do it again!
ALEX HANNA: Thank you. This is great.
EMILY M. BENDER: Misery loves company. Thank you all so much for joining us.
ALEX HANNA: Yeah thank you for joining it was a pleasure and thanks for chatting
in the chat you know. Y'all are y'all are great and you know we hope you'll join us next
time for Mystery AI Hype Theater 3000.
EMILY M. BENDER: Thank you!
ALEX HANNA: All right thanks Emily! Bye all!
ALEX: That’s it for this week!
Our theme song is by Toby Menon. Production by Christie Taylor. And thanks, as always, to the Distributed AI Research Institute. If you like this show, you can support us by donating to DAIR at dair-institute.org. That’s D-A-I-R, hyphen, institute dot org.
EMILY: Find us and all our past episodes on PeerTube, and wherever you get your podcasts! You can watch and comment on the show while it’s happening LIVE on our Twitch stream: that’s Twitch dot TV slash DAIR underscore Institute…again that’s D-A-I-R underscore Institute.
I’m Emily M. Bender.
ALEX: And I’m Alex Hanna. Stay out of AI hell, y’all.