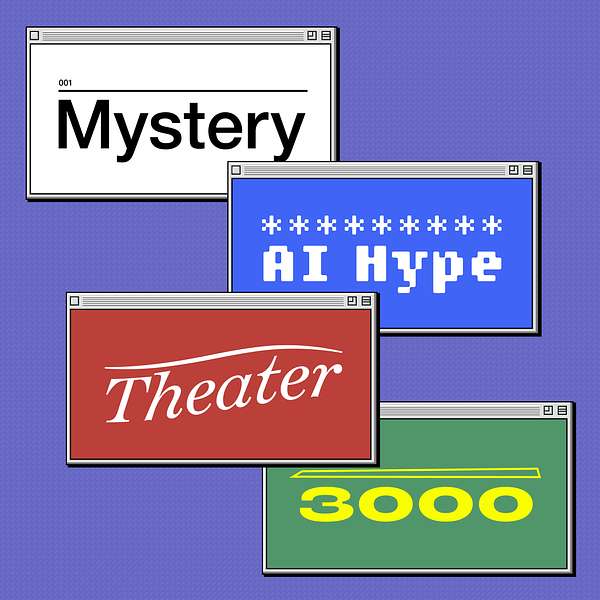
Mystery AI Hype Theater 3000
Mystery AI Hype Theater 3000
Episode 3: "Can Machines Learn To Behave?" Part 3, September 23, 2022
Technology researchers Emily M. Bender and Alex Hanna kick off the Mystery AI Hype Theater 3000 series by reading through, "Can machines learn how to behave?" by Blaise Aguera y Arcas, a Google VP who works on artificial intelligence.
This episode was recorded in September of 2022, and is the last of three about Aguera y Arcas' post.
You can watch the video of this episode on PeerTube.
Check out future streams at on Twitch, Meanwhile, send us any AI Hell you see.
Our book, 'The AI Con,' comes out in May! Pre-order now.
Subscribe to our newsletter via Buttondown.
Follow us!
Emily
- Bluesky: emilymbender.bsky.social
- Mastodon: dair-community.social/@EmilyMBender
Alex
- Bluesky: alexhanna.bsky.social
- Mastodon: dair-community.social/@alex
- Twitter: @alexhanna
Music by Toby Menon.
Artwork by Naomi Pleasure-Park.
Production by Christie Taylor.
ALEX: Welcome everyone!...to Mystery AI Hype Theater 3000, where we seek catharsis in this age of AI hype! We find the worst of it and pop it with the sharpest needles we can find.
EMILY: Along the way, we learn to always read the footnotes. And each time we think we’ve reached peak AI hype -- the summit of bullshit mountain -- we discover there’s worse to come.
I’m Emily M. Bender, a professor of linguistics at the University of Washington.
ALEX: And I’m Alex Hanna, director of research for the Distributed AI Research Institute.
This is episode 3, which first recorded on September 23rd of 2022. And it’s actually one of three looking at a long…long blog post from a Google vice president ostensibly about getting “AIs” to “behave”.
EMILY: We thought we’d get it all done in one hour. Then we did another hour (episode 2) and then we did a third (episode 3, that's this episode)...If you haven't listened to the first two episodes yet, we recommend you go back and do that first.
EMILY M. BENDER: Now we're live. Hi Alex!
ALEX HANNA: Hey Emily how you doing?
EMILY M. BENDER: I'm doing pretty good. I am looking forward to being done with this terrible blog post. That is a goal for today.
ALEX HANNA: I'm also looking-- Hold on so that now there's an echo. Oh I see why there's an echo because I haven't turned my sound off in in Twitch. Okay great.
That that helps a little bit. I'm also going to turn this down a little bit because there's a little bit of noise yeah.
EMILY M. BENDER: Should I be concerned that we seem to have zero people in the Twitch stream? No seven. Okay that's better.
ALEX HANNA: Yeah people are people are here.
EMILY M. BENDER: Okay good great.
ALEX HANNA: Give it a second to file in.
EMILY M. BENDER: Okay.
ALEX HANNA: All right.
EMILY M. BENDER: I think we should tell the people about these silly hats that we're wearing.
ALEX HANNA: Yeah. These hats are great, yeah tell the story.
EMILY M. BENDER: So you know I I want you to help me. We were watching something and like back channeling in our um Twitter group DM thing. I think it was maybe even during the NeurIPS conference where we were presenting this paper?
ALEX HANNA: Yeah.
EMILY M. BENDER: Have I got that right?
ALEX HANNA: I don't know when it was but it was some time.
EMILY M. BENDER: Yeah and we were somehow I mean Deb had made those fabulous slides where she had taken images from the book. So this is "AI and Everything in the Whole Wide World Benchmark" paper, memorialized as these trucker hats. And the little graphic that you probably can't see is from the "Grover and the Everything in the Whole Wide World Museum," a wonderful book from 1973. That was sort of our motivating metaphor in that paper and we were just having fun back and forth in that chat saying you know wouldn't it be cool to um you know like what's the what do these things say?
So the it says everything else. So this is this is literally just the picture from the book and then we added this "everything else" font and I'm sure this is your graphic design Alex and not mine because you're better at that.
ALEX HANNA: No I I edited this a little bit to make the things bigger but it's like where he says "Look at this! Buildings! Bushes! Windows! And mountains!"
And I mean the story of the book is that he's looking at this you know this kind of taxonomy that's within the museum and then you know there's like a room of very tall things like a room of very plushy things and then he's like, "But where is everything else?" So then he goes outside and he's like–
EMILY M. BENDER: There's a door that says "Everything Else."
ALEX HANNA: Everything, yeah.
EMILY M. BENDER: Here it is!
ALEX HANNA: Yeah, yeah.
EMILY M. BENDER: And then everything else is the actual world.
ALEX HANNA: That's right yeah.
EMILY M. BENDER: So anyway it was just fun back and forth somewhere someone said "We should have hats!" and I'm like I can make that happen.
ALEX HANNA: Totally hats hats are how you know it's official.
EMILY M. BENDER: Yeah, we have we have paper swag.
ALEX HANNA: Yeah.
EMILY M. BENDER: All right but we only have an hour to get this done.
ALEX HANNA: Yeah.
EMILY M. BENDER: And we are we're gonna be done with it.
EMILY M. BENDER: So um for folks who are joining us for the first time, this is our third episode um of Mystery AI Hype Theater 3000 in general and also the third one focusing on this blog post that maybe I should share now so you can all see it.
Um here it is um and machines... Did it work? Can you see?
ALEX HANNA: Yeah yeah it is working.
EMILY M. BENDER: All right I'm gonna go up to the top though. Um so this is a blog post by Blaise Aguera y Arcas entitled, "Can machines learn how to behave?" And we were going like paragraph by paragraph in episodes one and two and it was just too slow. So we've now taken on the homework of each reading the rest of it, um re-reading in my case.
ALEX HANNA: Which was very hard actually. It was a slog you know?
EMILY M. BENDER: Wasn't it? yes.
ALEX HANNA: But we're doing it now.
EMILY M. BENDER: Yes so we've both like picked out things that we particularly want to share our reactions to and we're just gonna take turns. We're going to be hopping around in the text a
bit, we're not being thorough about it anymore um because it's time to be done with this.
ALEX HANNA: Totally.
EMILY M. BENDER: So, Alex, what's the first thing that you want me to um control F to in this document?
ALEX HANNA: Oh my gosh okay. Why well why don't you go first? Because I think we stopped at the last thing and we had just finished talking about um Asimov's laws and we're going to this AI for human interaction um and so you know I think the last thing we were talking about was the sentence about "thoughtful human judges" um and we and if you're interested in what we were saying about that you can go to uh our last video on YouTube and um but then we were started talking about um those kinds of ideas of self-driving cars and using language to program things and translation...
So I I'd actually love to hear your thoughts on this Emily as a linguist. I have some thoughts uh but I I so I'm going to riff off what you say so you go first.
EMILY M. BENDER: Yeah well so I think this picks up on that point, though it's a little bit further down, where he says "As a corollary, self-driving cars, unlike industrial robots also need ethics."
Again I'm not convinced. Like self-driving cars are still tools they need to be designed to be safe, they need to be designed um to interact well with the environment and the people in the environment. I don't think that's the same thing as them having ethics. So we have a you know another instance of that category error there.
Um and uh so "not the trolley problems–" Can we just stop talking about the trolley problems? They are they're not relevant. Um so thankfully he's sort of dismissing them. But then he says "So as odd as it may seem, fully autonomous driving may require general language capable AI---not just so the passenger can tell it where to go but for the AI to be instructed how to behave. That is, not just what to do but what not to do."
And this seems to be this idea that it would be somehow more efficient to just speak to the car and tell it what to do and somehow the car would be you know sufficiently um developed mathy math that it would understand and be able to generalize and like I don't see why that is any more efficient than um coming up with computer code to tell the car what to do right? When when we train human drivers it's not like what they learn in driver's ed is the only information they're going to use to become good drivers right?
This whole thing about wanting to be safe in themselves and wanting to not cause harm to the people in the car with them, the other people that they're in their environment like all of that is outside of this. Like we're teaching people how to drive thing and this just yeah so. Anyway you had thoughts too though.
ALEX HANNA: Well it was interesting because it was sort of you know I think and I think this gets a little bit about um in thinking about this it is getting a little bit to this kind of idea or this sort of thought process and a lot of this kind of line of thinking which I think is very much in the kind of Marvin Minsky, Seymour Papert kind of idea of like everybody could be a programmer and let's we're going to use language and because we have a more robust way of using like language with ethics I think he thinks excuse me he thinks that's a good idea.
Um there's a great book by uh Morgan Ames who's at Cal and it's it's and it's about this idea of thinking that and it's about this one laptop per child program that is this very neo-colonial idea of if you ship laptops to people over here they're gonna you know like in Uruguay or Peru they're going to just automatically program.
But it ignores the huge infrastructure problems that are associated with that. And but she goes into this sort of intellectual history of Papert and Minsky and the kind of MIT Media Lab. Negroponte is another one. And um and kind of like how there's some thought of like making these things universally programmable without really understanding you know what's embedded in that. And I mean this sort of gets into a little bit of the discourses on democratizing AI, "I'm
bringing it to the people" when you know that's not you're not actually democratizing you're basically uh you know conglomerating power in certain companies through their models and then you know people have that have mass infrastructure or finances to do that anyways.
EMILY M. BENDER: No democracy means shared governance, not shared access, and yeah. But I think we could go on for a whole hour about democratizing AI but I think we should move to the next bit of this blog post. Um so now it's your turn. Tell me to control F to something and I will--or start reading the bit you want to react to and I will get to it.
ALEX HANNA: Okay so I think the next part-- Well now Anna is sitting on my notes so hi Anna I need you to go off my notes ah and not get caught off my cords. Oh no we have a cat error.
[laughs] The next thing I want to dig into that I think it's the next paragraph or the next section which is um which is about gender bias. So it's this section: "In 2018 the Google Translate team–" It starts out with that.
And so in this section um what he's talking about is this problem this problem and I'm seeing some comments now as I'm reading. Andrew says as a roboticist who works with industrial robots I can attest the premise that industrial robots don't need ethics. Yeah, 100 percent.
Yuthcara says airplanes don't need ethics but the software designers that insert process to do. Are robots different? And I kind of want to get to that but I'm going to bracket that in a second.
And then um Shamik Bose says something to break it down a little bit and um we'll get into that a little bit later because I kind of want to get into it it's been a running theme for this piece.
EMILY M. BENDER: Yeah.
ALEX HANNA: But I think there's a way we can concretize that in the end. So this part here where he's talking about "she's a doctor.” This is a thing where basically Google was allowing these gendered translations from Turkish to English and provided two kinds of mitigations but also "worried about the engineering effort involved" which is kind of weird.
I mostly want to take issue with this next thing he says: "Gendered assumptions in language are such a small corner of the larger ethical landscape." And that is a really um of course that's a very uh patriarchal view of kind of thinking about language itself, um thinking about the idea of gender bias as being this sort of corner of ethics, as if ethics has to do with these kind of things of uh you know large-scale harm or I don't know some kind of an ideal sort of thing that exists in the minds of something you know if you are a true Asimovian or something.
But that's been really unpacked by feminist technoscience scholars for a long time, to center gender as a central analytic in in ethics itself um I'm thinking about Nancy Fraser for instance and her critique of the Habermasian public sphere and Nancy Fraser says okay you know this kind of critique uh you know in of you know anybody can come to the public sphere.
You know that's that's not how this works. There's not this kind of uh neat division between the public and the private. Actually the private is a place of immense harm and immense policing
um and so the idea of gender and language as a small part of an ethical landscape is really ignoring that work by feminist ethicists and feminist technoscientists who use it as a central
analytic. So I just wanted to dog on that and just yeah go ahead.
EMILY M. BENDER: I really appreciate that because that is that is a sort of a different take and I think a really valuable one on what set me off in this paragraph. Um so yeah so let's not minimize gender like that's an incredibly important lens to look at things through and but at the same time even if it weren't even if it were small his argument doesn't make sense, right? He's basically saying oh no these you know, there's going to be all these separate little issues. What about this going to be too much engineering effort to go address them one by one.
And it's like well okay first of all you could decide not to try to build the general purpose thing that has all these problems. Like no is a possible answer. If it's got too many problems and you don't have the time to fix them, then don't build it, right. That's one way to go. And another way to go is you know you were saying this thing about like you're a perfect Asimovian, as if there is one sort of underlying thing you could do that would make everything ethical, um rather than going bit by bit.
And it's like I don't think there is. I think you really have to engage all of the aspects of the use cases you're putting this thing into and those are going to be situated. They're going to be idiosyncratic. It's going to require talking to lots of different people. And that doesn't get any easier if you are programming your computer through natural language, as opposed to some other way. Like the the work of talking to the people and understanding the problems is the same.
ALEX HANNA: Yeah absolutely yeah I mean I think this is sort of thinking about this net thinking about this I mean. Right you're going to if this is a thing that I actually I'm trying to think about-- I
think you said it perfectly I don't need to add more.
EMILY M. BENDER: I want to kind of–
ALEX HANNA: Yeah go ahead go!
EMILY M. BENDER: I'm gonna go to the next one.
ALEX HANNA: Yeah I was I was going to too and it was sort of related but I'll let you go into it.
EMILY M. BENDER: Okay. I'm going to um which is uh there's a couple things I want to hit really fast up here. Um so uh describing the foundation models and that term right?
ALEX HANNA: What a term.
EMILY M. BENDER: Um yeah we've got the foundation model and then "LaMDA is fine-tuned to be sensible, specific, inoffensive, and internally consistent–" I'm sorry all of those as a dialogue partner.
Um and this is you know these are actually technical terms, right. They have created some training data that is designed to measure sensibility, specificity, inoffensiveness and internal consistency with you know humans doing annotation tasks.
Um and the result of all this is um the if this establishes something like a quote mindset and I like that is one of the wishful mnemonics. Um so Melanie Mitchell points to this term but it comes from Drew McDermott um where when we describe computers as having things like a "mindset" or an "opinion" or "knowledge," we are pulling in all these ideas of what we would like it to be–well "we" I don't actually, I'm not interested in building AI–but people building it I would like this to have a mindset but it doesn't, right.
Um and sort of in the same area it's talking about um this pre-training task where um the pre-training task is is just language modeling, right. So we're going to drop out certain words and the system has to guess what word was there. But he tries to pretend this is lots of different things.
So: "If a word like "volcano" were blanked out, this would be a test of reading comprehension (What are we talking about? a kind of volcano). If "cone" were blanked out, it would be a test of general knowledge (Are volcanos shaped like cubes, spheres, cones, something else?). If "Mount Melbourne" were blacked out it would be a test of specialized knowledge (in this case of esoteric geography)."
And so on. And the thing is no. This is always and only a test of quote unquote knowledge of the distribution of word forms. It's not those different things. Right. So this is this is where um this is where you need linguists, people who are used to focusing on the language and understanding that the language itself is this um a layer in between the information that we're using the language to convey and um the what we're doing. So I have this metaphor of raindrops on a window.
All right. So if you're looking through a rain spattered window, you can look at the view beyond and it's going to be shaped like what you can see if it's going to be determined by the patterns on the window. Or you can actually draw your focus in and look at the raindrops instead, right. And there's some neat like photography where people will switch the focus of the camera, like you're either looking at the raindrops or you're looking through the raindrops. And I like to say that the language is the raindrops and linguists spend our time focusing on that.
Um and so we have a lot to say about how language is a real thing and how it affects what you can see on the other side.
ALEX HANNA: Right.
EMILY M. BENDER: And computer scientists who want to pretend that they're just getting at the information by doing natural language processing are totally missing the window and the raindrops.
ALEX HANNA: That's what-- I love this metaphor. That's that's really that's really great. I mean I I think the kind of reduction of some of these things to slotting problems or you know or kinds of things where it's just we're just using language as a mechanism for information I mean I think yeah you're really missing you're missing what this is, right. You're missing the sort of conveying of ideas or the kind of um I mean the whole thing is I'm not a linguist I'm not going to pretend to to articulate all the different you know the different dimensions that's such a good point.
EMILY M. BENDER: All right so you should take us to the next one.
ALEX HANNA: Yeah.
EMILY M. BENDER: We're gonna bounce around and that's.
ALEX HANNA: Okay yeah I wanted to go to this next one um when and where it's AI's uh ENIAC moment and–
EMILY M. BENDER: Grrrrr.
ALEX HANNA: You know so this problem okay so–He's come making this comparison of of of AI and this kind of development of this general machine um and this you know the huge thing and so I should say sort of goes over this history uh really without much of a citation for one of many of the many of the histories of computing. The thing that set me off-- I was set off by two things in this section.
One was this um this paragraph: "To get ENIAC to perform a new task, i's programmers, the hidden figures–" And then there's a list of people and and this is infuriating one because "hidden figures" refers to-- yes Timnit's in the chat just like losing her shit. So hidden figures you know the book and the movie of the same name refer to um not the ENIAC programmers but the programmers in NASA's flight program who were Black women calculators which were a role and and these are all Black women who are doing these calculations by hand and it is a wild omission that you know that he did not name any of the Black women actually involved.
So Katherine Johnson um I'm gonna I'm going to uh look cheat not look look at Wikipedia because I was up on a plane and did it actually when I was reading this and didn't have everyone's name but no mention of Katherine Johnson, Mary Jackson, Dorothy Vaughn who are literally the hidden figures um powering this and you know Katherine Johnson went off to was was honored uh by I think it's a presidential medal of freedom or the Congressional gold medal uh and the National Women's Hall of Fame.
I mean just infuriating that the man here and this is just like set me off to actually use this term but not actually to use it in the kind of lineage that it had in it.
EMILY M. BENDER: And talk about further erasure right?
ALEX HANNA: Yeah absolutely.
EMILY M. BENDER: The whole thing about hidden figures was people's contributions had been erased and so he's doing it again.
ALEX HANNA: Right exactly. So he's doing it again and with this and and I'm like and in addition to this is sort of the kind of thought of the way that I mean he kind of does this not only in his omission of the section but he's also sort of talking about this this kind of dynamic of programmability and this kind of I and it's really um I just really like I was infuriated by this and it was endemic because I think he also later on says "data scientists are the hidden figures of the deep learning era" and I wanted to smash everything.
Because the idea of the data scientist, which is already a very masculinized field, not even mentioning this history and I mentioned I think last time about the the writings by by Janet Abbey, by Mar Hicks for instance on this kind of process of turning this programming which was feminine and was done by Black women into this professionalized engineering field scientific field. I mean that was intentional the idea that women were written out and with it the kind of writing uh and the kind of status that came with that position, the kind of earnings that happened in that system. I mean it was not too long ago when uh women were the most adept programmers and were doing it while balancing childcare, were doing it within the home.
I mean we had remote work in writing software in the 1940s and that history is nowhere here. And this analogy is-- just sets me off, not only by the erasure which is a pretty big um pretty big thing but also this idea that sort of these data scientists are doing this hard uh manual labor, this you know this masculinized work. So it's just there's so much here that I just I'm raging about.
EMILY M. BENDER: Yeah ah. It is it is so, so frustrating. And it's just like he's gonna grab this term that has some cultural cachet and it has cultural cachet um because of the work that other people had done to um you know show the history of these Black women who had been erased from history. And then he just grabs the term and misuses it. But it keeps the cachet and it's just and then he wants to claim it. Well I'm not sure he considers himself a data scientist but like he's sort of saying to the readers you too if you're a data scientist you can be one of these special people, one of the hidden figures and yeah.
ALEX HANNA: But I I don't but I don't think he's saying that. He's sort of saying data scientists are the hidden figures because they're the ones doing this really hard labor of of manually making these models. He actually considers himself a bit more he considers himself and the people at Google and DeepMind that he cites um as the ENIAC creators. The people who are going to save all this kind of labor um you know you data scientists you have done your good uh you know your your duty thank you for the cause. Now let's make you into you know the new uh AI programmers by giving these things instructions and language so it's it's just uh it just this set me up the wall.
So Daniel's thing in the in the chat: "I thought data scientist was roughly the most hyped title of them all. It's hardly hidden."
ALEX HANNA: Yeah right? Wasn't it wasn't it titled the the sexiest um profession of the 21st century by like 18 different Harvard Stanford publications you know?
No. Timnit, we're not doing 10 episodes on this.
EMILY M. BENDER: We're done with it after today.
ALEX HANNA: I do not have the energy.
EMILY M. BENDER: I think that's a cue to move on to the next thing. We could go on for an hour and it would actually be really fun to have Mar Hicks on to hear their um you know–
ALEX HANNA: Oh yeah.
EMILY M. BENDER: –thoughts about this.
ALEX HANNA: I mean I would love just the whole thing about the kind of masculizing of computing I mean this would be a good kind of foil for it but I would.
EMILY M. BENDER: Yeah but yeah.
ALEX HANNA: Yeah yeah all right let's go to the next thing.
EMILY M. BENDER: So I think it's my turn isn't it? Um yeah and oh yeah huh all right I want to go grab the "veridical bias." That was my next one. Okay so we're still um in the uh translation from Turkish to English. Or back in that because I'm I'm hopping around.
So Blaise writes, "This is an example of veridical bias [footnote 38]---meaning that today it's true that more doctors are male than female and more nurses are female than male. The balance is changing over time, though." Hold on. So basically I think the the valuable point in here is to say there are cases where when the system is reflecting the distribution in the actual world we might still not want to use that output. I think that's the–
ALEX HANNA: Yeah.
EMILY M. BENDER: –maybe okay way of saying this although there's a lot of like hops to distribution in the actual world. But the phrase veridical bias kind of pissed me off. I'm like, what's that? That makes it sound like oh there's a kind of bias that's biased towards the truth and it's also one of these things where like "veridical" is one of these fancy sounding words, right. So this this must be a real thing because... Well. So I'm like okay let's follow the footnote.
Um so footnote 38 here um and I got to get down to the um footnotes.
ALEX HANNA: Yeah this is–
EMILY M. BENDER: I can't stand this interface.
ALEX HANNA: So this is the piece by Caliskan, Bryson and uh Narayanan, the "Semantics derived from automatic language corpora."
EMILY M. BENDER: They do not actually use the phrase "veridical bias" in that piece. That is not a term that they coin. Um and what did I get here? They do write, "Our results indicate that language itself contains recoverable and accurate imprints of our historic biases, whether these are morally neutral towards insects or flowers, problematic as towards race or gender, or even simply veridical, reflecting the status quo for the distribution of gender with respect to careers or first names."
They don't call it a bias. They don't call it a type of bias, right. So that little rhetorical flourish just kind of pissed me off and it's got a footnote on it, so if you don't go follow and say but hold on–
ALEX HANNA: Yeah.
EMILY M. BENDER: –what you know is that actually in there, you might think okay fine he got that from somewhere. He did not um he made it up and then just slapped a footnote on it inaccurately.
ALEX HANNA: And this is something I posted this on Twitter, just thinking about his citational practice. You know like and I I wanna I'm gonna use this as an opportunity it's just to be be very you know different fields have different kinds of citational practice. I accept that. Different fields also use different kinds of uses for kinds of thinking about things like how you know how we bring in things like arXiv and pre um pre-prints, right.
But the way that the different uses of citation here are either referring-- Most of the citations and I mean we should just do a count how many of these are arXiv links and for those of you who are not in this field but arXiv is this is a pre-print site, you can publish anything it doesn't go through pre um any peer review and which is fine people will respond and say okay arXiv we use arXiv because um you know stuff comes out so quickly you know whatever.
But it's more of the and I accept that but I'm also going to retort and say his kinds of citational practice is in a way that sort of says we can't do X but this is changing as sort of this kind of thinking and kind of this is happening and it has only been published as an arXiv comment. Or: we have mastered this and it is a Google or a DeepMind blog post, right. I mean there's very much a kind of political economy uh and it's sort of an economy of what gets cited and the citational economy in this right.
And so the real citational practice in this piece really set me off and sort of the citations don't really you know they're not there's claims that are stated, outright for instance, the claims about who was doing the labor in ENIAC, how it actually changed it with no citations to any kinds of historians of technology or science. Most of the citations are these pre-prints um to things that may happen. They're the one paper um that's supposed to prove that you know the machine can do XYZ, that it can be programmed by the language, that it can be uh you know we have this theory of mind which which Emily really knocked down.
I'm just reading the ones on the screen right now and it's really set me off because I think the citational practice on this piece was sloppy uh at best and disingenuous at worst.
EMILY M. BENDER: Yeah yeah and just while we're talking about arXiv and the way it gets used in computer science, and there's this whole like fast science approach. I think it's bad for the field bad for the scientists and whatnot, but I also get really grumpy when you know people will have a peer-reviewed paper and because there's a delay between when you've submitted the camera-ready and when it's actually available to the world people will use arXiv sometimes for that purpose.
Um but then the various search engines privilege arXiv, especially Google Scholar does this. And so you get people pointing to the arXiv preprint, when there's an actual like published peer-reviewed version. And even if it's the same, like it's really important I think to give the citations where you can see the venue, because then you see which ones were peer reviewed
ALEX HANNA: Yeah.
EMILY M. BENDER: And it's possible that some of these things in here that are showing up as pre-prints actually are peer-reviewed and he just can't be bothered to go find that version.
ALEX HANNA: Yeah.
EMILY M. BENDER: But you know also like arXiv 2022, probably not you know.
ALEX HANNA: Yeah I mean I don't think I don't think so and and I mean yeah I mean that's a whole thing with Google Scholar I mean we can hold have a whole thing on Google Scholar because boy howdy the way that that privileges pre-prints and arXiv and actually down like kind of downvotes books and kind of longer manuscripts
EMILY M. BENDER: Yeah.
ALEX HANNA: I'm like I'm seeing a lot in this in the chat by Sergia um talking about having worked with with with Blaise in kind of a fascinating this like kind of thing um saying that um she had worked with Blaise and talked about ethics and safety. I don't think you should feel responsible for all this.
Please don't feel responsible for any of this. Even if you were discussing some of this stuff. So she's saying–or sorry I I shouldn't um presume pronouns. They're saying um cryptic emails about how recommendation systems are changing the consciousness of quote the nations and are briefly touched on the consciousness of AI. Was how people can do more to improve information online and important topics like covid. There are ways other than censorship and free speech. Those are two extremes.
I didn't know I'd grow in all this calling calling Blaise if they are reading this for discussion–yeah and NDA. Well there's a whole thing on NDAs, yeah I'm I'm thank you for sharing that Sergia I mean, and I'm sorry this was a real like traumatizing experience and working with them. I mean that is um really heart-rending to hear.
I hope we are definitely listening to each other and I mean Blaise is writing this in a certain kind of way is definitely not you is and this is also seen a lot of eyes uh and the acknowledgments and we know from personal experiences this stuff has to go through Google publication approval. So many comms people also read this stuff too.
EMILY M. BENDER: Apparently the comms people don't know how to fact check or check the citations.
ALEX HANNA: Yes that's not that that's not their job yeah.
EMILY M. BENDER: Yeah and the thing that that makes that particularly galling is that you know one of the apparent complaints about Stochastic Parrots when people were trying to use the pubapprove process at Google to you know quash our paper, was that we didn't cite things and–
ALEX HANNA: Yeah.
EMILY M. BENDER: –as reviewed I think had 128 citations and then as published it was over 150 and the things that they-- Yeah as Timnit is saying, they didn't tell us what we didn't cite.
ALEX HANNA: Right.
EMILY M. BENDER: It sounds like eventually it was like Google internal work.
ALEX HANNA: It was Google intern yeah it was Google internal work on um their sloppy-ass paper on–
EMILY M. BENDER: No that came later.
ALEX HANNA: –on carbon, yeah.
EMILY M. BENDER: yeah I think that was yeah maybe that was in process because they were angry about the Strubell et al paper.
ALEX HANNA: Yeah.
EMILY M. BENDER: But yeah I guess there was some work about like trying to do more carbon efficient compute and stuff, like that that was happening at Google, that was Google internal and we weren't citing because it wasn't published. So anyway but this doesn't need to be turning into a Stochastic Parrots rehash except we're going to get to that.
ALEX HANNA: Except we're going to get that. Let's do that because I actually want I actually want to get into that because I had a few I had a few things–
EMILY M. BENDER: Okay so–
ALEX HANNA: –I actually want.
EMILY M. BENDER: –this starts here.
ALEX HANNA: But it starts at yeah it starts at, “Is AI fake?” I actually wanted to set the premise a little bit because there's a place where he's he starts and says this um this this paragraph that starts with "AI skepticism is part of a larger backlash against tech companies, which is in turn part of a broad reassessment of the narrative of progress itself, both social and technical."
There's a lot embedded in this statement. There's a lot here that I want to break down and I think it really has to do with kind of why he thinks people have resistances to AI or mathy maths. And it's not just because it is AI I mean people have been resisting lots of other parts kind of parts of of uses of data-full technologies.
There have been resistance to things not only called AI but machine learning also when it was called big data also when it was called data mining. I mean this stuff had backlashes for years. What and this is a bit of revision to say this is a revisionist statement to say that "AI skepticism is part of a larger backlash against tech companies."
The truer claim would be people have been criticizing types of technologies that hoover up data, that are these elements of mass surveillance, and now that they have featured in tech companies and AI has become the the instantiation, now we are encountering this kind of AI skepticism. To say that it is part of this larger backlash really is missing these kinds of resistances to these kinds of technologies for years, okay, and so I am really annoyed with that kind of framing.
Now we have at the nexus of deep learning and these technologies is that to get deep learning to actually work you need huge amounts of data, and to get this you know and to go beyond GOFAI you need these huge amounts of data. And this is only going to be done and possible if you are um you know if you are a big tech firm or if you're one of these specialized firms that focus on AI, such as OpenAI or Anthropic or Clearview or Clarif.ai, these things which are happily taking up all these data and using it towards other kinds of surveillance and kind of potential other civil liberties violating uh uses.
EMILY M. BENDER: Alex I just want to remark on on the way you're using language here because it's a really interesting contrast. So he talks about backlash against tech companies, I think also implying backlash against AI. And backlash sounds like this like sort of reactive reflexive. "No, I don't like that" and you're describing resistance.
ALEX HANNA: Yes.
EMILY M. BENDER: And resistance sounds like you know people are you know looking around at their situation and the systems that are you know systems of oppression that they're having to deal with and figuring out how to collaborate and organize and push back, and those are those are two very different descriptors and I think resistance is a much better lens to look at it through.
Um and talking about it as backlash sort of also buys into this victim narrative that the deep learning people want to cling to that, right, "nobody believed us.” "People you know laughed us out of the room." "We are we are the victims here.” And "hah! we're showing them." And now we're experiencing backlash so once again we are the victims and–
ALEX HANNA: Right.
EMILY M. BENDER: –no, you're not.
ALEX HANNA: Right.
EMILY M. BENDER: You're sitting on all the money and all the power, you're not the hidden figures right.
ALEX HANNA: Yeah, right. Yeah yeah and I mean he's really I mean and I mean he is obviating this in a very easy way I mean he sort of says in the pair-- in the prior paragraph too he cites Sasha Costanza-Chock who says that you know when people when when Abeba asks what is artificial intelligence you know um the site this first citation, "A poor choice of words in 1956" was by Michael Zimmer who's an who's an ethicist and um researcher at Marquette. "It is nothing" is some random Twitter account and then and then Sasha Costanza-Chock who's–Sasha who is the director of research and design at the Algorithmic Justice League says, "A glossy pamphlet papered over a deep fissure where underpaid click work meets ecologically devastating energy footprints, in a sordid dance w/VCs ending in the reproduction of the matrix of white supremacist capitalist cisheteropatriarchal settler colonial abilist domination," which is a very good summary.
And is also you know he doesn't address that at all um or take that on it's like you know like okay would you actually like to address any of those other than saying ignoring it and just saying these people are luddites who are against technology um and their ludditism you know is impeding this real scientific work.
EMILY M. BENDER: Yeah, okay.
ALEX HANNA: All right you're you're–all right.
EMILY M. BENDER: Sasha has a way with words, yes we're definitely in the same space right, so on the next paragraph here yeah, "These anxieties relate to AI in a number of ways." Let's just pause and reflect that this is establishing or presupposing AI is a thing that exists in the world um and always ambiguous between the research program of AI which yes is a thing that exists in the world and the mathy maths which don't exist, at least the way they're constructed as as you know through the hype.
"One worry is the direct ecological impact of large language models although in real terms this is small today." Here's the obligatory footnote pushing back um against-- uh oh no it's not it's somewhere else.
ALEX HANNA: What is this?
EMILY M. BENDER: Maybe it's just a remark, so he's basically saying yeah yeah this has been overstated.
ALEX HANNA: Oh yeah it's a it's in another one I mean it's in another it's in another foot note. He he uses that to sort of nitpick on uh Kate Crawford's Atlas of AI book yeah.
EMILY M. BENDER: All right so, "Another very real con-- Another is the very real concern that AI-enabled systems learn human biases, thereby potentially worsening social inequity when such systems, are deployed especially in consequential settings such as credit approval or criminal sentencing." So I wanted to um just talk about the framing of this paragraph. So anxieties, again. Anxiety is a real thing that people actually experience like when you are feeling anxious. You are feeling real feelings um but it is often something that comes up when your brain is sort of flooding you with fear in a case where you don't want it, it's not helpful.
So using the term anxiety here um sort of sets this up as oh those people, those silly little people are just worrying about stuff they shouldn't worry about. Um and yes "it's a very real concern that AI enabled systems learn human biases." But that just sort of feels like it's captured and set aside in a way.
And I'm thinking about the really cool talk that Anna Lauren Hoffman gave at the Berkeley group AFOG a couple weeks ago, where she pointed out there's this whole metaphor now of AI being a child that needs to learn and then also all this language that talks about the potential that goes with the child that's going to grow and learn right. And we have to balance the potential with the risks of harm. And she points out that that um that framing basically sort of captures and neutralizes all this work that's been done pointing out the harm, and I feel like this is sort of in that same mode and–
ALEX HANNA: Yeah.
EMILY M. BENDER: –felt so much easier so much more able to spot it after hearing her talk and so yeah great that was a really useful thing to learn. I'm really glad that it was to get to that talk.
ALEX HANNA: Yeah she's she's writing a whole book on that so keep your keep your eyes out on–
EMILY M. BENDER: I'm excited about that book and it's not going to go on arXiv I bet, right, it's going to be a book.
ALEX HANNA: No, it will be a book, a book book.
EMILY M. BENDER: Yes, book book book. Yeah yeah okay yeah. "Perhaps, too, there's a more inchoate anxiety about human uniqueness which we associate closely with our intelligence." Let me tell you, the people who are upset about or you know putting time and effort into trying to push back on all these harmful applications of mathy math are not doing it because we're afraid that our humanity is is going to become ununique because the AI is going to be so great it's going to be like-- No that is not where we're coming from.
ALEX HANNA: No definitely not. I mean this is maybe that's an anxiety that one has you know if you're a human in this field that thinks you're so great.
EMILY M. BENDER: Yeah we have to explain mathy math because we failed to do it at the beginning this time.
ALEX HANNA: Oh yeah.
EMILY M. BENDER: Timnit's asking in the chat. So um we were objecting, I think in episode one, to the way that he uses the the term AI to refer to a specific system, right. So there's an AI right. Or the AIs are going to do this. And you know as many people have pointed out in fact one of those tweets was was you know this was a bad choice of words in 1956. It does not help to call either the research program as a whole or specific instances of systems AIs, and so Alex came up with the brilliant rephrasing as mathy math and so–
ALEX HANNA: Yeah.
EMILY M. BENDER: –we try to use it.
ALEX HANNA: Mathy math and the same in the same lineage as Boaty McBoatface
EMILY M. BENDER: Okay and Parsey McParseface for that matter.
ALEX HANNA: Let's let's okay we got we got 15 minutes left. He dogs on Stochastic Parrots in this parenthetical, "yes that parrot emoji is part of the title.” I think he's just a hater there.
EMILY M. BENDER: I notice that he can get the parrot emoji in the title, good that's part of the title. He can't get my M in my name.
ALEX HANNA: So you know it's it's in things–
EMILY M. BENDER: But the thing I wanted to say here-- So footnote 61 is the rebuttal to section three where it was like but Google is actually not so bad on energy like–
ALEX HANNA: Right.
EMILY M. BENDER: –that's not the point yeah um but um so first he quotes Gary Marcus right, saying ‘neither LaMDA nor any of its cousins are remotely intelligent’ um “and then a similar position was articulated two years earlier by Emily Bender and colleagues–” First of all Stochastic Parrots is jointly first authored with Timnit, so it should always be cited as Bender, Gebru et al and it's not just me, but um fine um and, no, my position is not similar to Gary Marcus's right. I am not interested in saying uh language models LaMDA etc are not AI, a better way to do AI is whatever he does with his neurosymbolic processing.
My interest is to say, you're making unfounded claims and those unfounded claims lead on to various other harmful things. But yeah yeah so anyway that I wanted to react to that and then the other thing I want to react to here.
Um a little bit further down it says "real insight". Where did that come from? Um yes "Whether meaning can be gleaned from language alone is a long-standing debate, but until the past decade it has been a fairly abstract one."
Um so first of all I have a paper in 2020 with Alexander Koller, where we argue that you can't learn meaning from the form of language alone. But Blaise here isn't the linguist and so he doesn't understand that that language is a symbolic system with a form pairing meaning, and so he's like misstated the problem in the first place.
Um but also then he goes on to say "Real insight began to emerge with word2vec" which is one of these first sort of neural approaches to language modeling. Um and it's like no all of the work in linguistics prior to 2013 that's looking at at the relationship between form and meaning, all the work on distributional semantics before then, none of that is real insight? Real insight is when the engineers come in and throw their mathy math at it?
I don't think so. Anyway, your turn Alex.
ALEX HANNA: No no this is great I yeah I mean the kind of thing that you're saying is like if you you've you've had a chance now to put things in an embedding space and truly now that's the real work. All right all right we've got 14 minutes. Damn. Okay. This is like like it feels like a lightning round. Okay uh there's a comment about Nazis, which was questionable so… uh where he says, "A reminder that even about Nazism argument is isn't universe– agreement isn't universal." This is footnote 74 and I was like yes and Nazis should be shunned actually.
Okay. I wanted to actually kind of skip ahead to this things about planet- planetit- planetarity. Okay. All right. So he says in the beginning of this as planetarity. This is what he's getting at and his follow-up here is non-kind of nonsense this idea of oh like and here he's criticizing people who suggest that you should take out things that are toxic in a data set because he's saying well then you don't know how to model it. And I have a lot of problems with this um but I also want to but I actually didn't want to even go after him on those grounds.
I want to come after him on this on this paragraph, in which he says, "Ultimately, as a society we should aim to build a ‘foundation model’--” Again I'm going to put that in scare quotes. “--that includes every kind of digital representable media reflecting every constituency, perspective, language and historical period. The natural world, too--- why should it not include whale song, bacterial genomics and the chemical quote languages of fungi? The scientific, technological, and ecological potential of such a model would be hard to overstate." And I just wrote and this is me paraphrasing Claire Stapleton in one of her newsletters, "That's colonialism, Sweetie."
And this is um so really this idea that every kinds of digital kinds of media that is available–his argument is to have such a totalizing model. We need to have everything of this to put it in one kind of model that would actually be the most democratic thing we could do. No that is the Borg model, the Borg model of assimilation and of domination and even for a model to know these knowledges would be a dominating procedure. There are things that are not exposed because they are matters of defense.
Um and that comes from kind of the Black tradition and Black feminist tradition of defense against the collection of all kinds of data that comes in Indigenous traditions, that certain kinds of knowledges should not be known to some kind of state or some kind of sovereign, does not being recognized. And in this we're getting to this question of governance again a foundation model should not from a perspective of justice know everything. That is not uh that is not even dignitarian in proposal even according if you if you take seriously something like the GDPR, that does not even fit within that proposal. But it doesn't even fit it doesn't fit into these proposals that are more Indigenous in nature.
Um so I was I that just really um that really set me off in in in in in a really strident way.
EMILY M. BENDER: yeah yeah I think we need to explain the Borg metaphor for the–
ALEX HANNA: Yeah, yeah.
EMILY M. BENDER: –non-Trekkies in the audience.
ALEX HANNA: Yeah so the Borg um so the Borg are this race in Star Trek and you know the kind of they are known for saying uh you know for not um they go to other cultures and if they find something that's technologically advantageous, they integrate it into their culture uh in in into their and they do it but they do it by dominating everybody basically. They they kind of erase a whole society and then integrate that technology into their bodies or into their spacecraft.
EMILY M. BENDER: Isn't it like one big like shared mind?
ALEX HANNA: Yeah.
EMILY M. BENDER: Not individuals within that?
ALEX HANNA: Yeah it's like a hive mind. They don't have individuality which I think is kind of interesting and I actually kind of like that part of them um because they're always collective. But then the Borg collective is more like the Borg empire you know. They're they're they're they're dominators right, they're not–
EMILY M. BENDER: There's a difference yeah between and collective and sort of like forced assimilation.
ALEX HANNA: Yeah it's it is like a forced and their literal thing is you will be assimilated right yeah.
EMILY M. BENDER: Resistance is futile isn't it?
ALEX HANNA: Resistance is futile, you will be assimilated. So–
EMILY M. BENDER: Yeah.
ALEX HANNA: –if you want to know more watch the end of season two or three whichever one where um Picard becomes uh assimilated as Lucutas. Anyways okay Borg all right yeah all right nine minutes nine minutes left, let's let's do it yeah it's your turn.
EMILY M. BENDER: Yeah my turn. I guess what I wanted to to come down to um so there's I don't want to leave too quickly, your point about this like the planetarity, the totalitizing sort of just completely colonizing metaphor that's going on here is really problematic.
Um and it's like the that this is something to aspire to as a problem on one level. And then on the other level there's this idea that it would work, that by somehow feeding all this data in it would have that knowledge and that's also not true right so yeah it's a bad idea and it wouldn't work.
ALEX HANNA: Yeah.
EMILY M. BENDER: But it doesn't mean that it's harmless to pursue it because all kinds of harm that can happen, both through claiming that it works and in the data collection that would get there. But I'm going to I'm going to take us down to the very last ones that I have and then we can go back up.
Um this is just back to pure AI hype So I have to spell it right. Episodic. "However, one of the shortcomings of transformer models like LaMDA today is their limited short-term memory coupled with an inability to form long-term or episodic memories on the fly the way we do." Let's go check footnote 92. Zoom is getting in my way.
What does footnote 92 say? "Many research groups are working on adding these capabilities. They're unlikely to be long-term roadblocks." So this is like a citation to the future. Okay so where it is here it is this is, "Shortcomings of models like LaMDA today" suggests this path of progress where okay this is just temporary. This is going to happen right. We're going to get there.
"Limited short-term memory coupled with an inability to form long-term or episodic memories–" This whole thing is just a category error. LaMDA is not thinking, it doesn't have a mind. It doesn't have a model of the world that it's integrating information in. It is just learning distributions of word forms in text and um then in the fine-tuning stage some additional sort of preferences about which ones to output, based on these labels that came from humans who were told that they were labeling things like sensibility and inoffensiveness and whatever the other ones are.
Like this is not a description of what LaMDA is actually doing.
ALEX HANNA: Right.
EMILY M. BENDER: And let's remind ourselves that this thing was approved by Google. So this is this isn't just Blaise speaking. This is Google coming in with some absolutely false AI hype about um-- It's subtle though, right, because its saying well it doesn't do it now but it's making it sounded like something that this type of thing could do and it's just a question of developing it a bit further.
ALEX HANNA: Right.
EMILY M. BENDER: And that that's really not okay.
ALEX HANNA: And that's really that I mean that's emblematic of the citational practice too, you know.
EMILY M. BENDER: Yeah well we're gonna we're gonna fight the future!
ALEX HANNA: We're gonna we're gonna get there we're gonna get there.
EMILY M. BENDER: And then one other quick one like this and then I'm going to give the floor back to you Alex.
So he talks about how uh LaMDA quote never worth reading those– "Exchanges like these highlight the way communication is inherently an act of mutual modeling. Lemoine models LaMDA and LaMDA models Lemoine. Lemoine models LaMDA's model of Lemoine and LaAMDA models Lemoine’s model of LaMDA and so on." No, not true that is a description of the joint activity that humans do when we use language to communicate with each other, but there's no evidence at all that language models are doing that.
Um and you know this is like but that goes on this other thing about "inner monologue," which is sort of language model driven robot control systems. "This suggests that intelligence within the robot may also be dependent on mutual modeling within a kind of Society of Mind." That does not exist. It is not a thing this is just–
ALEX HANNA: Yeah and the Society of Mind the thing is is a citation to Minsky you know when it's in this old AI Pioneer um which I don't know what that means I think uh you know this is somebody that's really uh invested in the project. Yeah the Society of Mind and this one thing so yeah.
EMILY M. BENDER: So that was my last one but you've got five more minutes. Do you want to another one?
ALEX HANNA: Well I'm gonna take the last the my last thing to have this thing where it starts as, "It is acting" and this kind of idea of moral agents.
EMILY M. BENDER: Oh yeah.
ALEX HANNA: It's a moral yeah moral I think moral agency is the key word here.
EMILY M. BENDER: Okay.
ALEX HANNA: Yeah so okay so um yeah okay so yeah.
EMILY M. BENDER: Agency is agency earlier?
ALEX HANNA: I think it's the one where it says, "LaMDA can be said to have moral agency." That one.
EMILY M. BENDER: Yep that was in my notes too but I'm not picking it up.
ALEX HANNA: Yeah, I mean the um I just while you're finding it I mean I really um you know like I while you're while you're getting there I mean the kind of thing that I want to get at here because he does this kind of dodge here which is um this idea of the comparison of a model that I think another philosopher makes and says. You know it's more like a search engine or something of that nature which I would probably agree with a bit more, but he says that these things are agents.
So this uh this Allison uh Gopnik um who's I think is a psychologist who gave a talk at that Simons-- Simons Center on this. Now this kind of thing that it is an agent that interacts with you. I went to this I went down this rabbit hole of uh Actor Network Theory which is a field within um which was within Science and Technology Studies. I'm not going to do any kind of justice and explaining Actor Network Theory in three minutes, but the tldr on it is thinking about actors and what what Latour, Bruno Latour who's a a Science and Technology Studies scholar, calls, and he says you know this we can think of different kinds of technologies as actants.
So they're kind of big or more radical part of it is he says that um techno certain kinds of technologies can be actors. And so I don't actually disagree with the sort of statement that there are robot agents, that they are exhibit quote-unquote agency. That is, I I don't I don't really buy everything from Latour but I'm willing to suspend disbelief for a bit.
But that then the claim to say that moral-- LaMDA can have moral agency, that is patently false. Because agency requires and more morality requires intention and requires some kind of thought that one is going to uh intend to do something uh and consider some of those consequences. And to call LaMDA or Inner Monologue or Gato or whatever these things as moral agents.
This is highly objectionable. And here he also refers to Joanna Bryson's work uh namely the kind of idea in this piece by Joanna Bryson called, "Robots Should be Slaves.” Which, first off, what the fuck are you doing?
And she had kind of an apology in 2021. It actually wasn't an apology. It was it's wild in 2020 that we would be talking about robots as slaves. Um and in it she's sort of in the original piece she says something to the degree of um "you know we used the word slaves before the African slave trade." Okay but the African slave trade is paradigmatic as this notion of slavery and you cannot talk about slavery without talking about the Middle Passage and the immense amounts of violence, dispossession, and genocide that that was. And so the idea of kind of and and I get the point saying Bryson is saying robots should be wholly subservient to humans.
But to use that language is–and that he's citing it is is really–
EMILY M. BENDER: Just calling it "provocative."
ALEX HANNA: Yeah calling it "provocative" and not what it is uh which is you know playing into these kinds of ideas of of structural racism and non-acknowledging this um violent history uh violent colonial history.
Oh my God yeah all right um so.
EMILY M. BENDER: We're at time!
ALEX HANNA: We're at time. Oh my god yeah. Oh my gosh all right so this we're done with this article. We don't have to deal with it anymore. There's going to be an episode four and we are going to have an episode on AI art uh and I think what we're going to do is convene a few people together.
Um the preview I think we're going to have um I'm going to ask them if they want to come for probably some folks. I'm actually going to name them not name them yet.
But that will be soon? Eventually? I don't know, but that will be episode 4 in our series.
EMILY M. BENDER: This is fun so I'm totally game to keep doing it. They are next and
then we'll see. There's unfortunately an ending supply of AI hype to–
ALEX HANNA: Yeah.
EMILY M. BENDER: –suffer through. And so thank you for joining us in the suffering.
ALEX HANNA: Thank you all for bearing with us. See you next time Emily!
EMILY M. BENDER: See you, bye!
ALEX HANNA: Bye!
ALEX: That’s it for this week! Our theme song is by Toby Menon. Production by Christie Taylor. And thanks, as always, to the Distributed AI Research Institute. If you like this show, you can support us by donating to DAIR at dair-institute.org. That’s D-A-I-R, hyphen, institute dot org.
EMILY: Find us and all our past episodes on PeerTube, and wherever you get your podcasts! You can watch and comment on the show while it’s happening LIVE on our Twitch stream: that’s Twitch dot TV slash DAIR underscore Institute…again that’s D-A-I-R underscore Institute.
I’m Emily M. Bender.
ALEX: And I’m Alex Hanna. Stay out of AI hell, y’all.