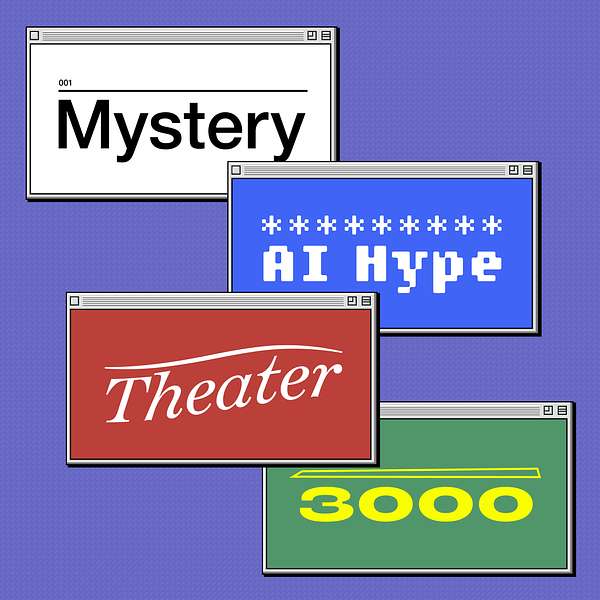
Mystery AI Hype Theater 3000
Mystery AI Hype Theater 3000
Episode 20: Let's Do the Time Warp! (to the "Founding" of "Artificial Intelligence"), November 6 2023
Emily and Alex time travel back to a conference of men who gathered at Dartmouth College in the summer of 1956 to examine problems relating to computation and "thinking machines," an event commonly mythologized as the founding of the field of artificial intelligence. But our crack team of AI hype detectives is on the case with a close reading of the grant proposal that started it all.
This episode was recorder on November 6, 2023. Watch the video version on PeerTube.
References:
"A Proposal for the Dartmouth Summer Research Project on Artificial Intelligence" (1955)
Re: methodological individualism, "The Role of General Theory in Comparative-historical Sociology," American Journal of Sociology, 1991
Fresh AI Hell:
Silly made-up graph about “intelligence” of AI vs. “intelligence” of AI criticism
How AI is perpetuating racism and other bias against Palestinians:
The UN hired an AI company with "realistic virtual simulations" of Israel and Palestine
WhatsApp's AI sticker generator is feeding users images of Palestinian children holding guns
The Guardian on the same issue
Instagram 'Sincerely Apologizes' For Inserting 'Terrorist' Into Palestinian Bio Translations
Palette cleanser: An AI-powered smoothie shop shut down almost immediately after opening.
OpenAI chief scientist: Humans could become 'part AI' in the future
A Brief History of Intelligence: Why the evolution of the brain holds the key to the future of AI.
Check out future streams at on Twitch, Meanwhile, send us any AI Hell you see.
Our book, 'The AI Con,' comes out in May! Pre-order now.
Subscribe to our newsletter via Buttondown.
Follow us!
Emily
- Bluesky: emilymbender.bsky.social
- Mastodon: dair-community.social/@EmilyMBender
Alex
- Bluesky: alexhanna.bsky.social
- Mastodon: dair-community.social/@alex
- Twitter: @alexhanna
Music by Toby Menon.
Artwork by Naomi Pleasure-Park.
Production by Christie Taylor.
ALEX HANNA: Welcome everyone to Mystery AI Hype Theater 3000, where we seek catharsis in this age of AI hype. We find the worst of it and pop it with the sharpest needles we can find.
EMILY M. BENDER: Along the way we learn to always read the footnotes and each time we think we've reached peak AI hype, the summit of Bullshit Mountain, we discover there's worse to come. I'm Emily M. Bender, 1950s version, a professor of linguistics at the University of Washington.
ALEX HANNA: And I'm Alex Hanna, director of research for the Distributed AI Research Institute. This is episode 20, which we're recording on November 6, 2023 and we're going to do some time travel. Picture it, the year is 1956. Women's fashionable--women's fashion is pretty uncomfortable. The study of so-called thinking machines is in its infancy and on the campus of Dartmouth College in Hanover, New Hampshire, some male computer scientists are about to spend their summer vacation quote founding the field of quote artificial intelligence.
EMILY M. BENDER: This was the Dartmouth summer research project on artificial intelligence, and from the beginning it was hype-tastic. Today we're going to look at the grant proposal that funded the summer workshop, with researchers John McCarthy, Marvin Minsky, Claude Shannon and Nathaniel Rochester, based on quote "the conjecture that every aspect of learning or any other feature of intelligence can in principle be so precisely described that a machine can be made to simulate it." Which has already given me flashbacks to our episode on Artificial General Intelligence. But we're here to witness how AI hype is as old as the field of AI itself, and to see what forms it took in 1956.
ALEX HANNA: Oh gosh and for for y'all listening in, that can't see us, uh Emily's got this amazing um uh uh um Daphne-style wig on from Daphne from Scooby-Doo.
EMILY M. BENDER: And these are meant to be cat-eye glasses here.
ALEX HANNA: They're amazing horn-rim glasses with these beautiful gold inlays uh and this and this wonderful uh uh purple cardigan. Uh I've got on uh kind of a um just my regular glasses, but also like a tie and a shirt my and and and and a coat. Uh I I was going to get a bow tie but I didn't have time. Um you know you can't--you you take what you can get here.
EMILY M. BENDER: Yeah so I I pre- purposed this outfit for my Halloween costume, and everyone thought that I was trying to be something from Scooby-Doo. I'm like no. And initially I was saying I'm a computer operator from the 1950s, until Christie our producer said 'Doesn't that make you a computer?' Like yes, in fact I'm a computer from the 1950s.
ALEX HANNA: Literally. Literally a computer.
EMILY M. BENDER: Also probably look something like the person who did the typing in this artifact we're about to look at.
ALEX HANNA: That's right.
EMILY M. BENDER: Because as as we're reading this--all right here we go, here's the artifact. Um this is a--you can see it, I can't see it yet. Let me fix that um.
ALEX HANNA: Yeah we're--this is the--"A Proposal for the Dartmouth Summer Research Project on Artificial Intelligence." I printed it out just to feel much more retro, uh in in in this very nice um um typewriter set, um probably mimeographed to all hell. Um.
EMILY M. BENDER: Yeah.
ALEX HANNA: Et cetera. Yeah.
EMILY M. BENDER: Yeah um. So this is um it--yes definitely mimeographed multiple times, um and you know I'm a little surprised we don't see any comments in the chat yet. I'm wondering if we are actually streaming on Twitch?
ALEX HANNA: Oh we are.
EMILY M. BENDER: We are? Okay I guess we have just like bowled over our audience and people can't stand the costumes, um, so. [Laughter]
ALEX HANNA: Yeah so there's some hellos. Yeah.
EMILY M. BENDER: Yeah. So this was clearly typed on a typewriter um, and therefore not typed by any of the authors right. We're talking 1956 um these are you know um--there was some secretary somewhere who took probably some longhand notes that were written. Probably fixed the grammar, probably fix the you know argumentation in several places. Anyway.
ALEX HANNA: Right.
EMILY M. BENDER: You know the project 'Thank you for typing?'
ALEX HANNA: Yes--no I don't know that project. That's that's wonderful, I I get it immediately. Yeah.
EMILY M. BENDER: Um okay um so where are we going in this, Alex? You've got the hard copy, I've got it digitally, what are we doing?
ALEX HANNA: Yeah, so the first page--I mean so we can't search here but and I think this is um you know uh the--you mentioned it in your intro Emily, um but you know that they describe the parameters of the project, uh you know, "We propose a two-month uh 10-man study uh--" And literally men. uh "--of artificial intelligence be carried out in the summer of 1956 at Dartmouth College in Hanover, New Hampshire."
And then the quote here, um "The study is to proceed on the basis of the conjecture that every aspect of learning or any other feature of intelligence can in principle be so precisely described that a machine can be made to simulate it." And so this kind of language of artificial intelligence, just to contextualize it, the language of artificial intelligence is used you know.
And it's it's it's typically uh attributed to John McCarthy um and sort of as a means of um basically uh warding off other competing sort of schools of thought around this, notably um uh notably Norbert Wiener--Um I'm saying that right?--um and in his study of cybernetics. Uh basically kind of a personality thing, we want to like head him off at the pass.
Um and so there's a bunch of people um that are convening at this conference and it's it's sort of this titular event that is used to uh kind of have the foundation of that. Although there's been other histories uh written that have that have gone and said, you know there's a lot of different things done in this.
EMILY M. BENDER: I want just just briefly look at the budget here. Do do know you who the funder is of this?
ALEX HANNA: Rockefeller, Rockefeller is the funder. Yeah, yeah so. Before we get to the budget, because I got stuff to say about the budget too, um this is the proposal for Rockefeller and I forget if Rockefeller provided the um the requested budget.
But we can get to it. Uh so they talk and they say--and and just the first graph is is helpful just to contextualize it. So after they say um we're trying to describe the brain, basically so that the machine can simulate it, they say, "An attempt will be made--" Love that passive voice. "--to find how to make machines use language, form abstractions and concepts, solve kinds of problems now reserved for humans, and improve themselves. We think that a significant advance can be made in one or more of these problems if a carefully selected group of scientists work on it together for a summer."
Which this is just such a funny hubristic statement.
EMILY M. BENDER: Yeah. Although I have to say that--and this is foreshadowing a bit when we go look at the language thing--they're not actually talking about natural language processing. They're talking about designing programming languages there.
ALEX HANNA: They are, they are, and there's some elision here and again--I've mentioned this dissertation a few times because it it is a nice history, of Johnnie Penn, uh and the way that um developing programming languages, there is elision in the and thinking that programming languages themselves as being kind of a means of what is scoped as artificial intelligence. Which which I think is is is kind of fascinating itself. And so they're all kind of talking about different things. Um but yeah let's there's there's a few different sections in in in in in what what these are and I wonder if we want to get into this.
Uh there there's kind of seven uh sub-problems and this this thing itself is contained of four subproposals of the initial conveners um, so McCarthy, Minsky, Shannon and then Newell and Simon have a joint one.
EMILY M. BENDER: Yeah. But I think yeah we should go through these um quickly, these aspects of the artificial intelligence problem, and I love how already it goes from you know, the title is "Artificial Intelligence," we're going to work on something called artificial intelligence--my apologies for the background noise there--um and then it's "the artificial intelligence problem."
Like that's that's just like a thing that we're going to assert exists and here's how we you know conceptualize it.
So "Automatic Computers," as opposed to the human computers like me, um "If a machine can do a job, then an automatic calculator can be programmed to simulate the machine." Okay, fair. "The speeds and memory capacities of present computers may be insufficient to simulate many of the higher functions of the human brain--" Wait, what, human brain? We were talking about machines. "--but the major obstacle is not the lack of machine capacity but our inability to write programs taking full advantage of what we have."
And so presupposed in this is the idea that brains are machines.
ALEX HANNA: Yeah.
EMILY M. BENDER: Um and it's not argued for it at all, it's just it's just presupposed there.
ALEX HANNA: Yeah it is it is it is uh just stated. The next one, "How can a computer be programmed to use language: It may be speculated that a large part of human thought consists of manipulating words according to rules of reasoning and rules of conjecture. From this point of view formalizing a generalization consists of admitting a new word in some rules whereby sentences containing it imply and are implied by others. This idea has never been precisely formulated nor have examples been worked out."
Um and then one in in the kind of marginalia, I think one of the I think this is um what's his name Solomonoff, one of the kinda of invitees, says, "I could certainly write a lot about this." Which I think is kind of funny but anyways.
EMILY M. BENDER: Um and this is this is interesting because this is uh not distinguishing between language per se and reasoning in language, right? This is like how a computer can be programmed to use a language and then what they're talking about is human thought. Um.
ALEX HANNA: Yeah.
EMILY M. BENDER: So you know and it's obviously very speculative. Okay, part three, "Neuron Nets: How can a set of hypothetical neurons be arranged so as to form concepts. Considerable theoretical and experimental work has been done on this problem by Uttley, Rashevsky and his group, Farley and Clark, Pitts and McCulloch, Minsky, Rochester and Holland, and others. Partial results have been obtained but the problem needs more theoretical work."
So well there's two things that are interesting to me here. One is that that they're called 'neuron nets' here but we're going to see some different words um yeah in the in the body of the text. And also that this is--so first of all they they um acknowledge that they're hypothetical neurons, I appreciate that, um and what they expect them to be forming is concepts. Which is--I guess that persists a bit. Um the people who work with representation learning think that those representations are concepts, but it's gotten far fuzzier, I think, in the intervening decades. Um like what is it they're expecting to find.
ALEX HANNA: Yeah, there's a few--there's a bit of work on what is considered a concept, so for instance I know there's there were people at Google working on um TCAVs or uh concept activation vectors. I forgot what the T stands for. Um, but it's really unclear what they mean by concepts and it's I haven't seen really good explanations of that um it's and and it's so--yeah I don't I don't know like what the scope of that explaining what a concept is here in that work.
EMILY M. BENDER: Yeah.
ALEX HANNA: Uh okay moving on. "Theory of the Size of a Calculation: we--if we are given a well-defined problem," uh parenthetical, "(one for which it is possible to test mechanically, whether or not a proposed answer is a valid answer)" end parenthetical, "one way of solving it is to try all possible answers in order. This method is insufficient and to exclude it one must have some criteria for efficiency of calculation."
Um, "Some consideration will show that to get a measure of the efficiency of a calculation, it is necessary to have on hand a method of measuring the complexity of calculating devices, which in turn can be done if one has a theory of complexity of functions. Some partial results on this problem may have been obtained by Shannon and also by McCarthy."
Um I mean okay, this in itself is not is probably not a bad sort of thing to mention and I think I don't know if this is um um pre uh a prelude to the work on just kind of uh Big O and and Big you know Omega kind of complexity computations, I don't know enough about the history of those--of those devices or not. Are you familiar with--do you know more about that Emily?
EMILY M. BENDER: I I I know roughly what they are and how to use them, but I don't know their history um--
ALEX HANNA: Okay.
EMILY M. BENDER: --so but this is what it sounded like to me too, that this is basically you know Big O Notation because this is really early in computer science right, and it's sort of interesting to me that um AI was uh sort of so intertwined with the project of computer science this early.
ALEX HANNA: Yeah.
EMILY M. BENDER: And then you know when I came up, and you know through linguistics but there were computer scientists around me, AI was like you you did not claim to be working on that. That was just ridiculous right. So computer science is entirely separate from AI through like the you know 80s and 90s which is where, 90s is where I was experiencing it. Um.
ALEX HANNA: Yeah.
EMILY M. BENDER: So this is like just yeah throwing we're throwing in programming languages, we're throwing in Big O Notation, like this is this is all artificial intelligence for them.
ALEX HANNA: Yeah.
EMILY M. BENDER: All right. "Self-Improvement: "Probably a truly intelligent machine will carry out activities which may be best described as self-improvement. Some schemes for doing this have been proposed and are worth further study." No citations in this one. "It seems likely that this question can be studied abstractly as as well." So what's what's striking to me here is, um it's not called self-improvement anymore, but the idea that systems can learn things beyond their programming is sort of core to how we talk about art--how people talk about artificial intelligence now. Um.
ALEX HANNA: Right.
EMILY M. BENDER: And also this 'a truly intelligent machine,' like you hear stuff like that in 2023, this is this is like we have--we haven't defined intelligence but we are going to distinguish between something that is and isn't by calling out the ones that are truly intelligent and ascribing these properties to these hypothetical things. So that it's an interesting sort of prefiguring of the way the hype comes across now.
ALEX HANNA: Yeah, yeah, no completely. And I think it's there's sort of different ways I think self-improvement has had different guises, some in reinforcement learning some in um in kind of like active learning but then also kind of the elusive notion of emergent properties I think also has a lot of flavors of this.
EMILY M. BENDER: Yeah.
ALEX HANNA: Um okay, number six, "Abstractions: A number of--a number of types of 'abstractions'--" in quote "--can be distinctly defined in se--several others less distinctly. A--a direct attempt to classify these to describe machine methods of forming of abstraction ab-- abstractions from sensory or other data would seem worthwhile." This is this is so abstract. [Laughter]
EMILY M. BENDER: But this is--this comes back in one of the specific proposals, we we'll see it, basically saying you know 'if if the machine can come up with generalizations over what its sensors are saying than it has learned something.'
Like this is sort of you know a lot of wishful thinking here. Um and you know I have really I have really mixed feelings about this you know this is full of language like 'would seem worthwhile' and I'm like yes there should be science funding for scientists to just go and explore things without having to like make the case that it's gonna you know uh you know further the military project or whatever the thing is--
ALEX HANNA: Right.
EMILY M. BENDER: But on the other hand it's like seriously? They got this funded with this kind of a weak proposal. [laughter]
ALEX HANNA: Yeah I mean fun-- funders. And yeah funders were more--and and I forget I want to I'm gonna I'm gonna dip out to Wikipedia in a second just check how much of this got funded, to see if if it did, but I mean I I believe it did get funded to the full uh extent and then I'm going to like probably also check another source.
EMILY M. BENDER: Yeah.
ALEX HANNA: Yeah. But let's let's let's take number seven last. You want to take that Emily?
EMILY M. BENDER: Sure. "Randomness and Creativity: A fairly attractive and yet clearly incomplete conjecture--" This is--what kind of a grant proposal? Okay. "--is that the difference between creative thinking and unimaginative competent thinking lies in the injection of some--of a some randomness. The randomness must be guided by intuition to be efficient. In other words, the educated guess on the hunch include controlled randomness in otherwise orderly thinking."
I have a feeling that that this was scrawled so incoherently that the secretary who did the typing couldn't quite make sense of it, that's why there's so many like weird grammatical artifacts in here. Um but this is basically uh 'we can't just create algorithms for things we haven't understood yet, we want the so-called 'AIs' in quotes to be able to solve problems for us, so we have to figure out how to get them to do things that they aren't programmed to do.' I think is what that boils down to.
ALEX HANNA: Yeah, yeah. Um and I I think kind of what it is also getting at is a notion of kind of um heuristics. So rather than thinking about kind of--and I mean this is you know like if you want to sort of get at something, you need to have something that guides you towards things but we can't really define it and so maybe this is like a sort of--this is a 'and everything else' sort of category.
EMILY M. BENDER: Yeah, and it does it does come up in the specific proposals. I mean what I do have to give them is that these seven things, I think all of these themes are called out in the proposal.
So they must have like collected those for and then written this summary afterwards.
ALEX HANNA: Yeah.
EMILY M. BENDER: Yeah. All right I think we don't have to do the what their plans for the summer was, but--
ALEX HANNA: Yeah, I think we--I I do want to like before we go I do want to kind of just note out who the people are um. And so the first one is, "Originators of this proposal are one C.E. Shannon, mathematician uh Bell Telephone Laboratories." Um and it gives a little bit of his background, um kind of in focusing he and Tom McCarthy on um a theory of automata. Uh, "Marvin Minsky, uh Harvard Junior Fellow in Mathematics and Neurology." And then this is this is another this is the instance of another word here for neur neur--neural nets where it says, "Minsky has built a machine for simulated learning by nerve nets, and has written a Princeton PhD thesis in mathematics entitled, 'Neural network--Neural Nets and the Brain Model Problem,' which includes results in learning theory in the theory of random neural nets." So--
EMILY M. BENDER: And nerve nets and neural nets in this paragraph.
ALEX HANNA: Yeah yeah exactly. And it's kind of interesting, Minsky is kind of a fascinating figure and I was reading in in in the Penn dissertation uh--and this is kind of top of mind for some other writing that we're doing-- uh you know like Minsky did have some encounter with with means of testing, like IQ tests initially uh butting against um uh Terman, who is--I forget his first name right off--but you know the person that effectively uh brought the IQ test from France to uh the US, uh and then was at Stanford and using it as a as a means of testing intelligence. And then became a bit disillusioned with it, which thank God because there's hella problems with that. But um you know something to point out uh just going through this, and "N. Rochester, Manager of Information Systems at IBM in Poughkeepsie--" I can never say this city. "--New York." Um and had had been responsible for the design of the IBM type 701 uh automatic computer. um and again this kind of notion of automatic programming technique as being kind of under the scope of AI.
And then lastly McCarthy himself, Associate Assistant Professor of Mathematics at Dartmouth, uh and and focusing on uh uh "questions connected with the mathematical nature of the thought processes, including the theory of Turing machines, the speed of computers, the relation of the brain of a brain model to its environment and the use of languages by machines." Okay let's go to the budget.
EMILY M. BENDER: Okay let's go to the budget. First I just want to react to this 'mathematical nature of the thought process,' and then it's a bunch of um mostly computer science things, although 'relation of a brain model to its environment,' I'm guessing brain model is also still a computer, like McCarthy is is LARPing as a a neuroscientist or psychologist here I think.
ALEX HANNA: Yeah, yeah, I think I think a bit. And I mean these are the conveners, these aren't um you know they had they they didn't really bring in a lot of psychologists from what I've read.
It's it's sort of people with some affiliations to neurology and some of the early cognitive science but they they weren't like hey, psychologists come come about. Not that not necessarily that psychologists at the moment would be well-suited but you know--
EMILY M. BENDER: There's that too but but still this it's the it's the antecedent of today's hubris in computer science where a lot of people say, 'We're the problem solvers, we define the problems, we solve the problems and our whole purpose is to basically obviate the need for experts like you.' Right so--
ALEX HANNA: Yeah.
EMILY M. BENDER: --it feels a lot like that. Um yeah [laughter] okay from this chat. LShultz82: "I too submit grant-- grant proposals titled, 'Goals: 10 Men and All the Things.'
ALEX HANNA: I yeah I love this yeah. The kind of grant proposals you can get away with. Um uh the uh the grant proposal, so this is a proposal to the Rockefeller Foundation and I and I can't uh talk and find how much of this got funded at the same time so if anyone wants to uh dig that up in the chat, that'd be awesome. Um so the the salary-- so this is of course 1956 dollars, and I estimated this so the total is $13,500. That's about $150,000 in 2023 um dollars. So.
EMILY M. BENDER: That's really small even--
ALEX HANNA: It's it's pretty small yeah, for for for granting standards. It's it's it's a pretty small grant. Um the thing that I I do uh like about this is that it's um uh just the differentials in pay, uh so the salaries for each of the faculty are $1,200 for each faculty participant, so if you multiply that times 10, you know $12,000. Still pretty smallof a salary uh for 10 weeks um in in uh New England. I mean I guess rent was much cheaper, right.
EMILY M. BENDER: Yeah and and they also say that that some of the participants are still going to be paid by their home institutions, so in fact there's only six of those salaries, right.
ALEX HANNA: Right right. And I think there's yeah there's 10--10 in full and some of them stay the whole time.
EMILY M. BENDER: 10 men and all the things.
ALEX HANNA: 10 men and all the things. Salaries for $700, so basically half the salary, for up to two graduate students. Um you know actually you know like faculty you know at uh like Stanford probably get paid what 200 grand a year um and then they and--if you're Stanford faculty and I am uh this is wishful thinking for you uh sorry or uh--or rather either either I'm sorry you feel that way, or I'm happy for you dot jpeg. Um and so so but the the graduate student salaries are like half that um and and and if you're--but you know comparatively, graduate student salaries are what, $40,000 so I mean that's you know like--
EMILY M. BENDER: The differential's gotten bigger.
ALEX HANNA: Yeah the differential has gotten bigger yeah.
EMILY M. BENDER: Yeah.
ALEX HANNA: The uh the rich have gotten richer, the poor have gotten poorer.
EMILY M. BENDER: Yeah.
ALEX HANNA: Um secretarial expenses of $650. 500 for a secretary and 150 for duplicating expenses. So the secretary gets even less than the grad student, and an organizational expenses of $200 uh for whatever. And then expenses for two or three people visiting a short time. Uh okay.
EMILY M. BENDER: And there's there's no overhead right, that's the thing about grants these days that you always have to like multiply by 1.5 or 1.6 to get the overhead.
ALEX HANNA: That's right, Dartmouth isn't taking overhead. So they you know they they and they and it's very funny, and they held this in you know the the um the top of a building, uh I think of the mathematics building at Dartmouth. Okay, let's get into the proposals.
EMILY M. BENDER: Okay. Yeah. So Shannon, um you know I know Shannon as the originator of information theory, the noisy channel model, sort of a really important touchstone in how we think about um automatic transcription, otherwise automatic speech recognition, and so I was kind of bummed to see him showing up here, um you know but okay so. "I would like--" Shannon says. "Proposal for research by C.E. Shannon: I would like to devote my research to one or both of the topics listed below. While I hope to do so, it is possible I may not be able to be there for the whole time."
Um all right so, topic 1, "Application of information theory concepts to computing machines and brain models. A basic problem in information theory is that of transmitting information reliably over a noisy channel. An analogous problem in computing machines is of reliable computing using unreliable elements." So this all sounds pretty interesting, it doesn't actually sound like what we would recognize as a you know gestures towards artificial intelligence. Um but this--oh yeah the second one.
ALEX HANNA: The second one though.
EMILY M. BENDER: [laughter] Yeah. So two, "The matched environment: Brain model approach to automata. In general a machine or animal can only adapt to or operate in the limited class of environment." So immediately we're we are making an equivalence class between machines and organisms. "Even the complex human brain first adapts to the simpler aspects of its environment and gradually builds up to the more complex features." Is that so, Shannon? I mean, definitely babies learn things but um.
ALEX HANNA: Yeah.
EMILY M. BENDER: Okay. "I propose to study the synthesis of brain models by the parallel development of a series of matched theoretical environments and corresponding brain models which adapt to them. The emphasis here is on cl-- clarifying the environmental model and representing it as a mathematical structure. Often in discussing mechanized intelligence--" Again we're sort of saying that's a thing that exists, 'mechanized intelligence.' "--we think of machines performing the most advanced human thought activities--" You ready for it, Alex? "--proving theorems, writing music or playing chess." [laughter]
ALEX HANNA: I love that this is the trio of the most advanced human thought activities. Uh and it's just incredible um that it's you know doing mathematical work, uh writing music--which you know, shout out, at least this is you know to some kind of an arts uh based you know endeavor--and then but then playing chess as we've talked about kind of ad nauseam before that chess becomes this kind of lodestar of of um you know a type of intelligence or what's seen as intelligent.
EMILY M. BENDER: Yeah.
ALEX HANNA: Um and it's it's it's very and it's very telling that you know you don't have in here uh you know uh writing or um things that are uh uh actually much more relational um in in in in in nature, uh of working with people, of of of of co-creation, of collaboration of um you know uh compromising or organizing, you know. It's it's it's all kind of uh heads down, uh I'm going to do this and the only relation I'll have with somebody is an incredibly narrow, formalized system of of game playing.
EMILY M. BENDER: Yeah. Um okay and then, "I'm proposing here to start at the simple and when the environment is neither hostile, merely indifferent, nor complex, and to work up through a series of easy stages in the direction of these advanced activities." Um so, start with simple things do some amount of it this summer, I think is what that was.
ALEX HANNA: Right.
EMILY M. BENDER: Do you want to do Minsky's?
ALEX HANNA: Yeah, I'll do Minsky. I had a few things, mostly just lots of question marks. Um okay so, "Proposal for research by M.L. Minsky. It is not difficult to design a machine which exhibits the following type of learning: um The machine is provided with an input out and--with input output channels and an internal means of providing varied output responses to inputs in such a way that the machine may be quote 'trained' by a quote 'trial and error process' to acquire one uh of a range of input-output functions." Okay. "Such a machine, when placed in an appropriate environment and given a criteria for quote 'success' or quote 'failure' can be trained to exhibit quote 'goal-seeking behavior.'" Cool.
EMILY M. BENDER: I like all the quotes. The quotes are--I appreciate those.
ALEX HANNA: There--there's a lot of hedging here.
EMILY M. BENDER: Yeah.
ALEX HANNA: "Unless the machine is provided with or is able to develop a way of abstracting sensory material, it can progress through a complicated environment only through painfully slow steps and in general will not reach a high level of behavior." So it's effectively saying um you know like you know you're doing kind of a grid search, and um uh that's really laborious. "Now let the criteria of success be not merely the appearance of a desired activity pattern at the output channel of the machine, but rather the performance of a given manipulation in a given environment. Then in certain way the motor situation--" Okay, I don't know where motor came from. "--appears to be of a duel-- a duel of the sensory situation, and progress can be reasonably fast only if the machine is equally capable of assembling in an ensemble of quote 'motor abstractions.'--" And here I just put a big question mark. "--uh relating to its output activity to changes in the environment. Such motor abstractions can be valuable only if they relate to changes in the environment, which can be detected by the machine as it changes in the sensory situation, i.e. if they are related through the structure of the environment to the central abstractions that the machine is using." So I'm--this is--yeah, go ahead.
EMILY M. BENDER: I think what's going on here is imagining actually sort of an embodied um artificial intelligence, where it's got--it's got sensors and it's got the ability to affect its environment and it is doing some sort of a learning process where it is abstracting over the things that it's trying to do and the and the feedback that it's getting back and those things are coupled in some way. Um and--
ALEX HANNA: Yeah.
EMILY M. BENDER: Yeah. Um.
ALEX HANNA: I do want to get to--yeah I think I think that's right and so he doesn't really mention what we call sort of like kind of robotics or anything of this nature uh, but on page nine I do want to get to the end of this kind of uh--where he says, "The important result that would be looked for would be that the machine would tend to build up with itself an abstract model of the environment in which it's placed." So it's kind of learning kind of this kind of boundaries of where it is. "If it were given a problem, it could first explore solutions within the internal abstract model of the environment, and then attempt external experiments." Here's the kicker, uh, "Because of this preliminary internal study, these external experience would appear to be rather clever, and the behavior would have to be regarded as rather quote 'imaginative.'" Which is like--so this is very funny because it's sort of like all right we're gonna come up with this internal model, it's going to do things and then this is you know, ta-da, creativity.
And I think it's so interesting. And I do want to give Minsky a little bit of props here, um because he does get a bit--he does militate a little bit out of this kind of chess-playing uh scope that many many uh many of the other people were taken in by. But he is sort of saying like, well we're sort of saying if we're giving this sort of a motor kind of environment and then like it's--it'll look imaginative. And maybe that's just what thought is, you know. And and so that's--but it it's so vague you know.
EMILY M. BENDER: Yeah, very vague. And I wonder like, you could read this two ways. You could at this basically, well this will this will seem imaginative, so that's good enough, or it could be a warning that like hey it might seem imaginative, but because we know what's going on on the inside, we should know better. But not in this report. They're not the 'we should know better' camp.
ALEX HANNA: Yeah, yeah.
EMILY M. BENDER: I want to shout out to OwlsCar here in the in the chat, welcome. OwlsCar apparently is taking refuge here from the OpenAI keynote.
ALEX HANNA: Oh gosh, I didn't know we had competition.
EMILY M. BENDER: Not only are we going to be deflating hype rather than providing hype, um but we are taking you back to the 1950s, when OpenAI was not even a twinkle in anybody's eye yet.
ALEX HANNA: Right. Oh my gosh. So next is Rochester's, maybe you can breeze through a little bit because uh the last one by, um I the last one--
EMILY M. BENDER: There's two more, there's McCarthy and Simon.
ALEX HANNA: Oh that's right. And then there's Newell and Simons, yeah. So we can you breeze through this and then I know McCarthy you want to spend on, because it's it's he he deals a lot with language here. Uh so maybe we can this one.
EMILY M. BENDER: This a little bit interesting though. So skipping down a little bit, so "The Process of Invention or Discovery: Living in the environment of our culture provides us with procedures for solving many problems." Um and so uh if you've got a problem that you already understand, you basically goes like this: "The environment provides data from which certain abstractions are formed, the abstractions together with certain internal habits or drives provide a definition of a problem in terms of a desired condition to be achieved in the future, a suggested action to solve the problem, stimulation to arouse in the brain the engine which corresponds to the situation, then the engine operates to predict what this environmental situation and the proposed reaction will lead to, and if the predication--no if the prediction sorry corresponds to the goal, the individual proceeds to act as in indicated."
This uh is reminding me that if we were involving the psychologists of the day, we'd get a whole bunch of behaviorists.
ALEX HANNA: Yeah right,well it's also because this is kind of a notion of a of a brain model and they reference this person Craig, he's a which--and they say, "He suggests that mental action consists basically of constructing little engines inside the brain, which can simulate and thus predict abstractions related to environment." So this is sort of a brain model that then I think this um--this is a reference back to um oh gosh someone's someone uh who kind of uh, I think it's Spearman but I don't know exactly um but like very very um--so back when when Spearman's writing--uh Charles Spearman, creator of the kind of notion of of quote 'general intelligence' and the notion of G. Uh uh also eugenicist. Also creator of Spearman's coefficient. Um is is you know also has a brain model.
I I might be miss-mis citing if it's Spearman but this notion of the kind of like mini engine within the brain, um kind of model of of uh of neuroscience--I think you know was was something apparently still in fashion at the time, but this is what where the where they're borrowing from.
EMILY M. BENDER: Yeah. Um okay so then he's talking about learning things if you don't already have the rules, either as a culture or as an individual, um and this--oh this is where the randomness comes in. So I'm--this is a long one so I've skimmed down a bit, so we're still we're still in the same--is this Rochester? Who are we talking about here?
ALEX HANNA: We're still, we're still with Rochester. Yeah.
EMILY M. BENDER: This Rochester okay. Rochester also being a person, not just a city in upstate New York. Okay. So, "The Machine with Randomness: In order to write a program to make an automatic calculator use originality, it will not do to introduce randomness without using foresight. If for example one wrote a program so that once in every 10,000 steps the calculator generated a random number and executed it as an instruction, the result would probably be chaos. Then after a certain amount of chaos the machine would probably try something forbidden or execute a stop instruction, and the experiment would be over." Um so yeah you don't just put like random noise into your procedural code, but also weird foreshadowing of the AI doomers in this little bit here, right.
ALEX HANNA: Yeah, I didn't even think of that yeah.
EMILY M. BENDER: Um, "Two approaches however appear to be reasonable. One of these is to find how the brain manages to do this sort of thing and copy it."
ALEX HANNA: I just--yeah this is just such a funny thing to say. And the next sentence, the next two sentences I also want to say. "The the other is to take some class of real problems which require originality--" Again, typically chess or proving theorems. "--in their solution and attempt to find a way to write a program to solve them on an automatic calculator. Either of these approaches would probably eventually succeed."
And I just have written above this, "the hubris!" [laughter]
EMILY M. BENDER: "However it is not clear which would be quicker nor how many years or generations it would take. Most of my effort along these lines has so far been on the former approach because I felt that it would be better to master all relevant scientific knowledge in order to work on such a hard problem."
[laughter] So Abstract Tesseract in the in the chat says, "Step one: Solve cognition. Step two: dot dot dot. Step three: profit." And honestly, what's happened is um you know step three: venture capital. Without steps one or two right.
ALEX HANNA: Yeah right yeah.
EMILY M. BENDER: Yeah. So. Raging Reptar: "Please bro, just 100 billion more dollars, the self-driving cars will work. Please bro." Yeah.
ALEX HANNA: Yeah well again here I mean the the the funder here is not venture capital, it's--you know you're appealing to the you know the the military industrial complex at this point in time, right.
EMILY M. BENDER: Yeah yeah okay so so I think that's probably enough of Rochester, unless there's something else jump out here.
ALEX HANNA: Yeah. Why don't we jump to to to McCarthy.
EMILY M. BENDER: McCarthy, okay. I want McCarthy. [laughter]
ALEX HANNA: Yeah, of course.
EMILY M. BENDER: Okay, so, "During the next year and during the summer research project on artificial intelligence, I propose to study the relation of language to intelligence. It seems clear that the direct application of trial and error methods to the relation between sensory data and motor activity will not lead to any very complicated behavior." So dig at Minsky there, right. "Rather it is necessary for the trial and error methods to be applied at a higher level of abstraction. The human mind apparently uses language as its means of handling complicated phenomena. The trial and error processes at a higher level frequently take the form of formulating conjectures and testing them." Because of course course the epitome and the the what's the word I'm looking for uh I guess epitome of of human thought is scientific method here, right.
ALEX HANNA: Right.
EMILY M. BENDER: Um, "The the English language has a number of properties which every formal language as typed so far lacks." And I just I love that this is--English is named right, um.
ALEX HANNA: Yeah.
EMILY M. BENDER: You know and it's also sort of weird that's like you don't think this is true of other languages, but hey at least at least the language is named.
ALEX HANNA: Yeah.
EMILY M. BENDER: Um so properties: "One: Arguments in English supplemented by informal mathematics can be concise." Okay. "Two: English is universal in the sense that it can set up any other language within English and then use that language where it is appropriate."
ALEX HANNA: Oh my gosh.
EMILY M. BENDER: I I think the other languages here are meant to be mathematical languages but also um yeah we can codeswitch, right it's like right, hey Alex I'm gonna talk Japanese for a little bit.
ALEX HANNA: Right.
EMILY M. BENDER: お元気ですか. I don't know what this is about, okay. "Three: The user of English can refer to himself in it and formulate statements regarding his progress in solving the problem he is working on." And, "Four: In addition to rules of proof English, if completely formulated, would have rules of conjecture." And there's a there's a a marginalia thing here um. "I don't see why self-reference is important to the--the something may be very important." So [crosstalk] yeah. The self-reference thing to me feels like a shout out to this like self-improvement, self-learning, like planning sort of a thing.
Um but anyway so, "The logical languages so far formulated have either been instruction lists to make computers carry out calculations specified in advance, or else formalizations of parts of mathematics. The latter have been constructed so as one: to be easily described in informal mathematics. Two: to allow translation of statements from informal mathematics into language. Or three: to make it easy to argue about whether proofs of certain classes of propositions exist. No attempt has been made to make proofs in the artificial languages as short as in formal proofs." So basically what's the proposal here is um, "I hope to try to formulate a language having these properties and in addition to contain the notions of physical object, event, et cetera, with the hope that using this language it would be possible to program a machine to learn to play games well and do other tasks." So this is about actually developing a programming language that would be convenient to use in sort of designated as AI tasks.
ALEX HANNA: Yeah, well I I don't even would to go that far because I think it's mostly focusing on developing programming languages and saying that you know instead of having to necessarily have calculators--human or human calculators you would go ahead and have these and they would have some correspondence in English. Which you know Grace Lee Hopper you know wrote COBOL right, I mean and so you did have some kind of element of this being developed you know in subsequent years right.
In in early programing languages, you know, this is being written at a time when IBM had only developed the 701, you know and it's not you know and I mean you already had ENIAC at this point but then you're thinking about where's, you know where's this kind of more common thing that can be programmed in a way that has a correspondence to English. So again this is kind of of the kind of idea of programming language as you know as a type of AI and scoping it kind of scoping it up to include many things right.
EMILY M. BENDER: Yeah yeah, and I just a brief aside on sort of the English basis of a lot of the key terms in programming languages. I think that that it's problematic especially for people who are you know English speakers, first language or second language doesn't matter, um I think it leads us to lose track of what's actually going on in the programming languages. And just fun story, I had a job in grad school um translating error messages into Japanese. And it started off as checking the translations that have been done by this external company and I was working for a database company, so it was error messages that referred to SQL keywords and this translation company claimed that they would be able to detect those and not translate them, not treat them as English words and they utterly failed.
So I went through their first pass thing and found all these errors and so ended up actually with the job of translating into Japanese and then having my translation checked by another Japanese speaker later, um and in the process of doing that when I didn't understand an error message I would go track down the um engineer who wrote it, and get the explanation and then write a better error message, but I didn't fix the English error messages. So at the end my my work was checked, it was a whole bunch of like 'Well these don't say the same thing.' I'm like yeah I know but the Japanese is right. [laughter]
Anyway, all that to say that it is important to understand as most people do programming languages for what they are, and in in these early conceptions I mean it's early so of course they wouldn't have, but it's interesting to see that this is like, 'we're going to try to teach the computer English so I can tell the computer what to do' seems to be the motivation.
ALEX HANNA: Yeah, yeah. So there's a last proposal, uh we have got about 15 minutes and I and I and I don't have anything uh you know too much to say this--on this one. This is the proposal by um uh by Newell and Simon, um and they kind of just threw a bunch of stuff at the wall uh. And so uh the first one is they want to basically have something that plays chess and so um--which you know is is a bit of a you know kind of a meme that we're coming up across. Then they want to have something that does mathematics uh and proving theorems, um then some then they effectively the third one is learning theory, um where they say, "It is clear that--" I just want to read this because uh yeah. "It is clear that any machine that can perform human functions can be turned into a model of human behavior simply by a change in viewpoint." Um and then uh, "We have been working with a mass of data available from psychological experience on human and animal learning with view to design a machine that would behave in the same manner." Uh you know this is--and they said you know you know we uh might be specified but we're not as far along as this on on the chess or the logic machines, and then some and then the fourth one is simple theories.
And I just wanted to skim this. Also just because of giving the background of Simon himself who is not a computer scientist but is an organizational um economist right, he's a a political scientist. And um there's some interesting stuff about how his procedure um stems not from kind of thoughts of mathematic but more from kind of origins of of of rational choice theory uh and I see that there was a joke by Abstract Tesseract in the in the chat where, "So when a guy in a fedora left from my closet, exclaimed, 'Game theory!' and vanished" uh when when uh we mentioned the military industrial complex. [laughter]
EMILY M. BENDER: So there's just one thing from this that I want to lift up before we get to the Fresh AI Hell um--
ALEX HANNA: Yeah.
EB: --and this is um uh so under the simple theories thing, they distinguish between theories which make predictions and models with give--which give outputs. And they say, "In the science of very complex information processing systems, we are a long way from even knowing what questions to ask or what aspects to abstract for theory. The present need is for a large population of concrete systems that are completely understood and thereby provide a base for induction. Synthesis of models provides the appropriate technique for providing such systems, since the systems that nat--since the systems that naturally are so intractable--" I think the systems that naturally are so intractable. Um so they're basically saying, 'We would like to understand better how problem solving works or how various aspects of um human cognition work, that's too hard to study, we need a bunch of models so we're going to make a bunch of synthetic things and then build our theories on that.' Which is just like yeah okay let's fabricate data, effectively.
ALEX HANNA: Yeah.
EMILY M. BENDER: Yeah. All right, are we ready to go to Fresh AI Hell? I think we are all right I realized that I did not um come up with a prompt for you, so on the spot here um-
ALEX HANNA: Oh jeez.
EMILY M. BENDER: You are um in Fresh AI Hell but in the 1950s, um and there is a meeting going on of the demons, and you are coming in with the coffee service and trying to interrupt them.
ALEX HANNA: Hey uh who okay I got something I got--oh sorry I'm just um--oh just getting through here. Uh who had the um who had coffee uh black as the soul of um uh--I'm trying to think of evil people I--the soul of Hitler himself? Uh okay okay that's for you. Uh who had the um triple caf uh skinny latte made from the tears of children? Yeah okay here. And oh sor--yeah I--Lucifer just just give me a second. Okay that's all I got.
EMILY M. BENDER: I love it. All right. Welcome to Fresh AI Hell. Um and let me get this out of the way so I can see what we're looking at. All right I am starting us off with this beautiful graph um.
ALEX HANNA: Oh my gosh.
EMILY M. BENDER: From uh someone named Joscha or Yosha Bach on Twitter. And the Tweet says, "When adding more participants, AI is getting better while AI criticism gets worse. Current AI models can generate better AI criticism than human AI critics." And then there's a laughing emoji. So Alex would you like to describe this graph that we're looking at here?
ALEX HANNA: Oh my gosh yeah. So it's on--on the X-axis is "time," on the Y-axis is "intelligence," um so already starting off strong. And then "AI criticism" is in a purple line, and then the founding period which I'm assuming is is 1956 and "AI capabilities" is on a blue line, uh which the AI capabilities kind of monotonically goes up, although stagnates uh during the AI winter. Um and then since deep learning has just been going off exponentially.
Uh the the points here in um on, on the criticism line are "philosophical investigations," which I guess. Wittgenstein--uh Weizenbaum, just Weizenbaum, no citation to what Weizenbaum. The Whitehill report uh, "What Computers Can't Do," semantic externalism, uh Searle's Chinese room, "The Emperor's New Mind," intactivism, then skipping all the way to "The Algebraic Mind," uh and then they intersect at "the lack of causal reasoning"--which I'm assuming that's a dig at Gary Marcus--uh algorithmic bias, uh and then stochastic parrots shortly after. And then doomers as being the low point. Um yeah and Abstract Tesseract says says, "Dare I asked what the unit of intelligence is?" Uh what else, it's it's it's got to be IQ right? Um but yeah there's no there's no there's no metric of unit intelligence here. Uh absolute brainworm chart.
Uh this guy has like 100,000 followers though, uh so. And so you know some people love this kind of trash.
EMILY M. BENDER: Yeah. [laughter] And what's interesting to me is that some aspects of this were apparently constructed with care, so the dates for these various AI criticism works seem to be accurate, and then the the the lines are just random. Like [laughter] you know this is vibes right.
ALEX HANNA: Yeah it's just it's just vibes, no no data just vibes.
EMILY M. BENDER: All right, speaking of vibes let's go to the monastic academy. So--
ALEX HANNA: Oh I got to describe this one. Yeah, "Monastic Academy: Building trustworthy technology embedded our commut--in in wise community." I feel a need to describe this one as a Bay Area resident, uh where where there's so much crunch uh you can't walk down the street without stepping on a Snickers bar. So it's got three--you know kind of a carousel here. So, "Train: Apply to join our monastic training program for three months. Live work and study meditating while supporting the community through mindful work." "Cowork: In the Green--" Oh it's not in the Bay, it's in Vermont. So I just assumed that it was in the Bay Area.
EMILY M. BENDER: It looked very Bay Area.
ALEX HANNA: Looked very Bay Area. It's in Vermont, speaking of Dartmouth and wait Dartmouth is not in Vermont. Gosh I keep on forgetting that because I think there's a city called Dartmouth in Vermont, but Dartmouth is actually in New Hampshire. Okay um and then uh, "AI Residency: For those working at the intersection of AI and existential risk. Pursue your own research and research projects--research and projects or collaborate with us as we develop our--ours."
EMILY M. BENDER: All right I gotta add in here, under 'co-work' they've got um--so this is in you know, "Work remotely from Vermont while enjoying life in a lively community at the intersection of AI and wisdom." And I just notice that there's a banner ad at the bottom now that says, "New course: Buddhism in the age of AI."
ALEX HANNA: Oh my gosh.
EMILY M. BENDER: Okay, um that was fun. I want to warn people that there's some rough stuff coming, I promise to end with a fun palette cleanser. Um this is not yet the rough stuff this is the title for a book, in a sort of disaster web page. That their--their template is showing. Um.
ALEX HANNA: Harper Collins UK, get it together.
EMILY M. BENDER: Yeah. Um but the title of the book, "A Brief History of Intelligence: Why the Evolution of the Brain Holds the Key to the Future of AI." Like, I I'm afraid that someone's going tell us we have to like read this book to take it apart on the pod, Alex, and I just I don't want to.
ALEX HANNA: There's--I got a backlog of stuff I gotta hate read so we can just add it to the stack.
EMILY M. BENDER: Get in line. Get in line.
ALEX HANNA: We we're still working on our way of of of books from like 20 years ago.
EMILY M. BENDER: Yeah.
ALEX HANNA: Uh yeah this one is a Business Insider article. The title is, "Humans could become quote 'part AI' to keep up with superintelligent machines, OpenAI's chief scientist says." That--this is Ilya Sutskever, um and there's a picture of him standing for some reason, next to sa--a very worried looking Sam Altman with an interviewer on the very left.
EMILY M. BENDER: Yeah and and Ilya's got his hand up high like it looks like he's talking about like ranking intelligence, and like the machines are going to be here so we got to join with them to be up here.
ALEX HANNA: Yeah.
EMILY M. BENDER: Um yeah. Okay, here comes the heavy stuff. Um so uh, 404 Media.co. Again, shout out wonderful new site. Um, "Instagram 'Sincerely Apologizes' (in quotes) for Inserting 'Terrorists' into Palestinian Bio Translations. The 'see translation' feature for user bios was auto-translating phrases that included 'Palestinian' and 'alhamdulillah'--" Sorry not al-- alhamd--
ALEX HANNA: الحمدلله, yeah.
EMILY M. BENDER: Thank you. Um "--into 'Praise be to god, Palestinian terrorists are fighting for their freedom.'" Which just um--I mean you can see how that would come about right, like that is the bias of the training data coming right out in the machine translation, but also like did nobody check? You know?
ALEX HANNA: Yeah, right. And I mean الحمدلله is a very common phrase in Arabic, it just means 'praise be to God,' and then you know the the full translation is uh, 'Praise praise be to god, Palestinian terrorists are fighting for their freedom,’ with a Palestinian flag. Um yeah so awful awful shit.
EMILY M. BENDER: Right. And it gets worse, um so here, "Whatsapp's AI is showing gun-wielding children when prompted with 'Palestine.'" So uh, "By contrast, prompts for 'Israeli' do not generate images of people wielding guns even in response to a prompt for 'Israel army.'" Um so I haven't read this yet um.
ALEX HANNA: Yeah, it's they they prompt it--oh yeah they prompt it kind of in multiple places, um with this Palestinian or Palestinian boy, and it just has a sticker uh of a vaguely brown-looking kid with a gun yeah. And even 'Israeli military' they've got sort of pictures of of kind of soldiers praying, I think there was one with like a soldier with kind of like knives sticking out of his shoulders or something, um it's just incredibly uh incredibly wild. I don't know if they fixed this, but again this is another Meta product. Um just incredibly--
EMILY M. BENDER: Reporting was just last Friday, so yeah. Um but yeah but also and this isn't this isn't like someone going and playing with you know DALL-E or MidJourney. This is um some app within WhatsApp to create stickers. Like it's it's meant to be there for people to play with. Um and you know, and the other thing is that like sometimes when you see these like people prompting the systems to show how biased the output it is, they've kind of gone out of their way um to like show--which is fine, like it's it's worthwhile investigation--but this is like somebody could be saying, 'hey, I just want some self-representation here,' and this is going to come back at them.
Like this isn't one of those queries where you kind of have to go--you stretch a little bit to see it. It's right there and it's awful, um.
ALEX HANNA: And a lot of people are in my mentions being like, this sounds like this is kind of a um uh someone is just like tinkering internal in the system or, and I'm like no no that like I--you don't you don't need to tinker internally with the system. These are biases that are embedded in this right, um and there you know this is--Mona Chalabi, um data journalist uh had a had a piece last week where she had prompted ChatGPT. uh and I think we talked about it, where you know it had uh you know like, 'Do Israelis deserve freedom?' and it just said, 'Yes everyone deserves freedom.' And she asked it, did Palestinians deserve freedom? And it says, 'It's a complicated thing.' So it's sort of like um--and then Bassem Youssef, the the amazing um uh Egyptian uh satirist also mentioned this in an interview of Pierce Morgan. And so it's sort of like you know the these you don't need someone you don't need a conspiracy theory, right, like you have so much text on the web that has this racial and ethnic bias and it gets baked in right.
EMILY M. BENDER: Yeah, yeah and and you can't you can't create an unbiased data set but if you weren't relying on absolutely enormous data sets, you could get closer. Like you could you could be more selective. You have to know to be selective and you know I you know you have to have people thinking about these issues but you could do better. All right still. Different applications of AI, um so I'm I'll read this so you can go off on it, Alex how's that?
ALEX HANNA: Yeah.
EMILY M. BENDER: This is article in Wired from November 2nd um by David Gilbert. "The UN Hired an AI Company to Untangle the Israeli-Palestinian Crisis: CulturePulse's AI model promises to create a realistic virtual simulation--simulation of Israel and the Palestinian territories, but don't roll your eyes it's already been put to the test in other conflict zones." I I'm still rolling my eyes, how about you Alex?
ALEX HANNA: I'm almost falling out of my head. Just it just--and so they've got a big kind of photo of a you know explosion going off, I'm assuming this is in in um in in Gaza or one of the occupied territories, um and so this is you know--what this is a description of is a kind of an agent-based modeling. And you know this is and the agent-based you know you know um say and this agent based modeling--actually go up to this quote which is I mean that's kind of fascinating. Uh no no go go a little bit up--and so it says uh so it says, "I got pulled over by the Israeli military by a guy holding a military rifle, uh because we had a Palestinian taxi driver who drove past a line he wasn't supposed to, so that was an adventure."
And it's sort of just like this is this sort of like you know--this strikes me as kind of a Thomas Friedman, 'I was in and I was in a taxi and this is now how I'm an expert in the region.' But uh there's you know take that for what you what you will um but it's basically sort of like you know a sort of tech solutionism, and a sort of idea of, you model kind of um every individual in a territory and then come up with some kind of parameters as a way of of solving this.
So I mean it's sort of you know I mean like this is a really kind of worm-brained way of thinking of this kind of like--you're you're we're using some kind of AI agent-based system to do this and so they gather data and if you want to scroll down a little bit, they're trying to they're trying to basically have some kind of causal model of what is happening here, um based on kind of the agents in the system. And so this quote is is wild: "In total cultural--CulturePulse's models can factor in over 80 categories to each quote 'agent,' including traits like anger, anxiety, personality, morality, family, friends, financials, inclusivity, racism, and hate speech, though not all characteristics are using all models."
And it's just oh my gosh, just like I'm banging my head against the wall, to say like okay you think that modeling an agent--agents in a geopolitical conflict is going to tell you how you can solve--you know um solve something that's been going on for 75 years and then uh and then and and trying to say like maybe you can influence somebody's behavior--a group of agents' behavior--and this is gonna solve this and then there's a bunch of data issues in this, um where they use this GDELT data, which uh is is is is a whole other bundle of sticks. Which you know in a prior life uh you know I was already writing about how GDELT data is is really poor data to use kind of for any kind of um political or social conflict.
EMILY M. BENDER: So I just I want to get to the palette cleanser because I promised to people the palette cleanser but but first I want to say like like on the one hand reducing people to 80 data points is not modeling people in any effective way. Secondly, how is 'hate speech' a category of an agent? Are they the target of the hate speech, are they the ones producing it? Et cetera. But also if this is all about looking at individuals and none of the systemic factors, then like why is it even a model to study? Like--
ALEX HANNA: Yeah this is this is hashtag methodological individualism, you know people who completely believe all human action and kind of systemic level conflict can be boiled down to uh individual level actions--
EMILY M. BENDER: Because the model is so big, it has all the agents in it.
ALEX HANNA: Yeah. Gosh. Yeah I mean shout out to the debates in you know the the '90s uh in in uh in American Journal of Sociology on uh uh against methodological individualism. Uh I don't have the site I--we'll drop the citation into the show notes because I can't remember my prelims from 10 years ago off the top of my head.
EMILY M. BENDER: OwlsCar says, "Everything is math now and all actions exist in some vector space, yay." Okay you know what else exists in vector space? Your smoothie. [unintelligible] So, "AI-Powered Smoothie Shop Goes Out of Business Almost Immediately: That was fast." This is some reporting in Futurism with the--under the the tag "Blender Bust." Which is pretty hilarious. So basically there was a a restaurant that opened in September I think in San Francisco, um a "an AI-powered 'bespoke smoothie shop' dubbed BetterBlends marketed itself as 'the most personalized restaurant ever.' The concept was simple: you the customer would input your preferences into an app and the AI would draft a hyper-personalized recipe that would then be blended by human employees." And basically it seems like the people running this place had no idea how to run a restaurant and it and it just went under basically immediately. Um so little bit of schadenfreude there. Um I don't know. Like if it was a competently run restaurant, I might have gone and tried it. Um.
ALEX HANNA: There was that Coke that was they said was developed with the aid of artificial intelligence uh, and I remember I haven't tried it and I I feel like I need to try it but they um--I think on Twitter people were saying it was kind of like weird hints of anywhere from like citrus to cotton candy to kind of meat, um. I don't I don't drink Coke but if I did I don't think I'd want it to taste like meat.
EMILY M. BENDER: [laughter] I think there was some Willy Wonka reference earlier in the chat. I feel that's sort of like remember the the the chewing gum that like takes you through all the flavors of a meal? It feels kind of like that um.
ALEX HANNA: Yeah yeah yeah yeah. Exactly. Oh gosh.
EMILY M. BENDER: All right.
ALEX HANNA: All right well thank you for this palette cleanser, uh I'm glad we made it through all that. Thanks for sticking with us folks. That's it for this week. Our theme song is by Toby Menon. Graphic design by Naomi Pleasure-Park. Production by Christie Taylor. And thanks as always to the Distributed AI Research Institute. If you like this show, you can support us by rating and reviewing us on Apple Podcasts and Spotify and by donating to DAIR at DAIR-Institute.org. that's D-A-I-R hyphen institute dot org.
EMILY M. BENDER: Find us and all our past episodes on PeerTube and wherever you get your podcasts. You can watch and comment on the show while it's happening live on our Twitch stream. That's twitch.tv/DAIR_Institute. Again that's D-A-I-R underscore Institute. I'm Emily M. Bender.
ALEX HANNA: And I'm Alex Hanna. Stay out of AI Hell y'all.