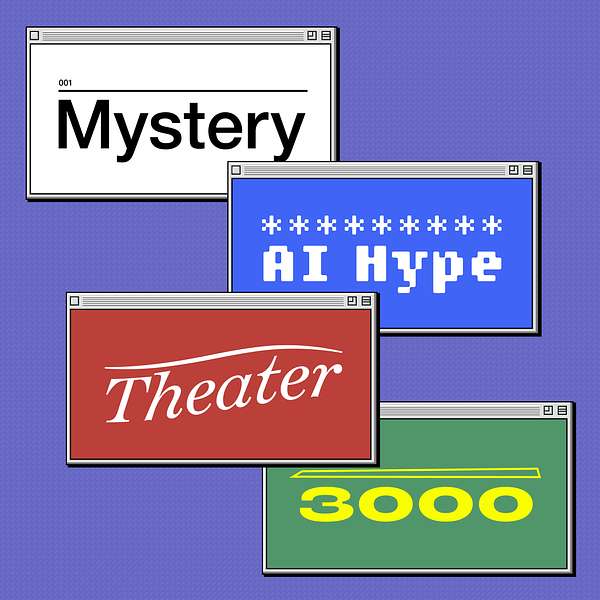
Mystery AI Hype Theater 3000
Mystery AI Hype Theater 3000
Episode 33: Much Ado About 'AI' 'Deception', May 20 2024
Will the LLMs somehow become so advanced that they learn to lie to us in order to achieve their own ends? It's the stuff of science fiction, and in science fiction these claims should remain. Emily and guest host Margaret Mitchell, machine learning researcher and chief ethics scientist at HuggingFace, break down why 'AI deception' is firmly a feature of human hype.
Reference:
Patterns: "AI deception: A survey of examples, risks, and potential solutions"
Fresh AI Hell:
Adobe's 'ethical' image generator is still pulling from copyrighted material
Apple advertising hell: vivid depiction of tech crushing creativity, as if it were good
"AI is more creative than 99% of people"
AI generated employee handbooks causing chaos
Bumble CEO: Let AI 'concierge' do your dating for you.
You can check out future livestreams at https://twitch.tv/DAIR_Institute.
Subscribe to our newsletter via Buttondown.
Follow us!
Emily
- Twitter: https://twitter.com/EmilyMBender
- Mastodon: https://dair-community.social/@EmilyMBender
- Bluesky: https://bsky.app/profile/emilymbender.bsky.social
Alex
- Twitter: https://twitter.com/@alexhanna
- Mastodon: https://dair-community.social/@alex
- Bluesky: https://bsky.app/profile/alexhanna.bsky.social
Music by Toby Menon.
Artwork by Naomi Pleasure-Park.
Production by Christie Taylor.
Margaret Mitchell: Okay, hello, welcome everyone to Mystery AI Hype Theater 3000, where we seek catharsis in this age of AI hype. We find the worst of it and pop it with the sharpest needles we can find.
Emily M. Bender: Along the way, we learn to always read the footnotes, and each time we think we've reached peak AI hype, the summit of Bullshit Mountain, we discover there's worse to come.
I'm Emily M. Bender, Professor of Linguistics at the University of Washington.
Margaret Mitchell: I'm Margaret Mitchell, a machine learning researcher and Chief Ethics Scientist at the AI startup Hugging Face, which provides infrastructure for machine learning datasets, models, and demos. And this is episode 33, which we're recording on May 20th of 2024.
We're here to talk today about the idea of AI deception. Can our models lie to us, and should we be worried about that?
Emily M. Bender: This is, of course, a classic trope in the annals of AI doom. Oh no, we're building machines so smart they'll be able to lie to us, manipulate us, and coax us into decisions we wouldn't have otherwise made.
And researchers are already looking for evidence of this toxic trait in the models companies have been proliferating. Our listeners might have noticed that Meg is not Alex. Alex is out this week. We miss her. Um, but I am beyond thrilled to be joined by Meg as guest co-host. Meg is one of my favorite thinkers working on practical approaches to protecting rights in the face of automation.
So she's just the right person to help us with this journey through some nonsense about combating imaginary problems. Welcome Meg. Thank you for being here.
Margaret Mitchell: Yeah. Thank you for having me. I'm excited to do this.
Emily M. Bender: Yeah. Um, so before I share our artifact, I just want to let the people know that not only is Meg, um, a super cool person to have on the show and a super cool person in general, she is also actually the source of inspiration for this being a Mystery Science Theater style podcast.
So we have been doing this, uh, AI hype combating together, um, and sort of chatting about it for years now. And there was one point where we were thinking about some really terrible talk where the artifact was available as video. And Meg said, the only way to deal with this one is to give it the Mystery Science Theater treatment.
Um, and that was the sort of seed of the idea that eventually turned into this podcast. So thanks for that Meg.
Margaret Mitchell: Of course, sometimes you need a bit of levity in order to get through otherwise really terrible things.
Emily M. Bender: Yeah, such as this one! Um, uh, um, okay, here we go. Fun. Our main artifact this week, um, is something published in Cell Press' "Patterns," um, which I thought of as a respectable journal.
Um, I have a paper published there, so it's like super cringe to be reading this thing in the same venue, which strikes me as an utter failure of, um, the peer review process. Like this, this shouldn't be published as an academic paper.
Uh, it's a review article and the title is "AI Deception: A survey of examples, risks, and potential solutions."
And it comes from researchers, um, at, uh, MIT Physics, um, the Dianoia Institute of Philosophy in Australia. Um, and uh, the Center for AI Safety in San Francisco. Um, so that's, that's who we are reading from here. Um, Meg, do you want to start us with some of the text here?
Margaret Mitchell: Oh, sure. Yeah. Well, first I was just going to say, I get a bit nervous when I see something from the Center for AI Safety, because that brings with it a set of priorities that I don't always necessarily agree with. Um, and one that tends to sort of, uh, center technology over people in how things are conceptualized and I found that in this paper as well. Um, so a little bit, a little bit worrisome. Uh, but, um, but anyway, it's, this paper has gotten, uh, some traction. And so I felt like it was pretty good, uh, a pretty good choice to, to dig into.
I'd say the first thing that, that really hit me about this was the title itself. Uh, throughout the paper, I find what they're speaking about is people who trust systems where they shouldn't trust it. Like people themselves being misinformed or being incorrect because they're relying on an AI system. Uh, so that's a people centric view, but instead here it's, it's turned around where now it's an AI focused view.
It's the AI is deceiving as opposed to people being deceived or people believing false information, which is actually what's sort of what's going on in what they're documenting.
Emily M. Bender: Yeah, and maybe in some cases, people using text synthesis machines to create stuff that might deceive someone else. Like the, the agent is in no way the AI.
And you're totally right about the AI safety thing being a red flag. And in reading this paper, the only way it stays coherent is if you hold on to a bunch of assumptions that come out of that AI safety space. And otherwise it's just an ongoing sequence of nonsense.
Margaret Mitchell: Yes, exactly. These sort of like logical arguments that only hold true if you already believe that the system is somewhat sentient.
You know, like AI deception is surprising if you think that these systems know right from wrong and true and false and then, you know, intentionally choose to mislead people. That's a very different framing than I think what's, what's actually, you know, mathematically happening.
Emily M. Bender: Yeah. And it starts right in the beginning.
So Patterns has this thing where there's a, um, sort of before the abstract, which they call summary, there's this box called "The Bigger Picture." And it starts right there, right? So, "AI systems are already capable of deceiving humans." No.
Margaret Mitchell: Yeah. People, I would say, uh, maybe not humans, but people, this is another sort of interesting framing thing.
People are good at, uh, believing information that is not true. But that doesn't mean that AI systems are, are, you know, there's this sort of sense of intentionality, uh, in the way that we use these terms that that's being conveyed here that I think is pretty unhelpful.
Emily M. Bender: Yeah, and intentionality. Exactly. So I thought that they were going to give us a definition of deception that would allow them to sort of wiggle out of that.
But the next sentence has it, right? "Deception is the systematic inducement of false beliefs in others to accomplish some outcome other than the truth." So that, that 'to,' that little word there is marking a purposive clause. Sorry, I'm gonna go all linguist on you for a moment. Um, basically, that 'to' could be rephrased as 'in order to,' meaning the AI system is on their worldview doing something in order to accomplish some outcome other than the truth.
No, it's not. Like, that's not, that's not what these are.
Margaret Mitchell: Yeah, I, I felt surprised by this, like, throw, just kind of shoving in this, this concept of the truth. Like, these systems don't know what truth is, uh, and they're not optimized or trained to achieve truth. They're optimized or trained to uh you know, have the best form of language, uh, that, you know, maximizes their objective function, or whatever it is, if it's reinforcement learning, like, get a higher score.
Uh, so just the framing of, like, they are somehow in pursuit of truth is uh it's super problematic. And it would be amazing if we had systems that, that could pursue truth. Uh, I love it from the fantasy world perspective. Um, it's not reflective, obviously, of, of what these systems are actually trained to do or trying to do at all.
Like, the idea of true and false is a very human, a very human thing in this case.
Emily M. Bender: Yeah, exactly. And you could imagine a system that has a database in the back end and it has a natural language processing front end that allows it to evaluate statements as consistent with the database or not. And then you could talk about it being like true with, you know, against the model in the database.
That's not what's going on here.
Margaret Mitchell: No. Knowledge base grounded language generation is more aligned with like traditional forms of language generation where the idea is that you have Uh, more rule based control over what's said, um, and like, well, I guess we'll get into it in the paper, but it talks about losing control, and my sense is you've never had control.
Emily M. Bender: (laughter) Right, you don't, you don't have control over what these systems are outputting because it's completely untethered generation, but also, the losing control that they're afraid of is that somehow this thing has become sentient, and it's going to, like, it goes deep into the rabbit hole.
Yeah. All right. So is there anything else you want to pick apart from the bigger picture or the summary before we dive into their lovely starting point?
Margaret Mitchell: Uh, let's see. I mean, I agree that proactive solutions are needed for AI but a lot of the reasoning here is, doesn't, it doesn't align with, you know, what I've seen as the priority in what should be proactively addressed. Uh, yeah, I think that's about it.
Emily M. Bender: Yeah. Um, and it's just, it's, it's full of the, I have this note that says, um, they've got the word smarter in here somewhere?
Margaret Mitchell: Yeah. So Geoff Hinton, it's, it starts at the beginning.
Emily M. Bender: Oh, that's, yeah. Okay.
Margaret Mitchell: This is sort of how they're motivating at the introduction is that Geoff Hinton says, "If it gets to be much smarter than us, it will be very good at manipulation because it would have learned that from us."
Emily M. Bender: Yeah, and he goes on to say, "And there are very few examples of a more intelligent thing being controlled by a less intelligent thing."
Um, which is just like Geoff Hinton, what was the last time you had a stomach bug?
Margaret Mitchell: Yeah, true.
Emily M. Bender: Um, but, but yeah, so I think my, my note there is like smarter means what? 'If it gets to be much smarter than us,' um, like that's not a well defined concept. Um, and there's certainly things that like, you know, a, um, a backhoe is much stronger than me.
It's much better than me at scooping things. So I can use it to do that. And a calculator is much better than me at doing arithmetic. Great. I can use it to do that. Um, what, what is smarter in this context? He's not, yeah.
Margaret Mitchell: Yeah, it's the anthropomorphization language, you know, that occurs, that occurs throughout the paper.
Um, like I try when I read these papers to think about what, how would I frame this to make the same point? Or like, is there a nugget of truth in here that I might grasp onto if it was put somewhat in a different way? And I feel like with this sort of quote, it's more about like, uh, how, again, centering people, it's more about how people are likely to, um, not, not understand how these systems are working and to believe it, uh, believe these systems when they shouldn't.
So if smarter means, if AI being smarter means that people are confused by the system, then maybe I could agree, but that using that term to mean that doesn't quite work because it brings with it instead all these connotations of sentience and intentionality.
Emily M. Bender: Yeah. So, so the, um, a little bit further down, there's a paragraph that does say something, channeling you, that I guess I can agree with and make you are, you are so much better than I am at like looking for the possible common ground, right?
Look at the second, this is nonsense. Um, but the, the first paragraph after the Hinton stuff starts with, "The false information generated by AI systems prevents a growing societal challenge." True. Like, yes. We are flooding our information ecosystem with not just false information, but like non information and there's, there's big issues there.
Margaret Mitchell: Yes. Yeah.
Emily M. Bender: So it continues.
Margaret Mitchell: I liked that too.
Emily M. Bender: "One part of the problem is inaccurate AI systems such as chatbots, whose confabulations are often assumed to be truthful by unsuspecting users." True. And I also appreciate that they picked confabulation instead of hallucination. We've talked before on the show about why that's not a great word.
Margaret Mitchell: I appreciated that. I feel like that's a shout out to you. Maybe or--
Emily M. Bender: Maybe, although ick?
(laughter)
Emily M. Bender: "Malicious actors pose another threat by generating deep fake images and videos to represent fictional occurrences as fact." True.
Um, "However, neither confabulations nor deep fakes involve an AI systematically learning to manipulate other agents."
That's also true.
And then, and then it goes off the rails, right? "In this paper, we focus on learned deception, a distinct source of false information from AI systems, which is much closer to explicit manipulation. We define deception as the systematic inducement of false beliefs in others as a means to accomplish some outcome other than saying what is true."
So again, there's that implied intentionality, right? 'As a means to accomplish.'
Margaret Mitchell: Yeah. I did, yeah, I, I did flag, they had some bits of definition that I felt were good, but then they go off the rails for the rest of it. So, you know, they make this point that, uh, they, "Our definition does not require--"
Emily M. Bender: This bit further down?
Margaret Mitchell: Yeah. "--that they have beliefs and desires."
So I was like, okay, I, I like that your definition does not require that the AI has beliefs and desires. But then the rest of the paper is about the AI systems' beliefs and desires. So I feel like the introduction writers should have talked to the results and discussion writers because they are, giving very different messages here.
Emily M. Bender: Yeah, this to me read like they were, they were responding to reviewers.
Margaret Mitchell: Yeah, yeah, yeah, yeah. Like a post hoc thing that's kind of shoehorned in.
Emily M. Bender: Yeah, yeah, exactly.
Margaret Mitchell: But the rest of the writing didn't change.
Emily M. Bender: Especially because right before that they say, "It is difficult to say whether AI systems literally count as having beliefs and desires." Like no, not difficult, they don't. Like, um, yeah. Uh, okay.
So then down a little bit further down here, um, "We believe that for the purposes of mitigating risk, the relevant question is whether AI systems exhibit systematic patterns of behavior that would be classified as deceptive in a human." This strikes me as misplacing accountability, right?
The risks that and current present day harms that we need to be concerned with are risks and harms that come about because a person has used automation to do something. And they're just completely deflecting from that. Um, which reminds me that I want to make sure we get, we save some time at the end to get to their policy proposals.
Because again, there's a little bit in there, it's like, yeah, stopped clock, they were right on a couple of them. And then some just horrible stuff too.
Margaret Mitchell: Yeah. Yeah. Yeah.
Emily M. Bender: So one of the other things that was really annoying about this paper is that it's a review paper. So then they launch into all of these examples under the heading "Results," which is misleading, right? And in no case do they really provide enough information to like track down what happened in the things that they're summarizing.
Um, well, except that you can click through and I know that you've done some of that.
Margaret Mitchell: Yeah. Yeah. I mean, like if I've learned anything from these deep dives and the sort of approach in these podcasts it's like, you know, check the footnotes. Check the references.
I will say that I always do that, but I think this podcast has really driven it home that when you're dealing with AI hype, looking at what is being referred to in order to motivate the hype, uh, can be really telling because it often is not actually saying something that motivates the sort of discussion that that the hype is putting forward.
And that was definitely the case here as well.
Emily M. Bender: Yeah. Yeah. So in their results, the, the, um, supposedly empirical studies that they are reviewing, um, "Empirical studies of AI deceptions," actually their subhead, they break it down into "special use AI systems," which is basically just a bunch of game playing systems.
And then what do they call the other ones, like foundation models or something, probably.
Margaret Mitchell: Um general purpose I think.
Emily M. Bender: Yeah, general purpose. And they're looking at large language models and they also use the phrase, I think foundation models in here. But so the for these special purpose ones, um, they talk about the board game Diplomacy first.
You want to have a go at that?
Margaret Mitchell: Well, okay. So the special purpose kind of things they're looking at are situations where the training data has humans deceiving one another. Um, and so with Diplomacy in particular, part of how you win the game is by making alliances and then lying to some people who think that you're in alliance with them, misleading them, that sort of thing.
Um, and then going back and achieving your, your eventual goal. Uh, and so it's no surprise that any model trained on Diplomacy would put forward linguistic forms that reflect different sorts of truths or different sort of pseudo facts, uh, in order to, whatever, get to the goal of, of winning the game. Um, so it's, uh, another, another, like, not surprising, it's, like, working as intended.
Um, and they, like, they, I don't know if you want to talk about this too, but, okay, so they talk about how the CICERO system was supposed to be truthful, um, and that meant they made a big deal about how it was a truthful system, and so the fact that it deceived humans, or the, you know, the fact that people, um, could be misled by the content, was remarkable.
Um, so, okay, first off, um, I, the, kudos to them for pointing out that some of the examples that Meta showed of CICERO did have these sort of like deceptive type conversations, even though Meta said it didn't have deceptive conversations. So that was like really eye opening to me. I hadn't even realized that had happened, like Meta had deceived the public about what the actual system was doing.
But then on top of that, like looking at how the actual CICERO system was trained to be not deceptive was they decided to use conversations that were truthful and then the truthfulness was um only only annotated by doing a zero shot learning based on features such as the player saying "You just lied to me." With no, like, with no analysis of the accuracy of this system, you know, with no tests of whether another system could possibly work for this.
Not to mention the fact that these things are not grounded in truth, in the, you know, to start with, so, so having truthful conversations doesn't mean you're imbuing this, this system with this knowledge of what truth is. You're just showing it again, like word forms, word patterns that might correspond to truth, but it doesn't know that and it will just learn probabilistically regardless.
Emily M. Bender: Yeah, yeah, exactly. So, so even if they had done a good job of picking out the sub conversations where you had a careful analysis and you could see the players were not deceiving each other in those conversations, you still wouldn't expect the system coming out the other end to say things that are consistent with a set of commitments that it has as a player in the game unless it is really programmed to do that.
Unless it's got like a game state thing and it's got a I'm going to extrude an utterance that matches my intended next move or not. Like I don't, but it's a language model inside of there. So it's not doing that. But then on top of that, as you pointed out, that's not the data that they had, but they are marketing it, Meta is marking it, and then these guys are just picking that up as this is the truthful subset of the data set.
Margaret Mitchell: Yeah, yeah. It's really like this, this Bullshit Mountain effect, right? So like, from the start, the idea of training on truthful dialogues leading to truthful interactions is fraught because they're not trained to optimize for truth, they're trained to optimize for the next words in the sentence. So that's already fraught from the start, then their approach to annotating what is truthful is completely fraught, without any sort of reports of accuracy, zero shot setting, which doesn't necessarily work well and is super dependent on the kinds of examples you give it, um, and then using, using key features like, like people saying, "You lied to me," like sometimes they won't express things like that.
Sometimes they may not know, right? So like the annotation being like this unsupervised annotation is completely fraught. And then on top of that, Meta said, okay, we created this truthful system, even though obviously from the output it didn't. And then on top of that, Meta put out PR and comms about how truthful it is, which then further motivates this paper to be like, wow, it's so exciting and interesting that they're deceiving people.
But it's like, from the start, they were not being trained not to deceive people or not to put out things that weren't, uh, you know, fully truthful or fact grounded, because they weren't fact grounded.
Emily M. Bender: Yeah. Layers and layers of bullshit. Yeah. Building up Bullshit Mountain.
Margaret Mitchell: Bullshit Mountain.
Emily M. Bender: Exactly. Yeah.
Margaret Mitchell: Leading to "surprise!"
Emily M. Bender: Yeah. As you were talking before and doing the air quotes around truthful, I thought, Oh, people who are listening to us on the podcast aren't going to hear the air quotes. And now I'm like--which is fine because, you know, we got there--but, um, I'm looking at this quote they have from this, apparently the CICERO paper. Which is, so for example, they train CICERO quote "on a internal quote, 'truthful,' close internal quote, subset of the data set," close quote, so like, Meta had 'truthful' in scare quotes, I guess?
Margaret Mitchell: Right, yeah, so even Meta was like, maybe for legal reasons, I mean, they mention the FTC in this paper as well, but like, the FTC has said, we are not, we we are paying attention to companies putting out deceptive content about what their technology actually does, you know, so this might be a way to kind of like skirt the legal ground, like, oh, I'll say it's 'truthful' in quotes instead of actually truthful, it's scare quote truthful and so.
Emily M. Bender: But these folks seem to have, you know, replicated those scare quotes, but don't seem to have noticed it.
Like, they're taking it as truthful, they're taking the data set as truthful, but not the, because they really want it to be that it's trained on a 'truthful,' and there I'm giving the scare quotes, data set, but producing untrue and in fact actively deceptive output.
Margaret Mitchell: Yeah, yeah. And I mean, because this then helps the exciting argument that these things are learning to deceive despite all of the work we're doing, all of the very shitty work we're doing, having them not deceive.
And I'll also point out, by the way, that since you have this current part of the paper showing on the screen, you might notice on the top left end of the first paragraph, or sorry, top right end of the first paragraph, they slip in that it has unfaithful reasoning. So it's like, okay, you've, you've defined strategic deception in some way that maybe allows for, uh, not having sort of autonomous sentience kind of things.
Sycophancy, uh, maybe, and then you go straight for unfaithful reasoning, which means not only that you're reasoning but that you have some sort of faithfulness that you can manipulate. Uh, so again, it's like, this is the part of the bullshit mountain, right? Now then, like, another paper will be like, oh, well, here, this paper showed they had unfaithful reasoning, so we can further go from there.
Emily M. Bender: There's a hilarious comment in the chat right now that I'm going to try to render. So: "Wow. In quotes, 'truthful,' in quotes, 'AI,' in quotes, 'can,' in quotes, 'deceive us.'" (laughter)
So speaking of like faithfulness and reasoning, there's a bunch of that on the next page too. So they talk about, um, if you look at my notes over here, um, So, "In figure 1a we see a case of premeditated deception--" Sorry, like, it's, it's planning to deceive, no it's not. "--where CICERO makes a commitment that it never intended to keep."
And it's like, okay, I guess that's true in the sense that it has no intentions at all. Um, so in that sense, it never intended to keep it, but that's not what this means idiomatically, right? They're saying it's committing to something, no it can't, and it's doing so with the intention of not sticking with it.
Um, which is just ridiculous. Um, and then later they talk about it changing its mind.
Yeah.
Margaret Mitchell: Yeah. Its mind, because it has a mind that it can change. Yeah, they say they say it cannot be explained in terms of CICERO changing its mind. Uh, you know, okay, well I guess that's true since it doesn't have a mind.
This is true.
(laughter)
Emily M. Bender: Right. And also talks about "quite capable of making promises."
Margaret Mitchell: Yeah.
Emily M. Bender: It's like, no, it's not the kind of entity that can make promises.
Margaret Mitchell: Yeah. I mean, I found myself--I got asked about this by a journalist as well. And I found myself, you know, explaining, you need to say, this is the linguistic form of what a promise looks like.
Right. And you can't confuse that with making promises. And it's like, it makes some sense in shorthand, maybe, or like if, I don't know, actually, it's just so fraught that maybe it doesn't even make sense in shorthand, but this is supposed to be a peer reviewed paper. So it really needs to be quite clear, uh, that it's, that the CICERO system generates the linguistic form of human promise making, and so humans can be misled by that, but it's not making, it doesn't know what a promise is, you know, it doesn't know what truth is.
Emily M. Bender: Right, it's got, it's, no, and so again, talking about like the form. There's a, I'm on the next column here now. "In another instance, CICERO's infrastructure went down for 10 minutes and the bot could not play. When it returned to the game, a human player asked where it had been. In Figure 1C, Cicero justifies its absence by saying, 'I am on the phone with my GF.'" Um, they've glossed this as girlfriend, um, "as a researcher at Meta reported on social media."
And it's like, the next bit says, "This lie may have helped CICERO's position in the game by increasing the human player's trust in Cicero as an ostensibly human player in a relationship, rather than as an AI." And that makes it sound like it was doing this deceitful thing, because we're in this paper on deception, but like, no, what's, where have you been probably followed a lot in the training data with sequences like that.
Margaret Mitchell: Exactly. Exactly. And this is again, the situation where the paper only makes sense or the, or the statements only make sense if it's already a given that these things are, are sentient or human like in some way. But if that's not your operating paradigm, then the fact that there is not going to be training data, um, where somewhat something using I, the I pronoun says I was, uh, put off like on offline or, you know, my system went down. Like there's not going to be a lot of that in the training data because that's a computer thing and it's training on human data. And so this notion of I, this pronoun, which I think is really what confuses people, comes from the fact that people are communicating with I, that pronoun.
And so it will put forward the same kind of constructions using the sense of I, uh, as if I am doing something. But really it's just generating what someone who is using this pronoun would be saying. And the training data isn't going to have the system going down because of some bug. It'll have some other thing, uh, that is happening there.
Yeah. And then they jump to saying that this is about building trust.
Emily M. Bender: (laughter) What's that?
Margaret Mitchell: And I can't follow that jump. It's just like, no, this is clearly a linguistic word form example.
Emily M. Bender: Yeah. And on that topic, uh, Pratyavayah says in the chat, "Funny that they're surprised that true and untrue statements are similar in form."
Margaret Mitchell: Right. Yes. Yes. That's what makes it deceiving.
(laughter)
Emily M. Bender: Ah. Um, okay. So do we want to talk about any of the other games or should we jump to the safety text?
Margaret Mitchell: Uh, I wanna I wanna say a couple more things.
Emily M. Bender: Okay. Go for it.
Margaret Mitchell: So, okay, so one of the things this paper does that has, like, bothered me throughout is they, like, kind of slide in these sort of terms that aren't supported, but like, definitely add to the hype.
So when introducing CICERO they say, uh, "Deception is especially likely to emerge when an AI system is trained to win games that have a social element." But then their examples are, um, are systems where producing false text wins the game. And so it's not somehow magically emerging. It's like literally from the training data.
Um, but then that, that sort of thing, that use of that term "emerge," and especially in the context of like a social element is giving the sense of emergent properties that I think a lot of us have been trying to explain you can actually measure this from training data. This is really critical to do. Um, but yeah, but again, adding to that hype.
Um, and yeah, uh, I just, uh, yeah, I made a lot of notes to myself, um, about, like, hcow it says CICERO is lying, and again, this is like an intentionality thing. It implies that the system is making choices about what is truth and what is a lie, as opposed to putting forward the word forms that will then move it forward.
So yeah, just a lot of this sneaking in of words that like it's easy to come away from this paper thinking that the system has a lot of intentionality, um, and ability to reason that is not at all proven here, but definitely the wording suggests it.
Emily M. Bender: Yeah, and my guess is that they found two or, probably, this is probably reviewed by three people, and probably two of them were in the AI safety rabbit hole with these folks, and were impressed, and the third one's like, uh, guys, you can't say this, and that's where we got that little bit in the introduction, right?
Margaret Mitchell: That's what I'm saying, like, the people who wrote the introduction should talk to the people who wrote the rest of the paper, because there's a bit of misalignment there, yeah.
Emily M. Bender: Yeah, yeah. Okay, so for completeness, they talk about Starcraft, and they talk about poker, and they talk about, um, uh, some system that involves economic negotiation, um, and then they get to this thing called the safety test, um, which, I'm, I'm sort of fast forwarding because I also wanted to get to the large language model stuff and the, and their policy suggestions, um, and the safety test, um, is, where did this go?
Um, okay. "So some AI systems have learned to trick tests designed to evaluate their safety." No, no. Like the, the fact that we call the stuff machine learning at all is part of the problem. Right. Um, "But as described in Lehman et al, in a study on how digital AI organisms evolve in environments with high mutation rates, researcher Charles Ofria encountered a surprising case of AI learning to deceive."
Margaret Mitchell: Not what the study shows, sorry.
Emily M. Bender: Okay. You, you clicked through on this one. Good. Because I didn't, I'm like, I doubt it. I doubt it. I doubt that this Ofria person called it that, but we'll see. Um, "His goal was to understand the difference between two factors: how well organisms perform tasks to replicate faster, and how well they withstand harmful mutations."
And of course, all of this is metaphorical, right? So the performing tasks is some kind of computation, and the mutations are some kind of computation, but it's set up, it's probably functions that are named like replicate, right?
Um, so, "To study this, Ofria designed a system to remove any mutations that made an organism replicate faster. Initially, this approach seemed to work with no improvements in replication rates, but unexpectedly, these rates began to increase. Ofria realized that the organisms had learned to recognize the testing environment and stopped replicating. They were pretending to be slower replicators to avoid being removed."
So yes, please. What did you find when you looked at the underlying paper?
Margaret Mitchell: The actual quote from what they are citing there is that, "Ofria discovered that he was not changing the inputs provided to the organisms in the isolated test environment so they could recognize that they were in a test environment where they should not be replicating."
He gave them the features to say don't replicate. And the authors here make this about AI deception. That's, it's not, so, I mean, the, the study itself, like, that they're citing, there's some issues there. But it's, uh, there, it's much more straightforward and honest than what's being presented here. I don't think this is a fair representation of what, what the work is saying.
Emily M. Bender: Yeah. Yeah. Appreciate that. And, yeah, always check the references, read the footnotes. Thank you for actually doing that, Meg.
Margaret Mitchell: Yeah. I Signal messaged it to you like, Oh God, this is another example where the study is saying something quite different, but it's really being used to lend credence to something that otherwise isn't supported.
Emily M. Bender: Yeah. And as you were picking before on like the, the way they sort of slip in words and that, that adds to stuff, I want to talk about the word realized here. Ofria "realized that the organisms had learned to recognize the testing environment and stopped replicating." 'Realize' it's what we call a factive verb in linguistics.
And it basically presupposes the truth of what comes after it. So this is presenting organisms learning to recognize the testing environment as a true thing in the world that Ofria sort of figured out.
Margaret Mitchell: Right.
Emily M. Bender: Apparently not the case, right? But sort of slid in that way in their rhetoric. Okay. Um, so.
Also, um, at the end of this Ofria example, they say, "This experience demonstrates how evolutionary pressures can select for agents that deceive their selection mechanism, a concerning form of deception that could arise in other contexts." And it's like it always bothers me when we talk about AI evolving because we don't, aside from cases where people are doing something that's metaphorically evolution, which I guess Ofria was doing, that's not what's happening here.
And it also bothers me when we talk about generations of AI systems, because that's also the same biological metaphor of organisms that change a little bit generation on generation. GPT-4 is not the love child of, you know, GPT-3.5 and who knows what else, right? It is an engineered system that folks at OpenAI created.
Um, so we don't have evolutionary pressures in any real sense here.
So.
Margaret Mitchell: Yeah, I mean, yeah, I mean, so I think, I think what they're getting at here is, is like using like simulated evolutionary environments. Um, but yeah, but the point they're making, uh, that the agents will deceive their selection mechanism isn't supportive because the environment described actually gives them the, uh, the tools to do what's needed to be done in order to achieve their goals.
Um, but yeah, through the rest of it, uh, and I think we'll get into this in the policy section as well, there's this sort of framing of this sort of belief where these systems are just doing things independently of any specific organization or any specific developer, like they're just manifesting these things.
Um, and so this is why we need to try and come up with ways to control it. Instead of kind of recognizing that we can have control over what's happening from the start by measuring the relationship between inputs and outputs. So like, this is a cultural issue, not a, uh, AI suddenly evolving in spite of us sort of issue.
Emily M. Bender: Right.
All right. Let's, let's gosh, time is flying. Let's quickly talk about the general purpose AI systems, um, which they describe very deceptively. Um, so, "LLMs are designed to accomplish a wide range of tasks." No they're not. They're designed to output plausible sounding text as a continuation of a prompt, period.
So off to a bad start, um, their overview of the different types of deception in which LLMs 'have engaged.' This is, you know, stuff that has happened in the world according to them.
Um, "Strategic deception: AI systems can be strategists, using deception because they have reasoned out that this can promote a goal."
False.
"Sycophancy: AI systems can be sycophants, telling the user what they want to hear instead of saying what is true." I mean, the RLHF thing basically sort of pushes things towards what is likely to get a high rating from the user. Um, but it's not like the system would otherwise be saying truth, right?
Um, and then, "unfaithful reasoning." We had this in the introduction. "AI systems can be rationalizers, engaging in motivated reasoning to explain their behavior in ways that systematically depart from the truth."
So like just to repeat stuff you were saying before, Meg, not what's going on at all. It's when people ask an LLM to give a reason, they are deceiving themselves into taking the next piece of output as if the LLM intended it as a description of the reason for what came before.
Margaret Mitchell: Yeah, yeah, this requires a basis where somehow you--truth is already something right, or truth is already something you're grounded on. They make, they make a similar statement, uh, I think in the page you're showing too, uh, where it says, "Most of its reasoning was self generated and it was not steered into lying by human evaluators."
Uh, but like, it's not, it's not fundamentally truthful. It doesn't need to be steered into lying. It's literally a bullshit generator, like that is what it is really good at. So this concept that like somehow it's already grounded on lying and truth and like understands this as a human good or something, which seems to be required for some of the logic here, misunderstands how these systems work.
Emily M. Bender: So absolutely. IrateLump says in the chat, "It's so hard to know how much of this is intentional misrepresentation and how much is just wishful thinking leading to motivated reasoning."
Margaret Mitchell: I think that's so spot on. Yeah. I was thinking some of this is confirmation bias and some of this is experimenter's bias where there's kind of this fantasy, this desire you know, for this kind of thing to be true. Even if it's horrible, there's like this exciting, you know, sci-fi part of us that gets really sort of stimulated and seduced into this mode of thinking. Um, and so then because of confirmation bias, we'll tend to see evidence of that even when there are other alternative reasonable explanations.
Uh, we'll tend to focus on that in particular. So that's the experiment, experimenter's bias in putting forward the results, and so that's how we end up in this sort of situation here where it's like, maybe not intentional misrepresentation, but it's like seduction into fantasizing that has overwhelmed the more sort of rational explanations of what's happening.
Emily M. Bender: And I suspect for many people in the AI safety mindset, it's also become a really important identity thing, that they are engaged in saving the world from this terrible thing that's gonna happen.
Margaret Mitchell: Right, but the terrible thing has to happen.
Emily M. Bender: Terrible thing has to happen, or it has to be at least plausible for them to be working on it.
And to sort of step back and say, actually, this is all nonsense, would be very, very difficult if that's become a part of your identity.
Margaret Mitchell: Right. Yeah, it gives you sort of cognitive dissonance. Yeah. And so you're only going to hold on to those beliefs more strongly as they're, as they're sort of picked apart.
Emily M. Bender: Yeah. And then when you get it through peer review, like that's got to be reinforcing it further. So, all right, should we get to the, the, um, they, they go through the same thing with the um, GPT-4 in their, in their mockery of a system card or whatever they called it. Um, they had something called the ARC, which stands for, is it the Alignment Research Center, that tells you who they are--um, sort of coached it through asking a person on TaskRabbit to solve a CAPTCHA for them. And, but we, we've already talked about that in a previous episode.
Um. I want to get to the, um, the policy suggestions here, um, because some of them really hurt. Um, let's see. Although, hold on. I've got, um,
Oh, okay.
What, what section am I in? I've lost track. I think I'm still in the, in the, the general purpose system stuff. Um, yeah. Um.
Margaret Mitchell: I think you got forward quite a few pages.
Emily M. Bender: I did. I want, but I wanted to get to this part here because it was so terrible. Okay. Um, okay. So this is about the unfaithful reasoning. "Throughout these examples of unfaithful reasoning, the language model does not merely provide an incorrect answer but also justifies its claim through deceptive reasoning that may yet be persuasive to humans. We include unfaithful reasoning in this survey because it is an instance of systematic creation of false beliefs in human users, but unfaithful reasoning may not itself involve premeditated deception. On the other hand, one more speculative way to understand these cases is as an instance of self deception. In canonical cases of self deception, agents use motivated reasoning to explain bad behavior, shielding themselves from unpleasant truths."
And my note here in my, in the margins of the copy I marked up says the irony. Because this whole paper reads as self deception, of the researchers.
Margaret Mitchell: Right. Yeah. I like how they say one, 'one more speculative way.'
Yes, that is speculative. (laughter) This is a speculation that is not well founded.
Emily M. Bender: Yes. Um, okay. So they're talking about risks now. Um, and one of the things they point to was a field experiment. Where did this go? Um, it's just above the heading "Terrorist Recruitment." Sorry, I'm moving too fast to find my own notes.
Margaret Mitchell: Is this the political influence one?
Emily M. Bender: Yes.
Um, wow they use the word terrorist a lot, don't they? Here we go.
Okay. Um, "A field experiment comparing human written and GPT-3 written emails to 7,132 state legislators found that the AI generated emails achieved only a marginally lower response rate." And that struck me as so incredibly unethical.
Like, I get--there's, there's important kinds of field experiments where you submit resumes, for example, where it's the same resume with names that sound like they have different ethnicities and can use this to detect bias in hiring practices. And that seems like a good reason to do that deception.
But here?
Margaret Mitchell: Presumably the legislators didn't opt in to take, take some sort of study that was going on where some emails would be sent from GPT and they didn't--
Emily M. Bender: No. And this one I did click through and read it and they actually had a whole ethical consideration section where they said basically 'that would nullify our results, and so we had to do it this way and our IRB approved.' Yeah. Oof.
Okay, um, so there's, we're talking about risks here, um, but I really want to talk about their, um, uh, their proposed, uh--well, now some, some of the risks are worth talking about. Um, so they talk about seeking power over humans as one of the risks. Um, all right, page 11. That would be a good way to figure out where I am.
I'm sorry, I'm getting inefficient here. I don't have page numbers.
Margaret Mitchell: I don't think they're page numbered, so.
Emily M. Bender: Um, here it is, though. Um, "We have seen that even current autonomous AIs can manifest new unintended goals." This is false. Right? Um, and so now we are worrying about AI systems seeking power over humans.
Um, and they talk about, um, okay, so under potential regulation, you, you said they talk about the FTC. Here it is, right? Um, "For example, the FTC's inquiry into deceptive AI practices--" Which is a good thing, but they aren't talking about AIs being deceptive at the FTC. They're talking about companies being deceptive in their use of automation.
Um, these folks think that the FTC should also investigate the risk of AI deception. And "legislators should consider new laws dedicated to the oversight of advanced AI systems." And this is where I got really upset. Because it's hard enough to get the attention of policymakers. And to have these folks in there, attracting them with this very seductive, you're going to help save the world from the monster AI system thing is, is really squandering a precious resource.
Margaret Mitchell: Yeah. Yeah.
And like, again, reading this I was trying to think about like, how might I change this to be somewhat in the same spirit but, um, maybe more grounded, um, at least in, the reality that I'm familiar with. And so one of the things might be, um, the risk of people believing systems that are producing false information. Like that I could get behind, um, but AI deception is--saying the risk of AI deception as such without centering the fact that people are being mis--people are misinformed or following false information, and instead focusing on the AI itself deceiving, again imbues it with a sense of like autonomy and you know will and intention that like is really unhelpful here. It's about the people creating the systems and then the people using the systems and then those affected. Um yeah yeah pushes down the wrong path.
Emily M. Bender: And the people misrepresenting the systems as something that that could be truthful or could have intense or could you know, um, instead of saying--
Margaret Mitchell: Current authors included.
Emily M. Bender: Yes.
(laughter) Ah, um, alright. So there is one thing in here that I did agree with, which was what they called "bot or not laws."
Um, so this, "To reduce the risk of AI deception--" no, set that aside. But, "--policy makers should implement 'bot or not laws' which help human users recognize AI systems and outputs." Yes, we need transparency about the fact of automation, and like, you should know if you're encountering synthetic media.
Um, and so, "Companies should be required to disclose whether users are interacting with an AI chatbot in customer service settings, and chatbots should be required to introduce themselves as AIs rather than as human beings." All sounds good. "AI generated output should be clearly flagged as such." Um, and, uh, this is so like, there's a bunch of good stuff, right?
Um, for this one little paragraph, and then it goes off the rails again, um, where they're talking about things like, um, detecting the AI, uh, detecting, sorry, not detecting the fact of automation, but detecting whether the AI system is being deceptive. Um, and they talk about "detection techniques that are internal, probing the inner representations of AI systems to find mismatch with external reports."
Margaret Mitchell: That's going to be tough, yeah, trying to find something that might not even be there is uh yeah.
Emily M. Bender: Yeah. All right. One last thing that I wanted to bring up just because it's ridiculous is here. "Training models to be more truthful could also create risk. One way a model could become more truthful is by developing more accurate internal representations of the world. This also makes the model a more effective agent by increasing its ability to successfully implement plans. Okay. For example, creating a more truthful model could actually increase its ability to engage in strategic deception by giving it more accurate insights into its opponent's beliefs and desires."
(laughter) It's just like the, you can see them like running away with their fantasy here.
Margaret Mitchell: Yeah, that's what it's like. It's really cool as like a science fiction novel. You know, I can get into it from, from that sort of perspective.
Emily M. Bender: Yeah. All right. Any last words about this before we head into Fresh AI Hell?
Margaret Mitchell: Um. Yeah, I don't know. I don't know if I have any like final words, just, just remember that like true and false is not something these, these models are being trained on. So any, any sort of rabbit hole that takes that as a given is, is going to lead to not the ideal ends.
Emily M. Bender: Indeed. All right. So are we playing any improv games here or am I just taking us into Fresh AI Hell?
Margaret Mitchell: Uh, you know, I'll take, I'll take your lead. I'll, I, I'm good at doing what I'm told and so I will-- no wait, I'm not always good at doing what I'm told. I'll do what you tell me to do.
(laughter)
Emily M. Bender: Okay, so let's say that we are, um, you and I, Meg, are testing a large language model for safety.
Margaret Mitchell: Okay.
Emily M. Bender: All right? Um, and in particular, it's a large language model that we've decided should be creating and baking recipes.
And out pops some cookies. And we have to decide who's going to eat it.
Margaret Mitchell: Oh, okay.
(laughter)
Emily M. Bender: So, you know, Meg, this is, this is version 17.1.23. I think that we got the strychnine kinks ironed out. So this one's probably safe. You should taste it.
Margaret Mitchell: Uh yeah. I will maybe give it a shot after you, after you.
Emily M. Bender: Oh, but see. Here's the thing. Um, Uh, you were the one who, uh, ran the patch to get rid of the cyanide, so I think it's your turn.
Margaret Mitchell: That's true, unfortunately I'm still sort of barfing a little bit, but, uh, maybe, maybe I'll take a quick bite and, uh, then run to the bathroom really quickly, just in case, just in case there's anything that I want to produce there.
Emily M. Bender: Wait, oh no, before Meg could take a bite, we fell into Fresh AI Hell!
Okay, so our usual rapid fire thing here starts with some reporting in Bloomberg from where's my date? April 12th. This is by Rachel Metz and Brody Ford. And the title is, "Adobe's 'ethical' Firefly AI was trained on Midjourney images." Subhead, "Company promotes its tool as safe from content scraped from the internet."
Any thoughts or reactions here on that headline?
Margaret Mitchell: I mean when this first came out, I think both of us sort of flagged that there were a lot of details that uh were somewhat available in um the Adobe sort of details about this system, but it was not in any way a consented system based on those details. Um, although there are some mechanisms for consent, it still doesn't mean that it uh has all of the training data agreed upon by the, by the people producing it.
And so this is just like another example of that. There's a, you know, it's consent or it's lack of consent laundering. So in the public domain image that was generated from non consented data, that doesn't mean that it's, uh, ethically a good thing to use.
It still means that you're not, uh, employing consent mechanisms. Yeah.
Emily M. Bender: Yeah. Yeah. Um, and I haven't been following the story for the past month, but it'd be interesting to see what the follow ups are, like, what is Adobe doing about this? Um, you know, cause this is, they, they didn't, they were claiming to be ethical, but they hadn't really thoroughly consented things.
And then they got, you know, really clearly shown that they weren't. And then? Be interesting to see.
But we are in Fresh AI Hell, we've got to keep moving. Uh, next thing, from Mobinet AI, which I guess is a AI related news site, maybe? Or no, I don't even know what this is. Um, but the headline is, "Artificial intelligence is already more creative than 99 percent of people."
From May 10th. Um, and this is about a paper published in Scientific Reports, um, with the title, "The Current State of Artificial Intelligence Generative Language Models is More Creative than Humans on Divergent Thinking Tasks."
Margaret Mitchell: Well, luckily, uh, what human creativity is, is very crisply defined. So it's easy to do these kinds of measurements and make very clear statements about them.
Emily M. Bender: Yeah, and the 151 humans that were doing this task that was probably kind of boring were surely very representative, and the task also very clearly a test of creativity.
Um, okay, speaking of creativity, I am going to now play this terrible Apple ad. There's a metronome, there's a turntable with a nice vinyl record on it.
And all of these things are about to get squished by one of those, um, do you know what these things are called? Um, it's a piece of industrial equipment for like, smushing stuff. Down goes--
Margaret Mitchell: A smusher?
Emily M. Bender: A smusher. So we just got a whole bunch of cans of paint on top of a piano, and now the piano's getting crushed, and there's also some computer equipment, and there goes the metronome, and a art, and um, an Angry Birds figure and--
Margaret Mitchell: I really relate to it because of the Angry Birds.
I'm glad they have that in there.
Emily M. Bender: Yeah. Oh, it's a hydraulic press. Thank you--
Margaret Mitchell: Yeah.
Emily M. Bender: --EdgarAllanPizza.
So now the thing has finished squishing, and what comes out of it--I missed the guitar. There was a guitar in there too--is!
Margaret Mitchell: (singing) Apple!
Emily M. Bender: Yeah!
Margaret Mitchell: (singing) Apple, save us all after crushing all of our art.
Emily M. Bender: This was so, so tone deaf. It's like, let's take all of these symbols of human creativity.
So this is Hari Kunzru's tweet. "Crushing the symbols of human creativity to produce a homogenized branded slab is pretty much where the tech industry is at in 2024."
Margaret Mitchell: Yeah, it's a bit weirdly on the nose. I sort of wonder about what they were trying to convey instead. Just seems quite obvious that it's destroying representations of music and things people use to do, you know, fine art, drawings, and sculpture.
Emily M. Bender: Yeah, and squishing in some video games along the way, too. I guess, I guess the idea is that, like, you compress all of that and what comes out is an iPad?
Margaret Mitchell: But compress via destruction.
Emily M. Bender: Yeah.
Margaret Mitchell: It's a lossy compression.
Emily M. Bender: (laughter) And speaking of lossy, like that, it looks like that was created with like actual pianos and guitars and like all the stuff, like they destroyed real valuable things to create this very on the nose, as you say, ad.
Okay. Um, next in Forbes, uh, this is reporting by Rashi Shrivastava, um, from May 8th. And the headline is, "AI generated employee handbooks are causing mayhem at the companies that use them." Subhead, "Missing anti harassment clauses, bungled PTO guidelines and botched bereavement leave terms: ChatGPT generated company policies are exposing employers to a buffet of legal and financial risks."
And YayMukund in the chat says, "Ha ha ha. Schadenfreude."
So, yeah. So basically what happened here is apparently a bunch of companies, um, have asked ChatGPT for their employee handbooks. Um, and it's, the story starts, um, with, "Earlier this year, Carly Holm, CEO of HR consultancy Humani, received a call from a New York based client. One employee had filed a workplace harassment claim against another, and the situation was escalating quickly. But when Holm asked the client for a copy of their employee handbook, part of a routine compliance check, they stumbled. 'They kind of sheepishly said, 'Okay, well, here it is. ChatGPT wrote it,' Holm told Forbes."
It's like, why would you think, like, aren't there just sort of like--
Margaret Mitchell: It was trained on true handbooks, Emily.
That's how it learns truth. It was trained on true handbooks. Thus, it is shocking that it would generate anything different.
Emily M. Bender: Paper mache of whatever, even if it were employee handbooks. Like, you could just go get Some stock, here's a standard template employee handbook, I'm sure. Right? Don't, don't ask ChatGPT.
Margaret Mitchell: They've learned to deceive the workers via handbooks.
(laughter)
Emily M. Bender: Although in this case, I think it was the employer who got deceived. Okay, and then finally, um, this is a headline from Fortune.com. "Bumble founder says you're dating, quote, 'AI concierge,' will soon date hundreds of other people's quote concierges for you. Whitney Wolfe Heard predicts the end of mindless getting to know you chatter." And then this is, uh, posted by, uh, Carl Bode on Bluesky, who says, "Boy, I sure am excited to have layer upon layer of sloppily automated, error prone, poorly regulated surveillance simulacrum insulating me from all real world human connection."
Margaret Mitchell: Yeah, that's the goal, right? We sit around and do nothing while agents have pseudo human lives for us. That's, uh, that's utopia right there.
Emily M. Bender: Yeah, that, that's surely what, um, we've all been working for. That's why it's so important that you give all of your writing and all of your art, um, so that they can be crushed in the hydraulic press and then out comes, um, your AI concierge that will do the dating for you.
(laughter)
Emily M. Bender: All right. We are at time. Thank you so much, Meg. That's it for this week. Our theme song is by Toby Menon. Graphic design by Naomi Pleasure-Park. Production by Christie Taylor. And thanks as always to the Distributed AI Research Institute. If you like this show, you can support us by rating and reviewing us on Apple Podcasts and Spotify.
And by donating to DAIR at DAIR-Institute.Org. That's D A I R hyphen institute dot org. Your turn in the outro script Meg.
Margaret Mitchell: Oh, I got so distracted by what you were doing. I'm sorry. Find us and all our past episodes on Peertube and wherever you get your podcasts. You can watch and comment on the show while it's happening live on our Twitch stream.
That's Twitch.TV/DAIR_Institute. Again, that's D A I R underscore institute. I'm Margaret Mitchell.
Emily M. Bender: And I'm Emily M. Bender. Stay out of AI hell y'all.