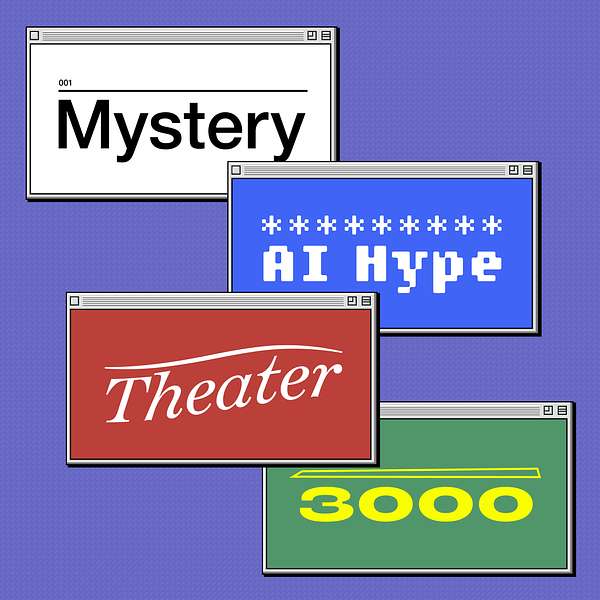
Mystery AI Hype Theater 3000
Mystery AI Hype Theater 3000
Sam Altman's Fever Dream, 2025.01.13
Not only is OpenAI's new o3 model allegedly breaking records for how close an LLM can get to the mythical "human-like thinking" of AGI, but Sam Altman has some, uh, reflections for us as he marks two years since the official launch of ChatGPT. Emily and Alex kick off the new year unraveling these truly fantastical stories.
References:
OpenAI o3 Breakthrough High Score on ARC-AGI-Pub
From the blog of Sam Altman: Reflections
The brain is a computer is a brain
Fresh AI Hell:
"Time to Edit" as a metric predicting the singularity (Contributed by Warai Otoko)
Seattle Public Schools calls ChatGPT a "transformative technology"
A GitHub pull request closed because change would have been unfriendly to "AI" chat interface
Elsevier rewrites papers with "AI" without telling authors, editors
The UK: mainlining AI straight into their veins
Check out future streams at on Twitch, Meanwhile, send us any AI Hell you see.
Our book, 'The AI Con,' comes out in May! Pre-order now.
Subscribe to our newsletter via Buttondown.
Follow us!
Emily
- Bluesky: emilymbender.bsky.social
- Mastodon: dair-community.social/@EmilyMBender
Alex
- Bluesky: alexhanna.bsky.social
- Mastodon: dair-community.social/@alex
- Twitter: @alexhanna
Music by Toby Menon.
Artwork by Naomi Pleasure-Park.
Production by Christie Taylor.
Welcome everyone to Mystery AI Hype Theater 3000, where we seek catharsis in this age of AI hype. We find the worst of it and pop it with the sharpest needles we can find.
Emily M. Bender:Along the way, we learn to always read the footnotes, and each time we think we've reached peak AI hype, the summit of Bullshit Mountain, we discover there's worse to come. I'm Emily M. Bender, Professor of Linguistics at the University of Washington.
Alex Hanna:And I'm Alex Hanna, Director of Research for the Distributed AI Research Institute. This is episode 48, which we're recording on January 13th of 2025. And we're starting the new year right, with a focus on OpenAI. Not only is their new o3 model allegedly breaking records for how close an LLM can get to the mythical human like thinking of AGI, Sam Altman also has some reflections for us, as he marks two years since the official launch of ChatGPT.
Emily M. Bender:Yes, in a new blog post, Altman opines about his abrupt firing and return to the company last year, and predicts a, quote, "glorious future" of AI agents, quote, "joining the workforce," as soon as this year. He's sure, so sure, that the company knows how to build AGI, and spins a yarn about future in, quote, "super intelligent tools" that could accelerate scientific discovery, innovation, and at last, abundance and prosperity. For whom, though, he doesn't specify. So, let's get into it and start unraveling this fantasy story, shall we?
Alex Hanna:Let's shall. We shall. What is the phrase? Anyways, let's do it.
Emily M. Bender:Yes, let's do it. All right let me just make sure that I've got my things queued up the right way and I'm going to take us first to the ARC Prize presentation here. Where this is by Francois Chollet from December 20th of 2024. And it is published on ARCPrize.Org. ARC is A R C and the, um, headline is "OpenAI o3 Breakthrough High Score on the ARC-AGI-PUB.
Alex Hanna:Yes. So just a little bit of background of like what the ARC prize is. Just on their, from their main page,"The ARC prize--" And I'm pretty sure ARC stands for Alignment Research Center, um, "The ARC prize is a $1 million plus--" I don't know why it's plus, um."--public competition to beat and open source a solution to the ARC hyphen AGI benchmark. Hosted by Mike Knoop, co founder of Zapier and Francois Chollet, creator of the ARC AGI and Keras." Um, and they're the, uh, folks at the helm of this situation.
Emily M. Bender:Yeah. So it's not alignment. Cause we see here over in this next little bit, ARC-AGI, they say"Most AI benchmarks measure skill, but skill is not intelligence. General intelligence is the ability to efficiently acquire new skills. Chollet's unbeaten 2019 Abstraction and Reasoning Corpus for Artificial General Intelligence--" So that's what it is. Abstraction and Reasoning Corpus.
Alex Hanna:Oh, got it. Yeah. They're overloading ARC, yeah, sorry.
Emily M. Bender:"--is the only formal benchmark for, of AGI progress. It's easy for humans, but hard for AI." That's their tagline. And then they have some examples here. So it's these visual puzzles. And, you know, they've got these, uh, input output pairs and you get a few examples to try out or just to sort of figure out the pattern is and then the test is taking new input and give the output. And I guess the thought here is that each one of these represents a different skill that the system is supposedly acquiring by studying the examples, but it is, all of the examples I've seen are sort of this general genre of, of task.
Alex Hanna:Yeah. So it's, it's getting at the sense of generability and, uh, we can try to describe what this looks like. So this, there's this one puzzle that, um, shows up on the first one and they're like these grids. And--
Emily M. Bender:Oh, you want the ones down at the bottom here?
Alex Hanna:Uh, yeah, no, that that's the one I'm looking at. Yeah. Yeah. Oh, that one, that one. Yeah. Yeah. The, the one that was looking, no, no, the one that we were looking at on the image page. Yeah. So it, so there's, there's this kind of like a partial Tetris piece and there's like, um, that are at a right angle and effectively you're trying to, the output is like dropping the missing piece here. Uh, and they're all in different configurations. So they make that two by two square, uh, and then they have kinds of different kinds of grid things. And the graph, uh, a little lower is kind of interesting because it's, you know, it's, it's this fallacy of, of basically saying what is human capability. So they've got, uh, common benchmarks we've talked about before, including a SQuAD and GLUE and SuperGLUE, um, and ImageNet. Uh, and then there's this line that is "human capability" in quotes. And then the thing that's really silly here is that there's a ARC-AGI, uh, benchmark, and then there's a, like, kind of an, a, uh, something that kind of approximate, like, approximates a logarithmic curve that would say like, before we had this prize, it would be negative 0.5 percent or negative 0.5, but then we launched the prize and like, look how much, and it's just like, goes straight up. So exponential growth, because we had a benchmark on it.
Emily M. Bender:I mean, talk about when a metric becomes a metric it ceases to be a metric, right? Like there's a better way of saying that.
Alex Hanna:Yeah, yeah.
Emily M. Bender:But yeah, this is, this is so frustrating. So they, yeah, they had this dash line, which is the "status quo forecast pre ARC Prize". So, you know, completely fake line on the graph. Um, and then we put the prize out and instead of following that fake line, look, the systems were being trained to do the thing. And the score went up.
Alex Hanna:Yeah. No, we made, we made the score get up. We did, we did Godwin's law. Check it out.
Emily M. Bender:Thank you. Yes. That's what I'm looking for. So they also have a couple of paragraphs here on AGI and defining AGI. So AGI says, "LLMs are trained on unimaginably vast amounts of data yet remain unable to adapt to simple problems they haven't been trained on or make novel inventions, no matter how basic. Strong market incentives have pushed frontier AI research to go closed source. Research attention and resources are being pulled towards a dead end. ARC Prize is designed to inspire researchers to discover new technical approaches that push open AGI progress forward."
Alex Hanna:Yeah, it's, it's, it's a, it's a bad, it's some bad vibes. And this, their definition of AGI is, uh, great. I mean that in a parentheses derogatory.
Uh, so the "Consensus, but wrong:AGI is a system that can automate the majority of economically valuable work." Which is, uh, I'm pretty sure, pretty close to what's in the OpenAI charter. It's also pretty close to what I think Mustafa Solomon says, um, an, an, uh, former, um exec at DeepMind. Now they define "correct," which is very funny. No citations or, you know, like any kind of backing of this, but, "Correct: AGI is a system that can efficiently acquire new skills and solve open ended problems." Okay. Like, why is this now, why is this now the definition? And secondly, why does that you know, why have we moved? It's so interesting. Like why has this moved from like a political economy, um, angle, which to, to, to be fair, should be ridiculed, but, uh, also has sort of like some kind of externality to something that can quote,"acquire new skills," not defining what a skill is or an open ended problem.
Emily M. Bender:But they say, Alex, definitions are important.
Alex Hanna:Yeah. They say definitions are important and yet.
Emily M. Bender:Yeah. So--
Alex Hanna:I have questions. Yeah.
Emily M. Bender:So the next bit is, "Definitions are important. We turn them into benchmarks to measure progress towards AGI." Which comes right back to this thing. And so, um, SJayLett is helping us out."It's when a metric becomes a target, it ceases to be an effective metric." And Kh0rish in the chat also saying, "Benchmarks: definitely for sure, always perfectly measuring what they say they are." And then the last thing like gives Right."Without AGI, we will never have symptoms, sorry, systems that can invent and discover alongside humans." And it's like, maybe we don't actually need that.
Alex Hanna:Yeah. At the end of the page, it's also interesting because it looks like Knoop and Chollet, like are, it says that they're actually sponsoring this 'cause I'm like, well, where are they getting this money?
Emily M. Bender:Mm-hmm. Alex Hanna: And are are Knoop I don't know. Knoop and Chollet are, are they putting up their own money? Their own one, one mi--they just think it's important enough that they-- Uh, they're making it look like that.
Alex Hanna:Yeah. Well, good for them.
Emily M. Bender:Okay, so, so this is the background on the prize. Let's go back to their thing about OpenAI's o3. So, uh, "OpenAI's new o3 system trained on the ARC AGI 1 public training set--" So this is not like completely held out kinds of tasks. We're going to show them this thing, right?"--has scored a breakthrough 75.7 percent on the semi private evaluation set at our stated public leaderboard, 10,000K compute limit. A high-compute, 172X o3 configuration scored 87.5 percent." So what they've done is they have taken the public training set, which is a whole bunch of these things. And so the idea with generalization here is that each one of the little picture puzzles has a different generalization in it. But the general shape of it is the same across all of them, as far as I can tell. And so OpenAI had access to, um, I think about 300 of those and train that and also probably, who knows, did some architecture changes in their system to like optimize it for this and then handed it over. Um, uh, I think we're going to skip this little bit of hype here. Um, somewhere it says, yes, "At OpenAI's direction, we tested at two levels of compute with variable sample sizes." So ARC now is like collaborating with OpenAI on this.
Alex Hanna:Yeah, I mean, they're, they're, I mean, they're effectively saying, like, you need to run it like this, which, you know, like, to some degree is like, okay, like, there's certain configurations that you need to get right, and I will grant you that, but it is then saying, um, is then saying, like, you know, this is not a true benchmark. This is not kind of like a withheld sort of thing. There is like some collusion in this that makes this like not a very useful research instrument. Um, and there's a lot of things that are not reported in this, which is, um, which is a hallmark of hype, you know, um, effectively saying that this is a semi private sort, um, this is kind of a, this is a semi private eval in addition to sort of saying, and they kind of give something at the end where they're saying, we're going to open source this kind of at the end, but the kind of thing that's being said here is like, well, OpenAI, we're working with them closely. And not disclosing all of this because there's strong incentives to be like a first mover here. Um, so we're going to hype them up a bit, uh, and then we'll just open source it eventually, which is, um, pretty annoying.
Emily M. Bender:Yeah. So they, and they're also sort of like hemming and hawing about the cost per task. Um, and they say, "Despite the significant cost per task, these numbers aren't just the result of applying brute force compute to the benchmark. OpenAI's new o3 model represents a significant leap forward in AI's ability to adapt to novel tasks." This is so frustrating because they are talking about AI as if it were one thing, right? OpenAI tuned their o3 model to be able to do this task. And now, um, Chollet and Knoop, Knoop, whatever his name is, are saying, look, AI as a whole has made a leap forward. There's a new ability here.
Alex Hanna:Well, it's interesting too, because when you kind of go down on this and there's, they talk about cost and performance and tasks, and we'll get to the, the kind of estimation of sustainability and how much carbon this takes, which I mean, when you look at compute costs, you also need to always look at energy and sustainability. Um, but the, the discussion of the architecture here, so there's a heading that's what's different about o3 compared to older models. So. So there's this thing here, which, which Chollet says, "My mental model for LLMs is that they work as a repository of vector programs." So first off, that's like, let's break that down. So like a repository of vector programs. So that's sort of saying, this is such a weird epistemology or like, this is a weird metaphysics of like what an LLM is, because it's sort of saying that tokens themselves are programs or like in, in vectors of tokens are programs and you just need to sort of execute that, which is like such a bizarre internal mental model of what these things are.
Emily M. Bender:Right. And I forget whose point this was, but someone was pointing out that if you asked ChatGPT to do arithmetic, its response took exactly the same amount of time, no matter how much time it would take to actually compute the problem that you're asking it to do, which is a very clear indication, as if we didn't know already that it is not actually executing a program.
Alex Hanna:Yeah, yeah, that's right. That's right. That's, that's an excellent point. And so to pose it, I mean, and just to back up, like, as a repository of vector programs, like a, you're kind of, is that like an analog of saying that--then what's, an LLM then is like importing a class library and a programming language, is that an LLM? Like, is it, is, is, is, is a, is a set of bytecode encoded like the string library in Java, is that an LLM? Like what is now? And I'm so, I'm just like, I'm so, I'm very befuddled at that, at that claim. That said, if you believe Cholet and you start there, you say, "When prompted, that they will fetch the program that your prompt maps to and quote, 'executes' it--" Again, doesn't execute it at all. Uh, it just predicts, uh, most probable next token to some degree with, with some, with some, um, some like reinforcement learning on the tail end and, "--on the input at hand. LLMs are a way to store and operationalize millions of useful mini programs via passive exposure to human generated content." And I'm like, wait, wait, that's not what it's doing.
Emily M. Bender:And on top of that, that requires thinking about people as computers. Right, so this human generated content is, you know, either just the text that's been scraped by the web or OpenAI commissioned a whole bunch of chain of thought sequences probably. And so Chollet is saying those are programs, they're programs that are produced naturally by people and LLMs can execute them now because they have an index of all of them.
Alex Hanna:Yeah. Well, it's, I don't even, I would say like, you don't have to go as far as seeing it's humans as programs is that you think that human generated content is, has effectively like, you know, humans generate outputs and there's ostensibly maps to some kind of internal input at that you can operationalize those as programs as if like humans--I mean, like, yeah, I mean, I could see it, but I'm just like, it's such a, it takes you down like a model of thinking that is bizarre. And one of these days we have to dig into, Chollet has this essay on intelligence that I've been meaning to read because it's, it's sort of like an insight into like, what he thinks humans are doing and what intelligence is.
Emily M. Bender:So there's a wonderful paper by Baria and Cross that I don't, I think it only exists as a manuscript called something like, uh, "The brain is a computer is a brain," and it's talking about the computational metaphor in neuroscience. Um, and it's, it's a really valuable piece where they sort of call out this thinking that basically says our mental models of what happens in machines are a good model of what happens in humans. And I think that Chollet is, or whoever wrote this is deep in that here.
Alex Hanna:Yeah, completely.
Emily M. Bender:Yeah. So there's some stuff in the chat to bring up.
Alex Hanna:I know. I just wanted to say this thing from Scupper Club, first time chatter. Hey."JVM--" As in the Java Virtual Machine."equals, equals LLM," which is very, very funny. Yeah.
Emily M. Bender:And it's also a bunch of commentary in here about, um, how benchmarks aren't, there's no construct validity. So I think it was Abstract Tesseract probably? Yes."Apparently you don't have to worry about a benchmark's construct validity if it's the only one."
Alex Hanna:That's, that's, that's very funny.
Emily M. Bender:And Faster And Worse points out like, "No concrete purpose means no criteria to determine success or failure." And that's exactly it. Like, what is this benchmark actually measuring? And if we were doing serious science or engineering in the world, we would be either saying, this is a way that we know that we can use this safely and effectively for this particular task. Or we'd be establishing construct validity because we're doing science. And neither of those things are happening.
Alex Hanna:Well, I was, I was having this conversation this morning with one of, um, one of the PhD students that works at DAIR, Raesetje Sefala, uh, who's doing some really interesting work on, on error analysis. And we were just having this conversation about construct validity and how the field of AI slash computer science really doesn't do anything with it, doesn't really think about measurement, hasn't engaged with the giant literature on measurement from, from scientometrics, from political methodology, from education. Yeah. Just like giant kinds of portions, uh, like, um, um, you know, social sciences and even, I mean, like, especially the quantitative social sciences, yeah, what the hell is a Lickert scale actually. Um, but no, there's no, there's no engagement in that at all.
Emily M. Bender:Yeah. Yeah. All right. What else do we want to say about this in here? Um, I guess also this, yeah, so this, the whole idea of like, it's retrieving these things and executing them. No, it's not. As you say, Alex, it's just outputting the next word. And that was then conditioned on the so called program that it wrote. Um, and it's just, this is--the other thing that's super frustrating here is that even though OpenAI has collaborated with the ARC Prize people on this. They have not been transparent about what's inside their system, how they built it. And so here we have Chollet like, making stuff up, sort of, you know--
Alex Hanna:Yeah.
Emily M. Bender:--guessing what might be going on in the inside when there are people who design that system who could answer these questions.
Alex Hanna:Yeah, that's that's very true and very frustrating that they do this. And, and Chollet kind of speculates. He said, basically there's certain kinds of spaces of possible chains of thought, which are, uh, may or may not be human annotated, describing the steps required to, to, to solve a task in a fashion, and this is what gets me that I think is really funny. Um, in a task, "in a fashion, perhaps not too dissimilar to Alpha Zero style Monte Carlo Tree Search." And so I'm just like, okay, so if you're, if you're developing something like Alpha Zero which is aimed, which is with the goal of either solving, uh, Go or chess, which I think, was AlphaZero chess? Um, I think it was originally chess and then AlphaZero Go was chess. And so if you actually are like doing some kind of a tree search of possible outcomes and you're just sort of, you know, optimizing perhaps like the most optimal way to do a tree search. Then again, like, why do you think these tasks have any kind of external validity to not just filling in patterns on a little grid format? Uh, like. Like, why does this have any construct validity outside of this little game scenario? And I thought that was just so bizarre as like in really unreflective to even assert that.
Emily M. Bender:Yeah. And I think basically because when Chollet came up with this thing, none of the existing systems could do it. He then gets to claim, this is the thing that's going to drive people towards AGI because we don't have a solution for it yet. And then OpenAI goes and takes a bunch of the examples as training data, like, hey, look, we can do it. Yeah.
Alex Hanna:Yeah.
Emily M. Bender:So I love this comment in the chat from, um, Method And Structure,"Kremlinology around LLM contents is kind of like SEO snake oilers trying to divine what the Google algorithm is doing."
Alex Hanna:Yeah, no, that's, that's a, that's a great, um, that's a great comparison there.
Emily M. Bender:Yeah. Um.
Alex Hanna:Okay. What else is in this, uh, artifact? Um, there's, um, some stuff, um, there's what comes, so this is the, what comes next, which I thought was pretty interesting."So what comes next? First of all, open source replication of o3--" Uh, I'm like, okay."--facilitated by ARC Prize."
Emily M. Bender:Based on what? Based on what?
Alex Hanna:Yeah, I, I don't know. It says, it just asserts that. Uh, "--facilitated by ARC Prize competition 2025 will be crucial to move the research community forward. A thorough analysis of o3's strengths and limitations is necessary to understand its scaling behavior, the nature of its potential bottlenecks, and anticipate what abilities further, these further developments might unlock." Um.
Emily M. Bender:Can we just appreciate the video game metaphor here?
Alex Hanna:Yeah.
Emily M. Bender:Right? These developments are going to unlock abilities. In what world does that happen? Oh, right. Video games.
Alex Hanna:Well, I'm also, like, very, it's just, I'm so tickled about, like, what is the, well the next, the next sentence kind of throws me because I don't understand what this means and how you quantify this. And I've never seen this language in AI. And I'm like, how do you even describe these problems? Anyways, they say, "Moreover, ARC AGI One is now saturating." Um, which I don't, I've never heard as like, well, I've heard of this term saturation in terms of benchmarks, but like, again, what does that mean with regards to real world, real world problems?"But setting that aside, beside o1's, o3's new score, the fact is that a large ensemble of low compute Kaggle solutions can now score 83 percent on the private eval." So that's effectively say the pub, so the public, the public eval are these quote quote low compute Kaggle solutions. Uh, and for those of you, those of you listening, Kaggles like this, um, you know, it's a, it's kind of a competition platform that was once independent and then Google bought them up. And so it's sort of like, and it's had small little data science problems, like, you know, predict--what was the Titanic one, like predict who like got off the Titanic or whatever, um, and then--which is macabre in its own right. But then they've also got other things, like predict, you know, income from this old census data set and all these other toy problems.
Emily M. Bender:Yeah, basically like a machine learning leaderboard. It was an interesting moment because prior to that, you would have a bunch of shared tasks, but they were always like connected to academic events where people would come and talk about what they did. And then Kaggle's like, oh, yeah. Here, let's just have the competitions without the actual discussion and then people would put it on their resumes like I won, you know, I was the top of the leaderboard on this Kaggle thing. And yeah, so this thing about saturating.
Alex Hanna:Yeah.
Emily M. Bender:Um, like that is a metaphor that we talk about, right? You see it when they're talking about the benchmarks and what's sort of shocking here is that to anyone who's not wedded to this idea that these benchmarks are actually driving progress, it just clearly shows that benchmarks get saturated or worn out because they're not effectively measuring anything. But the people who are down inside this rabbit hole are like okay, well this one saturates we got to build a new one.
Alex Hanna:Yeah. Yeah. And it's really it's pretty upsetting, like people get really taken in about this. I saw, um, like, you know, Terence Tao, the famous mathematician, he's become like very fascinated with like LLMs and like solving a mathematical problem.
Emily M. Bender:I know. Super disappointing.
Alex Hanna:Yeah. And I'm like, ah, he was actually a, A friend of mine's advisor and I'm like, uh, I'm like, ah, your man is, come get your man. He is just like completely like, like, uh, engrossed in this LLM uh, nonsense.
Emily M. Bender:Yeah. Yeah. So before we leave this, I just wanna point out that there's a really obnoxious visual effect. The font is terrible. It's hard to read. And then the background, they've got this moving thing that looks like you're in the Tron universe. I was like, I didn't need that while I was trying to read this. Okay, so here's the open source analysis that I think we can skip in some examples, but we should go to this analysis that went up on LinkedIn.
Alex Hanna:Yeah.
Emily M. Bender:Um, someone named Boris, um, Gamazaychikov, whose tag is "head of AI sustainability at Salesforce", um, sat down and tried to estimate the carbon emissions for each of those tasks.
Alex Hanna:And I want to, I want to note just his first sentence because I think it's, it's a little deceptive. So, "OpenAI has announced o3, which appears to be the most powerful AI model to date." And it's first off, no, because it seems like o3 has just been engineered particularly for this ARC AGI challenge, if I'm not wrong, um, where it is, it is meant to do a certain kind of search, like deep search according to like Chollet's analysis. So like, and again, like there's, there's, it's one of those situations where you're like, what, what do you mean by powerful? And what do you mean by AI? But that said, um, you know, it's the, it's the environmental cost. And of course, they didn't really release this. They had sort of a, a, um, a measure of compute, which he is then, um, doing some, um, uh, um, some calculation. So, "Each task consumed approximately 1,785 kilowatt hours of energy, about the same amount of electricity an average US household uses in two months. This translates to 684 kilograms of, um, of carbon dioxide emissions, based on last month's US, uh, uh, grid emission factor. To put that in perspective, that's equivalent to the carbon emissions from more than five tanks of gas, full tanks of gas."
Emily M. Bender:Wonderful. And there's details of assumptions down below, which is, which is nice for sort of substantiating that this was a serious analysis. Um, and yeah, it's like, and for what? Right?
Alex Hanna:Yeah. To solve a little, a little, a little graph, um, problem here, not even a graph problem. I mean, you could formulate as a graph problem, but OpenAI is, is formulating as a token problem.
Emily M. Bender:Oh, and I guess I wanted, I wanted to, um, click through to that too, because they do give the link here to the prompt that they gave to o3. Where did that go? Oh, Um, but just my little search function here. Um, uh, here's the prompt. So we keep seeing these pictures where they're colorful. But that's not how the GPTs from OpenAI are built. And so what they've done instead is they've, they've basically, I guess, numbered the colors and said, here's the input, here's the output. Um, and I don't understand why these grids aren't the same size, um, but basically the prompt is, "Find the common rule that maps an input grid to an output grid," example one, input output, example two, input output, example three. And , "Below is a test input grid, predict the corresponding output grid by applying the rule you found. Your final answer should be just the text of the output grid itself." So it's all translated to these numbers.
Alex Hanna:I think that the grids aren't the same because the task is like an expansion task. Effectively, it's like, um, so you have this five by five grid.
Emily M. Bender:Oh yeah. It's just doubling everything, isn't it.
Alex Hanna:And then it, and then what it does is that each, each, uh, five in it, each color, let's say, uh, becomes four squares and then it draws like a line through it, it looks like. So there's like--anyways.
Emily M. Bender:Yeah, it's got it's got something is doing there. And that's why these ones are getting bigger. Okay, that makes some sense. I'm kind of curious how often o3 gave back something that wasn't even the right format. But that's a separate question. So should we shift on over to Sam Altman's reflections?
Alex Hanna:Ugh, if we must.
Emily M. Bender:So this is a blog, um, on, so it's Blog.SamAltman.Com. And this is dated January 5th of 2025. And the title is just, "Reflections," which kind of made me wonder, like, does he reuse titles or does he just not reflect that often?
Alex Hanna:It'd be very ironic.
Emily M. Bender:Yeah. So you want to take us into this one, Alex?
Alex Hanna:Yeah, let's see. What's the ch--what's the, what's the chonk of this? So the first paragraph, he says,"The second birthday of ChatGPT was only a little over a month ago. And now we have transitioned into the next paradigm of models that can do complex reasoning." Um, and this I'm just assuming that he's, uh, referencing, um, o3 and also GPT-4o."New years get people in a reflective mood. And I want to share some personal thoughts about how it has gone so far and some of the things I've learned along the way." Ah, okay.
Emily M. Bender:"As we get closer to AGI--" There's some serious presuppositions going on in that phrase, that AGI is a thing, that we're getting closer to it, but he's just sort of sticking in there as a presupposition, so you got to go along with it, right?"As we get closer to AGI, it feels like an important time to look at the progress of our company. There is still so much to understand, still so much we don't know, and it's still so early, but we know a lot more than we did when we started."
Alex Hanna:Yeah, I want to, and let's discuss, but I really want to read this next paragraph. So, "We started OpenAI almost nine years ago because we believe that AGI was possible and that it could be the most impactful technology in human history. We wanted to figure out how to build it and make it broadly beneficial; we were excited to try to make our mark on history. Our ambitions were extraordinarily high. And so is our belief that the work might benefit society in an equally extraordinary way." And just like, man, talk about someone that is just completely disbelieves they're, they're the best thing since sliced bread. Just, just absolutely nonsense.
Emily M. Bender:But meanwhile, we also have the like, "still so early," which puts me in mind of Anna Lauren Hoffman's analysis of this like infancy metaphor. Um, okay. So, uh, 'people didn't care cause they thought we had no chance of success.' And then, um, "In 2022, OpenAI was a quiet research lab working on something temporarily called, 'Chat with GPT-3.5'." And then parenthetically, oh, so humbly, "We are much better at research than we are at naming things." And I'm like, okay, maybe OpenAI wasn't like a household topic of conversation in 2022, but they were sure making enough noise to annoy, like all of the field of natural language processing. Like, so, you know, um, and yeah, so, um--
Alex Hanna:they're also saying that we are much better at research than we are at naming things. I mean, I would say you're not very good at research, but you know,
Emily M. Bender:But you know, also they did not run their name past speakers of other languages. You know, the whole thing about ChatGPT in French, right?
Alex Hanna:No, I don't.
Emily M. Bender:You pronounce that, in France it's "Chat, j'ai pété," which is "Cat, I farted."
Alex Hanna:I say this as I'm holding one of my cats. And hopefully she does not fart on me.
Emily M. Bender:Okay, then, uh, "We always knew abstractly that at some point we would hit a tipping point and the AI revolution would get kicked off, but we didn't know what that moment would be. To our surprise, it turned out to be this--" And this is the launch of ChatGPT, and it's like, no? I mean, certainly, what changed in the world with the release of ChatGPT was a tsunami of AI hype and everyone trying to get on board and all that kind of stuff, but it's not the technological revolution that they're imagining.
Alex Hanna:Yeah, that's, that's, that's right. I mean, the kind of what you did is that you had a public interface and then hyped it up incredibly lots and then made teaching a lot harder and were the bane of every instructor's existence.
Emily M. Bender:Yeah. And lots and lots of other things too, as we document in our book.
Alex Hanna:Yeah. Yes.
Emily M. Bender:Medusa Skirt says,"Truly the writing of a man who really wants you to think he's the Oppenheimer of machine learning."
Alex Hanna:Oh, it also reminds me of this, I don't know if we covered it on the podcast. It was, but it was the, one of the people who was, um, developing like weapons and using AI for weapons, and they were saying, oh, I'm like Oppenheimer. This is great. I'm like, that's, what? Are we watching the same movie? Okay.
Emily M. Bender:I need to know better than to take sips of tea during this podcast.
Alex Hanna:Yeah. Sorry. I apologize.
Emily M. Bender:I avoided the spit take, but just barely.
Alex Hanna:So there's a bunch in here basically about, you know, the, the palace intrigue, um, you know, about OpenAI. Which, you know, is, is, is funny and sad and annoying. Um, also give it, giving a shout to Karen Hao's book. She's, she's coming out with a book called Empire of AI. I gotta search this. And I'm sorry to, um, sorry to Christie, clacky I just typed on my very clacking keyboard trying to search for this. Uh, yeah."Empire of AI." Which is coming, gonna come out in July, but it's all about the, um, the kind of drama around OpenAI. So, um, but he goes into this, talking about like how he got fired. Um, he learned the importance of a board, a board with complex, "with diverse viewpoints and broad experience in managing a complex set of challenges." And "good governance requires a lot of trust and credibility." Um, meanwhile, you know, half of his board resigned, um, in the, in the intervening period.
Emily M. Bender:And this, this next paragraph at the end of that little section, which he's got set off by horizontal rules, uh, "We all got back to the work in a more cohesive and positive way, and I'm very proud of our focus since then. We have done what is easily some of our best research ever." And then the way he substantiates that, next sentence, "We grew from about 100 million weekly active users to more than 300 million." That's, that's not a sign of doing good research. That's a sign of marketing.
Alex Hanna:Yeah. Like the sign of just selling this and selling big enterprise contracts is what you're doing.
Emily M. Bender:Yeah.
Alex Hanna:Yeah. Um, okay. The last, the last part of this is really, really, really rough. Do you want to kick it off?
Emily M. Bender:Yeah."Nine years ago, we really had no idea what we were eventually going to become. Even now we only sort of know. AI development has taken many twists and turns and we expect more in the future. Some of the twists have been joyful, some have been hard. It's been fun watching a steady stream of research miracles occur. A lot of naysayers have become true believers."
Alex Hanna:That's what you want. You want true believers.
Emily M. Bender:Right. Like that, that's a real sign of science. Isn't it? Um, "We've also seen some colleagues split off and become competitors. Teams tend to turn over as they scale and open AI scales really fast. I think some of this is unavoidable. Startups usually see a lot of turnover at each new major level of scale and open AI numbers go up by orders of magnitude every few months. The last two years have been like a decade at a normal company."
Alex Hanna:It's all that AI they've got in the sauce.
Emily M. Bender:"When any company grows and evolves so fast, interests naturally diverge. And when any company in an important industry is in the lead, lots of people attack it for all sorts of reasons, especially when they are trying to compete with it." Oh, poor, poor OpenAI. They're just trying to be in the lead. They don't deserve all of that.
Alex Hanna:I know they don't deserve all this smoke. And hey, the people that are attacking it. It's just, it's just that, it's just that they just want to be us.
Emily M. Bender:Yeah.
Alex Hanna:Yeah. Yeah. Really rough. Um, so the next part,"Our vision won't change. Our tactics will continue to evolve. For example, when we started, we had no idea we would build a product company. We thought we were just going to do great research. We also had no idea we would need such a crazy amount of capital." Sorry. I didn't expect to cackle at this. I swear I read this and I just thought, you know, and I just go back to the, what is it? The 7 trillion amounts or whatever he says they have needed.
Emily M. Bender:And also this, "we had no idea". Like we had Brian Merchant on here two episodes ago calling BS on that.
Alex Hanna:Yeah.
Emily M. Bender:Right.
Alex Hanna:Yeah. That's not a, if you have someone like like Musk as an early investor. Yeah. You're going to make products and you're going to aim to make boatloads of money. Um, that's the goal. Uh, "There are new things we have to go build now that we didn't understand a few years ago. And there are new things in the future we can barely imagine now.""We are proud of our track record on research and deployment so far--" Um, well, that's, that's interesting."--and are committed to continuing to advance our thinking on safety and benefits sharing.""We continue to believe that the best way to make an AI system safe is by iteratively and gradually releasing it into the world, Giving society time to adapt and co evolve with the technology, learning from experience, and continuing to make the technology safer." Um, I'm going to finish the paragraph and then I want to come back to open the sentence.
Emily M. Bender:Yeah.
Alex Hanna:"We believe the importance of being world leaders on safety and alignment research and in guiding research with feedback for real world applications."
Emily M. Bender:Excuse me, but what real world applications have we actually seen that are worth anything?
Alex Hanna:Yeah. I mean, you're not, you're not getting to real world applications. You're infesting different products with, with more synthetic texts. And then the thing that really like grinds my gears on this is the"giving society time to adapt and cool evolve with the technology." Like what kind of Asimovian, you know, Foundation bullshit is this?
Emily M. Bender:And who do you think you are?
Alex Hanna:Yeah. Who do, who the fuck do you think you are that you're like some kind of people who are doing this kind of really forward thinking or predictive sort of, you know, predictive sort of technological breakthroughs and that we're doing it iteratively to let society catch up. And I'm like, no, you're not. You're careening from here to there. You're basically trying to skirt every regulatory constraint there is, even though there aren't that many, you're trying to buy up as much data as you can, and you're being as reckless as possible.
Emily M. Bender:And taking no accountability for the damage that's being done to the actual environment, to the information ecosystem, to all of these other industries that are falling for the AI hype. But if it's framed as, well, society gets to evolve because we're doing this little by little, that's basically saying, none of the hardships are our fault. None of the harms, it's not us. We're just taking the AGI, which was going to happen anyway, and sort of slowly putting it out into society.
Alex Hanna:Yeah. It's, it's ridiculous. Um, and I'm just very, it's just absolutely infuriating. And there's just such a delusion from the head of the stinky fish, um, of what, you know, they think they're doing here.
Emily M. Bender:Yeah. I, there's, there's a wonderful discourse in the chat here about, um, how we're talking about how they don't want to be beat up. They're just trying to leave. So people in the chat, starting with Steal Case says, " Wow, they're so widdle, just widdle boys." And then Abstract Tesseract comes in with, "$7 trillion smol bean," and Six Pairs Of Feet, "Still just a little stand on the sidewalk selling LLM-onade."
Alex Hanna:Very good. The chat's very good today. Keep going. Love it. And, uh, C Graziul says, "I was going to say Borg, resistance is futile, but very Foundation-y." Yeah. So, I mean, it's, yeah, it's, it's. And then SJayLett says, "Oh, he absolutely would love to imagine he's Selden."
Emily M. Bender:Oh, of course. Yeah. Yeah. Actually, not Selden in the original Foundation, but Selden in the pretty terrible TV adaptation that we've got right now.
Alex Hanna:I haven't seen that. People said, it's it's worth watching, but you don't like it?
Emily M. Bender:Eh, parts of it are, it's super slow.
Alex Hanna:Okay. I also heard that. Yeah.
Emily M. Bender:Yeah. No, I I think there's things that people object to about it, which are not the things that I object to about it but anyway, um, okay, so uh we've got, I think just a couple paragraphs left. So, "We are now confident we know how to build AGI as we have traditionally understood it. We believe that in 2025, we may see the first AI agents, quote,'join the workforce' and materially change the output of companies. We continue to believe that iteratively putting great tools in the hands of people leads to great broadly distributed outcomes."
Alex Hanna:Oh Lord. There's so much here. Um, yeah. Abstract Tesseract says, "We are here for the glorious future. Did anyone hear the ominous change in background music?" And, um, yeah, so it's, it's, it's just really um, absolutely bizarre. And then AI agents. We've already, we talked about this, I think, on the last podcast. And when we were going through the, uh, the hell backlog of that weird San Francisco company that has like hire an AI employee. So it's really giving, giving that. And then 'materially change the output of companies.' I don't know what that signals or what--is itit likehe amounts of output, like just putting, you know, are they going to become more productive and kind of a later economic sense? Are they just, are they literally, is, is Microsoft now going to become a company that you know, generates ponchos. I don't know. Like what is, what is materially change the output of companies mean?
Emily M. Bender:Yeah, yeah, exactly. I think it's looking at companies as functions from, uh, yesterday's stock prices to tomorrow's stock prices.
Alex Hanna:Yeah.
Emily M. Bender:So, okay. But it gets worse.
Alex Hanna:Yeah, it does get worse.
Emily M. Bender:Because this was"confident we know how to build AGI as we have traditionally understood it," was previous paragraph. And now you want to do the honors on this one, Alex?
Alex Hanna:Oh, geez."We are beginning to turn our aim beyond that to superintelligence in the true sense of the word." What the fuck is the true sense of the word? We don't have, that doesn't mean anything.
Emily M. Bender:But definitions are important. The ARC Prize people taught us that.
Alex Hanna:I know, but, but Sam's not listening."We love our current products, but we are here for the glorious future." And here's the, oh, I thought glorious future was in the prior, uh, graf. But no, here, here it is. The glorious future, Dear Leader says."With superintelligence, we can do anything else. Superintelligent tools could massively accelerate scientific discovery and innovation well beyond what we are capable of doing on our own and in turn, massively increase abundance and prosperity." So we're back here to this idea of science as being this black box, this kind of notion, this thing that we've gotten from, uh, Sakura, Sakura? No.
Emily M. Bender:Sakana.
Alex Hanna:Sakana. Thank you. Of course, uh, confusing it with another Japanese word. And, um, and then, uh, the, uh, the Nobel, um, Turing Prize. Um, and these kinds, I feel that, like, science is this thing that you just do more thinking, or you execute more tasks, and then, brrr, you know, more economic productivity happens. And it's just, ugh, just absolute, this is, this is the, the bullshit of the highest degree.
Emily M. Bender:Yeah. Uh, so last paragraph, right. Oh, and I want to say, you know what would actually increase abundance and prosperity? Doing something about the climate crisis.
Alex Hanna:Yeah. Yeah. Well, also the idea that like you, as if this is an aspect of, you know, we're, we're recording this the second week, I already said the second week of January and at this moment. Yeah. You know, we're seeing the massive devastation in LA County and the Palisades and the Eaton fire. Just mountains, just mounds and mounds of people displaced. And it's not like this stuff and climate refugees weren't being made. Uh, but it's now we're seeing this massive thing in the US and you know, what we're also seeing is landlords now using it as an opportunity to extract even higher rents from people fleeing LA County, um, in the surrounding area. We're seeing, uh, insurers drop people right before their homes had burned. We're seeing this, um, like massive destruction of people's personal property and huge state failure. And I'm just like, in what world would burning through all this carbon aid any of that? Increase abundance? We have the material necessary and it's a political, it's a distribution problem.
Emily M. Bender:Exactly, exactly. And it, abundance gets harder and harder and harder, the more we make the planet uninhabitable.
Alex Hanna:Yeah.
Emily M. Bender:Right? Okay, so last paragraph."This sounds like science fiction right now and somewhat crazy to even talk about it. That's all right. We've been there before and we're okay with being there again. We're pretty confident that in the next few years everyone will see what we see and that the need to act with great care while still maximizing broad benefit and empowerment is so important. Given the possibilities of our work, OpenAI cannot be a normal company." So Magidin the chat says, "We've been there before and we're okay with being there again. Sounds like you aren't making progress and you're okay with that. Nice to know." And then he ends with how lucky and humbling it is to be able to play a role in this work. I don't think Altman knows the meaning of the word humble.
Alex Hanna:No, no, certainly not. Every time, every time I think about the, you know, the kinds of illusions that he says, the, the magnanimousness of his company, I think of him driving that 2 million car um, around the streets of San Francisco. Like oh, yes, you're very fe--oh he also says something here. I think we we left it, but he was like, it's in the middle part, but he's like, you know sometimes I think--yeah this I love this paragraph and then after this we'll get into Hell. He says, "These years have been the most rewarding, fun, best, interesting, exhausting, stressful, and particularly for the last few, unpleasant years of my life so far. The overwhelming feeling is gratitude. I know that someday I'll be retired at our ranch watching the plants grow, a little bored, and we'll think back at how cool it was. I got to do the work I've dreamed of since I was a little kid." First off, only, only folks like you have a ranch, you know, like most of us don't have a ranch by far. Uh, second off, you know, like my vision of what you're going to be doing 30 years from now is probably hunkering down in your ranch because you've, uh, really sown and aided and abetted in the destruction of the natural world. And you're just hiding away thinking that you could, you could avoid any kind of, you know, accountability that you should be encountering now.
Emily M. Bender:That ranch is probably in New Zealand where all the billionaires have bought up their escape. Yeah. Okay. So are we doing musical or non musical for the transition this time?
Alex Hanna:No, I'm feeling, I'm feeling pretty musical today.
Emily M. Bender:All right. Okay. So what's the, what's your, what's your musical genre?
Alex Hanna:Uh, well, we're talking about a ranch, so.
Emily M. Bender:Okay. Um, so here's the deal. Uh, OpenAI and company have figured out, you know how all of this stuff is usually people in the background, right? And they're shipping the tasks off. Well, they figured out how to ship those tasks off to the demons of AI hell. And so you're there in the, you know, the mines doing the, the, the, what are they called on Mechanical Turk? The human--
Alex Hanna:Yeah, like that click work.
Emily M. Bender:Yeah, the click work. But, um, and then these ARC Prize graphs start falling on you. And so you're going to give me a what? Country music appropriate for the ranch hand's song about that.
Alex Hanna:This is so deep. All right. So you're a demon. You're like, all right, so let me think of it. Down here in the content minds, storing matrices, putting them in line, doing tasks that only we can do. Just so Sam can say he's smart too. Winning the ARC Prize down in AI Hell. Winning the ARC Prize, AGI's going swell. Wining the ARC Prize. We're doing it for humanity, but don't look too closely or you might not like what you see.
Emily M. Bender:You might not like what you see, but I love the song.
Alex Hanna:(speaking) Thank you. I think this is the first one in a while I just haven't completely bailed on.
Emily M. Bender:It went really well. Okay. So here we are in Fresh AI Hell. I've got an ambitious number, but most of these, we agree, we're basically just going to hit the headlines. So this is from Popular Mechanics by Darren Orff published November 30th of 2024. Um, headline, "Humanity may reach singularity within just six years, trend shows."
Alex Hanna:'Trend shows' is doing a lot of work on this headline.
Emily M. Bender:Exactly. And so here, the, the main point I wanted to bring up is in their summary,"A translation company developed a metric, time to edit, TTE, to calculate the time it takes for professional human editors to fix AI generated translations compared to human ones. This may help quantify the speed towards singularity."
Alex Hanna:This is, this is absolutely wild. Do they have a link? Like who's, what metric is this?
Emily M. Bender:Uh, so.
Alex Hanna:Oh, it's a translation company, so they don't even have a paper, like an arXiv paper or anything, do they?
Emily M. Bender:Yeah, it just, yeah. So basically the idea is, we can see some kind of progress because this translation system is getting better and better on first go. So that change is going to tell us how fast the singularity is coming. Because those are the same thing. And this, this comes back to the problem of treating all of this as AI, as one thing and like, including like various technologies that actually exist that work well or not on there for specific tasks, and then like, imagine things like the singularity.
Alex Hanna:Yeah.
Emily M. Bender:It's. Yeah.
Alex Hanna:Yeah. Just incredible category error.
Emily M. Bender:Okay. Next. Um, you want to read that one headline?
Alex Hanna:Yeah. So this is from the BBC. The, uh, the author is David Robson, December 23rd, 2024."An AI started, quote, 'tasting colors and shapes.' That is more human than you might think." And what, what is this actually about, the brain? So, "The brain often blurs the senses, a fact that marketers often use in the design of food packaging and AIs--" Infuriating linguistic thing, uh, um, of using AIs as a, like a, um--
Emily M. Bender:Common noun? Not even a common noun, but a, um, uh, count noun.
Alex Hanna:Count noun. Yeah. Yeah."--AIs appear to do the same. What is the flavor of a pink sphere? And what is the sound of a Sauvignon Blanc?" Um, and then, yeah. So anyways, I don't know.
Emily M. Bender:Right. So, so basically I think what's going on here is that the large language model will spit out answers to those questions because it's doing next token prediction and someone got excited.
Alex Hanna:Yeah. Yeah. Okay.
Emily M. Bender:All right. So speaking of who asked for this, this is something DHGate.Com. This was contributed by, by the way, through our form where people can send us Fresh AI Hell. And only one of these things that I pulled up had a person's name associated with it, like the person who contributed it. And that name was Warai Otoko. Which is, looks like someone's trying to write 'funny guy' in Japanese. So I don't think it's really a name. If it is, I'm sorry, but that doesn't look like a Japanese last name in the least. Um, anyway, so, um, this came in through that form and we will post a link to the form in the show notes. Um, but DHGate.Com, "AI smart display stainless steel kitchen faucet with LED temperature display." What? You just need to turn the water on.
Alex Hanna:Just turn, turn the water on and use the switch.
Emily M. Bender:Yeah.
Alex Hanna:And wait, wait, like, and it's doing your prediction. Like, what if I'm doing the dishes with like really hot and then, and then I want to get a glass of water, anyways.
Emily M. Bender:I can't tell what the AI is supposed to be. Anyway, I'm going to take us to this one next, because I want it to be your turn and you get to talk about this one.
Alex Hanna:Yeah. Yeah. So this one is. I posted this on, on BlueSky, but this is a, this is a pull request for something called WL, uh, WSL, and this is a config file. And so basically someone said they were rewriting the lead for clarity. Um, this, it's a, it's a GitHub pull request. Uh, those of you don't know what GitHub is, uh, for open source projects, if you want to contribute something, a change, you do a pull request, and then a maintainer decides that they want to merge it or not into the main code base. So this person re rewrites it, blah, blah, gives this, okay, cool, doesn't look very complicated, scroll down a little bit. They go down and then, the maintainer, Matt, uh, Wojo, says, "Thanks for the contribution here and appreciate your attention to detail. We have decided to keep it as is. Part of that decision is that more and more folks are using AI chat to access guidance, and tables don't always translate well in that context." And this has 906 thumbs downs, 58 laughing, and then 44 just like sad faces. Um, and so people are, developers, these are software developers, they're like, what the hell is wrong with you? These are terrible. Like, um, the next user in the chain, uh, Peter K Murphy says, "Would you and your team be kind enough to revisit your decision to accept the commit? As a WSL user, I found the documentation easy to understand and very helpful. As a developer, I would never trust an LLM to understand text for me. The professional thing to do would be to always read it myself." Uh, 400 hearts to that. Uh, next person, Scott.Web, "This is hands down the worst response to a documentation patch I've ever seen." Um, and then, uh, someone else, "Yep. Microsoft are losing their fucking minds again. A normal day in 2024." Um, next person, "More and more folks are using AI chat because it's being forced down their throats without expressing a desire for it. Less accessible documentation is not the way." And then, um, scroll down. There's a great comment, which is basically like, um, let's see, this, this one's great. Someone wrote, "PR bad cause AI can't read. Sad face." Uh, and then there's, there's been some good things here. Like basically, like I've always taught that, like. Oh yeah, here, I like this reply from Mike Taylor who says, um,"After decades of being told, quite rightly, you should write your code first to be comprehensible to humans, and only secondarily for computers. Now we have Microsoft telling us our freaking documentation is supposed to be written for computers instead of humans. Oh dear, poor dear." Um, and then a quote from somebody named Martin Fowler, who says,"Any fool can write code that the computer can understand. Good programmers write code that humans can understand. Anyways, the long and short of it is that they, uh, griped enough and they reversed the change.
Emily M. Bender:Right. After, after whining about it.
Alex Hanna:Yeah. After it was, it was like a day and like hundreds of comments.
Emily M. Bender:Yeah. And then this, this, this maintainer says, "Okay, fine. Everyone seems upset about the AI comment. Rest assured humans are my number one priority for these docs, y'all."
Alex Hanna:Yeah. My God. Yeah. Someone in the chat, uh, John Near Seattle goes, "Ratioed on GitHub." Yeah, uh, and, and Steal Case uh, helpfully says, "WSL is Windows subs, uh, subsystem for Linux. It's basically how you can run a Linux terminal locally on Windows, uh, and many developers use it." Thank you. I've, I've used Cygwin to do that in the past, but I didn't know Microsoft maintained a tool like that. Okay.
Emily M. Bender:All right. So taking us over to Seattle Public Schools. Um, and this is near and dear to my heart. I went to Seattle Public Schools. My kids went to Seattle Public Schools. I want them to be doing better than this. Um, and here is their documentation on AI tools. And this is linked from another page where they're talking about like their principles for AI use. And like reading that, it's like, okay, they're a school district, they have to deal with this somehow, they have to come up with a policy, and the way they were putting it together, it was, you know, grounded in their principles, and like, that was all well and good, but it's done without an actual understanding of how the systems work, and so it falls apart. And in particular, um, I wanted to look at these FAQs here. Right, um, so, um, "What is generative AI and how can it be used? Generative AI is a type of artificial intelligence that can produce various types of content, including text, images, and audio. ChatGPT is an example of generative AI that creates content based on receiving prompt, a prompt from someone using the program." And some, somewhere they talk about it being transformative. Yes."When and why did SPS unblock ChatGPT access for staff and students?" First answer, "SPS has never blocked ChatGPT on staff devices. Fall 2023, SPS opened ChatGPT on SPS student devices. This decision ensures our students have access to this transformative technology." And it's like, no, like you could have resisted and I'm sad and I'm, you know, I'm right here. I would have volunteered my time to help you understand it. If anyone from SPS is listening, um, I'm here and I care. Okay. Uh, next we can do these last couple ones really quickly.
Alex Hanna:Yeah. So this is TechCrunch and this is, um, written by, Charles Rollet, from December 16th. Uh, "Cohere is quietly working with Palantir to deploy its AI models." Um, it's what the tin says. Big companies are working with, uh, Palantir and Anduril and every Lord of the Rings, um, name that has been twisted to be a stupid product.
Emily M. Bender:And if you thought Cohere was somehow better, cause it was a research lab. Um, no, of course not.
Alex Hanna:Well, Cohere is not, Cohere is like Hugging Face where they have a business side of the house and then a product side of the house. So Cohere for AI, that Sara Hooker directs, is not. That said, it doesn't make it any better.
Emily M. Bender:No. Okay. Uh, next, "Elsevier rewrites academic papers with AI, without telling editors or authors." And this is by Amy N. David on January 5th of 2025, um, under, on a site called PivotToAI.com. Um, and so apparently, um, a journal published by Elsevier, um, so Journal of Human Evolution, and Elsevier went in and edited things with some AI editor and, um, didn't tell the authors or the editors that this was happening.
Alex Hanna:Yeah, well, then this also resulted in the editors, the editor, a bunch of editors assigned from the board, the editorial board of this, just absolutely nightmare.
Emily M. Bender:Okay. And then, as sort of a look ahead, you want to do the honors on this one, Alex?
Alex Hanna:Yeah, so we're previewing an episode for next week, uh, where we look at the UK and their turn to AI. Um, so if you thought the Labor government was going to be any better, you would be wrong. Uh, the, the, uh, headline, "'Mainlined into the UK's veins,'" which is an actual quote from, um, I believe a minister."Labor announces huge public rollout of AI, plans to make the UK the world leader in AI sector, including opening access to NHS and other public data."
Emily M. Bender:And I just, I want to sit for a moment with that metaphor there, like mainline, so they're basically saying this is a drug and it's like, what, what is the entity that is going to get the high from this drug? It's their stock index, right?
Alex Hanna:Yeah. Well, yeah. Well, no, it's, it's, it's the UK public. Don't you know?
Emily M. Bender:Yeah. Right. Of course.
Alex Hanna:Yeah. Uh, well that's it. Join us next week. We'll have a very special guest, uh, joining us to talk about that. And we'll, we won't tell you who until later. That's it for this week. Our theme song is by Toby Menon. Graphic design by Naomi Pleasure-Park. Production by Christie Taylor. And thanks, as always, to the Distributed AI Research Institute. If you like this show, you can support us in so many ways. Rate and review us on Apple Podcasts and Spotify. Pre order The AI Con at TheCon.AI or wherever you get your books. Subscribe to the Mystery AI Hype Theater newsletter at ButtonDown or donate to DAIR at DAIR-Institute.Org. That's D A I R hyphen Institute dot O R G.
Emily M. Bender:Find all our past episodes on PeerTube and wherever you get your podcasts. You can watch and comment on the show while it's happening live on our Twitch stream. That's Twitch.TV/DAIR_Institute. Again, that's D A I R underscore Institute. I'm Emily M. Bender.
Alex Hanna:And I'm Alex Hanna. Stay out of AI Hell, y'all.