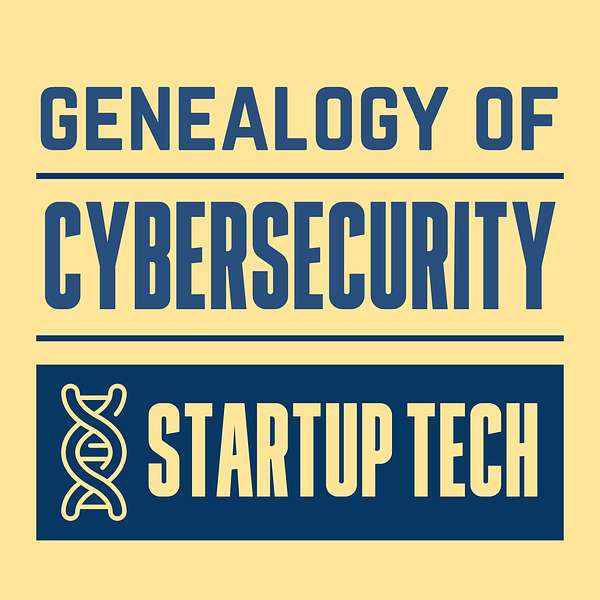
Genealogy of Cybersecurity - Startup Podcast
Interviews with founders, startup-advising CISOs, venture capitalists, and analysts discussing the issues of cybersecurity, new threats, and emerging technology. The Genealogy of Cyber Security brings listeners into forward-thinking conversations with industry visionaries, to explore big ideas, and discuss out-innovating the competition.
Genealogy of Cybersecurity - Startup Podcast
Ep 9. Concentric AI on NLP, ChatGPT, and Data Security Posture Management
Concentric AI Founder Karthik Krishnan discusses the new Data Security Posture Management market and answers the age-old questions of what data you have, where is it, and who’s accessing it. Karthik discusses advances in AI, natural language processing (NLP), Open AI ChatGPT, Large Language Models (LLMs), and what it all means to data classification and society.
Karthik Krishnan explains the incredible expenses and human power required to classify and govern data, and how Concentric AI’s DSPM product reduces costs. Paul and Karthik discuss why the cloud native and AI DSPM products differ from data security products of the past, and Paul grills Karthik to see if there are any hidden costs in the cloud.
Concentric AI can be found at Concentric.ai, on LinkedIn.com/company/concentricinc, or Twitter @IncConcentric.
Concentric Founder Karthik Krishnan can be found on LinkedIn.com/in/kkrishnan/.
Send feedback to host Paul Shomo on Twitter @ShomoBits or connect on LinkedIn.com/in/paulshomo.
I met the ciso financial services company. He was asking me, is there any off the cuff remark? He was like, hey, do you guys do anything in data security? Because we're struggling with understanding where all our data is. We're worried that it's in locations that it's not supposed to be in. And more specifically, he was like, look, if I were to have an SSCR audit tomorrow, I'm likely likely to get fined, and I'm fairly terrified of the prospect. And when I took that feedback to a broader set of security professionals, that just became a consistent theme where we're struggling to understand where our data is, and if we don't know what we have, how can we monitor it much less protected? A surprising finding for us was the existing approaches that customers had were so inefficient, often they were spending three to $5 on people for every dollar in tool. And which if you understand the security industry, there's just a dearth of qualified security professionals in this industry. So it was just an unscalable approach that was only getting worse over time. There was not a single customer we met. There was actually happy with our data security program. Everybody was just underwhelmed by the results that we were getting. And also knew that the problem was only getting worse over time. And then we applied, we looked at language models, we looked at deep learning to see if there is an applicability and when we found it, very quickly for us, it became clear, look, this is a foundational problem for enterprises. I mean, this is a, you know, this is the data security layer, which is, in my view, one of the four key pillars within enterprise. The genealogy of cybersecurity is a new kind of podcast. Here we'll interview notable entrepreneurs, startup advising cisos, venture capitalists, and more. Our topic, the problems of cybersecurity, new attack surfaces, and innovation across the startup world. Welcome. I'm your cybersecurity analyst, Paul shomo. Last year, a new category called data security posture management appeared in Gartner's hype cycle report. And within a few months or a few months around that time at least, a number of strategic investments were made in a large handful of DSPM startups. Data security isn't necessarily as sexy as threat detection as far as press coverage, but data security is fundamental to everything we do in cybersecurity. So I wanted to bring on concentric AI, which is one of the DSPM startups. Yeah, my name is Karthik Christian. I'm the founder and CEO of concentric AI. At concentric AI, we use deep learning and specifically language models to help enterprises discover monitor and protect all of their mission critical business sensitive data. My background in AI have been in the security space for 20 plus years. This is actually the second version of an AI company that I've been associated with. Prior to this, I was part of a team that had built a big data analytics platform for doing anomaly detection on users and devices. The company was called Yara. We built a platform for doing behavioral analytics, looking at users and devices, profiling their activity across the network, using both traffic as well as logs to figure out where their outliers and anomalies as an advanced intrusion detection anomaly detection company. That was my first exposure to bringing AI to the world of security. And in the second version now, with concentric AI, we're doing that. I'll be not at the infrastructure layer, but at the data layer. What are your thoughts on chat GPT? A lot of people have strong takes. Yeah, so you know a language model in its essence essence can actually assess what the probabilities of any word to follow like a given sequence of words, right? So a simple analogy is if you look at online services like Amazon or Netflix, they can analyze the user's sequence of actions, and based on the activities of millions of other users, they can recommend the next likely action. Language models themselves are not new examples include you can go back and for a long time, you can go back to things like Markov models and a hidden Markov models and so on. And the only difference is that the newer models like LLMs or large language models, they're essentially deep learning models with a large number of parameters. Think hundreds of billions as opposed to say tens or a few hundreds of parameters in traditional ML based language models. And that's what obviously makes them that much more powerful. GPT itself, I know people think of it as sort of an autocomplete engine. Chat GPT in itself is more than autocomplete. The engine underneath that is GPT. GPT is very much a large language model that can be thought of as sort of a super powered version of autocomplete. Chat GPT is in some ways a specific realization of capability. This capability, vehicle built. To demonstrate the power of the underlying technology and charge GPT itself is built using reinforcement learning, a machine learning technique where you can use rewards and penalties to help the system learn desirable behavior on top of large language models using human feedback. So that's really what it is in sort of its essence. A model that obviously can work through a lot of parameters compared to what was traditionally possible. And maintain a conversational style format without losing context. How impactful will it be in your area, you think? You know, we're exploring with it. It's a little early days for us. To look at it, as I said, we've been using LMs. The underlying GPT technology is what is very, you know, we were in talking about it before because people understood semantic context people didn't understand what language models are. Now, increasingly, we're realizing that. So in many ways, what we do is LLMs and it's hard because what we're doing is we're looking at the sequence of words, to figure out structural associations and using that to develop a semantic context. Chat GPT itself is something that we're looking into to kind of see what the applicability is. Again, I'll go back to what I just said. The use cases have to fit the problem, not the other way around. And so we're playing with it, trying to see where it may be applicable. Well, one of the things that's interesting to me is people, people are throwing around the word meaning a little too much, I think. They say chat GPT understands meaning. I can ask it, go create an essay to tell me what kind of breed of dog to buy, right? But it doesn't really understand. Meaning there's a lot of gradations of that. It doesn't really understand what a dog really is. It's never seen a dog. An event computer vision, it maybe could see it, but it doesn't understand the difference between eating a dog, a human and a dog because it's not conscious. It doesn't have a real personality, so it doesn't understand the personality of a dog. It doesn't know what it's like to cuddle with a dog on the sofa and feel the emotion, right? So meaning it's a very light level of meaning, but to pivot into data classification, which I know you have a background in is a lot of times in security when we're trying to map out where our sense of data is. We only need a light level of meaning, right? It's like, I don't need to understand the engineering document. I just need to understand it is an engineering document. It's IP, right? This type of AI that the large language models that maybe were overselling for replacing humans is actually really useful in your area. Can you speak about that a little bit in the history of data classification and how this all fits? Yeah, absolutely. So if you look at the world that I live in, which is helping enterprises protect their data, let's talk about what that really looks like for enterprise. If you look at an enterprise, data that they care about is everything from financial data to intellectual property, to business confidential data operational data, like contracts. And many documents. And term sheets and income statements and so on. And even PII, PCI, PHI, in a privacy related data. Now, the challenge with struggling, the challenge with securing this in the past has been any sort of a security program requires you to first of all know what it is you want to protect, which means you have to have a good idea from a discovery standpoint. And the traditional ways of doing this has relied on patterns. And what's a pattern? You're looking for a word. You're looking for the word confidential. Are you looking for the word secret? Are you looking for a specific project name? Now, when you're dealing with the 80% of our customers data is unstructured, which means it's not sitting in a database, it's mostly sitting across your Office 365 SharePoint repository or box or Dropbox. And the challenge with a lot of unstructured data is unstructured data follows natural language syntax, which means you've got all the problems. There's a problem in the natural language called polysemy, which means the same word, depending on the context can mean very, very different things. For example, if you look at the word architecture, it can refer to a building construction document, much the same way I can refer to a software design document. And so when you're looking for a pattern, all you're doing is you're looking for a word without the context for how that actually word is represented within, for example, a file. And so previous generations of technologies which are relied on words and regexes and pattern matching have failed, they lack the context necessary to understand the true meaning of what they're looking for, which means you're going to get a whole bunch of false positives associated with I'm going to look for this word. And it may not mean anything. And in many cases, you may be looking for documents that may not contain that word, but maybe very much what you're looking for. And so what we do in application of natural large language models is essentially looking at the sequences of words, looking at the document itself, to understand its broader import and meaning. And that then allows you to develop a semantic understanding of the data to give customers a thematic view into all that information. So I can tell you, here are all your contracts. Here are your wire transfer documents. Here are your intellectual property. And that's exactly back your point. In this specific use case, LLMs have LLMs work, and they work extremely well. Once you've understood the data really, really well with high degree of accuracy, you can then monitor it. You can go in and identify risk. You can help, for example, find out where data may be inappropriately shared. If you're inside the company or outside the company, I don't have to write a rule to define what my wire transfer documents look like because concentric you have done the work for me to find out where all of my wire transfer documents are, all I have to do is I've got to go in and classify all of these things as being confidential documents to make sure that they don't end up in the wrong hands. So that's how LLMs fit within this world. And helping prevent data loss. Associated with risks that may get presented to an enterprise sensitive data. Software vendors or providers sound so different these days. With the new era of AI, it really sounds more like to use a crude analogy. There's this giant brain in their basement. They're just shoveling data into it. And their experts are around it, training it supervising it, reinforcing its learning. And that sounds very different than the past generation of software vendors where they were talking about adding lines of code, adding feature sets. So you mentioned a few things you know, large language models and deep learning. What are you doing to build that core intelligence? So we have what's called concentric mind, which is a reinforcement learning engine that we have that continues to learn as we see in newer categories and thematic data types. And over time, we have built up a virtual network effect where every customer benefits from the learnings that we do across customers. And absolutely. So you're building deep learning, what did you call the semantic mind? The product is called concentric semantic intelligence. Concentric mind is the engine that powers semantic intelligence that is this virtuous learning engine, but you can think of as what basically, if you see data types, mind is what informs a specific tenant. Okay, this is a tax document, or this is a wire transfer document, or this is intellectual property. And that is actually continuously learning. As we see newer data types, as we see new datasets, it's continuing to learn. And so the brain is growing over time. But you mentioned thematic types like, what are some of the themes thematic types that you concentric mind to understands and has come to learn? Yeah, we have 500 data models today supporting 250 thematic categories of data. So we can usually go into a customer environment at a highest level it'd be things like HR finance and product data. But underneath it, it could be everything from sheets to M&A documents to NDAs to something as Monday's resumes to intellectual property to