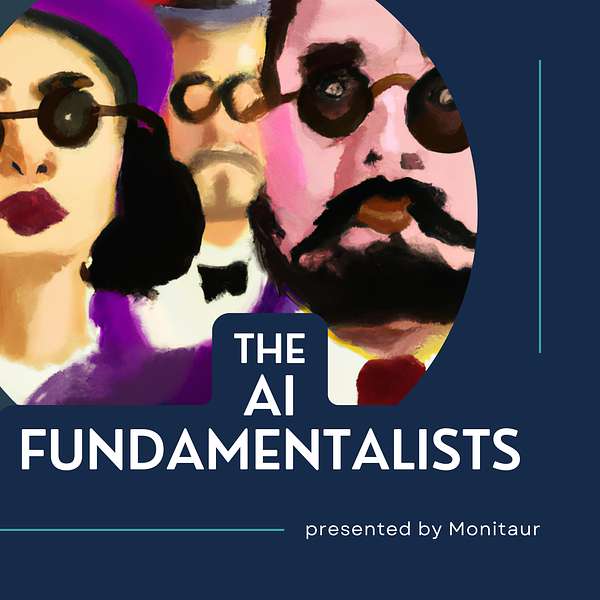
The AI Fundamentalists
A podcast about the fundamentals of safe and resilient modeling systems behind the AI that impacts our lives and our businesses.
The AI Fundamentalists
Non-parametric statistics
Get ready for 2024 and a brand new episode! We discuss non-parametric statistics in data analysis and AI modeling. Learn more about applications in user research methods, as well as the importance of key assumptions in statistics and data modeling that must not be overlooked,
After you listen to the episode, be sure to check out the supplement material in Exploring non-parametric statistics.
Welcome to 2024 (0:03)
- AI, privacy, and marketing in the tech industry
- OpenAI's GPT store launch. (The Verge)
- Google's changes to third-party cookies. (Gizmodo)
Non-parametric statistics and its applications (6:49)
- A solution for modeling in environments where data knowledge is limited.
- Contrast non-parametric statistics with parametric statistics, plus their respective strengths and weaknesses.
Assumptions in statistics and data modeling (9:48)
- The importance of understanding statistics in data science, particularly in modeling and machine learning. (Probability distributions, Wikipedia)
- Discussion about a common assumption of representing data with a normal distribution; oversimplifies complex real-world phenomena.
- The importance of understanding data assumptions when using statistical models
Statistical distributions and their importance in data analysis (15:08)
- Discuss the importance of subject matter experts in evaluating data distributions, as assumptions about data shape can lead to missed power and incorrect modeling.
- Examples of different distributions used in various situations, such as Poisson for wait times and counts, and discrete distributions like uniform and Gaussian normal for continuous events.
- Consider the complexity of selecting the appropriate distribution for statistical analysis; understand the specific distribution and its properties.
Non-parametric statistics and its applications in data analysis (19:31)
- Non-parametric statistics are more robust to outliers and can generalize across different datasets without requiring domain expertise or data massaging.
- Methods rely on rank ordering and have less statistical power compared to parametric methods, but are more flexible and can handle complex data sets better.
- Discussion about the usefulness and limitations, which require more data to detect meaningful changes compared to parametric tests.
Non-parametric tests for comparing data sets (24:15)
- Non-parametric tests, including the K-S test and chi-square test, which can compare two sets of data without assuming a specific distribution.
- Can also be used for machine learning, classification, and regression tasks, even when the underlying dat
What did you think? Let us know.
Do you have a question or a discussion topic for the AI Fundamentalists? Connect with them to comment on your favorite topics:
- LinkedIn - Episode summaries, shares of cited articles, and more.
- YouTube - Was it something that we said? Good. Share your favorite quotes.
- Visit our page - see past episodes and submit your feedback! It continues to inspire future episodes.
The AI fundamentalists, a podcast about the fundamentals of safe and resilient modeling systems behind the AI that impacts our lives and our businesses. Here are your hosts, Andrew Clark. And Sid Mangala manglik. Welcome to our first episode of the AI fundamentalists for 2024. We hope your year is off to a good start. And just in our regular style, we're gonna jump right into a deep and meaty topic about nonparametric statistics. But before we do, of course, while everyone was on holiday, we came back to some news. In particular, open AI has made it clear that they do plan to open their GPT store next week. You know, what should you know what should modeling and IT teams? Keep in mind before running wild with this? I know you Andrew, we've talked about this a lot. And I'm sure you have some thoughts. Oh, more worthless
Andrew Clark:little widgets? What could go wrong you now have I mean, it makes sense. So basically, they're trying to make an app store or like any, like Android or iOS or anything. They're just trying to build a platform. I mean, the VC firms are probably in there, you're trying to have a more platform centric, so it makes sense from a business perspective. I mean, one of their examples even was like a millennial meme interpreter or something. So it's like sounds like it is kind of trivial nonsense. So we know like real company is going to use this for anything materials. So I mean, sure, if you want a millennial meme interpreter, it's probably a great, great thing. And if they can make a little bit of money off of it, and he said that developers can make money too. They're just trying to make her their own app store. So they're not anything inherently wrong with it, I just find it amusing.
Susan Peich:It does say that it's going to be in the enterprise version, or G and GPT. Plus, which limits it limits its availability to, you know, hopefully the right audience. But still, if you don't have governance in place, or you don't have like a really good AI infrastructure in place, like what should we be considering that anything you bring for this marketplace?
Andrew Clark:Well, this, this will just make it make it worse, because I am not sure the details were very spotty, at least what I was able to read, maybe there's more out there. Now. I'm presuming it's built off of GPT. Four, and those things that sounds like very, they're optimized applications, or they're fine tuned for specific use cases, where you basically said, like open AI, as we've talked about, we're not really sure about how well they do model governance and all those different aspects, it doesn't seem like there's a lot there. But now they're saying, okay, cool. Now anybody can start adding applications for enterprises that probably have even less governance on top of the fine tuning or whatever the they're doing. So it just needs to have some some notion of quality. It's really no different than like iOS or anything, but I think that's maybe the is a different set, it's for enterprises, and they're building these applications on top of it, that probably aren't gonna be as stress tested. And I mean, I'm, I'm wary about what I even download from iOS. And I know they're scanning and things like that in place. Because, you know, like, some calculator apps are probably just mining your phone for information and things like that. So you have to be very careful with that. So be interesting to see what type of controls if I mean, an opening eye style, they're probably not going to say anything about it. But are they going to be scanning for malicious? Potential? Or how are they evaluating? Are they just accepting anything under the store. So there's just very, not really many details out yet. But there are some pitfalls that you want to be aware of.
Susan Peich:Over the past few months, they, if you are assigned a new user of GPT, or GPT, three or GPT, four, there have been a lot of emails going around with terms and condition changes and things like that. So I think as far as like it from an AI, the fundamentals of AI perspective, just make sure you're reading your agreements, and anything that you go into before you're downloading apps from the store, and know how you're using them.
Sid Mangalik:Yeah, for sure. I mean, in this is kind of response to people really wanting to fine tune the GPT four model, but no one having access to it. So it's like, well, maybe the store is going to be my way to kind of sneak in there and get my fine tuned model. I'm not sure that's, that's what you're gonna get out of this. And like Susan saying, read your terms and conditions, because you might be sending over your data if you're not careful. I know at the enterprise suite, they're trying to be careful about not ingesting your data. But you want to make sure that as part of the monetization scheme, you're not handing over your data.
Susan Peich:Absolutely. In another breadth of privacy. This hits my world, especially Google began their slow slow roll into deprecating their third party cookies. So in the name of the 1%. Now it's the 1% of Chrome users. As of this month have started. Google has started disabling third party cookies, and hat tip to Gizmodo, they did have something In their article about how you can tell if you are one of those Chrome users that for which third party cookies have been turned off, we will put that link in the show notes. Yeah. For me poor marketers. But I think like we've said on previous podcasts, there, you know, there is something good about what Google is doing, even if it's in a slow roll with privacy. And what are you guys thoughts on that?
Andrew Clark:Yeah, I'm really happy to overall see that Silicon Valley seems to be moving a little bit more into privacy centric. Apple has been doing it for a while trying to make better security. And I think it's pretty much because there's been so many exploitations and things like that that have happened. I know some people aren't, aren't too happy. And marketers will be sad about some of these things. But I think like what cookies have been, has gotten pretty intense amount of cookies tracking and things like that. So I think it seems like Google is starting to move back a little bit more to the good do, or what does it do? No evil used to be their slogan that they then they dropped. I mean, for the consumers cookies aren't great for marketers and businesses, they're great. But for consumers are not, they're not fantastic. So I don't know, I don't have, I'm not a marketer. So I don't have a really strong opinion. But definitely I like to see companies that are trying to do better for the, for the end users versus just like, for companies that will pay them money for ads or whatever. So I do like to see that.
Susan Peich:Yeah, I mean, as far as Franek, as my, as the practice of marketing is gonna get I, I agree with you to it, it really is, in many ways, even with AI we've talked about it is much better to err on the side of like, what does the consumer actually need and want, and more likely to use? And I think this is a step in the right direction. Despite the headaches, it'll cause for me.
Sid Mangalik:Yeah, and I, and I'm glad, like you have this optimism about this, I guess I'm a little bit more cynical, that's like, it feels like it's like maybe just getting right ahead of the right, like the regulations around this, I think the writing is kind on the wall that like the government has turned their eyes towards the big tech companies. And if there's very obvious and surface ways that they can kind of like attack this and get what they want. It's there's a lot of incentive for these companies to be like, let's just do it two months ahead and get the good optics, because you're going to be asked to do something like this. Anyways. I think we saw that a little bit with like, the, you know, the USPC ification, of of iPhone, the addition of RCS and iPhone, the regulations are here, and they're coming. And so it's better if these companies can be on top of it, and do it the way that they want ahead of time. Oh,
Andrew Clark:I didn't think about that. It's, uh, yeah, maybe there is something going on behind the scenes. We haven't that's not public yet. That's very interesting.
Susan Peich:So with that, that being said, we, as we dig into today's topic, you know, while it may not be headline worthy, or you might be, or in the mainstream news, you know, nonparametric statistics, you know, has, it's that micro impact within the business within your modeling teams, that in the spirit of the right model for the right job. We felt this is worthy of taking a deep dive into this topic, because there's some things that your, especially your modeling teams are going to learn from this. And you know, among those who listen to this podcast,
Sid Mangalik:so let's hop in here. So we're not here to, you know, make this episode and say, you know, you're switching your whole company over to nonparametric statistics, this one that you're going to use, forget everything else use this the new hotness, no, that's not what this is going to be about. This is going to be about understanding what these types of statistics can do for us. And where they can be better than the standard statistics that you probably already know. And probably already using your machine learning and AI pipelines or your statistical learning and modeling. So Android, I work professionally with a lot of other data science, fraternity teams and firms. And they bring their own data, right, and we're not on the ground with them, we don't have the subject matter experts. And so we don't know everything about their data. And so we need to build systems that are generalizable and as close to assumption free as we can get. And so as we'll kind of get through here, nonparametric statistics are a really great fit for when you need to do modeling in environments where you don't know everything about the data, or you can't know everything about the data. So specifically, you know about the shape of the data. And we'll we'll dig into that a little bit. But you want to think about this as a really good system for generalizable modeling and assumption free modeling. And I'll let Andrew hop in and walk us through a little bit of what parametric statistics that you know and love is good for and what its pitfalls are.
Andrew Clark:Definitely great, great interested. Yeah. Most people don't even really work with statistics and a lot of data scientists. It's kind of the cursory thing you don't really learn often for data scientists. It's, it's you know, set As we said, on this podcast before, we're trying to bring stats back, we're trying to do things that fundamentalists approach from first principles. And you really can't do anything in modeling without understanding statistics. It's really the language of modeling. And oftentimes we see it as kind of like a, just a backburner topic versus something that should really be the forefront. And there's a lot of assumptions that are built in when you're building these systems and a lot of different modeling paradigms depending on you know, if you're coming from systems engineering, if you're coming from, from statistics, econometrics, or data science, or where whatever background you have, they're gonna look at the world a little differently. And the, the modeling paradigms and algorithms they use will be different. But one thing that pretty much underlies most of those is statistics. But there's a lot of in like econometrics has specific statistics, they like to use statistics as a discipline is specific to statistics. And machine learning often pretend they don't use statistics. But they do. They all have these different different approaches and different paradigms. So it's good to look at the let's just look at the statistics at their baseline so we can understand what the pros and cons are, and and know what the built in assumptions are. So parametric statistics is what most people are familiar with is what you've probably learned in high school statistics, maybe college, you know, linear regression, things like this, where you learn about distributions, and normally you have that Gaussian normal distribution, the bell curve everybody talks about, and a lot of statistics are built off of this normal distribution. And it's just very convenient. And all the math just works great. And everything same with I'm an economist by trade, and a lot of my background, everything talks about equilibrium, you always go to the equilibrium. Well, equilibrium doesn't exist really in the real world. They're not a representative agents for you know, America, you can you can model as a bunch of representative agents that all think the same way and the rational No, but there's all simplifications that people do seamless simplifying that you can represent all data with a normal distribution, and that's what was said was alluding to earlier is, a lot of people just just will default to that, where it's very convenient, it has a mean of zero, and as a standard deviation of one, they're not skewed, the tails aren't thick, it's perfect. And then a lot of statistics are based off of this normal mean based, you know, equilibrium, if you will, distribution. And that's all well and good unless your data doesn't look that way. And that's the problem.
Sid Mangalik:Yeah, and I want to emphasize here, that's like, it's not a totally misguided assumption, right? Because then we look at the real world. And we try and see where do these normal distributions occur? We do find them, we find them in retirement ages, you know, how tall is the average person SATs scores, this neural distribution is out there. The problem is, and we assume that it's everywhere, and that it's everything, right. So it's, it's well founded to use the normal models, but we forget that it's not the case for everything. And that's, that's the, that's the ketchup that we're getting.
Andrew Clark:Excellent, the great, great point. And there's a whole branch of statistics is essentially checking for those assumptions. So there are a bunch of statistical tests that can help you determine if your data fits that. And if your data does not fit the normal distribution, it doesn't mean game over you have to use a different distribution. There's also transformations, such as logarithmic transformations, if your data is a little skewed, meaning that maybe instead of being that perfectly normal distribution, the curve is a little like off kilter, essentially, where it's, it doesn't look perfectly like a bell, it's just kind of like really bunched up in one area or not. So you can use like a logarithmic transformation to then dependent depending on your base will then make the data look normal. But then you got to realize that you have to convert to the log, do what you're going to do, and convert back, and you have all these sorts of things. So there's all these branches, statistics that didn't have specific tests to test for all of these assumptions. And those things. And as I said, mentioned, like SAP scores is normally the bell curve. There are other distributions out there, like people want to talk about normal, but there's alpha, beta, gamma, lots of random. Now, there's so many different statistical distributions. And you can check to see if your data fits those different distributions. And then use those and understand the different assumptions with all those. But you have to know that your data fits those things. Because if your data is very skewed, or does not look like a normal distribution, but you're using normal distribution tests, you're you're you will be wrong. So this is what like what we talked about, in our bias conversations with Josh, is there statistical error that can get in there as well. So if you're just applying, you're saying normal distribution for everything. There's a lot of error and bad results you could have. And it's purely because you didn't use the wrong underlying statistics. So when we're starting to talk about modeling and modeling phenomenon, things, there's a lot of error there. And if you're just blindly using, I'm waiting for my podcast where I can go on my rant about auto ml, but if you're just randomly assigning just see what what do we think works best, you know, there's overfitting all these other issues, knowing what these underlying Buying assumptions are key. So before you look at any, that's why understanding the data as well, as we, as we've talked about, said, anything that to add on that.
Sid Mangalik:Yeah, no, I think that I think that's really great. And I'll let you spend some time talking about like some of the specific other distributions out there. But this is where the subject matter experts are important, right? If you just have a sample of data, and you're like, Wow, this looks normal to me, you like you have 30 pieces of data, and they fit the normal bell curve, and then you go ahead with it. And then you find out a month later, oh, my God, this data has an enormously long tail. There's some crazy outliers in here, because we didn't look at enough data. So when you come in with assumptions about, oh, well, the data is going to look like this space in our small sample, you're missing some of the power that the model is supposed to give you. Right, by not having that right shape. And by not having that expert to say like, we really expect it to look like this. So I'll let Andrew happened to some of the specific models, but just know that like, don't use it, because it looks right, you have to really evaluate Is this what we expect?
Andrew Clark:Excellent, great point. And this is where there's a lot of studied areas is as citizen, for we gave some good examples of where normal normally works, there is kind of experts no specific things can often be represented by different distributions. Because once you have a distribution is that generalized shape, you can shift the mean up or down. As we said, normal is normally a mean of zero, you can still you can shift that shape can be used with a different mean. And there's these variations of the Gaussian normal distribution you can use. For instance, the Poisson distribution is often used for like, doing wait times and counts and things like that. And people know how to model that. That is a discrete distribution. Another important difference. So discrete means it's like discrete events in time. Uniform distribution is another one where you have the same probability of like gaps between events happening. So those are discrete probabilities that represent those discrete events are not continuous versus a Gaussian normal is like a continuous bell curve, you could have an IQ of I don't know the exact IQ numbers, but focused around the, I'm not gonna make something up like I did with a Richter scale. So just gonna say there's specific IQ numbers, but you can have them at any point on that on that distribution. And the Gaussian normal is it's mirrored correctly on both sides. So then you get even really funky when you start talking about like compute cumulative distribution is the probability of where that is on like the left hand side of what's probability of a value being lower or higher than a specific point, all these sorts of things. So you have the continuous and discrete distributions as well. So we don't need to get in, we can do specific podcasts on some of these others as well, exponential, all these different where you'd use more of them. And I'll pause that said, add a little bit more if he wants to hear. But the the general thing to show here is the complexity of you need to really understand what distribution and then the specific statistical test to validate against that distribution. And then what hypothesis testing or what modeling paradigms or whatever else, you want to use generalized linear models, that example against that distribution. So there's a lot of complexity here, you can't just throw auto ml on it and think it's going to do it properly.
Sid Mangalik:Yeah, I mean, I don't have too much to add to that. I think that that's pretty, that's pretty apt. Right? It's, it's knowing the distribution need to use for the time you need to use it. And then doing your hypothesis testing, according to the distribution, you just ran, these tests change with every different distribution type. And so it takes some reading, it takes a fundamentals. And you really have to understand what the data is like and what you expect it to be like. What we're going to talk about for the rest of the podcast is going to be what happens if you can't do that? What happens if you have data that you don't know the shape of it, you can't expect the shape of it or someone else's gave it to you in a CSV? And what can you really do to understand this data now?
Andrew Clark:Fantastic segue to nonparametric. So we've defined what parametric statistics means It means based on a specific distribution, there's hundreds and hundreds of distributions. But you need to know the specifics around there. And you can you can learn what those distributions are. And those will provide you most often, if you can get the distribution write the best results. There's just a lot of excess work, as we've illustrated on using those things and knowing which ones to choose. And yes, you can make automated scripts that choose the best distribution of stuff. But that's a lot of work and a lot of assumptions and things you need to make sure you're on top of. I said mentioned that we can't always do that. So nonparametric statistics essentially means that we do not assume the data is fit to a specific distribution. So we can have specific tests that are more robust to the data looking a little wonky. For instance, you know, we said for a standard normal distribution can't have fat tails, which means that, you know, it's perfectly normal and as the probability goes down, it just goes into where the ends of the distribution are very unlikely. That's not true. As we saw with financial crisis and a lot of the like value at risk models and stuff, that's not the case, there's very fat tails, meaning that you can have extreme events. As an example, Gaussian normal type statistics won't catch those very well, because you're forcing on that assumption. So nonparametric allows you to be more robust to outliers, you can generalize across pretty much any datasets, and does not require as much domain expertise or knowledge or data massaging to be to understand them, what they normally do is they they rely on rank ordering. So essentially, what that means is you're ordering data within like an ordinal order. So one, so you're taking this as greater than that is greater than that even greater than that, of course, there can be times when there you can't really have that ordinal relationship. And that can be an issue. They do have less statistical power power is essentially noticing an effective one is present. And that's where why statisticians like to use the parametric methods because you have a lot of ability if an event is there. For instance, if I'm trying to see for a drug trial, I'm trying to know if is there an effect, if if people take this cancer medication or not, if you did all your your stats correctly, this is why statisticians exist. And there's a lot of as we've shown a lot of complexity there. And you really want to have the best probability of detecting something of exist, how do you design that experiment? How do you test it? How do you look at that power, that's where statisticians really shine and nonparametric doesn't do as good of job checking those up, it's kind of like that rough and ready, it's gonna get tricky, it's gonna get the job done. It's kinda like you're driving like a Ford pickup versus a Ferrari, like, you're gonna have a highly trained Ferrari Gaussian normal distribution that a statistician sets up, but you can't go off road, you hit a bump, and then wheels fall off, figuratively. I guess metaphorically, whatever, anyway, versus nonparametric statistics, you It's you can go off roading with it, you can go four wheeling, you can hit bumps, and it's gonna be fine. That's really that applicability across broad is why we want to use those methods to sit anything to add, they're
Sid Mangalik:gonna that that's, that's great. And I guess what I'm going to add to that, I'm just going to remind the audience here. Remember, when we're doing statistical tests, we're often thinking about, versus the null hypothesis, meaning that there's nothing interesting in this data. What are the chances that what's in this data is interesting. And so when you have that normal distribution, you have this really strong assumption. And you know, people will say things like, Oh, well, you'll need to, you only need like 30 samples to really tell like if this is not expected, but when you're doing these nonparametric measures, but you don't have those really nice underlying assumptions, you're gonna need a lot more data to be able to confidently say that, like, yes, there is a change here. And it's statistically significant. Right? So with that lack of assumption, comes with everything that Andrew is saying, right? It's, that's where you lose a lot of that inherent power. It's because you have to collect a lot of data to say something is meaningfully beyond chance.
Susan Peich:What about statistical testing? With models with nonparametric? Methods? Yes.
Andrew Clark:This is really why we wanted to focus on this topic. We've talked about synthetic data, we've talked about model validations. We've talked about even monitoring a little bit. And in previous podcasts, we haven't really talked about how we do that or why. So as we're saying, if like we're trying to monitor we're trying to validate models, we talked about trying to break them and stress test them. We're trying to figure out where does that Ferrari break down? If we if we, if it looks at a rock, does it freak out? Or like how bumpy can we make the ride and it still works? Were the manufacturers stress testing this thing is what we try and do. So in that use case, we want to have one method that's really robust. And so that's where oftentimes in the in the work that we do in validating models, we use nonparametric statistics. So there's a couple of our favorite methods that we often use. And I'll sit introduce, introduce these methods that are that are very applicable across a wide range of uses. Yes, you could make something that's more optimized for one specific use case. But it's the the benefit reward and what's the goal that you're trying to do if you're trying to break models, or check to see if major things are happening? Nonparametric is definitely the way to go.
Sid Mangalik:Yeah, so So let's top off with like the first. And this is probably one of the most popular nonparametric tests is the the Ks test, right. And so the Ks test is, is really important for anyone that's like comparing two different sets of data and trying to see are these different, has a change occurred. And so, like Andrew saying, this basically works by taking both sets of data a and b, sorting them, and then doing comparison of the sorted versions of them.
Andrew Clark:And what's interesting is what I love about this test, it is actually taking the two distributions. So you make them there are statistical distributions. It doesn't need to know you're looking at alpha and gamma or whatever distributions you're doing. And by the way, this is for continuous districts. missions only. So not Poisson or any of those, it's for continuous distributions. But you can have whatever those are. And you can actually define what those distributions are it but the test doesn't care it is seeing how similar or there are these distributions, is there a difference between them. And it's using those, those estimated cumulative distribution functions that's left half of a curve, to see how different they are. And you can still use p value and an alpha and r. And we can get into that's another podcast of like, what specifically are those tools in your toolbox in this statistical world, but you can still use it as normal hypothesis testing methods to determine but what the beauty of this is, is tells you are the distributions the same or different. But you don't have to know what they are.
Sid Mangalik:Yeah, that's exactly right. And he even got a really nice test statistic out of it, which is between zero and one, which is not a given. So it's a really, really useful test for a lot of cases where you want to compare two distributions. And you don't want to say that you know anything about them, you don't want to assume anything about the shape of the data, really, really great test. The other test that we really like, it's almost like a sister version of it is the chi square test. And that's used for the categorical set of data, right? So if your ks test is really great for your continuous data, your chi square is good for evaluating your coin flips, your dice rolls, right? So if we have one pool of dice rolls in distribution, a and one potential is and B, you want to say Are they different? Let's say this is an unfair dice, we don't know the underlying distribution of the unfair dice, we don't know how like if it really like six is really dislikes fours. And we don't want to know that. So then this test, ends up filling that gap for the categorical data. Likewise, this will also give you our P values we expect, so you get your nice statistical measures you want. The test statistic is not is directly interpretable. But this is this is gives you a lot of the same kind of power. And it's built on statistics, which don't require you to know anything about the underlying data from ARB.
Andrew Clark:And then finally, an example of we don't often use this in our work, but an example of you can use nonparametric algorithms for doing machine learning, classification, and regression as well. There's called K nearest neighbors, I'm a big fan of, you know, k means clustering and things like that, which is a nonparametric, as well. So essentially, what you're doing is in one feature space, using a distance metric, like a Euclidean distance, to check and it doesn't have to be Euclidean. But normally it is, basically you're seeing what's the difference between these these out these these data points. And you're grouping them into into groups. And then you can know in that feature space, what they look like, and then make predictions from there, that doesn't assume what the distribution is either. Because there are certain machine learning models that do that do have those, those assumptions, such as linear decision boundaries, and things like that, you need to be aware of where this doesn't have assumptions, it just as long as the data is on the same, make it into the same planes and things like that. You don't have assumptions, as long as the algorithm can interpret the data.
Susan Peich:And on the three tests, the Ks test, the chi square and the K nearest neighbors, is there any type of consistency that you need to keep either within the test themselves or across what what you find from one test into another?
Sid Mangalik:Yeah, I think that the one there's a little bit of secret sauce here and a little bit of secret sauce is you need to normalize the data before it goes into here, you'll get the best results if you if you really take care to normalize them, right. So in the case of a KS test, that could be something like z score in the data before you throw it in there. In the case of the chi square test, making sure that you have no crazy outliers and in one three compared to the other one, right, if B has like a dice that can roll a seven, and there are eight dice can not roll a seven, don't compare them directly. If you're an K nearest neighbors, make sure that all of your features are scaled, because k nearest neighbors really really struggles if your features are not scaled on this to the same length right, your your zero to one scaling. If you want one thing to zero to one and one zero to 1000, the nearest neighbors method doesn't work because it's built on distance. So with these a little bit, the secret sauce is making sure that everything is scaled appropriately and a little bit flatly to give these tests more of a chance of picking up signal.
Susan Peich:Andrew, anything to add to that
Andrew Clark:you have to be used doesn't mean you don't have to do any data cleansing or anything at all. But just normalizing so that you're talking in the same scales and making sure like there's not miles per hour and kilometers per hour type thing. Making sure you're singing from the same song book is definitely required. But that's a lot less than checking to make sure your data has the correct mean center deviation skew, all of tails all of those things and then doing all those those checks, finding the right tests using things just doing a little bit of data normalization Um, and things like that you have to always do feature engineering, but that's not near as much work. And these methods are the more robust versus the racecars. I was just saying we can we can do a more detailed podcast if people like this one on, you know, what is hypothesis testing. And some of the more We've even talked about some methods like at a higher level, like assuming you know, p value, we can go into more details on those as well. Oh,
Susan Peich:that'd be a great episode for sure. If there's nothing else on testing and wish to humor me with one request, the full pronunciation of chaos test.
Sid Mangalik:Oh, my God, this was my worst nightmare. I don't think this test would happen. I want to say, I want to say it's Kolmogorov. Smirnoff, if I had to, if I had to risk it. I think that's right.
Andrew Clark:I think I think that's good. Yeah, I could have said it.
Susan Peich:But you're telling me in the in the profession, like, pretty much nobody will take that on. It's a Gas test,
Sid Mangalik:no one will take that risk. You call it a KS test. Unless
Andrew Clark:you're from like Russian or Ukrainian or something descent, you're just calling a KS
Sid Mangalik:look foolish. It's not enough to put one last name on a test. But to put two last names on a test is asking for trouble. But
Susan Peich:we'd like to get things right here, all the way down to the fundamentals. So I had to I had to ask,
Unknown:Well done said
Susan Peich:yes. Good job. Anything else from you guys sit or Andrew?
Sid Mangalik:I'm just going to wrap it up and say, you know, there's a lot more that nonparametric there's a lot more tests out there. There's even more specific for like the types of use case use cases you have, you know, other two outcomes are there are there 20 outcomes is the continuous distribution working in a certain way. Read up, learn about it, and share it share with the world what you learned. I think this is a little bit of an underused tool or a slightly misunderstood tool. And I think that the role of modeling can really benefit from people talking about these types of tests and when to use them, right let's let's not used normal distribution, everything. Let's understand the test. And let's use the right one for the job. On
Susan Peich:behalf of Mason, Andrew, we thank you for joining us today look forward to more topics this year. In particular coming up we have upskilling and also maybe some more about anomaly testing, among others, and as always, drop us a line at the AI for topic