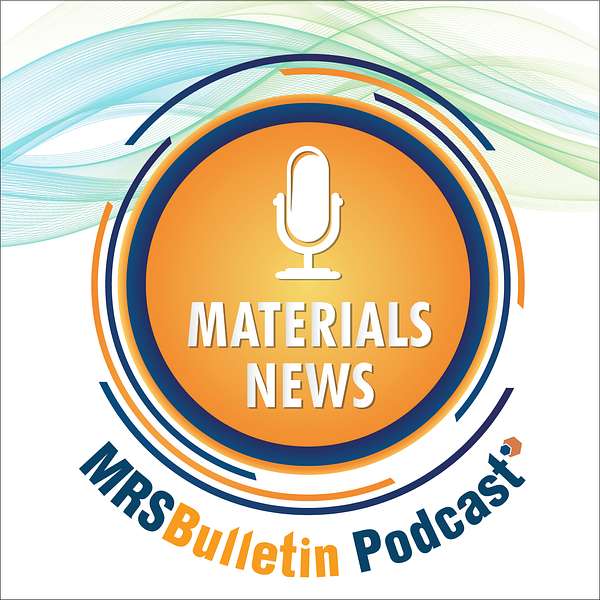
MRS Bulletin Materials News Podcast
MRS Bulletin Materials News Podcast
Episode 20: Resistance of analog deep learning device responds in ~5 nanoseconds
In this podcast episode, MRS Bulletin’s Sophia Chen interviews Murat Onen, a postdoctoral researcher at the Massachusetts Institute of Technology, about analog deep learning that could help lower the cost of training artificial intelligence (AI). The programmable analog device stores information in the same place where the information is processed. The resistor’s main material is tungsten oxide, which can be reversibly doped with protons from an electrolyte material known as phosphosilicate glass, or PSG, layered on top of the tungsten oxide. Palladium is above the PSG layer, which is a reservoir for the protons when they are shuttled out of the tungsten oxide to make it more resistive. “When protons get in, it becomes more conductive. When the protons go out, it becomes less conductive,” says Onen. The resistance of this device responds in about 5 ns. This work was published in a recent issue of Science (doi:10.1126/science.abp8064).
SOPHIA CHEN: Welcome to MRS Bulletin’s Materials News Podcast, providing breakthrough news & interviews with researchers on the hot topics in materials research. My name is Sophia Chen. These days, artificial intelligence systems known as neural networks, or deep learning networks, have demonstrated startling capabilities. In 2015, a neural network system beat humans at the board game Go. In 2020, the company Open AI released GPT-3, a neural-network based text generation system that can produce compelling essays. This year, a neural network-generated digital painting won a prize at the Colorado State Fair. But to make these AI systems produce results, engineers must first give the algorithms a lot of data. The algorithms extract patterns from that data in an expensive, energy-consuming process known as training. One estimate suggests that by 2025, training a state-of-the-art network will cost billions of dollars and generate as much carbon dioxide as New York City generates in a month. Murat Onen, a postdoctoral researcher in electrical engineering at MIT, is developing technology known as analog deep learning that could help lower the cost of training AI.
MURAT ONEN: The value proposition of analog computing is the acceleration of large scale deep learning at a fraction of the energy costs.
SOPHIA CHEN: Before we get into analog deep learning, let’s talk about what deep learning is in the first place. In deep learning, you have this computer algorithm known as a neural network that takes in a sequence of numbers, called a vector, as input. The algorithm applies a long sequence of mathematical operations on that input vector to produce an output, another vector, to fulfill a designated task. For example, say you designed a deep learning neural network to be able to identify whether an image contained a cat. You convert the image into a vector, and the network takes that vector as its input. Then the network performs a series of mathematical operations on that vector to finally produce a 1 or 0, where 1 means the image contains a cat, and 0 means the image doesn’t contain a cat. In order for the neural network to deliver accurate outputs, you would first need to train the neural network. You’d give the neural network many confirmed pictures of cats, and the neural network would figure out what mathematical operations to perform on those vectors to deliver the proper answer. Figuring out what operations to perform on the vector is known as “adjusting the weights” of the network. In deep learning networks today, a computer chip would perform all of this digitally. That means converting all the data into binary numbers, and then storing and retrieving them in memory as needed for the task. The energy required for data storage and retrieval adds up. Analog deep learning stores and processes data entirely differently. The relationship is similar to analog audio signals versus digital audio signals. Think about the human voice—an analog signal—versus a digital MP3 file. In the MP3, the sound information is converted and stored as binary 1’s and 0’s. In the analog signal, the audio information is the sound wave itself.
MURAT ONEN: The physical place where the information is stored is the same place where the information is processed.
SOPHIA CHEN: Specifically, Onen’s analog device consists of resistors whose resistivity he can tune. The value of each resistor’s resistivity corresponds to the weights of the neural network. If you recall from earlier, that means the resistivity corresponds to the numbers that the network needs to multiply its input by to produce an accurate output.
MURAT ONEN: You have these resistive elements, which stores the weight value, essentially. But at the same time, it does the multiplication as well to physical properties such as Ohm's Law, Kirchhoff's Law, and so on.
SOPHIA CHEN: By using the resistors to represent information rather than transistors, he can make a simpler device. He estimates you can replace a couple hundred transistors for storing and retrieving binary values with one resistive element. So how do they tune this resistor’s resistivity? The resistor’s main material is tungsten oxide, which they can reversibly dope with protons from an electrolyte material known as phosphosilicate glass, or PSG, layered on top of the tungsten oxide.
MURAT ONEN: When protons get in, it becomes more conductive. When the protons go out, it becomes less conductive.
SOPHIA CHEN: Above the PSG layer, they keep palladium, which is a reservoir for the protons when they need to shuttle it out of the tungsten oxide to make it more resistive.
MURAT ONEN: It's a great material that absorbs protons like a sponge essentially.
SOPHIA CHEN: In the last decade people have used various materials to execute analog deep learning. But Onen says their new design has one main advantage over previous materials. The resistance of their device responds in about five nanoseconds. The previous state-of-the-art was on the order of milliseconds.
MURAT ONEN: Roughly speaking, they are a million times faster.
SOPHIA CHEN: In future work, Onen says that researchers need to better figure out how to integrate these analog devices into digital deep learning devices.
MURAT ONEN: It's not purely analog; it is analog and digital architecture. So there are these interfaces that where the transitions happen.
SOPHIA CHEN: In addition, he says they need to develop deep learning algorithms so that they can run efficiently on these analog-digital hybrid devices. This work was published in a recent issue of Science. My name is Sophia Chen from the Materials Research Society. For more news, log onto the MRS Bulletin website at mrsbulletin.org and follow us on twitter, @MRSBulletin. Don’t miss the next episode of MRS Bulletin Materials News – subscribe now. Thank you for listening.