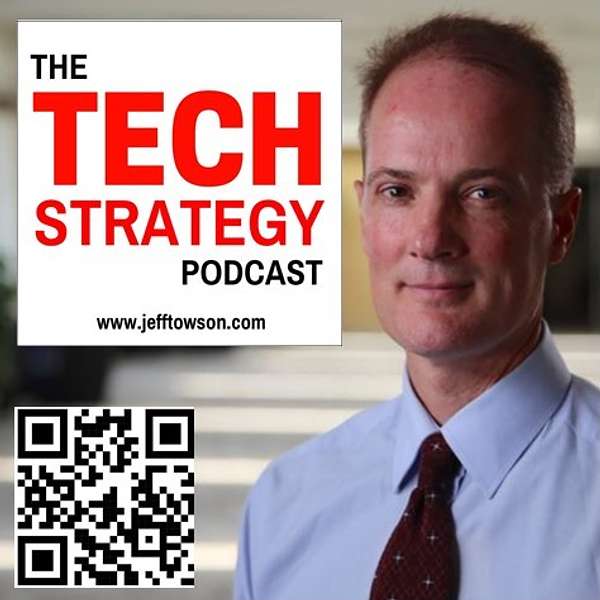
The Tech Strategy Podcast
A podcast by TechMoat Consulting on the strategies of the best digital companies in the US and China / Asia.
Tech Strategy offers:
-Deep dives into the strategies and business models of leading tech companies.
-Lessons on important digital concepts.
Lots more information available at Jefftowson.com and techmoatconsulting.com
To marketers, I do not have podcast guests.
This podcast is not investment advice. Me and any guests may get the numbers or information wrong. The views expressed may no longer be relevant. Investing is risky. Do your own research.
The Tech Strategy Podcast
How to Build GenAI into Operations - Part 2 (204)
This week’s podcast is Part 2 on my framework for AI Strategy. Part 1 was in Podcast 203.
You can listen to this podcast here, which has the slides and graphics mentioned. Also available at iTunes and Google Podcasts.
Here is the link to the TechMoat Consulting. Which has the details for the Beijing Tech Tour.
-------
I write, speak and consult about how to win (and not lose) in digital strategy and transformation.
I am the founder of TechMoat Consulting, a boutique consulting firm that helps retailers, brands, and technology companies exploit digital change to grow faster, innovate better and build digital moats. Get in touch here.
My book series Moats and Marathons is one-of-a-kind framework for building and measuring competitive advantages in digital businesses.
This content (articles, podcasts, website info) is not investment, legal or tax advice. The information and opinions from me and any guests may be incorrect. The numbers and information may be wrong. The views expressed may no longer be relevant or accurate. This is not investment advice. Investing is risky. Do your own research.
Welcome, welcome everybody. My name is Jeff Towson and this is the Tech Strategy Podcast from TechMoat Consulting and the topic for today, how to build generative AI into operations. So this is kind of part two of last week's talk, which was really a simple framework for generative AI strategy. I called it Gen AI strategy 1.0. This is kind of a continuation on that and we're going to get into really the heart of the whole issue, which is this idea of building an intelligence flywheel into the daily operations of a company. That's kind of the big thing at the center of this, and then there's a lot of other stuff around it. So that's what we're gonna get into today, and this will be a short one, hopefully 30 minutes, and there'll probably be one more, and then that should be it for this topic. For those of you who are subscribers, there's really five long articles coming your way. They're already queued up and done. It's pretty-- yeah, it's a little bit deep. It kind of went down the rabbit hole on how to think about AI strategy and generative AI and how learning happens by humans versus machines in an organization. Yeah, it's kind of a lot. The final one, number five, will be a summary and bubble up of here's a simple playbook. So if that's too much, just skip to the fifth one and that'll be the so what usable. But one through four, yeah, they got some depth to them. So anyways, yeah, I had fun with that. Okay, and let's see, how's keeping for this week? Not a lot going on. We have the Beijing Tech Tour. We basically have one spot left. We expanded a little bit the numbers. We've basically got one spot left. So if you're interested, that's May 22nd to 26th. Go into Beijing, gonna have a fun time. Four companies we're gonna visit, release the final name soon. They're great, like really, really good. It's gonna be great. And then some fun stuff, go into Forbidden City, go into the Summer Palace. We've got some restaurants lined up that are really fun, hot pots and some other things. We'll send out pictures of those soon. I thought that'd be fun. The restaurants are really cool. We're going to the drum tower. We're going out to the sort of lake with all the bars and that's really fun, by the way. Anyways, I'll send out the details. But there's basically one spot left. If you're curious, go over to TechMokeConsulting.com. You can find the tours there. All the details, including price, are there. Okay, I think that's it. Standard disclaimer, nothing in this podcast, or in my writing or website is investment advice. The numbers and information for me and any guests may be incorrect. The views and opinions expressed may no longer be relevant or accurate. Overall, investing is risky. This is not investment legal or tax advice. Do your own research. And with that, let's get into the topic. All right, let's start with the sort of digital concepts that are important, really three for today. One is something I've talked about before, which is sort of this machine learning flywheel talked about before, which is sort of this machine learning flywheel, or an intelligent an industry intelligence flywheel. Talk a little bit about that. Second is rate of learning, which is something I've talked about kind of a lot over the last couple years as a concept as something that is really a competitive dimension you want to think about. It matters in your tactics, it matters in your daily operations, and it can become a competitive advantage. So we'll talk about rate of learning, and then really how that applies to humans versus machines, because that's really the heart of what we're getting at here is there's a lot of thinking going back really almost 100 years on how individuals and organizations made up of individuals can learn and that can become an advantage. Well, this is the same basic idea but we're talking about how machines learn. That's kind of different, but it's arguably more powerful. So we're sort of layout those three ideas. I'm sorry, machine learning flywheel rate of learning is a concept. We'll also talk about the learning curve. So that's the three for today. And those are all in the digital concept library over at JeffTausen.com if you're curious. So that's the topic for today I'll go through. All right, now last week, the last podcast, I basically gave you a first pass framework for how to think about AI strategy. I called it AI strategy 1.0. It was pretty basic, which is you try and dial in as much AI into your products and services as you can. You either put it into your current services and you try and 10x the impact of those, or you build entirely new services that people haven't seen. And I put that all under the category of, this is what Steve Jobs does. He was very good at getting customers in growth, which is sort of your first main goal when you do strategy. Second goal, you start to energize and create operational excellence. I called that Elon Muskland. Okay, that's the other place where AI strategy 1.0 is playing out right now. When you look at what people are doing, they're putting this into products and they're trying to put this in their operations because obviously this is a huge productivity tool. You give AI to a person, their productivity goes way up, the quality can go way up. Although it's not as clear cut as you think it's going to be. There's actually some good numbers on this that have come out where if you give, what it does is if you give AI tools to let's say a legal analyst, it will have less impact on say, a legal analyst, it will have less impact on the high-performing legal analyst, but it will have a major impact on the low-performing ones. So it allows people with a lower level of skill to move up dramatically. And it has less impact on those with higher training, which kind of makes intuitive sense. The counterintuitive thing is the more you stray from what the AI is telling you to do and try and put in your own input, that makes the performance worse. For certain groups of people, you should blindly follow the AI and not do anything else, but for other people, if you put in your own input, not only do you not get to benefit your performance is worse than if you didn't use the AI at all. So it's really, that's an interesting question. But that was sort of the other aspect of, okay, AI strategy 1.0, put it into your daily operations, quality, timing, speed, productivity. And I called it Elon Musk, but I basically said, look, it's not going into gold number three, which is war and buffet land, which is build yourself a mode. We're not seeing it here, there. That's where I'm going to focus. Okay. Also within that, I talked about if you go back to podcast 197, which was basically Baidu's AI strategy. Now this is Baidu as an AI cloud service provider. So this is what they're providing. They're building the tech stack themselves, and then they're providing it as a service to companies. Now most companies aren't going to build their own tech stack. They're going to use an existing service like an AI cloud service like Baidu, or they're going to build some aspect of an AI core. But generally, yeah, and what they're building is what they call a flywheel in industry intelligence. And the basic idea, you can go listen to podcast 197, it's pretty important. I also put a quick summary of this in a couple slides. I'll put that in the show notes. If you don't want to listen to the podcast, look at the slides. But basically, there's two dimensions to think about. There is the idea that you want industry application and usage. You want people to use your AI tools, your intelligence tools, whether that's using a service, whether that's putting in an API, whether that's taking a baseline model like a GPT and customizing it for your scenario. The more industry usage you get, which is user engagement plus data, that will then feedback into fine tuning industry specific foundation models, which gets smarter and smarter. Those get smarter and smarter. That makes them more useful. More people use it. That's your flywheel. Okay, and this is really Baidu talks a lot about this. This is the center of their AI cloud strategy. Anyways, I've covered that in detail. I mean, kind of a lot of detail in previous podcasts on Baidu or look at the link in the show notes for a basic simple picture of you can call this a machine learning flywheel or highlight Baidu summary, which is this is an industry intelligence flywheel. That's really what they're building. Okay, so I've kind of given you two big pieces to think about. Here's a first pass of AI strategy 1.0. Here's kind of the big gun within all of this that everyone's focused on, this flywheel of intelligence that you can build within an industry, you can build within an organization. Okay, how do you start to dial that second piece into the operations of a business given that you're not by do? You're you're a supermarket, you're a bank. Okay, that's what we're going to talk about today. And that's where these two concepts of rate of learning and learning curves come up, which is pretty important. Now, this is what these five articles I've sent out are all about. But basically, for those of you who've read my books, the Moz and Marathons, I wrote a lot about rate of learning as I dimension you increasingly need to compete upon. If I talk about innovation, everyone knows that's a dimension you may compete upon. Now if you're Coca-Cola, you don't really compete on innovation. If you're SpaceX, innovation in space technology is the primary thing you are competing on and that you use to be Blue Origin. Everything else is second. So you got to kind of know what game you're playing. Other companies not so intensive of tech innovation, but a lot of digital companies have this. So innovation would be one dimension that you might compete on that's really digital and technology related. Another might be machine learning and another might be rate of learning. And if you look at my books I basically put this into the digital marathons summary that there's five marathons you can run that if you're very committed to them and you do them for a long time which is why they're called marathons, you can pull away from your competitors in an operationally significant way. And we see SpaceX doing that. They are so far ahead in their innovation and rocket technology. It's like it's like Marathon running and the person out front has disappeared over the horizon. You can't even see them anymore. That's an operational advantage that is largely sustainable. But rate of learning within the five I outlined, I called this Smile Marathon's SMIL. The L of Smile was rate of learning, L, and adaptation, which is like in some businesses like fashion or she-in or TEMU, your real dimension is not innovation. Your real dimension is being very fast in learning what customers want The real dimension is being very fast in learning what customers want, in constantly changing preferences. Maybe people in this city or this type of customer starting to buy hug boots. You are constantly learning very, very quickly and then adapting, changing your inventory, creating new products and meeting it quickly. So I call one of the marathons rate of learning an adaptation. And a lot of that is BCG, they're very good at the subject. The other place I put rate of learning is I said, look, and this actually does become a competitive advantage at a certain point. And I listed it in my list of competitive advantages. It's a variable cost advantage, which I called rate of learning and process cost advantage, which was CA7. If you want to look up that standard list of competitive advantages. And for those of you who follow Hamilton Helmer, who wrote seven powers-- seven powers, six powers, seven powers. He basically talks about the same thing when he talks about why does Toyota have a cost advantage over other traditional automakers? And the thing he points to is he calls it a process cost advantage, that there is such complexity with huge numbers of people in the cost structure and operations of the business that Toyota has built over 50 years, it's basically impossible to copy that sort of process cost advantage. They can build cars and design cars and upgrade cars and release menu models cheaper than others sustainably. Well learning and adaptation it's kind of the same idea but it's not manufacturing based which is what he was talking about it's more digital base that's more what I'm talking about. Anyways so I've talked about rate of learning as something that matters at almost every level of the organization. It matters in your tactics, it matters in your daily operating basics, it can show up as a digital marathon, and it can show up as a competitive advantage. So four of the levels in my sort of digital, you see rate of learning. Okay, that was kind of how I teed that up. Now, but when I was talking about all of that, I was really talking about learning by humans, how we learn individually, how we learn working in teams, how we learn as a larger organization like Toyota, how we learn as an industry in an ecosystem like something like semiconductors. And all of that was based on that. And I'll give a little background on how to think about that. But it starts to become different when we talk about how do machines learn? Very different. And that's what I've been trying to take apart. Hence these five kind of down the rabbit hole articles I've been doing, that's me taking apart that question. And I'll give you my summary my so what at the end. Okay. So let's move on to the next topic which is basically learning curves. And again this is something I wrote about a lot in the books. But based, this is an old idea. This is learning curves was how business people started to take apart the idea that humans learn. And the simplest version of that is, look, if you put an individual who's learning to memorize words or numbers, the more they try, the better they'll get at memorizing. That's how the human brain works. And that works with teams too. If you give teams something to do, like you're all gonna start assembling these aircraft engines, which is very labor intensive, you come back six months later and that team will get better and better and better. They'll be faster, they'll do it cheaper. Usually cheaper means if you do something faster it's cheaper with labor. They'll get better, there'll be fewer defects and problems. There's just something about how humans, we get better the more we do things. And this came out of psychology studies that showed this and then people started to apply this to manufacturing fields in the 1920s and 30s and 40s and especially not really manufacturing but assembly. Because assemblies when you have a lot of people putting stuff together, if it's machines doing things this doesn't apply. But when it's people putting together the parts, labor-intensive, we start to see this. So 1885, a German psychologist first labeled this, called it the learning curve. And basically humans get better the more we do things. And this led to what they call the learning curve, and I'll put an example in the show notes. On the x-axis, we basically have experience, and on the y-axis, the vertical axis, we have proficiency in learning. And as you move from left to right, you can draw a line going up, which is the more experience you do something. You get more proficient at it. Your learning gets better. So learning curves are kind of a graphical representation between proficiency and experience. Now, what does experience mean? Usually when people were talking about this, they meant cumulative volume. If you are assembling model T cars or aircraft engines, the more you have done cumulatively over one year to the better you get. What do they mean by proficiency, which is another word for learning? Usually they would look at the cost. The cost poorer completed engine would go down. The cost of a specific task within the workflow would go down. Or it would get quicker. Or there'd be fewer defects. Quality would go up. So you can kind of look at-- you can redefine proficiency and experience on these axes in different ways. And the reason they got really excited about this, and particularly in the 1960s and 1970s, BCG, one of the founders, Bruce Henderson, he wrote a ton about this, about learning curves and what they ended up calling the experience effect, which was basically if you go they also called it the right effect because there was an Air Force base in Ohio called the Wright Peterson Air Force base. And that's where they kind of noticed that when they were making aircraft at the right Patterson Air Force base in Ohio, every time they doubled the overall cumulative production of aircraft assembly, the time to assemble an aircraft would drop by 20 percent. And it kept happening. Every time they doubled the volume that they had done historically, they were doing it 20 percent quicker again. And so people started to think this was a power law and that we could, you know, if we keep doubling production and investing in our scale, we would get cheaper and cheaper over time by virtue of what they call the experience effect. Now, that didn't turn out to be totally true. It turns out nothing gets better like that 20% every time. Nothing goes on forever. So in certain industries like making laptop batteries, creating new keyboards, things like this, it turns out there is actually an experience effect when you jump into a new category that's very sort of labor intensive and very complicated. It doesn't work well when it's mostly done by let's say equipment in a factory and it's simple. You don't see an experience effect. If it's done by people and it's kind of complicated like making cars like Toyota, then we actually can see an experience effect for a while, but eventually the effect the effect does drop off. Okay, that's not a bad summary for how to think about learning curves and the experience effect. And you can draw different types of curves within these graphs. Some of them go up exponentially. The more experience you get, your proficiency just keeps going up. Most of them sort of asymatote where they, you know, they sort of go fast and then they flat line. That's pretty common for most things. Maybe you get an S curve and so on. Now, what I've done done and I'll put this graphic in the show notes, I've basically relabeled that as saying look these are human learning curves. That's different. Everything we've just been talking about those aren't learning curves. Those are learning curves for humans. Individually in teams in groups. And the KPIs we want to look at on the x-axis and the y-axis, which are important. I Basically detailed those out. So on the x-axis, which is the experience metric we would look at cumulative volume of a task or product we would look at cumulative time spent on a task or product, we would look at cumulative time spent on the y-axis, which we would label proficiency. We would look at things like the efficiency of a task or a product, the quality of the task or product, the advancements and improvements in that task or product over time. That's how we'd kind of map that out. So we can look at the KPIs, and we can map out a learning curve, and we can look at the KPIs and we can map out a learning curve and we can improve it over time. And this is something you really want to be doing in your operations. These are very good operational KPIs if you're in a world where you might see a learning curve advantage play out. Okay and I basically put the summary in the show notes. You can look at there for human learning curves. So for rate of learning, you now have a couple tools you can use. I've given you sort of how to think about it as a digital marathon, how to think about it as when it becomes a competitive advantage. And here's some KPIs you can look at just for doing this within daily operations. That's not a bad little set of frameworks for the rate of frameworks for the rate of learning for humans by humans. Now let's switch gears and get to rate of learning by machines, which is the whole point. All right, how is machine learning different? How is rate of learning by machines not humans different? Oh and by the way, I consider humans using digital tools in that first bucket I just talked about. If you give a human a tool, some software, maybe a co-pilot to work, it's gonna play out pretty much the same way. Now we're talking about learning without humans. We're talking about how the machine itself learns with no humans. So different. Well, the first question is like, okay, do machines, do they learn and become more proficient based on cumulative experience like we just said? You know, is the more cases they run and predictions they make, do they get better and better? And the answer is yeah. But is that the primary driver of their intelligence and proficiency? Like it is for humans? And I think the answer is no. I think that's secondary. I think the biggest driver for increasing proficiency, i.e. intelligence in machines, is probably the volume of unstructured relevant data that they have access to and that they can compute. All right, that was a wordy phrase. Let me break that down. One of the things Baidu talks about Robin Lee, who I think is incredibly smart about all of this, you know, he talks about the foundation models. They're building and the industry specific models and the industry specific flywheels that they are building. And he basically says, look, there's two factors that really matter. I'm paraphrasing, obviously. This is direct quote, large scale knowledge maps and massive unstructured data, resulting in more efficient learning with strong interpretability. That's a direct quote. So massive, I mean, what does generative AI need? What does basic AI need? What is generative AI need? It needs huge amounts of unstructured data that is relevant to this case, that is relevant to this industry, that's related to banks, that's related to supermarkets, that's related to chatbots that's related to supermarkets, that's related to chat bots that are good at customer service. So if that's the biggest driver, massive amounts of relevant data, if that's the biggest driver of proficiency, that's what we want to put on the x axis of a learning curve for machines. One of the things, okay, all that data has to be flowing in because keep in mind, generative AI machine learning, it does not store all this data. It's too much. The data flows in like a river. It has to process it mostly in real time and give a result, give a prediction, give a content generation, and then maybe update its knowledge map, which I'll talk about in a sec. So it's not stored like traditional AI, that's not the way it works. Traditional AI was based on predictive AI was based on relatively small models using mostly internal data to a company. This is not that. These are massive models dependent on a real-time flow of massive amounts of data that it can then process. So it needs access to massive amounts of relevant data, and it needs computational power to process that and it needs huge amounts of comp power. That's very different. When I think about that one, what is the primary driver of intelligence proficiency that I would put on the x-axis, the horizontal axis, I would put access and the ability to basically compute massive amounts of unstructured relevant data. That's what it needs. And not every company is going to get that. In fact, very few companies are going to be able to generate that internally. They're going to be dependent on ecosystems in their industry. They're going to be dependent on ecosystems of data coming from services like Baidu or AWS. So that creates the access part of that, the connectivity part is very important. The computational power is very important. OK. Then you also ask, all right, what kind of data are we talking about here? Are we talking about data that's just flowing in passively from cameras all throughout the factories that are watching the production line that are watching the security that, you know, is it IOT devices that are sensors in every bit of machinery? That's very sort of passive data that's flowing in. Okay. Or is this data that needs to be created by users and the fact that they are engaging with me in some form? All that IoT and camera data is not gonna help me predict what video you wanna watch on TikTok next. For that, I need you to engage. I need you to click and likes and flips. So there's passive data that just comes from the world or for senses. But often when we're talking about data that we need to do this, we're talking about users and users engagement that then generates data that we use. So this whole bucket is not just data, it's users, user engagement, and it can be passive data. And that can start to split into various buckets like, okay, some of this data is just going to be about something that's factually true, like mathematics or science. It's not going to change. So as my proficiency grows in that subject, it's kind of static. But other types of proficiency and intelligence is going to constantly change. Like if I'm flowing in all this data and getting very good traffic patterns for Beijing, well the traffic proficiency and intelligence I have today is going to be very different than what I had yesterday. So certain intelligence gets created and then goes away. Other intelligence is more stable like mathematics. Others, it becomes, it's not that it goes away because yesterday's traffic is worthless. It's because the users I'm getting some of the data from change their preferences and interests. What videos I want to watch on TikTok right now may not be the same as yesterday. May I watched a bunch of those videos yesterday, but now I'm sick of them. I was watching these funny standup videos. Now I wanna watch videos about elephants, right? So preferences can change. Therefore the data that's relevant, that's why I use that word relevant, relevance can change. So anyways, when you get into the data flows, that's kind of how I think about the x-axis for this. Now there's other stuff I would put in there. What I would put on the x-axis, if I was building a learning curve for machines, the x-axis would be industrial application. That is the metric I need to keep increasing. I need to have more and more people using this. It needs to be used in more and more cases. And from there, I need to see user engagement. I need to see relevant data. And I need to see knowledge maps. Now, knowledge maps are when all this data flows in, you start to develop industry specific knowledge maps that help you weed through that data and become smart. That's a whole nother topic, but that's kind of the three KPIs I have for the X-axis, and I've put this all in a graphic I'm putting in the show notes. So I've given you an X-axis for building learning curves for humans,. So I've given you an x-axis for building learning curves for humans and now I've given you an x-axis for building learning curves for machines. We get to the last point that I'll finish up for today. OK, what's the y-axis for a learning curve for a machine? For this one, I would say it's knowledge enhancement or we could call that intelligence. It's not proficiency, like it is for humans, 'cause proficiency is like I can make engines cheaper. That's a proficiency. It tends to tie to things like per unit cost, time that a human would spend doing this, which is a cost. Well, we don't talk about any of that for machine learning No for knowledge enhancement the three KPIs. I look for Knowledge and accuracy That's something like look. Okay. How good are you at mathematics? Okay, you've built an AI translator that can turn French into English How good is your accuracy for your translator? Is it good or is it bad? Is it 90%? Is it 85%? Fine. That would just be a metric that looks at quality accuracy. How smart are you? Can you pass the medical boards or not? What scores do you get? Okay, the second KPI would look for is what I call adaptability and relevance. Now, being able to pass the medical boards does nothing to tell what video I wanna watch next. That would be more something like relevance that you understand my engagement and what I've been responding to. So it's gonna tee up the most relevant video for me right now. That's not accuracy. That's more about what I'm interested in. And you could also say adaptability. Okay. What was accurate yesterday in terms of the traffic patterns of Beijing is no longer accurate. So we have to be adaptable and recreate our knowledge of this every day. So the second KPI I look for is adaptability and relevance. The third one, last one, efficiency. Efficiency. Now, okay, that's cost. That's a big deal. What does it cost you to run, you know, a prompt? What is your token read? What is your latency? Are you super cheap and fast? This company that Chamat Palahapatiya, I'm learning to say his name better, you know, the company he keeps talking about is Grok. Wait, is that the Elon Musk AI one? And it was basically, it's a service sort of token layer that focuses on being able to run prompts super cheap and super fast. Because the cheaper and faster you can make these prompts go through and get a response, the more this can become an interactive experience where suddenly you're having a real-time conversation with the AI as opposed to putting in a prompt and waiting 20 seconds. So anyways, that's time latency, but those are the three KPIs I use. Anyways, based on that, there's a machine learning curve, a learning curve for machines versus humans. I've put that in there with the KPIs. And then what you would obviously want to look for is as you plot these out, does it go up for a while and then flat line, Which would happen with a translator between French and English, right? You may be real good at this and your flywheel may be very powerful, but everyone's gonna catch you because the quality level is gonna flat line. Pretty much French English translators are pretty much ubiquitous. Anyone can build these now. So you don't want a learning curve that goes up in flat lines. Well, you may have to do that, but it's not going to give you a sustainable advantage. What you want is one that is more exponential or that goes up higher where it keeps going up and up. So the more data you have, the more engagement you have, the norm knowledge match up, you always have a superior product, which is basically what Google searches. Google search gives better answers for search queries because there is such a massive long tail of content and everybody uses it. So it's kind of got a bit of a network effect, but it's the same basic idea. A lot of this knowledge and intelligence is just going to become a commodity that companies just have to bake into their operations, but it doesn't give you any real advantage. In some cases, it's going to become a real powerful advantage. It's going to become a dimension with competitive strength like rate of learning, innovation, hence the digital marathons I talk about. OK, I think that's all I wanted to go through for today. That was a bit of theory, kind of quickly. I'll put the graphics in the show notes. The three ideas to take away from this are think about this flywheel for machine learning, this flywheel for industry-specific intelligence. Very important idea. Think about rate of learning as an operational requirement. Sometimes it becomes a digital marathon, sometimes it can become a competitive advantage in humans. And then this idea that like, now we have to rethink about this whole idea for machines, which is different. And the way you think about that, in my experience is learning curves with KPIs that you measure, and then you get a sense of the shape of the curve. And those are the three concepts for today. They're all in the concept library. And for those of you who are subscribers, I almost feel guilty for how much content I've spent. It's like five articles. They're each like two to three thousand. It must be 15,000 words I've written about the subject. No, I really like it. And I think there's a small subset of subscribers who will really get into it. But for a lot, it's going to be like, oh my, for those who it's too much, just look at the graphics and then wait for number five, part five, 'cause that'll just be a summary of what's usable. Okay? If you don't want to know all the mechanics. Anyways, that is it for me for today. I'm 34, 35 minutes a little. I'm pretty close to on time today. Anyways, I hope that is helpful and yeah, I don't really have anything fun going on right now just working like crazy. Waiting for the end of this TV show, Shogun. It's like the last two. I really love this show. It's just the best. The best description I've heard of this TV show, Shogun, is it's like Game of Thrones, if Ned Stark was super smart and super crafty, which is about right. Like that's what it is. It's game of thrones but instead of Ned Stark getting his head cut off in the first episode or whatever, he's super smart. That's the guy who's the center of this TV show. Anyways, that's it. Okay, I hope everyone is doing well. I hope this is helpful and I will talk to you next week. Bye-bye.