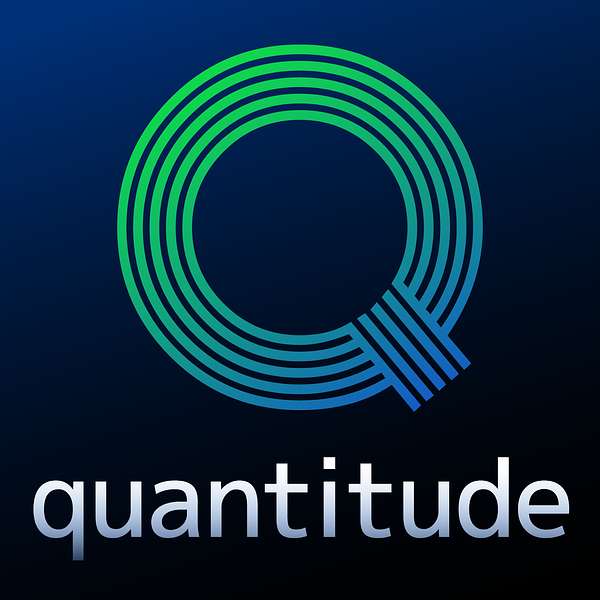
Quantitude
Quantitude
S4E02 Underachievers, Overachievers, & Maximum Likelihood Estimation
This week Patrick and Greg talk about maximum likelihood estimation: what it is, where it comes from, how it works, what it can do, and what it can't do. Along they way they also mention tour-bombing your kid, licking the turtle, Van Halen and AC/DC, orange mustaches, brandy snifter pong, bus #27, Ronnie Fisher, why people hate us, circus tents, night parachuting, flat spots, lazy parabolas, vanilla ice cream, and statistical bouncers.
Stay in contact with Quantitude!
- Web page: quantitudepod.org
- TwitterX: @quantitudepod
- YouTube: @quantitudepod
- Merch: redbubble.com
Hi everybody, my name is Greg Hancock and along with my asymptotically efficient friend Patrick Curran, we make a quantity food. We're a podcast dedicated to all things quantitative, ranging from the irrelevant to the completely irrelevant. In this episode we talk about maximum likelihood estimation, what it is, where it comes from, how it works, what it can do and what it can't do. Along the way, we also mentioned tour bombing your kid licking the turtle, Van Halen and ACDC orange mustaches, brandy snifter Pong, bus number 27. Rodney Fisher, why people hate us, circus tents, night parachuting, flat spots, lazy parabolas, vanilla ice cream, and statistical bouncers. We hope you enjoyed today's episode.
Patrick:So I couldn't help but notice that you just took your own sweet ass time to join us here today.
Greg:I am sorry, I am sorry, I hate being late. So one of the things about me early is on time on time is late and I was actually late late and I really, really apologize.
Patrick:So let's hear your air quote. Excuse. Honestly, it's not that
Greg:my son Quinn is taking a tour of campus with his really good friend Alex. They're walking around doing the official University of Maryland tour. And I went and tour bombed them. So I snuck up really bad. Oh, geez. Well, anyway, it did my heart good to sneak up in there and be a part of the tour and, and to see my kid you know, as he's making important decisions. It was so cool though. Just listening to the tour guide, you know, really inspirational college aged kid talking about all the great things that those students can achieve. While here at Maryland. It was nice. I got a little teary.
Patrick:I bet you did. Especially when everybody was made to lick the E. coli turtle on the quad. Isn't that the tradition?
Greg:You don't have to lick it, you can just rub it with your hand lifting is completely optional.
Patrick:And you know, we've done our tour kind of things. And I love that you can be anything you can do anything right? And all made me think of is almost TV game show. And what did you accomplish by age 20? Because I think we are part of an entire generation that came up through the most boring part of history in terms of having your character melded in the furnace of Oh my god. Yes. stress and strain. It's like yeah, no. By age 21 of my greatest achievements has been I saw Van Halen both with David Lee Roth and Sammy Hagar. No much this in perspective, my great grandfather came over on a famine ship from Ireland at age 16, unaccompanied. His son then founded a printing company, his son, who was my father was born nine months after the stock market crash in 1929. And did soup can collections for steel for World War Two. And I saw both David Lee Roth and Sammy Hagar
Greg:later we're going to expect great things from that Patrick.
Patrick:Nice still pales in comparison is, you know, famine ship. Your grandmother survived Auschwitz.
Greg:Yes, she did. And it kind of makes anything that I do. You know, I can only disappoint. By the time I was age 20 or 21. I had gotten kicked out of a college honors program and I grew an orange moustache.
Patrick:It does make me start thinking about when do you make your great achievements and again, with things like mathematics as people talk about that, if you haven't made a truly great achievement by your mid 20s. You're not going to Yeah, which makes me very sad. One of my favorite early achievers is our A Fisher and we've talked about on prior episodes, we also want to embrace what our history is. In one part, Fisher was a very unpleasant human being and for a period of time had very unpleasant philosophical beliefs and dedications. On the other hand, developed analysis of variance analysis of covariance degrees. sort of freedom. And in his 20s, what we might kick around a little bit here today, maximum likelihood estimation. And what did you do by age 20? Dr. Hancock?
Greg:Did I mention the orange mustache?
Patrick:We'll do the orange come from
Greg:I was born with red hair. And so I always sort of had this underlying red that was dormant. And then by the time I was able to grow mustache and beard, it came out looking like I had creamsicle on my Tell me one
Patrick:thing that characterized you in your early 20s, set against the stage of degrees of freedom and maximum likelihood.
Greg:Do you mean one achievement? Yeah. All right. I have no idea what my actual achievements were. I think there weren't a whole lot of them. But along the lines of you, I saw ACDC six times. Oh,
Patrick:okay. I am truly impressed. Okay, because you know what, I have never seen a CDC.
Greg:It's a shame. Angus is crazy amazing. Even at the age he is now.
Patrick:So why are we talking about maximum likelihood? First is it's cool as crapola. Second, last week, we kicked around ordinary least squares. Yeah. And we talked about if your camel wanders off, does it drop off the edge of a disk? Does it walk on a plane? That's a that's a Kindle, it just never comes back. Okay? Or is it a sphere where your camera comes back, but scares the crap out of you? Because now he's behind you. And so we talked about Genesis of least squares characteristics, and how there are some really magical properties of least squares. But Fisher, who was not tempted to see Van Halen, or AC DC, he was able to identify certain limitations of those approaches. Yeah. And invented maximum like, that's right.
Greg:So he stopped playing beer pong, as he was wanting to do
Patrick:it think it was brandy snifter upon at that point. So much class? Exactly, exactly. Yeah. So
Greg:this was Fisher's, I don't want to say answer to least squares. But if you think about trying to figure out what is a best fitting model, we talked about the least squares criterion as one way to characterize that, and that has legs that extend into many dimensions, because you go past simple regression into the multivariate world, and least squares carries forward, it really does a good job. But he had a very, very different way of looking at this idea of fit and models. And it was incredibly clever. And damn it, if he wasn't like 22 years old. It was
Patrick:another instance of Calvin Ball, where we talked about last week of let's redefine what we mean by best, right, and what we're going to maximize or minimize, depending upon how you go about doing things. And maximum likelihood is approaching the very same problem. That is, we believe these parameters to exist in the population, we are unable to observe them directly. And we want to obtain the best possible estimates of those given our sample data. And he just shrugged and said, I actually have another way of defining best and develop this whole new class of estimators, which, as we'll see, really is the coin of the realm in almost everything that we do.
Greg:Yeah, maximum likelihood is something that gets really hairy when it starts to get big in terms of the models and other things. But I don't want to spoil it. And we don't need to start there. Let's start small. Do you have maybe a little example just to plant some of the ideas of what maximum likelihood is and how it helps us to think about things I
Patrick:do? It was at this point that I proceeded to tell an 11 minute story on tank production during World War Two, of which Greg, the entire thing, I just thought it was very important to clarify how interesting that was, here's your job, you are sent to a town and your goal is to estimate how many buses are on the street at any given time. Now they send out buses sequentially numbered the first buses, one, the second buses, two up to the number of however many are out on the street at a time. And you have to here's the Calvin Ball, pick the best guess for how many total buses are on the street at that moment. Here's the trick. You're only allowed to observe one bus, you get a cup of coffee, you sit on the bench, and you wait for a bus to drive by. And bus number 27 drives by that's it, you're done. You have your one observation. And the challenge is estimate how many buses are on the street, given that you just observed 27 Well, there are at least 27 That's right. So the maximum likelihood estimate in that scenario is 27. Why? Because the highest probability that you would have observed that bus is if there were 27. On the street, there was a 127 chance that you would have observed that bus. If there were 54 buses and 27 drove by, there was a 1/54 chance that you would have observed that bus. So you would have picked a value that made it less likely that you would have observed that sample data. I really love that, how do we select an estimate of an unknown parameter that maximizes the likelihood that we would have observed our sample data? Yeah,
Greg:what you just said is perfect. That whole flipping of things on its head is at the core of maximum likelihood. We're so accustomed in the way we talk about things to say these are the characteristics of the population, for example, there is this mean, and there's this variance. And we talk about what is the probability associated with a particular observation. And this just completely flips it? So so cleverly that now we say, we have observed these data? What would the configuration of the population have to be like for our observations to have the maximum likelihood of having occurred? So when you talk about seeing bus number 27, the configuration for the population would not be that there are 1000 buses, because the likelihood of observing bus number 27, would have been so small. So at the crux of maximum likelihood is figuring out what would the population have to look like so that the observations we have in our hand have the maximum likelihood. And that was this beautiful flip that the young ra Fisher Ronald Rani, as I'm sure they called him, had this insight into how to think about things.
Patrick:And it does introduce a point of confusion of the use of the word probability and the use of the word likelihood, because you were very careful in your language right there and very appropriately. So if I give you a mean and a variance, and then talk to you about what is the probability of randomly drawn score will be above this, or below that or in between these two values? Typically, we talked about that as probability. Here, we're going to use a different terminology, which is, what value of mean and variance maximizes the likelihood of the data that we observed. So we already have the data. And we're talking about what values of these estimates would make this set of data most likely that we observed and that is that same thing with the bus, what number of total buses would maximize the likelihood that you saw that bus, and it's 120 summit.
Greg:Now, given that we don't have a ton of listeners who are in the bus sciences, let's translate this into things that people think about and maybe start with a set of scores. They could be heights, they could be scores on a test, we think about this in the univariate situation, how do we translate this into the maximum likelihood world?
Patrick:Okay, let me ask how you teach something. Because I think anybody who ever teaches this has to wrestle with this issue, we're going to talk about a univariate normal distribution. And much of what we talked about today is going to relate to naturally normal theory, maximum likelihood, it turns out, we don't have to do that there are other ways we can go about doing it. But we are going to assume this normal distribution, all of us have seen this beautiful expression for that one over sigma root two pi e to the negative z squared over two. Nice. Now what that does is it's just like a line, where if you have a plus Bx and you drop in points across X, and it draws a line, this is exactly the same, it's a sausage maker, and you drop in values of x in it draws out this beautiful normal distribution. Alright, so here's my question for you. You drop a particular value in crank it, and it gives you a specific numerical value, which is the height of the normal curve at that point? How do you define what that is to your class?
Greg:Yeah, I certainly don't refer to it as a probability. Nope. I talked about that value as being likelihood. And one of the reasons is that if you think about a normal curve, or frankly, any curve in order to amass a certain amount of probability, we usually have to frame an interval associated with it so that we can take the area under that curve and say, how much of the area under the curve are we talking about? If I give you a specific point and say, how much area under the curve does the number 14 Point to occupy, unless you assign the number 14 point to an interval, like an interval that goes down to a little bit below 14.2, and a little bit above, it's very hard to talk about that probabilistically. But of course, when you drop 14.2, in the sausage maker, it will still tell you how tall the curve is above it. But it really isn't in a metric that we would call probability. It's related to probability. But I will use the term likelihood associated with that. Yep, exactly.
Patrick:That's the value of the likelihood. Let me give you a pop quiz. What is the probability of a fair coin getting a heads point five? What is the probability of getting two heads in a row on that fair coin,
Greg:point five times point five, because they're independent events, point two, five. All right, so
Patrick:you did two things to that I was after you multiplied the individual probabilities. And you assumed that they were independent, what that allows is, we can get the joint probability of those two events because they are independent from one another, whether you get heads or a tail on the first one has no bearing on whether you get a heads or a tail. And the second one, we can apply that same principle to taking these individual likelihoods. But for a set of observations, compute the individual likelihoods. And if they are independent, we can take the product of those likelihoods and get an overall value that represents the likelihood for the set of observations. I love
Greg:that. So if you had a group of people standing on a number line, and you put this curve over their heads, the people who look up and it's a long way to the top Those are people are standing in a place, that's very, very likely. And if someone is standing out near the tails, they look up and that curve is crashing down on them, they have a value that is not particularly likely. So things toward the higher parts of the curve are more likely things out toward the answer less likely. And when you multiply a bunch of things, it will be really big if a lot of people have those high values of the curve above them. And it will be really small if a lot of people have really small values of that curve above them.
Patrick:Alright, so let's say we have five observations, let's go to your people, right on the number line, you have five different people in there scattered on the number line, we're going to start super simple, we are going to somehow know what the variance is. And we're going to hold that constant. So picture just a normal distribution, we are going to slide it up and down, we're not going to move the people we're going to move the curve. Oh, that's so important. I am going to start by rage hooking it. And then I'm going to let you sexy whole kit. All right. I'm gonna rage HK and I'm just going to randomly drop it down over the five, throw it down. Yep, just throw it down. And let's see where we start. Well, what I'm going to do is that normal distribution, that's going to be somewhere over the five cases, I'm going to take each case and go up to where their observation intersects the curve. And that is their likelihood. And I'm going to write down all five of them. And I'm going to multiply all five of them. And I'm going to get some product of those five likelihoods it's going to be some value, whatever. And then rage HK is going to grunt and move the curve over a little bit and redo it, and the product of those likelihoods. It's actually smaller than the first one. Alright, so that's less likely. And Rachel grunts and pulls it the other way. And now it's bigger and moves it left and moves it right and moves it into rage Hulk is raging and moves this intel, it's not changing very much around the largest value possible. And then range hope grunts and stops. And the mode of that distribution defines the center of the distribution that maximizes the likelihood of observing those five cases. Now sexy Hulk me
Greg:about rage Hulk by the way, rage Hulk stopped at some point moving that thing to the left into the right, right, Rachel was tired. But then sexy Hulk comes in and pushes up his glasses and says it is a calculus problem where you can take the formula for the normal distribution and the observations that you have. And you can actually derive what is the population value for the mean given a particular variance that would maximize the likelihood of these five observations. And if we go through the calculus of it, we don't just beat on this normal curve left and right. What we do is we take the derivative we say At the derivative equal to zero, and we're looking for the value, whatever it is of mu that maximizes this particular function. And through calculus, derivatives, all of that stuff, it comes out just so beautifully sexy Hulk has big tears flowing down his green face. And the sample mean, as we know, it is the maximum likelihood estimator of that best possible central location for the normal distribution.
Patrick:And what you wonderfully described in terms of derivatives and setting them to zero and solving. We talked about last week for the ordinary least squares, it's right, and it's a very similar criterion. It's just a different Calvin Ball rule. So last week, we said we're going to pick the values of the intercept and slope of a regression line that minimizes the sum of the squared residuals, we described, it sketches out this beautiful parabola, and rage, HK says, what about this? What about this, what about this, and we fill in a parabola, and sexy Hulk says, I can write an equation for that the first derivative that equals zero is the bottom of that trajectory. And that's going to be my best estimate, for least squares, we're doing a very, very similar thing here. But now we're changing best to what is the point for our estimator that maximizes the likelihood that we would have observed our sample data. So we're doing a very similar kind of thing. But just with a different criterion, instead of minimizing the sum of the squared residuals, we're going to maximize the likelihood that we would have observed our sample data
Greg:exactly. That is how maximum likelihood works. And we're talking about it right now in one dimension, but it extends to dealing with other things in one dimension, like the variance, and then multiple dimensions itself.
Patrick:If you've taken a multivariate class, especially a matrix based one, it is so elegant in how you can take that univariate distribution function that we just describe the one over sigma root two pi e to the whatever, and you just drop in a mean vector and a covariance matrix and a vector of observed values. And now it's a multivariate normal distribution, and everything just scales up. And instead of taking a derivative, you take partial derivatives, you take derivatives with respect to each parameter, everything just scales up. Here's where we can do one little fix up. And this is another very confusing thing that you encounter. When we do the independent multiplication of the likelihoods that we've been talking about, well, small numbers get smaller when you multiply them point one times point one times point one times point one starts getting really, really small. I mean, it starts getting small to the point where your first significant digit can be 810 12 decimal points out,
Greg:oh, yeah, at least. So
Patrick:we do a little judo move one thing out of the way to do something else with it. And I say, I remember in ninth grade, that logs are magical, we are going to take the natural log of that likelihood expression. And what that does is when you have an exponent in products, natural logs magically change products to sums. And so what we're going to do is we're going to use this natural log transformation, which by the way, is monotonic, meaning that the maximum value of one is the same as the maximum value of the other. But that is where you're reading your own work, or you're taught in a class that we're trying to maximize the log of the likelihood. That's why we're doing it's just a monotonic transformation. And then one last little thing we sometimes do is in the normal expression, that probability density function in the cockpit of the exponent is a negative one half. And it's just there, it's fine. It's just minding its own business. But if we multiplied by negative two, it just makes it go way tidies house a little bit. And so what we're ended up with is negative two times the log of the likelihood is what we're trying to optimize when we use maximum likelihood to get these parameter estimates.
Greg:And that was sneaky how you use the word optimize it, you see, because I didn't notice. I did I did so. So just to be clear, when we try to maximize the likelihood that is the same as maximizing the log of the likelihood of all of the observations. So rather than maximizing the product, which is the likelihood we maximize the sum of the logs, which is the log likelihood, but when you multiply that by minus two it flips so we don't try to match maximize the negative two log likelihood we actually try to minimize the negative two log likelihood. So this becomes a problem of minimizing a particular function. And I will tell you, even though it's maximum likelihood, the idea of minimizing this function still feels good because we're trying to minimize the amount of badness of fit. So I'm totally cool with the minus two log likelihood
Patrick:I am, but this is why people hate us.
Greg:That's it. That's the reason why the minus two,
Patrick:let me see, let me pull down the third volume, part C, section A, of why people hate us. You're trying to minimize the Fit function to get the maximum likelihood estimate. Yeah. Or it's not why people hate us, but it's certainly on the list. But as Greg just really nicely described, these are all monotonic transformations. And so the value that maximizes the likelihood is the same as the value that maximizes the log of the likelihood is the same as the value that minimizes negative two times the log of the likelihood. So they're all the same things. We're just using these things to our advantage, right? It makes it easier, it makes it more tractable. If you're a multi level modeler and you've heard the term deviance, well, deviance is negative two times the log likelihood.
Greg:So is that what that person meant when they said we are deviance? That's what I'm going to go with? Okay. Well, that makes sense when
Patrick:that person was my daughter.
Greg:Alright, so before we move on, I want to give one attempt at visualizing what's going on. And that is something I've mentioned before, which, which is the circus tent. Let's imagine just temporarily that we are doing the smallest multivariate example where we have two variables. And this extends to many variables, but we got two variables x one and x two, so that people can be thought of as standing on a plane on a grid. So if my score on x one is four, and on x two is 13, then I go stand at 413. We have everybody go stand on the floor at the location that corresponds to their x one and their x two points. And now what we have to do is put a tent overhead. But the criterion is that the tent is as high above people's heads as possible. Collectively. That means that if we are all standing toward the middle, we're not going to put the tent three kilometers down to the left, we want to have some tent that is overhead and the things that we're allowed to move. Well, if we decided to normal tent, a normal theory tent, we're just throwing that around as though Oh, it's a given that it's normal. But that's an assumption, right? If it is a multivariate normal tent, then what we have to do is decide where are we going to center this tent? And then how stretched out are we going to make that tent how stretched out in the x one direction, how stretched out in the x two direction, and then how much we're allowing the tent to reflect how much x one and x two might be related to each other. And rage Hawk is going to her moving the tent, squishing the tent, doing all of that kind of stuff. In the end, everybody has a tent above their head to a certain height. And if a lot of people have the tent nicely high above their heads, they are very likely in that multivariate space. And if the tent is crashing down on top of their head, then they have a very small likelihood in that multivariate space. And what do we do exactly what Patrick said we take everyone's likelihood, we multiply them, we take the logarithm, we multiply it by negative two to try to decide what is the negative two log likelihood associated with those data. Given that location of the tent rage Hulk moves the tent all over the place repeating this process over and over and over in two dimensional space. But hot Hulk sexy Hulk can derive exactly where that tent needs to be centered how stretched it needs to be in the x one direction, that's the variance how stretched it needs to be in the x two direction. That's the variance and how much those two relate to each other. That's the covariance. And all of that is done by calculus.
Patrick:But we still need rage, HK. This is a value it is always about me. In Episode 102, this is just dawning on you this just in it's all about Patrick sexy Hulk can do those normal equations, where you lay out the derivatives, you set them to zero and you solve up to a point. And then you can no longer for complicated models. You can't calculate these derivatives in closed form anymore.
Greg:You're absolutely right. I mean to a point you can do some of the mathematics because there is a whole matrix calculus where you can take derivatives with respect to vectors and matrices, and it does extend over. But as our models get more and more complicated, you're absolutely right. It just gets really really unwieldy even for sexy Hulk. And so what we can do is sort of feel our way over Round, let me give you an analogy. And I'm going to do it in terms of minimizing rather than maximizing, since we've earned our minus to log likelihood merit badge. Imagine that you parachuted into a valley at night, you can't see anything. But your job is to find the lowest point in that valley. But it's pitch black, you can't see anything. And so what do you do you, wherever you land, you take a step in all the directions around you, and you go, ooh, that's downhill. So then you move in that direction, and then you take a step all around, you go, ooh, and that's more downhill. And you keep going, taking incremental steps over and over and over until you believe that you have found that lowest point. And mathematically, we can set up computer algorithms that do a much smarter version of that, where they move their foot around a particular point that corresponds to starting values. And it doesn't just say, hey, things are going downhill that way, it says things like, it looks like it's really steep in that direction. So I'm not just going to take a little step in that direction, I'm going to take a big old step in that direction. So we have these different algorithms that sort of predict how far down it can go take a big leap in that particular direction, until it finds itself in a place where it sticks its toe all the way around and says everything gets higher around me. So congratulations, I must have reached that particular optimum,
Patrick:unless you didn't.
Greg:Other than that. Yeah, so with a particularly messy likelihood terrain, you might be very, very proudly sitting in this place, that's actually kind of near the top, I just found this tiny little lake up here, Oh, isn't this nice, when the minimum, the global minimum of this function is way the heck and down there. And with more and more complicated models, you have to be very careful about this problem. And what that means is ideally, parachuting into this valley and landing in a whole bunch of different places, and seeing which one of those ultimately takes you to the lowest point possible.
Patrick:And if you flip it the other way, if any of you are hikers or mountain climbers, I'm not a mountain climber, but I really liked hiking and did a lot of that in Colorado. And there's a thing called a false Summit. And you're climbing up and you see the top, and you get in your head that you're near the top, and you come out through the trees, and then the actual mountain is behind that,
Greg:just as you're about to yodel.
Patrick:So flipping that around to the minimizing, as you said, of getting into the little lake near the top is we call that a local minima as opposed to the global minima. And there are ways that we can protect against that. Fortunately, for a lot of models, that is not common. It is in other more complicated things, this is actually a real problem and mixture modeling. That's where randomize start values come from, and you do one or 2000 Different start values. So there are lots of ways we can deal with that. What we sometimes find is not so much a local minima, but at least in some of my work is it's what's called a flat spot. And that's where you get out on the likelihood. And there's just not a lot of change in any direction. And so you kind of look over here, and then you go over here, and then you go over here, and then you go back. And you're kind of back to where you were before, if you've heard the term is sometimes called a flat spot, very
Greg:technical term. In the sciences, we call that a flat flat spot.
Patrick:Exactly. So these are all different ways of saying how do we obtain a set of sample estimates of unknown population parameters that maximizes the joint likelihood of our sample data. That's what Fisher gave us in the field. Now, I will toss it back to you to say, Okay, we get a point estimate, well done you. But we need some kind of estimate of the variability of that picture to simple likelihoods around a parameter. Think of the egg that we talked about in the ordinary least squares, you can chart out a parabola. And the bottom of that parabola is the best possible fit picture two parabolas that have the same bottom, but one is a really steep parabola. And one is kind of a flat or parabola. It's just not as self confident. Walk us through the personality differences of those two parabolas. And how we can get an estimate of the variability of that maximum likelihood point that we derived
Greg:if we have found our way to whether we're talking about the top of the mountain No, no, right, right, fine, whatever. I'm gonna continue with my valley thinking about this as minimizing something right. If we think about it from an analytical standpoint, so sexi Hawk who finds out by setting where the derivative is zero, what that means is that the tangent to the curve of the function is flat, it has no slope. So that's how we find where the minimum is, you know, especially for a function that's easy to navigate. But just like Patrick said, it might be the case that the parabola just kind of lazily on confidently wanders away from that low point, as opposed to another parabola that is just super sure of itself. What that means is that that line, that slope that sits at the bottom that is perfectly flat, if in the lazy parabola, we move it a little bit away, the slope just changes a tiny bit, it just kind of goes up ever so slightly, in the confident parabola, that really tight parabola around the minimum, you move just a tiny bit, and the slope of a tangent to that parabola just goes shoots up way the heck up. Well, so how much the slope changes is the derivative of the derivative, which is the second derivative. And so by taking a second derivative, whether we do it analytically, which is how Fisher did it for simple functions, or we do it in some empirical way, we re chalk the heck out of that thing, and figure out how much change changes when you move, we get a sense of how stable our estimate is of a particular parameter. And that's going to be used to inform us as to a standard error associated with the parameters. So it is just gorgeous.
Patrick:It really, really is. And let's throw a couple of terms out there. So that when they come up at a cocktail party, you can be intolerably self righteous, because remember, that's a theme of this season, huge, huge goal, there are three terms that you may encounter when talking about this a gradient, a hessayon, and Fisher information matrix. All the gradient matrix is is the matrix of first derivatives. Alright, as we were talking about trying to find that bottom of the valley, the hessayon are the second derivatives. And as Greg just described, it's how fast are those first derivatives changing? No. So it's change in the rate of change, right? That's the hessayon. And then the Fisher information matrix, actually, is just minus one times the Halcyon. Fischer derive this as part of his early work that when Greg was at AC, DC, I was at Van Halen, he was inventing maximum likelihood, that is a matrix of second derivatives that has been multiplied by negative one. And what that negative one does is the second derivatives, by definition are negative, and it simply strips off the negative. And then the last one, you take the inverse of the Fisher information matrix, and the diagonal elements are the maximum likelihood estimate of the variance of the parameter estimates. Nice. What do we do with that we take the square root and make it a standard deviation. And that is our standard error. For every parameter, we estimate the square root of the inverse of the Fisher information matrix are our maximum likelihood standard errors. It is absolutely stunning,
Greg:gorgeous, and it just really makes me feel even worse about my 20s.
Patrick:There are a couple of interesting things to think about for traditional maximum likelihood. Now, I'm going to stress that because this can be applied in a lot of different settings for traditional maximum likelihood, we assumed that our variables are continuous, normal and independent. Well, why do we assume that for maximum likelihood? Well, continuity, we move up and down that number line to paint out the smooth curve? Normal, what we can't compute the likelihood if we don't know what the shape of the distribution is, or a fisher declares, I state that it is normal. And that allows us to calculate the likelihood. Why do we assume independence? Because we take these products of likelihoods to get the joint likelihood. These are the reasons that we make those assumptions.
Greg:So when Patrick said earlier that, you know we were going to put this curve over your head, it was decided it was decided what the shape of the curve was going to be. For reasons we actually talked about last summer, a lot of the popularity associated with the normal curve. A lot of observations in nature that subscribe to the normal normal curve was hugely popular. You know, if some other curve had been all the rage back in the late 1800s, early 1900s, then maybe different decisions would have been made, but the normal curve was chosen. And there are a lot of great things about the normal curve and as Patrick said, that serves as the foundation for so much of what we do,
Patrick:and there was more motivation for doing that, as you already alluded to, a lot of things in the world are normally distributed or near normally distributed. And given everything that we do in our day job, we're trying to make approximations to a more complicated system. And normality is not about approximation. But it is non trivial. When Fisher was doing these derivations by hand, on reams of paper, the normal is an easier, more tractable distribution to work with. So part of it is it does reflect a lot of what's in the world around us. But the other part is simplified the math at a time where you had to simplify the math, right? So for the moment, let's assume that we are meeting the underlying assumptions, when we meet those describe to us some of the remarkable properties that maximum likelihood gives us in these parameter estimates,
Greg:right? Well, the big three are unbiasness, consistency and efficiency. And the idea of unbiasness is that that estimate will not have a tendency to be to the left, it won't have a tendency to be to the right that as sample sizes get larger, it will be spot on. So it will be an unbiased estimate of the actual population parameter. Consistency means that the quality of that estimate gets better and better and better as sample size gets bigger. Another way of saying that is that your standard error start shrinking tightening up around that. And an efficient estimator means that it is the one that is the best bang for your sample size Buck like you can't get something with a smaller standard error for the price of that sample size than you can with a maximum likelihood process. So unbiasness, consistency and efficiency are hallmarks of the maximum likelihood estimation framework.
Patrick:And one thing that I love is all of those properties that are associated with individual parameter estimates also apply to compounds have parameter estimates. And you might say to yourself while you're cooking dinner right now, why would I ever make a compound estimate of multiple maximum likelihood parameters? That seems silly? Think about mediation, you have X to Y to Z, and there's an x to y coefficient and a y to z coefficient? How do we estimate that mediated effect, you take the product of those two parameter estimates? Well, if those are maximum likelihood parameter estimates, the product is also a maximum likelihood estimate. We take sums, we look at Conditional values, we probe interactions, we do this all the time. So this scales up in ways that are really quite remarkable. One way that we haven't talked about yet is not only do we get these wonderful parameter estimates and asymptotic standard errors, but we're able to do model comparisons,
Greg:I love model comparisons. And I love that that comes out of all of this. Now in the least squares world, we talk about model comparisons to we talk about a little bit differently, usually in terms of reduction in sums of squares, right R squared change, when we for example, in regression, add three more predictors, is our model better, what we're really asking is whether or not we have reduced the error sum of squares. And we have significance tests associated with delta r squared. And that's how we talk about model comparison in that particular world. In the maximum likelihood world, the idea is that if we have two models whose goal it is to explain why the data are the way that they are, the better models should yield an overall higher likelihood higher log likelihood, lower negative two log likelihood. And so I might have one model that has one will say negative two log likelihood for its observations, another model that has its negative two log likelihood for its observations. And I want to make comparisons between these two. Well, under the right conditions, conditions that Patrick and I have talked about before here, if these models are nested, if one is a special case of the other model, then we can actually test the difference between those negative to log likelihoods and that in and of itself becomes a testable quantity.
Patrick:And again, in getting an insight in where some of our terminology has come from historically, we talk about likelihood ratio tests. The lot of you have had this in class, you've done it yourself. You've read it in an article, and they say we use the likelihood ratio test to compare this and that. Well, that's what we're doing. We're comparing the likelihoods and one way that you can do that. And this is moving back and forth between exponents and natural logs, is you can take a ratio of two likelihoods and that is the law. Could ratio test. So that's where that terminology comes from.
Greg:Yeah, it's exactly right. You know, I don't tend to think about things as likelihood ratio tests, but absolutely they are. And you can even say, LR t if you're one of the cool kids. But I always think about it in terms of logarithms. So the logarithm of a ratio just becomes the difference between two things. And I like that because the least squares part of my brain thinks about differences in our squares or differences in sums of squares. In my maximum likelihood brain thinks about things in terms of differences between negative two log likelihoods. And that happens to be a nice metric, because that under the right circumstances can map onto things like chi square distributions, things where we know sort of how big that has to be to impress us.
Patrick:So let's think a little bit then about the Kelvin ball rules that Fisher came up with, right? You don't get this all for free. That's
Greg:Is there a Reaper coming? It's less of a Reaper and more
Patrick:like a bouncer? Okay. Everything that we've talked about so far applies under a certain set of conditions. A lot of these we've talked about somewhat implicitly a few explicitly, but let's think about the obvious ones. First, we assume independence, continuity, and multivariate normality. Those all have to hold because of what we talked about earlier, right? Those are obvious, a little less obvious, and one that has no real answer is we need a sufficiently large sample size. In order for those asymptotic derivations to kick in, I dare anyone to ask us what that sample size is. Nobody knows
Greg:it is the best kept secret in all of statistics.
Patrick:We often focus on sample size, just in terms of power. But we really, really need to pay attention to sample size in terms of have we got the ticket to get on the maximum likelihood ride. That is, we have to have a large enough n, where all of these properties come on board. But those are the obvious Calvin Ball rules. There are a couple of other trickier ones that are less obvious, that can really start to eat away at you. And one of the big ones is it assumes your model is properly specified.
Greg:Wait, what Didn't we say all models are wrong. But summary. Wait a minute. Yeah. So wait a minute, that's the fine print. So
Patrick:here's the fine print, we just described all the truly amazing things about maximum likelihood. And now we're going to go back to the office in the morning, and we are going to take our finite sample that is not normally distributed, and fit an incorrect model to it.
Greg:What could possibly go
Patrick:by I gotta go pick up the kids.
Greg:So for all of the beauty and elegance of maximum likelihood, and it is a workhorse, right? I mean, it's in everything that you and I do on a day to day basis. There is that fine print. And it means that there's an opportunity for a understanding how bad things can get, which sort of describes, you know, 20 or 30 years of simulation research. What happens if this isn't right? What happens if this isn't right? I'm sorry,
Patrick:that just described my dissertation. So thank you for that.
Greg:I knew that it did, actually. But then also an opportunity, how can we make it better? Right? How can we overcome these kinds of things? Whether it's, what if we don't have a super big sample size, even though we don't know what super big is? Or what if we don't have normality? Or? And this is really the tricky one. What if we have mis specification in parts of what we're doing that the model isn't completely correct. So how do we rebound from those
Patrick:vanilla ice cream?
Greg:You know, about hang on a sec, whatever you're gonna say after this, it doesn't matter for this moment. You were coming up to my house, and I wanted everything to be nice for your visit. So I texted your daughter and asked her what kind of ice cream was it that your dad likes again? And she said, Well, he does like vanilla. But you could also surprise him with something like a pretzel caramel something so I'll be damned if I didn't go to like six different stores looking for this exotic ice cream to have in the freezer. And when you came over and it was time for dessert, I opened up the freezer and I'm like, look at this and you're like oh, there's vanilla. That's all I need. Grab the vanilla. Pick. Damn it. I went to six stores to find that for
Patrick:the eyes remain styling way in a bathroom. So that is kind of my point. All right, last week was least squares and least squares as vanilla ice cream. This week is maximum likelihood. And what weirdo flavor do you get? I think Christie was just screwing with the I don't. She's good. There's very little in life that is more fulfilling than a single scoop of really good vanilla ice cream.
Greg:All right, how's that gonna save us
Patrick:ordinary least squares was vanilla maximum likelihood is that weirdo thing you bought that will probably be there next year, when I come up and visit. There's a time and a place for that right is maximum likelihood is a workhorse. And what we do anyone out there who does applied research, you've used maximum likelihood, whether you know it or not. But like everything, at some point, the bouncer is going to say enough. And he's going to grab you and pull your arm behind your back, which really, really hurts. And then he grabs with his other hand the color of your shirt, and then drives you out. And if you've been particularly egregious, he opens the door with your head. So
Greg:that was a lot of familiarity and specificity. Yeah,
Patrick:I've got the mouth of a 280 pound weightlifter. And I have the body of a 120 pound per sale swim. Oops. So at some point, the statistical bouncers open the door with your head, and you're gonna say I have a modest sample size, I have moderate non normality. I absolutely know somewhere within the confines of the model, I have a mis specification. So not only do these three things work independently, but they work in conjunction with one another. There are interactions between all of these things. You're rubbing your head, and you're sitting on the bench out front of the bar, and you're wishing that you had picked up your cell phone on the way out so that you could get an Uber home. And you say, you know why least squares was pretty damn cool. And I wonder if we couldn't reach past your fancy pants ice cream, and pull out what brought us to the dance and see if we can't design a version of that, that we can apply in these situations. Who do you know what's twice as good as least squares to stage least squares? Oh,
Greg:double vanilla.
Patrick:That's what we'll talk about next week.
Greg:I'm excited. I'm excited for least squares revenge.
Patrick:I love this stuff. We're going to post a couple of citations on the show notes. There's some really good tutorial resources on maximum likelihood. netural Wasserman Kutner has some wonderful stuff in it. Ken Bolin in his 89 book has a really nice exposition. That's a little more technical. It's not heavy. But if any of you want a really approachable, descriptive yet technically proficient discussion of it, one of my favorite is Craig Enders has a textbook on applied missing data. Totally. He has a brand new edition now. Yeah, in the first edition, chapter three is one of the clearest descriptions of maximum likelihood estimation I've ever seen. And so if you're interested in this, or you're looking to fold it into teaching your own classes, that's a really nice resource.
Greg:Totally agree. All right, well, so I am excited to talk about double vanilla next time. Thanks, everybody. I hope it was useful for you to understand maximum likelihood a little bit better, which is something that's likely going on under the hood and the types of models that you run.
Patrick:And we both hope that you achieved more by age 20 than the two of us did put together. It's not hard really, it's not hard. It has a low bar. Take care everybody see ya. Thank you so much for listening. You can subscribe to quantity food on Apple podcast, Spotify, or wherever you download your 1980's heavy metal music to help you relax and please leave us a review. You can also follow us on Twitter we are at quantity food pod and check out our webpage at quantity food pod.org for past episodes, playlists, show notes, transcripts and other cool stuff. Finally, you can join us at the d&d lunch table with your quantity and theme merch at Red bubble.com Where All proceeds go to Donors Choose to support low income schools, You have been listening to quantum tude where we've told every story and every joke we know. So we're simply going to start over at the top of the list. quietude has been brought to you by figuring out the new post pandemic normal in academia. Case in point. Today I had my first faculty meeting for the fall semester in which the meeting was via zoom followed by an in person reception. Oh, okay, by a new organizing framework for structural equation models that subsumes sem E, some D, some B some and M some into the maximally general WTF sound and by Twitter proudly replacing two centuries of peer review with a link to a preprint This is most definitely not NPR bom bom