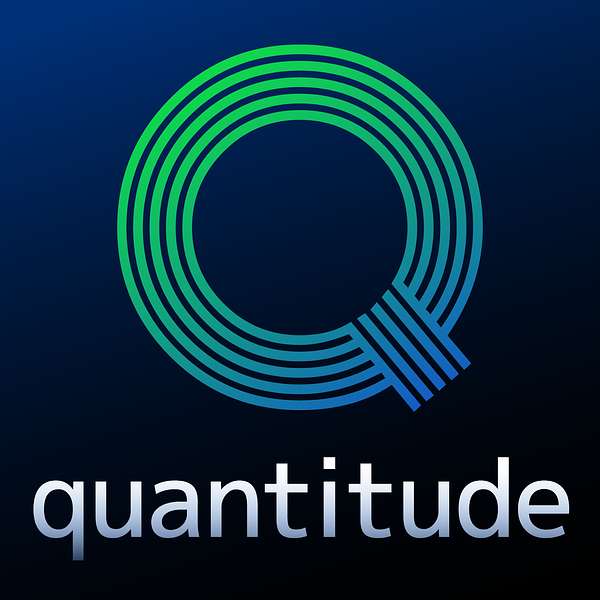
Quantitude
Quantitude
S4E03 Two-Stage Least Squares Strikes Back
In this week's episode Patrick and Greg explore the often neglected method of two-stage least squares; they take a walk down memory lane to explore its origins and then drag it kicking and screaming into the 21st century for much promising use within the latent variable model. Along the way they also mention magic dishwashers, being under-estimated, blind pigs & truffles, Sadie Hawkins, intellectual spinning hook kicks, Fisher's eight-pack abs, the fine print, Winston Churchill vs. Chewbacca, Guinea pigs, Mrs. Lincoln, butter snacks, and snipe hunts.
Stay in contact with Quantitude!
- Web page: quantitudepod.org
- TwitterX: @quantitudepod
- YouTube: @quantitudepod
- Merch: redbubble.com
Welcome my name is Patrick Curran and along with my never underestimated friend Greg Hancock we make up quantity dude. We are a podcast dedicated to all things quantitative ranging from the irrelevant to the completely irrelevant. In this week's episode Greg and I explore the often neglected method of two stage least squares. We take a walk down memory lane to explore its origins and then drag it kicking and screaming into the 21st century for much promising use within the latent variable model. Along the way, we also discuss magic dishwashers been underestimated. Blind pigs and truffles, Sadie Hawkins, intellectual spinning hook kicks, fishers eight pack abs, the fine print, Winston Churchill vs Chewbacca, guinea pigs, Mrs. Lincoln, butter snacks and snipe punts. We hope you enjoy this week's episode. Andrea and I have joked over many years that we each take one trip a semester. Just to remind the other one what we do. Andrea has said at one point that she for a number of years believed we had a magic dishwasher. Because it was just always empty. And the Patrick 3000. Exactly. And it wasn't until I was out of town for a couple of days that she realized, oh, it's not a magic dishwasher. But it made me think of a really funny research finding that's real. You have the two partners in a dyad. feel out what percent does each partner do in all the household chores? And you say which percent do you do? And which percent does your partner do? And I kid you not this is a real published finding. It sums on average to something like 158%. And it's really cool, because it's actually a starting point for marital therapy, which is you underestimate what the other person is doing. And it's kind of empirical. You can hold the two things side by side when you're working with a couple and say, okay, the percent that you each do in household management is 183%. It's just really interesting. It made me start thinking about not only the things you underestimate in your life, but the value of being underestimated
Greg:to surprise somebody with something that you got tucked up your sleeve. Exactly. Because then
Patrick:if you don't do it, they're not surprised. Right. So if you like you have no brilliant insight to share. They're like, yeah, no, I That's exactly how I thought about Patrick. But if you do somehow blind pig with the truffle yourself into some insight, as they say, Wow, I did not expect that. Yeah, exactly. One of my favorite examples of this is you and I both spent many, many years in martial arts. And for a few years, I did competitions and they're organized competitions are very structured. I trained with a buddy of mine who was much, much better than I was he was actually a fourth dawn in Taekwondo and hapkido and he would compete in the open divisions, which is any rank, any age, any size, and you just line them up and fight them. He had the most amazing strategy when he would go into these things. You've been to these competitions as well. And it's in some kind of Stadium. It's filled with mats all over the floor where all the different ones are going on. And there's this main center mat. Everybody is wandering around like a middle school, Sadie Hawkins dance. And what you're doing is watching your opponent's who you're going to have to fight sizing them up. Yep. And my buddy and I would start to warm up on the side and he would just warm up in awkward, horrible for deliberately, deliberately. The big thing is, is he would kick low and so he and I would trade kicks in a warm up exercise, and he would like never kick above my waist. While his opponent is watching this. Yeah, he bows in for the fight, the fight starts, you start to size up going around, he throws up waist level kick in the fight, does it a second time. And the third time knocks the guy out with the spinning hook kick to the head in his entire strategy was to have the guy underestimate him. I think that's something to aspire to. in life. I want to be intellectually, you think I can only throw a waist tie roundhouse kick, and then somehow have a spinning hook kick that you completely don't expect? That hasn't happened to me intellectually yet, but I keep striving for it.
Greg:Yeah, so you've been sandbagging for about 57 years, when it's your spinning Keep coming back to
Patrick:a point, you don't know. But you know, what does have a spinning hook kick is least squares estimation. Ooh, this is what got me thinking about it. So you and I, again, put a lot of preparation into today's episode. We talked last night when 10 o'clock. I think it was about 10 o'clock
Greg:after you emptied the dishwasher.
Patrick:So I put a lot of thought into this. And when I think about episode number one was ordinary least squares episode number two was maximum likelihood and maximum likelihood we'll review a little bit here today is spectacular. Maximum Likelihood is the workhorse that we use in nearly everything we do. But don't underestimate least squares. Because it turns out least squares has a spinning hook kick,
Greg:you think it's off on the side of the mat, just doing these low waist high kicks, just wait, just wait
Patrick:when you don't expect it. So I thought maybe what we could do is do a super brief review of least squares Gauss Markov, and what are the properties we get from that, and then talk about ml similarly, and then say, well, under what condition might we kind of want to go back to the table where least squares is sitting because we didn't realize they had a spinning hook kick. So let's start briefly with least squares. So we talked about this in episode one of this new season. And what we're trying to do is identify an optimal linear combination of our set of predictors that accounts for the maximal amount of variance that we observed in our dependent variable, we typically are going to assume continuity of the dependent variable. In old school, ordinary least squares, we don't have to assume normality. But we do have to invoke that assumption to get standard errors. Alright, Gauss Markov gives us the Kelvin ball rules that we have to meet in order for least squares to be the best Linear Unbiased Estimator. So we talked about that in the episode is that under assumptions Oh, well, less is blue BL UE best Linear Unbiased Estimator. And we won't go through the assumptions in detail again. But we have things like continuity normality, that the distributions of the predictors are fixed in known, what that means is their distribution free for the predictors, but we are also assuming that there is no measurement error. And then we get into some tricky ones, the model is properly specified. And one that I don't know about you all out there who have taught this I routinely sweep under the rug and pretend does not exist, the covariance between the predictor and the disturbance. Alright, so let's call the residual and our least squares regression model. What should we call it? r sub i? Okay, so picture in your mind's eye, the covariance between x sub i and r sub i equals zero, right? Just standard one. We make that assumption all the time. I never talked about that. I just say, yep, that's an assumption under Gauss Markov. Well, we're going to pick away at that a lot today of well, what if there is a nonzero covariance? Where does that come from? What do we do about it? And so anyway, if you meet Gauss Markov, there is no better set of estimates that lead to a smaller sum of the squared residuals than your OLS estimates. So that was all of episode one. I like it. Well, we could have done that a whole lot shorter. Then we said, Wait, who's that flexing in front of the mirror over there? Ra Fisher.
Greg:Fisher unopposed down?
Patrick:Yep. And we, we decided that your achievement in your early 20s Was you grew an orange mustache, which I have to admit I had a nightmare about. And my achievement was I saw Van Halen with David Lee Roth and Sammy Hagar, and RA Fisher at that same age invented maximum likelihood. So give us a whirlwind tour of maximum likelihood,
Greg:I just keep picturing Fisher, like flexing in the mirror is eight pack abs
Patrick:and an orange moustache.
Greg:Alright, so as we talked about last episode, maximum likelihood looks at things from a different perspective, as you would say, it's Calvin Ball, it changes the rules on you is very, very clever. The main theme of it is that you want to try to figure out what the parameters would have to be like in a population to maximize the likelihood of the data that you have in your hand. And there are, as we said, rage hoc ways of doing that. Where you just sort of turn the computer loose to try to find those things and then There are elegant, sexy Hulk ways mathematical ways to try to derive those six. But they rest on some particular assumptions. And one of them right out of the gate is that the observations themselves are independent. And that's because in order to characterize the likelihood of all of the data, we take the product of the likelihoods for each of the individuals. And you can only do that if those things are independent. We also in order to figure out a likelihood of anything, we have to have some distribution assumed, I can't tell you how likely a particular case is unless I know the distribution that that case is a part of a normal distribution, or more generally, a multivariate normal distribution is what Fisher primarily had assumed and what serves as the basis for a lot of the things that you and I do. So there's this baked in distributional assumption about multivariate normality. That gives us the ability to figure out what the likelihood is of each observation within that distribution. The assumption that I can't get rid of in maximum likelihood is something that you just alluded to that is that assumption that the predictor is independent of the error. I can cast that more broadly as the assumption that the model is right. Anytime anybody says, Yeah, well, you know, assuming the model is right, there should be a little bell that goes off in your head that says, Wow, wait a minute. What do you mean, assuming the model is right? There's a lot of stuff that goes on in these models, when we are doing maximum likelihood for something small, like a regression, or something a little bit more involved, like a logistic regression or something that is much more full blown. Whether it's a structural equation model, a confirmatory factor model, a mixture model, there are more and more opportunities for some aspect of your model to be wrong, we know that there are aspects for our model to be wrong, because we often consult modification indices to help nudge us toward truth, as if that's a thing. But it could be that their error covariance is missing, it could be that there are cross loadings missing, it could be more along the line of the relations themselves, the functional form of things where you think that it's a linear relation, it's not really exactly linear. There's so many places where a model can go wrong, and maximum likelihood is assuming that you got every single aspect of it, right. Because if that doesn't happen, then all of the beautiful things, the holy trinity of unbiasness, consistency, and efficiency, those start to get very, very unstable. This, I think, is something that we really need to poke at this Achilles heel about a model being properly specified. And maybe where that estimator sitting off on the sideline, doing low kicks can come in and help us out.
Patrick:We're going to talk a lot about Ken Bowens work in this area. And he has a wonderful line that I like, which is the fine print on maximum likelihood, we all focus on exactly what you said, maximum likelihood is a full information estimator that provides consistent, unbiased and efficient estimates that are asymptotically normally distributed, so that we have standard errors. And we have test statistics. And then Bolin talks about the fine print, that's when you have sufficiently large samples in order for those properties to kick in, that you have normally distributed disturbances, and that you have a properly specified model. The extent to which any or all of those are violated actually undoes all of those wonderful characteristics to varying degrees, right? That's right, it doesn't take it out back and hit it with a shovel. But we can no longer brag about those characteristics of maximum likelihood, think of you out there in your own work. Do you have an asymptotically, large sample? Do you have multivariate normal data? And do you have a properly specified model? I'm going to guess that a whole lot of people are saying, Yeah,
Greg:only when we simulate, I think that's the only
Patrick:part of the problem is is there's a whole lot of simulation work out there. That shows how amazing maximum likelihood is when you are under these unrealistic circumstances. Now to be very clear, there's an entire body of work, some of which you and I have contributed to, to varying degrees, that is looked at a thing called robustness. Well, to what extent is it robust to non normality? There are conditions where you say, no, there is a mis specification, but we're still close enough for government work, we can still use it. They're spectacular ways that we can correct for non normality that we can get robust standard errors and robust test statistics. So this is not a we have to do away with maximum likelihood. Here's this least squares guy sitting by himself at Sadie Hawkins. Alright, for those of you who are outside of the United States, Sadie Hawkins, is this God off? fourth thing that schools impose on preteens, it's usually in middle school. But Sadie Hawkins is this awful like Shark Tank of pre pubescent kids wandering around this room. But here is least squares estimation. And what Ken Bolin said is Hey, guys, we've been working with this stuff for 70 years. What if we apply it to the SEM. So what I would like to do is getting our Wayback Machine and avoid the temptation of bringing in Churchill. Alright, I'm still on volume three of Churchill's biography,
Greg:good that you didn't bring that in.
Churchill:We shall defend our Island, whatever they cost may be. We shall never surrender.
Patrick:I want to get in the Wayback Machine, not invoke Winston Churchill, but talk a little bit about the historical developments of what we're going to call to stage least squares. And then Bolin in 96. He kind of indicated it with a paper in 95, but really hit a ball into play in 96. Saying, we can take these really old approaches and apply them to a multiple indicator lighting factor to address some of the very things that we're talking about. Yeah. And then we could talk maybe at a 30,000 foot of what is to stage least squares within the SEM, because you could do a grad level class on that topic. But how does that sound as a storyline?
Greg:This is a storyline I like the idea that least squares has been lurking in the background and laying in wait for its vengeance. So this is good, please square strikes back or whatever we would call this.
Patrick:So I had to work at Churchill reference in and now do your Chewbacca, and we can just get it out of the way. Yep, there we go. All right, everybody, well mark that off on the list. So should I start?
Greg:Yeah, I'd like to know about the history of two stage least squares. All right, very briefly,
Patrick:picture in your mind's eye, everyone a predictor X predicting y. And we're gonna have a regression coefficient, let's call it gamma. Greg and I talked Two episodes ago, the least squares estimate of that is going to be the covariance between X and Y divided by the variance of x. Now that is unbiased if the model is properly specified, Gauss Korps said, one of the assumptions is Cove x, or where r is the residual equals zero. That's what we just talked about a few minutes ago. But now picture in your mind's eye that doesn't hold. So picture it is a path diagram is we have X predicting y. But what if that's violated? Well, we also have X predicting our econometricians have been talking about this for a century. It's called an endogeneity problem that x is both related to y, but also to the residual.
Greg:So here's the thing that I want to clarify, you know, whenever I run a regression, just I mean, like push the button or coded in whatever run a regression, I get out residuals that are uncorrelated with my predictor, I literally am subtracting things out and saying, Hey, this is the stuff that's left over, that's unrelated. So when you and I are talking about having predictors that are related to the residuals, correlated with the residuals, structurally impinging upon the residuals, or mutually influenced by something outside the system, what we're talking about is on a theoretical level, there are things that are contained in the residual things that are not x that are related to x. So this is an issue that is at a theoretical level, even prior to being able to do any kind of analysis, whether it's regression or something else.
Patrick:It's a byproduct of the estimation, right? Yes, the mean of the estimated residuals are always zero, they have to be, and the predictors are uncorrelated with the residuals, they have to be there's a circularity, the reason they are is we're making that assumption, right. But that's just a sample base. So you're exactly right, we have x predicting y, and then picturing your mind's eye in a path diagram, X also predicting are all right. So that's the endogeneity problem. We're assuming that's not there, but pretend that it really is. And we'll talk in a moment about how that can happen. Now picture omitting that effect. All right, and we do our regression in the usual way. Well, that gamma now is going to be the covariance between X and Y divided by the variance of x. That's exactly the beta like we usually have, but two that is added the covariance between X and R divided by the variance of x that's being absorbed in that single gamma. And that's the bias that we get. So if you have X predicting y and you get a gamma in your sample, if you have an endogeneity problem, that regression coefficient is going to be biased. Yeah,
Greg:this ties back beautifully to two things that we've talked about before. One is path tracing, everything that you just described is straight up path tracing, if you have the connections that you have there, those relations would fall right out. The other thing, which you raised years ago, is whack a mole. Yeah. And the problem that you have here is that when you're cutting off one avenue for AIX to be related to why, what's gonna happen, right? And the answer is, if anywhere else can try to compensate for that it's going to happen, and you've got one place for it to compensate. That is in the direct XY relation,
Patrick:but we've determined all of life as Whack a Mole. But setting that aside, in econometrics, this has been dealt with for nearly a century. Sure, this is old school stuff, we just tend to ignore it in social sciences. There are many ways this can happen. There are three big ones, and they all involve misspecified models to varying degrees, right? One is there's reverse or reciprocal causation, right? And we do this all the time. Is stress related to depression is depression related to alcohol use, I'm gonna say depression predicts alcohol use, okay, so you're not saying alcohol use at the same time, influences depression, so it's omitted reciprocal relations are you blown the causal direction, the other closely related when you have omitted variables, right, this is just old school that you say depression predicts alcohol use, but you've actually have a fundamental causal predictor. And this goes back to Gauss Markov, who gave the less than helpful guidance that the important variables are included and unimportant or excluded. That wasn't their exact wording, but that's a paraphrasing. Alright, so omitted variables. And then the third one that we could delve in an entire episode, and I'm just going to kind of set aside for now is that the independent variables have non random measurement error that we often assume that measurement error is random, is you're just as likely to be above as below as you step on and off your crappy scale. But that it's non random, that somehow the measurement error on the predictor is systematically associated with the dependent variable
Greg:for me of the three of those, it's the middle one that I think is the most worrisome on the first one having to do with reciprocal causation, I generally prefer that that be unpacked and not cross sectionally. But longitudinally, so maybe it's possible to do an end run around some of that by modeling it. And then the thing about the non random measurement error, I just want to avoid that here, because it's a lot for us to do. But the whole idea that your model has all the relevant variables on the table, that's a pretty big pill to swallow. Right. And so I think that's the main one that that we want to deal with right here. Alright, so
Patrick:those are the reasons why we may get this covariance between your predictor and your residual. All right, well, you know, what we're going to bring indirectly back guinea pigs, ah, Sewell right path tracing guinea pigs. His dad was a heavy hitter in this area in early developments of what became to stage least squares, wow, not suitable, right? But Phillip, right? I am. And Philip, right, initially incorporated what was called an instrument, alright, an instrumental variable. And this is the cornerstone to two stage least squares. So what it is, is, if you have an X predicting y, we're going to stay super simple with just a single predictor, and all of this scales up. And instead of the covariance between X and Y divided by the variance of x, we simply scale up to x prime x inverse x prime y and everything goes in our usual multiple regression, it's just easier to think about for a single predictor. Alright, so everybody pay attention, okay, because we need a quick definition that there's a lot of confusion about an instrumental variable is an additional measure. This is what you were alluding to with the omitted variable. This is bringing in to your x to y relation, for lack of a better notation, let's call it Z, we're going to bring in an external measured variable that is uniquely related to x the problem predictor, but it is not related to why why it's not a shocker that this came out of the Wright family. This is actually an indirect effect. It's a path model. It's a path model, we have z predicting X and X predicting y in this initial model. Now, we're not going to actually estimate it that way. What we're going to do, are you ready for this? This is the spinning hook kick from least squares. If you have an instrumental variable that is related to x, but not related to y, in stage one, we're going to use Z to predict X and we're going to save out the predicted values x hat, okay. Now it's important we're not saving up the residuals that actually under So the whole thing, we're getting x hat, that's stage one, okay? Stage two is instead of using x to predict y, we use x hat to predict y. That's the second stage that removes that relation between x and the residual. And when assumptions are met that x hat to y regression coefficient gamma that is unbiased. And that addresses that endogeneity problem. It's very clever. Oh, it's clever as hell, the biased regression coefficient is facedown on the mat saying What in God's name just happened. My final competitive fight, I was very fortunate. I made it to the finals. I survived a couple of minutes. And at one point, I found myself on my hands and knees. The ref was counting to 10. My coach was screaming at me to get up. And my only thought I had was I never saw it. And that was my last competitive fight. So gamma is facedown on the map. No, that was 1928. That right was writing about this. All right. And he developed this stuff in the teens and early 20s. People picked it up in the 1940s. And then big people who are identified with this as baseman in 1957. Thiel in 1971. So what Wright was trying to do, and this is another great example of having a substantive problem that just needed a solution. Right, I was trying to understand a supply demand curve for butter. All right, so this is back in the 1920s. He was an econometrician and he wanted a supply demand curve. And he wanted to use regression to get that. And the problem was there was reciprocal relation between supply and demand. So you have this endogeneity problem. But he went out to find an instrumental variable, and he selected amount of rainfall as the instrumental variable. Right? Well think about it, because a lot of this stuff that's so fun is, well, what is an instrumental variable? How do you determine what an instrumental variable is?
Greg:How do you find it in the wild, right?
Patrick:Well, not only how do you find it in the wild, but how do you pick one even conceptually, that would be related to the predictor, but not the outcome. Think about the logical syllogism his thinking was, rainfall is related to grass growth, grass growth is related to milk production, milk production is related to butter production. And so rainfall is related to the supply of butter, but it's not related to the demand for butter, you can make a reasonable argument that you aren't more likely to buy butter if it's raining.
Greg:Oh, come on, in out of the rain Kids, let's have a snack who wants a fresh stick of butter.
Patrick:But isn't that clever, very clever. Rainfall is used as an instrumental variable for supply, he was able to address the endogeneity. And he was able to achieve what he was trying to do with the supply demand curve. These scale up their whole decades of work on this, we're not going to belabor this. But this is kind of a workhorse in econometrics. Also in fields like sociology, where these things come up, there's a wonderful paper in 1978 that introduces two stage least squares to psychological researchers. Notice we haven't even gotten to the SEM, this is our Wayback Machine. This is realizing Holy crap. Least Squares has a spinning hook.
Greg:What I like and don't like about that is that information had been sitting around for a long time, right? But in the econometrics world, and then it's being ported over into the social sciences, like Hey, Guys, wake up, this thing exists over there, the same thing was true of his son's work, a lot of the path analysis stuff that didn't start finding its way into sociology, and the other, the other ologies until the 60s and 70s. So we're finally getting some of the cool things that were going on there. But actually, there are things over in that world that has ignored stuff that we have, right in our world, we talk a ton about measurement error and latent variables. And most of the examples that I see from the econometrics world deals with things that are measured. And so it feels like there is this union waiting to happen between the information that we have in our world about dealing with things that are fuzzy that have measurement error, and their world about having instrumental variables. Getting all those in the same place seems like it would do 180% of the work or something right, that it wouldn't be able to come together to be more than the sum of its parts
Patrick:joining. Together, we can move a galaxy. So I think there are two points at least I'd like to distill down to and what we've talked about thus far is first, if you teach regression if you apply regression in your own kind of work This is something really important to think about. If you have reciprocal relation, if you have omitted variables, if you have this non random measurement error, this is big ticket stuff and you get bias. So one is know this exists to remember least squares has a spinning hook kick, so stay the heck out of its range, right? You and I were messing around once a number of years ago, because you're a black belt in Judo, which for people who don't know, is primarily a grappling defense. My training is in taekwondo, which is primarily kicking my zone of comfort is about eight feet away from you. And you and I were kind of messing around in the backyard sparring, and you kept coming in and, like touching me, and I'm like, dude, back up. I can't kick you. If you're spinning me over your head like this.
Greg:Could you please back up so I may kick you I was very
Patrick:polite about it. Okay, point being recognize these problems exist recognize that there are approaches that can handle it. Respect least squares has a spinning hook kick, butt where we're going to pivot now is Boland woke up one morning and said, Oh, damn, I got an idea. And that's where we're going to go into two stage least squares for SEO. But first, I have to go to the bathroom. Okay. Okay, I'm back.
Greg:So now that you're about 14 ounces lighter.
Patrick:So at some point, I get tired of talking about bowling. Right? It's like bowling this bowling that. And you know what particularly pisses me off with him. He is so hard to dislike. He is a decent human being. He is kind. He is supportive. He is enthusiastic. He's in the game for the love of the game. And so when you have somebody who has done so much for the field, you really want to dislike them. Oh, and he
Greg:seen Jimi Hendrix in concert? No way he has. Yeah, he told me about
Patrick:it. I did not know that. Yeah, how do you top that? That's pretty cool. Alright, in the early 90s Ken, who is a card carrying sociologist and trained in demography and coming up through that substantive lens was fully aware of two stage least squares and all that it had to offer. But one morning he woke up he had this incredibly clever idea that you could take that two stage least squares method and apply it within the SEM. Alright, so we're gonna put all of these citations on the show notes. He has a 1995 paper where he Telegraph's this, but he has a 1996 paper that was really hitting the first ball into play, it was in psychometrics. And it's called an alternative to stage least squares estimator for latent variable equations. And it's a wonderfully written very accessible especially for psychometric a very accessible paper. He has written I haven't counted but maybe a dozen papers that have contributed. He and Albert may do Olivares did one for Pollack, Oryx, he and I did a few with our research group and looking at simulations of robustness and applications comparing to ml. But what's really neat is he's got a couple of very recent ones that I would highly recommend. One is 2017 and multivariate behavioral research, which gives an overview of this, and then a 2021 that he wrote with a number of his colleagues and students that's in psychological methods. And this is it takes a village kind of thing is Zach Fisher, and we will talk about Zach's work in a minute. He was a grad student here at Carolina worked with Ken is now an assistant professor at Penn State. Zach has written packages in order that automate a lot of this stuff. Michael Shear Dano also a grad student, Adam Lilly, Llandeilo IEA, that's in the psych method. So I highly recommend these. Let's pan back a bit. All right, we could do and I'm not exaggerating, we could do a graduate level course in two stage least squares estimation and all that's involved with that. We're not going to do that here. This is the 30,000 foot. The pilot says if you're sitting on the left side and you never are right, you're always on the right side. If you're sitting on the left side, you can see the Grand Canyon. If you're sitting on the left side, you can see two stage least squares that can be applied to FCM. That's what we're gonna do here. What he does, and he's a wonderful his 2017. I had read it before, but I reviewed it again. Last night, his opening line I love this is universal agreement is a rarity in life. Isn't that a great hook?
Greg:That is awesome.
Patrick:The second sentence is, and so it is among scholars using structural equation models. Okay, if that does not hook you in reading the paper than there's something wrong with you, I think that's a great opening. And he goes in, and he trumpets the advantages of maximum likelihood it's full information. It's simultaneous estimation. It's asymptotically, unbiased, consistent, efficient, normal distribution, there is no better game in town. Unless you have smaller sample sizes. You violate normality. And your model is misspecified. But other than that, Mrs. Lincoln, how did you like the play? And then he goes into what is it? What does it have to offer and all the things like that? The remarkable insight, at least in my opinion that Ken had in this whole thing was all the two stage least squares estimators, up till that point required an external instrumental variable. You alluded to that a bit ago in the conversation, assuming that didn't get cut, it depends who edits this, it'll get cut. If I edit it, it won't get cut if you edit. So we'll see. It was brilliant. It was brilliant. Alright, sometimes those are called auxilary. instrumental variables, those are just external things that we bring. In my opinion, this is one of the things that has led to the limitations of use in at least Behavioral Sciences is it's really hard to find these instrumental variables. Not only is it hard to find one that meets the requirements of being correlated with x, but not with y. But it's not like a bunch of us have these variables laying around that we don't consider. Yeah, gosh, I totally forgot about this predictor variable, right? We have a million dollar data set, and we're using everything that we have available. It's a bit easier to do that. Not easy, but easier in econometrics, because you can look at things like oh, the National Weather Service has rainfall rates for these different regions, and I can link those to where my regions are that I have butter production. So that is the old school to stage least squares. So auxilary Ken's Insight was we can rewrite the model where we have model implied instrumental variables missives, right, we have to have acronyms, and we have to have acronyms that we can pronounce. Unlike Bowers move. Model implied instrumental variable Am I V is mivv mivv, to stage least squares estimation. Everything we've talked about up to this point still applies. But instead of having an external variable we bring to bear, we are able to identify instruments that are implied by the model that we can use equation by equation. And that's the cornerstone to mivv sem
Greg:crazy clever that it was sitting right there in front of us all along within our latent variable model, right in our latent variable model, where we have multiple indicators of each of our latent variables. And then the latent variables themselves have some kind of structural relations among them, which is really what we usually want to test in those kinds of models. And so the question is, how can we deal with this instrumental variable problem and still maintain the integrity of the assessment of the structural relations among the factors? Well, that's what Bolin helped to show us this is really clever, it is incredibly
Patrick:clever. Again, we are not going to teach you how to do mivv to stage least squares, sem isn't a greatest actually, there are three acronyms that you use is it's May of two SLS SEM,
Greg:which takes us long to say as the words actually
Patrick:can lays out in his work. And when I say Ken, a lot of it is on his own, but also a lot of collaborative work with colleagues and students and postdocs and junior faculty. And so I'm just gonna say, Ken, and I mean kind of a whole group, but there are five core steps that he lays out in this. Alright, so we're talking about latent variable models. So we have latent factors that are multiple indicators. Step one is what we do in all of it, right? It's the rap that I want to have for all of SEM, which I won't do it because it will just be horrible
Greg:do it.
Patrick:Specification, but identification, estimation, evaluation, re specification, interpretation, interpretation, interpretation, interpretation.
Greg:Well, Patrick did not authorize that rap, he does understand that there are risks associated with letting me edit the episode.
Patrick:Those are the six steps of any sem that you all should have at your fingertips. Step one in mivv to stage least squares Sam, is you specify them up so all the usual ways. Alright, is it a CFA do we only have Leighton covariances in SEM, do we have structure? What items load on what factors? Do you have correlated residuals draw your models for whiteboard problem, there are two ways that we can set the metric of the latent factor, you can either standard isolating factor, meaning that we set the variance to one and the mean to zero and then you estimate all your loadings and all your items and intercepts. Or you use what's called an indicator variable, you pick one indicator, and you set the intercept of that to zero and the loading to one that sets the metric of the latent factor. And then you estimate the mean and variance of the latent factor, those two are equivalent, you get the same log likelihood you get the same standardized solution. What's really important in two stage least squares is you have to use an indicator variable to set the metric and only works if you do that, yes, the second step is where the really clever part comes in. And it's called Transform latent to observed and can does T L to O. At this point, I think he's just messing with us, what you're going to do is we have these latent factors, we are going to reexpress the model as a function of the scaling indicators, minus their errors. It takes the latent variables out of these equations. But here's where he was so freaking clever. When you do that for a system of simultaneous equations, you build in endogeneity. It is your fault. You have built into your model on purpose, correlations between certain predictors and certain residuals, right. That's the point. That's what he does intentionally. So you reexpress your latent variables in terms of what you observed. That's step two. Step three is what's always been the hard one is you go on a snipe hunt. I don't know if anybody's familiar with what a snipe hunt is, but it's an old gag is if you go camping with somebody new and you send them to go catch a snipe for dinner. And snipes don't really exist. But you sit around the fire drinkin beer, and they're out in the woods trying to catch something that doesn't exist. That's what a snipe hunt is. Hmm. Step three is you got to find the model implied instrumental variables and Ken describes how we go about doing this how you identify it. Zach Fisher, who we're going to talk about, Zach is one of my favorite people. And he is an assistant professor on the quant program at Penn State now. And he wrote a really powerful accessible package in or cleverly called MIB sem that automates this, but you have to identify these model implied instrumental variables once you get the instrumental variables, dude, x prime x inverse x prime y, all of this complexity that we're doing, you regress your problematic covariates on your instruments you say about the predicted values. And then in the second stage, you use the predicted values to predict the dependent variables, because you have unhooked those correlations between the predictors and the residuals. It is x prime x inverse x prime y, you do it equation by equation, and you get the estimates. And then I don't want to delve into this too much here. But the fifth step is, if you have an over identified equation, meaning more instruments than you need for estimation, there's a thing called a Sargon test, where it gives you an inferential test of whether the instruments are working in the way that they should. So if it's a non significant test, the characteristics of the data are consistent with that your predictors are uncorrelated with the residuals, if you get a significant test that is violated, which actually implies there may be a mis specification within the model. And that's
Greg:incredibly powerful. Because the modeling that you and I are accustomed to doing is generally very global, right? We assess the fit of the model globally, and we go, hey, it's working great, or oh, it's not working so well. And then maybe we use modification indices to try and figure out what's going wrong. We don't take a very local perspective, in practice, unfortunately, the beautiful thing about this strategy is that you get a whole series of equations out of this where you can assess the fit much, much more locally, you know, oh, this is locally just identified. This is locally over identified great, how's the fit in this over identified circumstance. So the idea of being able to pinpoint what parts of your model are working well, and what parts of your model are not is hugely important, I think from a diagnostic standpoint, and it's something that we just don't have a good handle on in the maximum likelihood world. So I love this aspect of MIB tools, some some,
Patrick:on top of that, that equation by equation estimator that you just described them, the tests of that, that actually is gonna give us a huge advantage under a lot of circumstances. Not always, but under a lot of them. And that is, you know, what it protects against Whack a Mole. Yeah, let's think a little bit about what are the advantages? What is can argue for why you would go this route? Because again, ml, man ml lifts weights, ml can dance. ML is funny. ML is not hard on the eyes, I'll tell you that. Why would we not do it? Well, this is that fine print. Yes, ML is the game in town, when you meet those assumptions, when you don't, that directly undermines all of those goodies that we brag about when we use maximum likelihood. That's right, it is not asymptotically, efficient, consistent and unbiased, when you have a misspecified model.
Greg:That's right, we can tinker with the non normality problem, we can overcome some of those things. But the structural problems, that is huge and maximum likelihood is not going to help you fix them to stage least squares helps you with that. It doesn't cure
Patrick:it, but it really gets you quite a ways. And we did some early simulations, Canon, myself and some of his grad students, when you apply to stage least squares in the way that we've described. And of course, there's Calvin Ball rules that apply to two stage least squares, right. And it's not spring break in Orlando with estimation. I mean, you have to invoke assumptions on that two stage least squares with MIBs, when done properly, is also consistent and unbiased and ask them topically normally distributed, which does give us standard errors, we can get analytic standard errors, but Zack put into this package where you can also get bootstrap standard errors. And those seem to do well. Here's a big one myth to stage least squares is non iterative. Oh, yeah, sure. It
Greg:makes sense. Right? Exactly right,
Patrick:it makes sense. Because it's x prime x inverse x prime y, all you got to do is be able to invert x prime x. And as long as you don't have a linear dependency in your columns, you need one more person than predictors. Now, we're not advocating that. But that allows you to invert x prime x, it's non iterative, we'll think about all of you out there who are working in smaller sample sizes, you have maybe a little bit more complicated models, maybe you have some mis specification, but you're not sure where it lives. And models are better than when you and I came up through the ranks non convergence was just a routine problem for you and me. And it's much better now. But it still exists, you get a model where ML does all the hill climbing and valley searching that we talked about last week. And it comes back to Greg and me and says yes, sorry, dude, I can't do it.
Greg:And let me give you an error message that is not helpful. Yeah,
Patrick:in all caps as if I'm yelling at you. But it's non iterative, you always get a solution into stage least squares. Yeah, here's maybe the most important part. In my opinion, we've talked a lot about Whack a Mole. And we've talked a lot about western Kansas. Remember, you have some misspecification, out in western Kansas? And you say I, it's not going to bother me, because it's way over there. But that can propagate through your system of equations, because the biggest advantage of maximum likelihood is it's a full information estimator. And the biggest limitation of maximum likelihood is it is a full information estimator. Exactly. Right. The term that I love that can use is is to stage least squares can serve to quarantine misspecification. There, it does not do away with it. All right. If you have a system of equations, and you have a particular equation, and you've misspecified that, yeah, tough crap, no estimator is going to fix that. But because it does it equation by equation, it drags barbed wire around that Miss specification, and it doesn't allow it to propagate to other parts of the model. That's a huge advantage. It is.
Greg:And my recollection is that even in models where it can't fully quarantine it, if you think about a latent variable model, where each of your latent variables has its own little measurement model, and then what you're really interested in are the relations among the latent variables in that structural portion, even when you can't completely quarantine misspecification associated with the measurement models, that you still get pretty darn decent estimates of the relations among the latent variables. Is that accurate? That's exactly
Patrick:right. Only on there was some hope that it would completely quarantine. Now, it's just a little bit gentler language is used in the way that you just described, it minimizes the propagation of misspecification to other parts of the model. Over the years, a lot of people in my opinion have been rather dismissive of two stage least squares. And the reason is, it is a limited information. What does that mean? It means we do it in two steps. They really tried to hide that in this method of estimation by calling it to stage least squares. It's limited information, we do the instruments as predictors. First, we go get a cup of coffee, come back. And then we do that second stage. And I heard one person make a joke about this at a conference, they said, Oh, it's two stage, who cares?
Movie Clip:Laugh it up Fuzzball.
Patrick:It does have lower power. Alright. limited information have lower power than full information. But it's can really nicely describes. That's only when regularity conditions are met. Sure, with the full information, right, it's like the circularity you keep going through around. Yeah, maximum likelihood is like flexing down on Venice Beach about all the things you can do, but only under regularity conditions. There are more and more simulations that are indicating that when you compare these estimators under conditions that we typically encounter in the kind of work that we do, either there's no difference in power, or there's a slight decrement in two stage least squares to a point that nobody cares about. But then you have to balance with, okay, I'm having a little bit lower power, but oh, by the way, my regression coefficients are less biased than yours are. So you are much more likely to find a significant effect for your biased regression coefficient than I am for my unbiased regression coefficient, you have
Greg:more power for your wrong thing exactly go you.
Patrick:We are skimming over the surface of a very deep lake in all the interesting things that can be done. But one of the big points we want to clarify here is one is remember least squares has a spinning hook kick and never ever forget that. So just respectively squares, and know that when maximum likelihood is rubbing baby oil on its muscles and flexing in front of the mirror. That is under conditions that are rarely if ever encountered in practice. Yeah, so that's point 1.2 Is oh my gosh, if you're quanti, and are looking for a dissertation, and our 21 application and our row one and NRSA to get some pre doctoral funding, the ground is littered with shiny objects to go pick up and look at what do you do with missing data? How do you extend this longitudinally? How would you extend this across multiple groups? This is a fertile ground for novel generative work. And Ken is really wonderful in both his 2017 and also 2021 paper, he identifies a lot of these things, not only are there analytic developments that can be done, there are simulation work that can be done. But there's also a real opportunity for substantive dissemination work. And so if your quantity but in primarily a substantive field, doing a compare and contrast, and how would you do this, Zack Fisher, Zack is awesome in collaboration with Canon as group but Zack really was the workhorse on this. This is Zach's package, he has written a package in order that automates everything that we've just talked about. He has used notation that mimics Lavon. So if you know with Lavon, you can do this, but the package is called MIB SEM, we will put a link to it on the show notes, he's written a paper, there's the usual documentation on it. And so well done. Zakat is elegant, it's beautiful, it's efficient, and I highly recommend you look at that.
Greg:Most impressive, that's huge to be able to put this in the hands of the applied user. And what I hope that we have done and when I say we it's like a lowercase w here, because you've done the lion's share of the work here, which I appreciate. You've opened the door for people out there, we don't expect that at the end of this, you understand all the nuances associated with MIB two as socialism, but that you know that it is there and you say to yourself, oh, you know, I have some of these problems. I have some concerns about this, this is an option for you. And we hope that you might choose to open that door and go in there yourself and find this very, very useful tool for your modeling needs.
Patrick:And you know, what I'd love to see a lot of the literature up to this point has been to stage least squares as a replacement for maximum likelihood. There is no reason at all that you couldn't estimate your model using maximum likelihood estimate your model using two stage least squares and compare and contrast the results as another form of sensitivity analysis. Yeah, which conclusions are robust, exactly what is robust and if there is no difference between the two? Well, then you can pick the one you have greatest confidence in and footnote the other to the reader, we re estimated the model using full information, maximum likelihood theory and blah, blah, blah, no substantive conclusions were different. But like any sensitivity analysis, if you find a difference between those two, it's on you to delve deeper into say, Well, why what is the source of that difference? So I love this. I love the weather least squares as the spinning hook kick, or is the Empire Strikes Back for you, in your clan? Know what's out there, have it in your back pocket at a cocktail party, when somebody says, well, there's no reason to use anything other than maximum likelihood and to say, Well, really, you know, and annoy them. Because that's the whole point of living. Maybe you use it in your own work. Maybe you don't, but at least you know, it's out there on the horizon.
Greg:Absolutely. All right. Thanks very much.
Patrick:Thanks, everybody. Have a wonderful weekend. We'll talk to you next week.
Greg:All right. Take care. Bye. Bye. Bye bye. Thanks so much for joining us. Don't forget to tell your friends to subscribe to us on Apple podcasts, Spotify, or wherever they go for things that are more bearable to listen to it double speed. Come on, admit it. You can follow us on Twitter where we are at quantity food pod and visit our website quantity food pod.org where you can leave us a message, find organized playlists and show notes. Listen to past episodes and other fun stuff. And finally, you can get cool quantity and merch like shirts, mugs, stickers and spiral notebooks from Red bubble.com Where All proceeds go to donors choose.org to help support low income schools, you've been listening to quantitative the podcast that listener Liz Huntley makes her infant son John listen to to help with critical pediatric development in the areas of irreverence, sarcasm, and social awkwardness. Today's episode has been sponsored by the all new 2023 Ford Edge, which focus groups prefer 20 to one over the Chevy collider. And by second order Taylor series approximations, helping us ignore the remainder terms since 1715. And finally, by regression trees, which given that it's just about autumn in the northern hemisphere are about to drop all those regression leaves for me to clean up and haul away. I might just need a bigger cart. This is most definitely not NPR