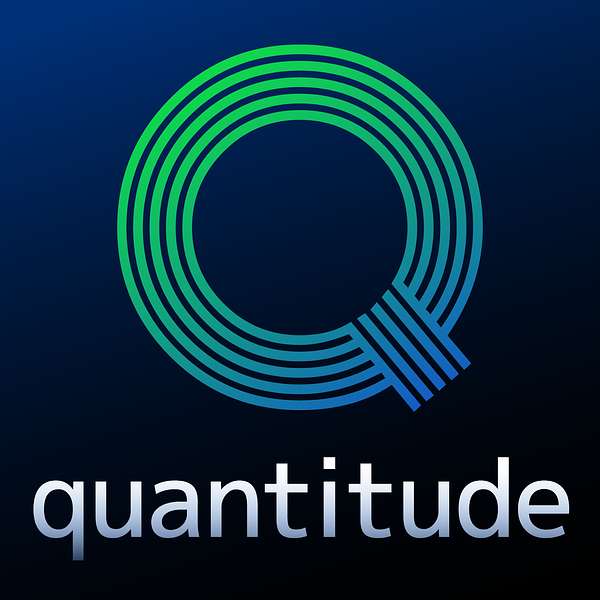
Quantitude
Quantitude
S4E05 Moderated Nonlinear Factor Analysis: A Conversation with Dan Bauer
In this week's episode Patrick and Greg spend a wonderful, if not at times awkward, hour talking with Dan Bauer about the genesis, application, and future directions of what may be the world's worst acronym: MNLFA, or moderated nonlinear factor analysis. Along the way they also mention unsolicited help from teenagers, gold stars, acronyms, words that start with "ci", aggressive mice, manipulating your advisors, 2nd spouses, MoNoLiFa, Quantitube, rewiring your brain, $1M calculators, and mod mod.
Stay in contact with Quantitude!
- Web page: quantitudepod.org
- TwitterX: @quantitudepod
- YouTube: @quantitudepod
- Merch: redbubble.com
Welcome. My name is Patrick Curran and along with my very best friend Greg Hancock, I'm sorry, Dan, I have to say that we make up quantity dude. We are a podcast dedicated to all things quantitative ranging from the irrelevant to the completely irrelevant. In this week's episode, Greg and I spend a wonderful, if not, at times awkward hour talking with Dan Bauer about the genesis application and future directions of what may be the world's worst acronym and LFA or moderated nonlinear factor analysis. Along the way, we also discuss unsolicited help from teenagers, gold stars, acronyms, words that start with CI aggressive mice, manipulating your advisors, second spouses, Mona Lisa, Quanta tube, rewiring your brain $1 million calculators and mod mod. We hope you enjoy this week's episode. Now I got a pop quiz for you.
Greg:Oh, God already? We're 30 seconds in to start ever not complain? No, no, go ahead and control me.
Patrick:We've had three episodes.
Annie:Hi, everyone. This is Annie Patrick's kid. When I was talking to my dad about his silly little podcast, he told me oh, by the way, I made a little mistake. There's a fourth episode before this one. Turns out it's because he missed the deadline. As a teenager, I learned a lot from those around me. Now, if you excuse me, I'm gonna go play video games and not do my statistics homework.
Patrick:The first was least squares estimation, right? The second was maximum likelihood the third was to stage least squares. And the fourth was partial least squares. When I spent the four minutes this morning on my back deck, organizing in my head today's episode, it's twice as much as usual. It is twice as much as I usually spend, I sought to organizing features to the episodes that we've done. So the pop quiz this morning, what are the two organizing features of our first four episodes. I mean,
Greg:one of them is that all the things that we do in our lives here, our statistical lives are about understanding parameters, estimating parameters, and whether it's a least squares kind of framework, or Fischer playing Calvin Ball, switching up the rules and going into maximum likelihood. And then we talked about some of the shortcomings of maximum likelihood and how those might be able to be addressed by some strings of least squares, specifically in the two stage least squares. So I guess one of the themes is really about estimation and all the different ways we can try and get at the things that we care about those parameters in the population.
Patrick:You're exactly right. Thank you. You get your first gold star for the day. Okay, it's probably the last one but you'll get a gold star for the day. What is the second organizing theme? I'm not sure I mean, I can make something up. I'll give you a hint. Okay, we compared Oh, well less PHYML to SLS MIB SEM,
Greg:and pls I have it dependent we are on abbreviations and acronyms. Am I getting closer?
Patrick:Just acronyms what I just said makes perfect sense. Yeah, it leads to a very, very small proportion of people who don't have a lot of friends no social skills at all. These become nouns and proper noun zeven. Yeah. Oh, well last PHYML mivv. Sem and verbs we ml did we OLS did I don't know if anybody in the history of humankind has said we ml didn't. Let's not overplay our hand here.
Greg:You never validate me.
Patrick:Give me a reason to and I will. All right, we talk about EFA, CFA, SEM, MCMC estimation, we should do an episode on that. Actually, that's pretty cool. One of my favorite that just rolls off the tongue is to 2PL-IRT. I mean it's a lyrical that's Shakespearean.
Shakespeare:From claiming Westfalen through my host, but he would have no stomach to this fight. Let him depart.
Greg:I have a true confession on the acronym front. I have a center here such as it is at the University of Maryland called Silver CIO VR. And I was in a meeting with one of our Dean's she was speaking to a group of people saying we really need more initiatives around centers. And so as she's talking, I started jotting down words that started with C because center de and I came up with silver and it took me the rest of a two hour meeting to find the words that were close enough Center for Integrated latent variable research. The firm is silent. Dammit silver sounded cool. I even had a logo for it before I had a mission or purpose of the center but that's the
Patrick:important stuff right?
Greg:I had a logo done and done did the Dean
Patrick:look at your yellow sticky and said so what is it killed over? A kid Si Si is an asset.
Greg:When you put an eye after a si in the English language, it softens the SI
Patrick:give me one word that follows that rule that begins with the SI city. Wow, that didn't take long. Not at all. All right, I'm gonna edit that out because it makes me look really stupid.
Greg:And he did edit it out. And I put it right back in. It just felt like my civic duty to circumvent Patrick. Well, I enjoy my cinnamon cider. But there are absolutely terrible acronyms. And I think if that's going to be a unifying theme, we don't have to look very far for this. Because speaking of terrible acronyms, we have a guest today,
Patrick:we are very pleased to welcome Dr. Dan Bauer from the University of North Carolina at Chapel Hill, who is singularly responsible for possibly one of the worst acronyms in our field. It is logically pronounced move. Welcome, Dan.
Dan:Thanks, guys. Really appreciate that introduction. And I take some exception to the word singularly.
Patrick:Are you now going to defuse blame back on me as well? Greg does this every time and it's really frustrating, because it's usually true.
Dan:You were the editor.
Patrick:So we will get to this in a minute. Dan is quantitative psychologist, he is in the LL thurlestone, psychometric lab, Dan and I go back more than 20 years, a long, long way. It's longer than either one of us wants to ponder, do we have fun here with origin stories, because I don't think one of us in the field takes a straight line shot to whatever it is what we're doing today, and you are no exception, that my
Dan:shot was probably as crooked as they get. I started out in developmental psychobiology working in a mouse lab. So I don't know how to connect dots from a mouse lab to a quantitative psychologist, I got interested in growth modeling. And we were looking at micro genetic changes in behavior happening through the modification of developmental trajectories. So how do you get a low aggressive mouse versus a high aggressive mouse is what maybe you can speed up the development of aggressive behavior. Or maybe you can slow down the development of aggressive behavior that kind of got me interested in growth modeling. And I pretty quickly realized I was more interested in the models than the mice. But I happened to be a grad student at University of North Carolina, and UNC has a really nice quantitative program. And I was just kind of taking the classes because I enjoyed them. And I could find them applicable in my research. I thought, Oh, well, maybe I could just get a masters and then leave and go do a masters and biostats or something a real masters that real Yeah, I'd like, like legit serious capital S. But then it occurred to me that the developmental program didn't actually require a master's degree. And so I was like, maybe I'll just stop calling it a master's, I'll start calling it a dissertation and see if anyone objects and nobody did. Like, I kind of got away with one I just like, well, and this will be my dissertation proposal, and everyone was like, okay, or I guess so. And so I skipped the masters and was able to get my PhD, as you probably not so fondly remember, Patrick, you were a member of my committee. And that was part of how we got to know each other aside from you hurling expletives at me during your classes. And so we arranged for me to put in an RSA application to do a postdoc with yourself and with Ken Bolin. And that's how I kind of moved over to the dark side of quantitative methodology
Patrick:joining me together, we can rule but galaxy. I like the professional development tip for the day for grad students out there who are currently working on your masters. Just start calling it your dissertation proposal, because I gotta tell you, advisors do not track where you are in the program. Pro tip from Dan Bauer, just call your masters the dissertation and move on.
Dan:It's like nobody noticed.
Patrick:So then where did you go after the postdoc,
Dan:I took a system Professor position at NC State University, like a lot of people I had some family constraints. And so I was excited to have an opportunity to enter academia in the area, didn't even have to move house just drove a little farther to get to Raleigh in the morning. And so I spent a couple of years at NC State University and then a position opened up at UNC
Patrick:I always make my students say in one sentence, describe what it is that you do in the field. What is your one sentence,
Dan:I am the director of the Thurstone lab. So most of what I do is manage budgets for small amounts of money and try to convince my colleagues to teach the classes that need to be taught Dude,
Patrick:this is really passive aggressive because my computer died and Yesterday, I asked him if I could please have a couple 100 bucks to have it repaired. And I still don't want to teach that undergrad class. So if there's something that you want to talk about, let's have it. No,
Dan:no, nothing. I was just trying to make the point that I ended advertently transition into a kind of administrative role where my day to day tends to be much less exciting in terms of like research and quantitative methods. It's more like, oh, we have this really tiny pot of money. How can I divide it up? So everybody feels it's fair.
Greg:I will just insert here that as Patrick's second spouse, I like watching you to fight. That petty of me,
Patrick:it is not okay, because I have a pair of teenagers and they refer to Dan at the dining room table when we're talking about like werkstoffe or whatever they will refer to Dan is first wife and Greg is second wife. I want to be trophy wife
Greg:can I be trophy wife? Awkward silence.
Patrick:Thank you so much for having the lack of judgment to join us this morning. Very briefly, I will clarify this whole acronym thing we did a special issue was published insight methods in 2009, dedicated to integrative data analysis, and it's the art of combining multiple independent samples and then fitting models to the joint sample. I was the guest editor for this special issue. And I handled Dan's paper. It was in part titled moderated nonlinear factor analysis M and LFA. In handling the paper and the reviews, I would like to say I wrote in an editorial letter back she shoved my chair back and leaned out into the hall and screamed, nobody can pronounce no fun. There was about a 10 second silence, and he yelled back, make it mono Leafa. I waited about 10 seconds and yelled back. MNLF A is fine. And I gotta tell you about that I see as one of my greatest failures.
Greg:And that's saying something.
Patrick:This could have been a discussion about the Mona Lisa, I may have authorized the world's worst acronym. You created it.
Dan:I think there's a Sinatra song that goes with that. Did it my way.
Unknown:I'd like to claim credit. But actually, MoNoLeFa came from a conference participant when I was doing some preliminary presentations of this stuff has like yeah, now it's kind of a hard to pronounce acronym and a participant came up. No, he's great. You call it Mona Lisa. I was like that'll get under Patrick skin.
Greg:I think we should contextualize why we invited your first wife here. So over the last, you know, over the last three seasons, because Patrick and I have been doing this podcast for quite some time together. It's an activity that we share for many, many hours each week. And even over the summer, when he comes to my house and hangs out. And we have a lot of fun. We have done an episode on invariance testing and the challenges associated with that we've done an episode on moderation. And we had an episode on integrative data analysis. Ida, as you cool kids call it their unifying themes associated with those three things that I will concede lead really nicely into the work that you did. And so we thought it would be really good for you to come and talk with us about some of your work on m mu. Dang it moving. I'll get it, I will get it. So can you start to get a situated within the moderated nonlinear factor analysis world in terms of how it ties to some of those things
Dan:before that. If I could tell one small anecdote. I don't know if you guys have mentioned map before, but it's a secret cabal of quantitative methodologists who meet once a year, not long after you guys started the podcast, Katherine Mason, I think has been a guest before came up and said, Are you jealous that Patrick Stewart this? And I said, you know, actually, no, it's okay. Patrick can have other friends. And you know, sometimes it's kind of nice, because there might be things Patrick wants to do with his other friend that I didn't really want to do anyways.
Patrick:Deanna know you're doing a thing. We haven't started it yet. But it's going to involve some live stream teaching on YouTube and proposed yesterday that we call it Quantitube.
Dan:But it's totally different because there's video.
Patrick:What we're going to see in the conversation as your work is huge here. I second that. I think that this is one of the most significant contributions to how we think about psychometric modeling and scoring. And I really do feel like in 10 or 20 years you This is gonna be a routine way of thinking about it. Where did the first genesis of the idea come from? And then how did you pursue it?
Dan:I feel like there are kind of multiple threads that kind of came together with that. So I mean, the immediate Genesis was that you, Patrick and Andrea has song and myself and a bunch of grad students and postdocs were involved in this integrative data analysis project. In integrative data analysis, there's often a lot of evaluating of differential item functioning, right, you have slightly different measures of the same thing in different studies. And you want to see if you can combine those and to be able to combine them, you have to demonstrate you have enough similarity and measurement that the scores are comparable. And the traditional approaches to evaluating differential item functioning. And measurement invariance just didn't work wonderfully in that context, because they're set up for groups that can kind of work if you just like saying, well, let's compare study one to study two. But what if you also have a really broad age range, which was the case that we were looking at, and you think there might also be some age effects running around in there where measurement is not the same at all ages. And what if you also think that there could be measurement differences between boys versus girls. And so you've actually got these multiple dimensions over which you need to evaluate measurement invariance, one of which is continuous age. And the traditional methodology all assumes that you have grouping variables, and you're kind of looking at one at a time, maybe you could sort across to, but you're pretty limited in terms of enamel go beyond a single categorical grouping variable. That's a problem that needs to be solved. If we can't do it the way that would be optimal, where we look at study differences, we look at sex differences, we look at age differences simultaneously and retaining age as a continuous variable. If we can't do that, using the traditional methodology, then let's solve that problem. I was in a position based on other stuff I'd been doing at the time to see a potential solution to that problem. Around that time, I don't know maybe like the early 2000s, there was a lot of interest in the relationships between multi level models and factor models. There's like a whole era Symposium on this where they were showing how multilevel logistic regression is sort of like a rush model. And you can kind of get a two PL in there if you do some extra stuff. And then some work I did with you, Patrick, on growth modeling, you can do growth models as multi level models, you can do growth models, or structural equation models, and how can we extend beyond the growth setting to other kinds of multi level models being like structural equation models, and so my head was kind of in that space of well, we can think of a latent variable model as the same as a multi level model. And I've been playing around with a SAS procedure proc NL mixed, which allows you to fit things like two PL models as nonlinear multi level models. So I thought, Well, okay, this NL mix procedure is incredibly flexible. We don't have to specify every item as a binary item, like in a two PL model, we could have different distributions for different items. And it's flexible enough that we can specify that the parameters, associating those items to the factors could be a function of some external variable. If that external variable is a binary predictor, like you're in study one versus study two, then it's just like a multiple groups model. But if it's age, all of a sudden, you've got diff as a function of a continuous variable. And you could put more than one of these into those equations. That's sort of where I started with seeing, Oh, I've got this flexible procedure I can use where I can specify the likelihood by like, literally looking up on Wikipedia, what's the negative binomial likelihood? Oh, here it is, I'll just write it into this AST. Now
Patrick:to clarify, NLMIXED was created by Satan himself.
Dan:It's got a learning curve to it, for sure. And I think it was Jack McArdle who said, Oh, and all mixes an amazing program, as long as you already know the answer before you run it, because you have to give it really good start values to actually get a solution. But it provided a very flexible framework. So all that thinking got me to a place where I was like, Well, I'd really like to do this. And here's a way I could implement this using existing software by sort of just drawing on that correspondence between what we think of as random effects or multiple models and traditional confirmatory factor IRT types of models. So I had that flexibility. I thought, well, we can solve the problem with that. And sure enough, if you specify it and you let it run for like, 20 days, you get an answer. As time went on, it became possible to implement these models in more efficient software. So like open MX can fit these models and plus can fit these models and much more efficiently. And that made it possible to do more research on these kinds of models.
Greg:One of the things that I love about this and about statistics in general is that we often strive for the bigger umbrella, right? We strive for that model and take some arrogance in doing so by saying, well your model is just a special case of my model. And what you have done with this thing minima is you have created an umbrella that's fairly broad. And I absolutely love that. Let's just start with something simple like a two group, multi group confirmatory factor analysis, can you tell us how the two group model is a special case of that, and then broaden it out for us
Dan:in that two group model, you got sample one and sample two, right, and you've collected data on both of them. And the traditional way of analyzing that is to say, I'm going to get a covariance matrix for sample one and a covariance matrix for sample two. And I'm going to see if the same measurement structure reproduces the covariance matrix in both groups, right? So can I have the same factor loadings or not, we can either constrain those factor loadings to be the same, or we can let them be different. If they're different from one another, then we'll get a factor loading for sample one, and we'll get a factor loading for sample two. And we can test whether they need to be different or not using a variety of approaches out in the literature for how to determine whether those differences need to be there or not. And the MNLF A, it takes a slightly different perspective on it, where it says, Okay, we're going to have a reference point. So you could choose sample one, or sample two to be the reference. And for that sample, we're going to get a baseline estimate of what those parameters might be. So there's going to be a factor loading for sample one. And then in sample two, we can either just have that same factor loading, which would be the invariance, or we can estimate a difference, right, so we're not going to estimate the factor loading sample two directly, we're going to estimate the difference of the factor loading for sample two relative to sample one. So essentially, what we're doing is sort of building an equation for what the factor loading looks like in any given sample. And sample one is just the baseline value in sample two, it's a baseline value plus perhaps a difference from that. Well, when you start to think of it as an equation for the factor loading, now you can start to say, well, what if I wanted to put in a continuous variable? Right? What if I wanted to put in Ah, okay, well, now your baseline loading is going to be for age zero, and then you're going to have some potential change in that factor loading as age goes up. So for each one unit increase at age, we might get a so many units increase in the factor loading, because it's an equation now we can also have more than one thing in that equation. So we could have one variable could be the study that someone came from another variable could be the age of that person, and other variable could be their biological sex. And we could even have interactions or curvilinear effects. So maybe the age effect on factor loading is not linear, maybe it's actually curved. And so once we start to think of it in terms of an equation, it really opens up the possibilities to do lots of things that we wouldn't have thought of, in the two group case, right? And the two group case, we've got a covariance matrix in sample one, and we get a factor learning, we've got a covariance matrix and sample two, and we get another factor learning and can they be equal? Or do they need to be different, but once we build an equation for it, now we kind of open the doors to lots of possibilities. So that's kind of how it at least in my mind sort of scales from that multiple group setting is it sort of reframes it in terms of equations for the parameters, and then you build out those equations. And if the only predictor in that equation is a binary or a grouping variable, then you're right back in that MultiSample two group case,
Greg:if you really only had a binary predictor, so if you were doing the equivalent of invariance testing across two groups, kids who identify as male kids who identify as female, for example, and you take the approach that you're talking about, and you build this predictor, in as a predictor of the loading will, the solution that you get out literally be identical to what you would get out of a multiple group,
Dan:you're gonna get exactly the same parameter estimates exactly the same solution. The one thing that MLFA won't give you, though, is all the fit statistics. And it's because imagine if instead of the grouping variable, you have a continuous variable. Now you don't have groups within which you can calculate covariance matrices and evaluate fit, because every unique age is going to have its own unique model implied covariance matrix. So you don't have those same tests of model fit as you would in the multi sample sem the traditional way of doing it,
Greg:I think I live without the fit, at least on the principle that if the model fit reasonably well, without you exploiting these interactions, then the interactions in my mind can maybe only make things even better. Is that reasonable? I think
Dan:so. And of course, you can still do relative fit. So you can look at likelihood ratio comparisons of a model without certain effects and a model with those effects. And so you have those, you just don't have the traditional chi square test to fit RMSEA CFI. But yeah, I think if the structure without these effects fits pretty well, it's reasonable to assume that the structure with these effects will continue to fit at least as well.
Patrick:When you start thinking about your framework. It's really wild to start thinking about parameters as dependent variables. We've talked a lot on prior episodes about the important statistical concept of it depends. So what is the value of the factor learning? Well, it's point seven. How about for treatment and control? It's like, Dude, you're not listening. It's point seven. But then you start to generalize and say, what is the value of the factor learning you say? Well, it depends. Are you in the treatment or in the control because that means it's either point five, or it's point eight. And then we're off to the races. Because now what I envision in my mind's eye with your general framework is we no longer have it's either point five or point eight, I see it as tuning right as age goes up a continuum with a factor loading goes up on a continuum. And it's not even figurative, it's literal. When you get the output from these programs. For a particular parameter, you have an intercept, which is the value of the parameter when all predictors are zero. And then you have slopes associated with each covariant or nonlinear effect or interactive effect, as you said, that then from that baseline value moves you up and down that number line, the elegance of thinking about that as a general framework changes the way I think about a parameter estimate.
Dan:One example of that, from a fairly recent paper I had on the SEC methods was I looked at an MLFA on delinquent violent behaviors. And one of those items was, have you ever been in a fight that led to someone having to go see a nurse or doctor, so one that really leads to some harm, and there was dif all over the place for that item as a function of age and as a function of sex and as a function of the interaction between age and sex. And I hadn't really gone into the analysis anticipating that I was going to find that. But once I saw that, I thought, wow, okay, yeah, because who is going to throw a punch and inflict actual harm? Well, it's not going to be a young kid, because they're just not big enough yet to inflict real harm, probably. But an older kid, especially an older boy is more likely throw in that very same punch to inflict serious damage. So it's the same behavior, but a more significant consequence. And because of that, we saw this age and age by sex interaction, looking at the item parameters for that particular item. And so I was like, wow, that's kind of cool. That taps into ideas about how developmental norms are shifting maturational effects, heterotypic, continuity, all kinds of cool notions, to see how the very same behavior might be indicative of something else at different ages. And for different kinds of people, I just want to
Greg:underscore something here, which is what we do a lot, the other person says something and then we repeat it. take credit for take credit for. And then we go back and edit out the first person who said it. I love it. You had snuck in there when you first started mentioning this putting in a variable that represents the study that it came from. And that's the bridge to integrative data analysis. Right, right. That's where you expand on stuff.
Dan:I mean, yes, it is the bridge. I'll just repeat what you said. But more profoundly, it is the bridge to integrative data analysis. And originally, that MLFA was proposed within that context to solve problems that arise there, because we're tackling all kinds of measurement and variance issues in an IDA setting. But it's not exclusive to that setting, right. So you can apply this really in any context within which you're interested in evaluating measurement invariance. There are lots of settings in which you might be concerned with measurement invariance, not just as a function of a single binary or grouping variable. And so it does open the opportunity to look at a lot of different kinds of measurement invariance than traditionally we do.
Greg:And I love that you were talking about having interaction. So it's not just being able to absorb the typical categorical moderator variable or grouping variable, being able to handle continuous and then being able to handle interactions among variables, just sounds so powerful and flexible, my brain has to be rewired a little bit,
Patrick:that rewiring of going all the way back to Meredith, your work is changing how we think about invariance. So go back to Meredith, he has a psychometric paper on invariants. And it was one of the most important papers written and all of us who work in this area know very well about configural, weak, strong and strict invariance, it was all built around a multiple group models, so group zero and group one, whatever that might be. So you estimate one model, where you allow all the factor loadings to be different across the two groups. And then you estimate a second model in which you make all the factor loadings be equal across the two groups. And then typically, you do what's called a likelihood ratio test where you say, is there a significant difference in the fit of the models when I allowed them to be different versus allow them to be equal? If there's no difference between the models, we typically conclude that there is weak invariance meaning that the factor loadings hold for both groups given what Dan has already talked about, you start to squint your eyes and caulk your head and say, Well As all of them are either equal or not equal, and then you say, oh, no, no, no, no, we can do partial invariance where we use modification indices often to go on a bit of a snipe punt and say, Well turns out a 10 items seven were equal, and three were not so then we have partial invariance. But when you think about any parameter in your model, in principle can be expressed as a function of one or more covariates. It almost sets that entire framework, not aside, but it makes it a very special case of the more general approach
Dan:with these kinds of models, I've actually come to like more and more the way the IRT people look at invariance. In factor analysis, as you describe, we tend to focus on the equivalence of certain model matrices. So if the factor loading matrices are equal, we have weak invariants. If both the factor loadings and intercepts are equal, we have strong invariance or scalar invariance. But if we go so far as to say the residual variances are all equal to then we get to strict invariants. So we're sort of focused on particular kinds of parameters and whether they're equal across groups. And that gives rise to certain properties for the latent variables and how they're comparable. Whereas in the IoT world, they tend to instead focus on items. So in factor analysis, we're focused on matrices, and we're evaluating whole matrices across items. And an IRT we tend to focus on one item at a time, I think focusing on the item level is more productive, particularly when we start to think about multiple variables involved in measurement invariance. And some of those potentially being continuous, because we might find that we don't have a single item that is invariant across everything, we might have 10 items, and seven are invariant to age, but not invariant to sex, or study. So what we really need, I think, is for any given dimension on which we're looking for measurement invariance, we have a subset of items that don't show diff. So we're looking at age, we need just a subset of items that don't show age diff, they might still show diff as a function of some other variable. It gets complicated to think about, but I think our usual rules for deciding on partial invariance and what constitutes enough invariance to make scores comparable, probably need a rethink. In this context, it does get at times a little overwhelming because there is so much complexity being built into these models. Thing was Roger Millsap who at one of these secret cabal meetings of SMAP, you say, Yeah, this is a really general model. But is it too general, very quiet happy? To clarify. That worries me a little bit, because it gets so general that it becomes difficult to use,
Greg:can we go through the steps that one would go through just in a typical example of an MNLF? A. So if we had, let's say, a two or three factor model, just talk us through what would be the typical practical steps that someone would go through for assessing the invariance of different aspects of that model.
Dan:It's something that I've been trying to tweak. So I'm going to sort of start out with how I've typically done it, and then maybe tell you where I think things can go to make things easier. Typically, the way we've done things is we usually pick off one factor at a time. So if you have like a three factor structure, pick off just one of those factors, fit a model in which there's full invariance. So no diff anywhere, just a standard factor analysis model, you can look at fit with that. And it's just a baseline. It's just a starting point. And then what I used to do is one item at a time, I would go in and I would say okay, for item one, is there any evidence of diff, or as a function of whatever my background variables, let's just say sex and study and age. So item one, is there any diff, okay, and then we go and we fit the model again. And now we take the diff out of item one, and we put it in item two, and then we take it out of item two, and we put it in item three, and each time we look at how much improvement and fit was there relative to this model with no diff. And the item that shows the greatest improvement in fit, we say, Okay, looks like we really need diff there. So we'll put that in. And now we'll make another sweep, do we also need diff and item two, do we also need different item through and so on. And so we kind of get to a point where there's no longer any improvement and fit by adding more depth. And so this is a kind of iterative procedure that involves lots and lots of likelihood ratio tests and model specifications. And it's a real pain to do some very enterprising young scholars Nisha Gottfredson. And Veronica Cole developed our package, a MLFA, automated MLFA. That makes this process a little bit easier to do as an applied person. It still involves lots and lots of these likelihood ratio tests. So that's sort of traditionally how I have done things, but we know from research looking at similar methods that that is prone to errors because when we test for the first item with diff, we're not including the diff for the other items and when we test for the for fourth item with diff? Well, if we'd identified that when earlier, did we really need the first three, we're not really doing things in an optimal way with that kind of testing strategy. And so more recently, I've been working on developing some ways of fitting the model in a way where you can identify those patterns of diff without having to do this sequential testing using regularization. What that does is it says, Okay, we're going to estimate the model, there could be diff everywhere, except that the diff is expensive, right. So to add a diff parameter to the model, it's going to cost something in the likelihood. So you don't just get the benefit of including it. There's also a cost associated with including an ad with a regularization procedure, you can run the procedure from place similar to that first model I described where there's no diff at all, the penalty is really high. So there's no diff, it's too expensive. And then you slowly ratchet down the penalty that allows the diff to enter the model. And at some point, you identify, we've hit a point where maybe it's the Bayes information criteria is at its minimum, and this looks like the best place to stop. And that's my pattern of diff. So what's nice about that, from the perspective of an applied user is that you can just hit run, and it does all this work in the background, where you get the most bang for the buck, right? Diff was expensive, but this is where you needed to pay for it because it gave you a good improvement to model fit. So we played around with that in a frequentist setting with lasso penalty. And we've also played around with that some in a Bayesian setting, which has some real advantages as
Greg:well, this is still for the one factor at a time part,
Dan:right, this is still for the one factor at a time because these models leads to their estimate in a maximum likelihood setting require the use of numerical integration and numerical integration becomes more and more computationally expensive. As you add factors, it becomes very expensive to add more latent variables to these models. Within the Bayesian approach, the computational speed doesn't slow down as fast, the numerical integration of maximum likelihood is like this exponential cost in terms of computational speed, whereas in the Bayesian, it's more linear. So it still takes longer to fit a model with more dimensions, but not as long as the maximum likelihood
Patrick:in addition to just the time and the computation, your 2017 paper, you did expand it to multiple factors were not only can you do that, but what I felt was really intriguing about that is you can write equations for the covariance between the factors
Dan:that was really cool. I mean, I kind of went into that wanting to show that you could do this with more complex models. And so the strategy I use there because they are computationally expensive, is identify the patterns of dif for each factor univariate Utley, I had the violent delinquency factor and the non violent delinquency factor, and I analyzed those separately to identify the diff. And then once I felt like I knew where the diff was going to be, that's when I bolted them together, and then looked at that covariance parameter. And what's cool about that is then you can look at a question like are violent and nonviolent delinquency more highly correlated at some ages than others, or for some groups than other groups, boys versus girls, for instance, it really opens the door in a sense into thinking that any of the parameters of my model, I could potentially allow it to be moderated by these background variables of interest. It doesn't even necessarily have to be in a measurement context, you could do it in any kind of context, whether it's a covariance parameter or variance parameter, or what have you. And in that sense, it expands upon how we think about moderation in general, and regression analysis, we do moderation all the time, we include these product interactions. And what does that mean? It means the slope of one variable is a linear function of another variable, right? Well, we're not restricted to slopes anymore, right, we can have a covariance be some function of other variables, we can have a factor loading be some function of other variables. So we can expand what we think of in terms of moderation as any parameter and a model could potentially be expressed as a function of other variables. And that I think is really cool.
Patrick:It's amazing to see because in Dan's 2017 paper picture in your mind's eye in x, y plot, where x is age, and y is the covariance between two factors, you can have a nonlinear relation that tracks increases in age with the model implied difference in the magnitude of the covariance between the latent factors. There's no existing readily available analytic method that allows us not only to numerically evaluate that based on our sample data, but imagine the types of theoretical questions that this opens.
Dan:That's absolutely right. In this multi dimensional setting is particularly well suited to questions like the differentiation of factors over time, or what I think sometimes we observe in our Our age set, which is the de differentiation hypothesis of cognitive aging, that what were previously kind of distinct intellectual abilities sort of glom back together, and it's all crap. That one Yeah, I think it's really well suited to evaluate those kinds of questions
Greg:in the factor model, how do you handle the scaling and potential non invariance on that
Dan:what we do is sort of analogous to what is often done in a multiple groups factor analysis, where you choose one group to set the mean of the factors to zero and the variance of the factors to one, imagine that you're not doing that in the standard way, instead, you're doing it as Okay, we're gonna have baseline parameters for one group, and then we're gonna have differences for the other group. So you're gonna have a coding variable 01, and that coding variables, either going to produce the difference or not, it's scored one, then you get the difference, and it's scored zero, then you don't. So similarly here, we just set the mean and variance to zero, and one where all variables are zero. So if you're going to look at something like age and sex, then you choose, okay, I want girls to be the reference group, I'm going to code them zero, and you choose an age either at the beginning or in the middle, or at the end of the scale point. So Center at somewhere meaningful and and that's it's not too different from how you would interpret an intercept in a regression, right as the intercept is the expected value, or all predictors are zero. Similarly, we're going to have a baseline factor loading, that's going to be the factor loadings where all predictors are zero, we're going to have a factor mean, where all predictors are zero, we're going to have a factor variance where all predictors are zero to identify the scale, we have to fix that mean invariance somewhere in a multi group factor analysis, you do it for one group here, we're going to do it for one level of those background variables where all our zero will have means of zero and variances of one.
Greg:What is Patrick sign of the zodiac?
Dan:I don't even know my own sign of the zodiac for goodness. So people helped me his birthday, I believe is in May,
Patrick:you had a one in 12 chance. Wow, you were to talk awkward in the measurement class that is taught by Hancock and Curran. We have an entire chapter on MNLF. A
Greg:It's like he's opened up his phone and showing pictures of you several hours. Look, this is Dan I miss Dan,
Dan:Patrick could make this amazing soup. Well, your dinners good too Greg.
Patrick:Damn in some of the way your work has been generative and broadly construed my work in collaboration with you using them and LFA has actually been very practical and very pragmatic. And that is I want scores. There are many uses for factor analysis. But there are two big ones. One is understanding the psychometric model that underlies the setting indicators that we observed in our sample, what are the factor loadings? What are the item intercepts, what is the variance of the latent variable, and then your method allows us to say, well do those vary in some deterministic way as a function of the set of covariates. That's very important, that's very interesting that moves us forward as a field, what I want to do is to take a set of indicators, be certain that I've worked in whatever differential item function exists in the way that you've described. And then I want to use it as a million dollar calculator to get a manifest variable score that I can put in my back pocket and take to more complicated analytic frameworks, like a growth model or a multi level model. Tell us about the scoring potential for these things.
Dan:That's a lot of where the initial motivation came from. With our integrative data analysis applications, we wanted scores on which we could fit some growth models, the promise, at least of this approach is to get scores that are more comparable, that are fairer, to compare, or to look for change on over time than what we would get if we failed to account for that differential item functioning and that lack of measurement invariance, some research that Patrick you had it up, but I was a part of looked at, well, what if we just did scoring, as usual? Didn't look for the diff. What if we do incorporate the diff? Some analyses make it look like well, maybe it doesn't matter that much traditional scores and the MLFA scores might correlate in the point nines somewhere. And you might say, Oh, well, that's good enough. I could just use the regular scores. But other analyses like well, what happens when we bring these scores into a model showed real differences and the MLFA scores better preserved the kinds of relationships that we knew to be there because we had simulated the data there is the promise to be able to get better scores from this kind of a modeling approach than what we currently do. with business as usual,
Patrick:I have a love hate relationship with these at the scoring level as I drank the Kool Aid on the MNLF a scores and we will put in the show notes. But Dan and I, together with a group of really talented colleagues and students and postdocs have written more papers on this than we probably should have. We did some simulation work, we've done applied work, we've done it within the substance use research, we've done it within internalizing and externalizing symptomatology. One thing we have to balance, I think, in using these things in practice, is that you and I found pretty unambiguously that when you build in diff, and here's the big thing, and when you know what that diff is more or less, if you do not incorporate that into your scores, there are going to be downstream influences that bias the use of those scores and other analyses. But Dan, and I have been really fortunate to have Dave thissen as a colleague, and Dave is an internationally recognized expert in item response theory. And paraphrasing one of his views is the sum score is wrong, but you always know how it's wrong. So if you just add up the number of items, that's always the wrong score, but you know how it's wrong. And it's always wrong in the same way. And one thing that has started to worry me a little bit about these models in the way that I've used them for scoring, the scores are only as good as the MNLF estimates that you get the goes into the calculation of those, and each of those coefficients that slide a lambda up and down, or slide a tau, up and down. Those are characterized by a standard error. And if you were to do a thought experiment, and redo your study, all of those parameters would take on slightly different values, the some scores are not going to change, but the estimated MNLF, a score is
Dan:going to make it worse. Please, wow, not only are the parameters, you estimate gonna take on different estimates across samples, but you might not even estimate the same parameters, because you're assuming that you would know the right pattern of diff. And we're always doing some kind of data based assessment of where is the diff well, on one sample, you might have a false positive here and a false negative there, and then another sample, it could be somewhere else. And so you might have different patterns of diff from one sample to another. So not only is it sampling error that we have to worry about, but also the accuracy of whatever diff detection method we're employing, and whether it really did recover the right pattern of diff or not.
Patrick:And yet, this gives us a tool to look at the characteristics of the data in a way that we didn't have before. So I don't want this part of the conversation to be taken as Oh, wow, you shouldn't be doing it. This is actually part of the benefit of the more general and complicated model. Not only do we have to deal with these things, but we have the opportunity to deal with these things. Because if you do a single group model, you're just rocking back and forth and saying there is no diff, there is no diff with the very same date. If you do a two group, you're saying it's equal to point five or point seven, it's equal to point five, or it's point seven. Here, it's saying, dude, you can write an equation for any parameter in your model, you got to deal with that
Greg:the stuff that you did, has changed the way I think about things. What I mean is, it's not so much in the context of invariance testing within a factor analytic model, or diff specifically as we think about it, psychometrically. But the broader idea that parameters themselves can be functions of other things as liberated my thinking about moderation, the person I used to be pre Bower BB would, or whatever that would be before the Bower era bbe Is that what it would be a person that was bbe would have been looking for thoughtful ways to take age and maybe partition it into meaningful age groups, and then just beat the heck out of multi group analyses and do the best that I could. But that's not even just in the context of factor models, right? I think about this in terms of a path model. So I recently was working on a grant that I had funded with a colleague in the School of Social Work, we got funded to look at direct effects, indirect effects, other kinds of effects as a function of a whole host of other variables. And this has nothing to do with a factor model. But it has everything to do with thinking about parameters, whether they're simple parameters or more compound parameters as a function of other things. Now, I just gotta give credit where credit is due, you have changed the way I think about moderation much more broadly. And I think that's just crazy. Cool.
Dan:So Thanks, Greg. It has kind of changed my own perspective as well as I worked on this as I teach our regression class. In some ways, what we're doing in regression is saying, hey, there's a mean of a distribution, and it's a function of these other variables. So you could think of that as we're taking a parameter of just a very basic model that a distribution has a mean. And we're saying let's let that mean be a function of other variables. And then when we get into moderated regression, now we're letting a slope be a function of another variable. And as you describe in a path analysis, you could allow direct or indirect effects to be functions of other variables, you could allow the correlation between two variables to be a function of other variables. I think of the location scale model that Don Hecker developed, you're allowing a variance parameter to be a function of other variables. I think in statistics, maybe because this is where the easy stuff was early on, as we've gotten so focused on how to means vary as a function of other variables that we've sort of lost sight of, well, we actually have the apparatus now that we could allow lots of potential parameters that describe distributions or bivariate associations or causal effects or whatever they might be, we could allow those to various a function of other characteristics. It's a more flexible way of thinking that opens up hypotheses that we might not have thought of otherwise, or at least not thought that could be testable and an optimal way.
Greg:So then, here's my question for you. If we think about it more broadly, then MNLF a doesn't work anymore, right? Because that's specific. So what do you call this?
Dan:Yeah, Patrick's gonna love this one, though.
Patrick:What is it called?
Dan:Somehow, this just came to me as an epiphany, a moderated model, we'll call it mod mod. Like that. It's mod mod. I don't really have a good name for it. A moderated parameter model, a conditional parameter model. So we called it parameter
Greg:moderation models in the stuff that I had written with another person. PMM is the acronym that we had thrown in there.
Dan:That's my homeowner's association. So that's no good.
Greg:Okay, yeah, well, no, God, we wouldn't want that's out.
Dan:Actually. No, wait, it's ppm. So you're good up. Okay.
Greg:Which is parts per million damage. All right. There's potential here. And the only thing I really know is that we're sure as hell not going with Mod mod.
Patrick:So definitely generative novel work needs to be done on naming the damn thing. I'm not sure I would prioritize that. But okay, we can put that on the list. Beyond naming the model, there are a lot of people listening who are looking for interesting research projects, their dissertations, their grant proposals. What do you see as the next battles that need to be fought in this war?
Dan:I mean, I think there are tons of possible interesting applications of the model in a substantive context. But I'll kind of focus on where I see quantitative research needing to go one is these multi dimensional models, Greg, mass, how do you do this with multiple factors. And it's hard because it is computationally expensive to do multiple dimensions. And maybe there are clever people out there who will figure out better ways to estimate these that makes that problem go away. Also embedded in there is that we don't really have good ways to parameterize the covariance matrix. So if you have two factors, okay, I can do some sleight of hand using a Fisher's Z transformation and make sure that that correlation stays between negative one on one and doesn't wander outside of that interval. But if you have three factors, I can keep any one of those bivariate correlations between negative one and one, but I don't have a good way of ensuring that that covariance matrix is always positive definite at all levels of the background variable. So I think there's got to be a better way of conditioning, a covariance matrix like a covariance matrix among factors on background variables like age, and so forth. So I think that's a potentially interesting area of expansion related to the multi dimensional is doing all this stuff in a longitudinal setting. And that's some stuff that a few of my colleagues and students are working on now have a very talented student, Marco Chen, who's working on one longitudinal application that's going to be multi dimensional, because you've got to factor at every time point, or you've got to deal with this multi dimensionality as you're trying to extend this into longitudinal settings. And I'm sure there'll be lots of room for expansions in that direction as well.
Greg:I think there's a lot to think about here. It's incredibly rich and potential topics for people to study. Again, I will concede that it does change the way that we think about a lot of things. And I'm pretty sure that when Patrick comes up next time, and he and I go sit on our favorite bench in the Inner Harbor,
Patrick:hi time here and even his favorite band, I want to thank Dan for coming today. Van Halen. Okay, thanks, everybody. We appreciate your time. We hope you found this of some use. Take care,
Dan:Patrick, why don't you go ahead and drop off Greg. I think we have lots of things to make some comparisons on hey, I can give you some advice on how to deal with some things that might come up II
Patrick:Oh, wait, I'm host of the zoom.
Greg:No, no, don't stop yet. I want to make just one point before. Thanks so much for joining us. Don't forget to tell your friends to subscribe to us on Apple podcasts, Spotify, or wherever they go for things that are more bearable to listen to than hearing your partner go on and on about how great their other friends are. Even if they were an Eagle Scout and their hair does this cool, whoosh thing when they throw their head back and laugh at your jokes that aren't even funny. And you've told like 10 times. You can follow us on Twitter where we Patrick and I specifically are at quantity pod and visit our website quantity pod.org a monument to all we have built and shared together, where you can leave us a message and find organized playlists and show notes. Listen to past episodes and other fun stuff. And finally, you can get cool quantitative merch like shirts, mugs, stickers and spiral notebooks from Red bubble.com Where All proceeds go to donorschoose.org. To help support low income schools, you've been listening to quantitative the podcast whose name is actually clear, and not some dumb acronym that you can't even pronounce. Today's episode has been sponsored by Patrick's favorite jazz musician Miles Davis, or for those of you and other places in the world 1.6 kilometers Davis and by posthoc power analysis which contributes about as much to our understanding as signs of the zodiac. Patrick sign by the way is Taurus which also like posthoc power analysis is symbolized as bull. And finally, my intent to treat because I have an intent to treat myself to a giant pitcher of margaritas after this episode. This is most definitely not NPR or as Dan might pronounce it. I'm okay. I'm okay.