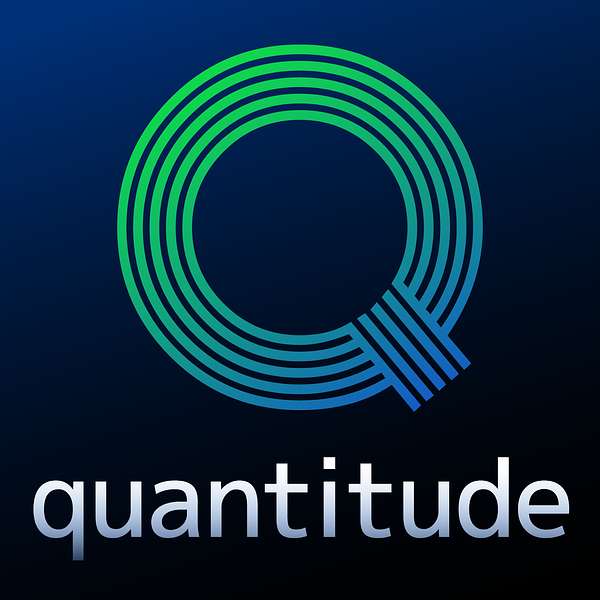
Quantitude
Quantitude
S4E06 Building the Model You Want: With Laser Cannons!
In this week's episode Greg and Patrick talk about the frequent gap between research questions and methods and ways to think about reengineering your variables, your parameters, or your entire model to help get what you want. Along the way they also mention: living in the dorms, Greek god sculpture rockets, not living in the dorms, Christmas tree timers, Def Leppard, Legos, Annie's flower shop, laser cannons, chin music, the tail wagging the dog, the Millennium Falcon, Greenday, stupid human tricks, Tony Robbins, Stuart Smalley, and Iron Man.
Stay in contact with Quantitude!
- Web page: quantitudepod.org
- TwitterX: @quantitudepod
- YouTube: @quantitudepod
- Merch: redbubble.com
Hi everybody. My name is Greg Hancock and along with my friend whose instructions were thrown out a long time ago Patrick Curran, we make up quantity food. We're a podcast dedicated to all things quantitative, ranging from the irrelevant to the completely irrelevant. In this episode, we talk about the frequent gap between research questions and methods and ways to think about reengineering your variables, your parameters or your entire model to help get what you want. Along the way. We also mentioned living in the dorms, Greek gods sculpture rockets, not living in the dorms, Christmas tree timers, Def Leppard, Legos, and his flower shop. laser cannons, chin music, the tail wagging the dog, a zero on the sa t, the Millennium Falcon. Green Day, stupid human tricks. Tony Robbins, Stuart Smalley and Ironman. We hope you enjoyed today's episode. Did you live in the dorms when you went to college
Patrick:until I was asked to not live in the dorms?
Greg:Okay, so one semester.
Patrick:It was almost two semesters, but it involved a friend of mine who was an aeronautical engineer. He took a sculpting class, he sculpted the head of some Greek God, he brought it back to the dorm. He and I decided to fill it with gunpowder from Estes rocket engines and see if we could blow it up. And it turned out that there was no explosion at all. But we made a giant Estes rocket out of the shape of a Greek god, which ricocheted up and down the hallway. I was asked to find alternative residency.
Greg:I lived at home for the first three years or so because I had a single dad and my brother was still in high school and so I was kind of helping out at home. But as Chris got older and was approaching his later years, I moved into the dorms, I had this massive stereo with speakers that were waist high. If you want to wake up to a certain song, and it's 1984, your options are a lot more limited. I had music on a cassette. So I put the tape into my stereo and I put it on the song that I thought would be the most totally amazing song to wake up to. I pressed play, I had the power out to the entire stereo because it was routed through and this is really the key, a Christmas tree timer. So I had my entire stereo routed through a Christmas tree light timer that at roughly the time I wanted came on at probably 120 decibels blaring the song that I wanted, and the song that I wanted in 1984 was high and dry by Def Leppard. The irony of the whole thing was that when a Christmas tree timer goes off, it makes such an insanely loud clip. That every morning, I woke up to this click and then the song came on, like eight seconds later, I was gonna wake up to a particular song. It just took a matter of putting the pieces together so that I could do that.
Patrick:In contrast, I simply slept through my class.
Greg:But that was basically the college version of me. Because when I was a kid, my dad gave me complete open access to his workshop. Son, you're six years old. Now I think it's time that you use the bandsaw. So I just love building all kinds of things.
Patrick:So my brother has two wonderful daughters. And for longtime listeners, his daughters are Jane and Maggie. And they were the quantity food community players that read this some net messages as part of a holiday episode a couple of years ago.
Maggie:Hi, Les. This is incredibly ridiculous. And it has been for years. This nonsense must come to an end at some point best car.
Patrick:They are addicted to Legos. When you and I came up through the ranks you just got a box of Legos.
Insert:Hey! Kids! Look! Legos is here!
Patrick:Well, now you get a box of Legos that build something with instructions, and you damn well better put them together the way that the instructions tell you to or else you won't get the thing that's on the front of the box right now what I like about what my brother and daughters have done, they must have 1000s and 1000s of Lego pieces. They unsnap them all and they made a giant pile in the middle of this side room. Awesome. And they'll say Hey, who wants to Lego they use it as a verb and the three of them put on music lay on the floor around this giant pile of Legos. And they build whatever their heart desires, there are no instructions, somebody will shout out, I need a three poster, I need a short corner, I need a long cord. And they have this whole obtuse language about what each of the pieces are. Right? I was part of this when I visited a while ago. And I thought, oh my god, this is quantitative methodology.
Greg:It is quantitative methodology. Even now, when you get the kits, you start off when you're little might have 20 pieces, 30 pieces, and there's a picture on the box and you open it up and you get instructions. Maybe the next set that you have has 100 pieces and you build something else and the next set, right? Eventually you work your way up to the Millennium Falcon or Hogwarts, Diagon Alley, but what do you do you put them together and then you just look at them? No, you get this giant pile, I love that image of your family.
Patrick:The creativity is some of the stuff that his daughters make. The ones I like, are a little bit more subtle, where there's like Annie's flower shop. But there's a laser cannon from those I love. I don't want to overdo an analogy. But following the instructions with the Legos that you get is kind of like your regression class. Here's one predictor, here's two predictors, we can do this. And then we do this. And then we do this. And one of the big things that you should do in taking regression is get to a point where you understand what are the strengths regression, what are the limitations of regression, Oh, I see this. It's the unique effect of this predictor above and beyond all other predictors. But that's not what my theory says. So you take a class in structural equation modeling and move on, you're building the things that are in the instructions when you get your Lego kit. And I think that's insanely important to learn how to do. And there's also a satisfaction of holding the thing afterwards and saying, Here's what I made. Yep. But at some point, you say, Where am I going to put this laser cannon?
Greg:You sit, you don't want to torture and analogy, dude, the whole episode is torture in analogy with laser cannon. But this is exactly what happens, right? You get all of these boxes of Legos. And you can imagine them sitting on the shelf assembled are back in their original boxes. You know, we learned a t test. And we learned correlation and simple regression. And maybe you learn a traditional analysis of variance, maybe you move into regression or the general linear model. But each of these things that you learn is like a kit, right? And they sit up there in the box. And the idea of you getting acquainted with these kits, at least as it seems to start is that as you develop your own research question, you will go over to the shelf with all of your Lego kits, and you will reach up there and you will pull the thing off that suits your particular research question. Problem is it doesn't always fit your research question, right?
Patrick:And then you get into the tail wags the dog. Yes. What that means is the kit that you need for your question doesn't exist. So you're going to pick one that's on the shelf that you can include is closest to what you need. But you may actually start modifying your theoretical question and your research hypotheses in order to fit that kit because you can't do what you really want. And so your selection of a statistical model is actually dictating your research hypotheses. Instead of your research hypotheses dictating your statistical model. I can't
Greg:tell you how many times I've heard somebody say, I can't really get at that question with this method. So I'm just going to focus on the other questions, because I can really get at that here. And there's a part of me that is just so sad to hear those words, because I don't want you to say, I have to build Annie's flower shop and only Annie's flower shop, I want to hear someone say, dammit, I need a laser cannon up on the corner there. And what are we going to do to regression? Or what are we going to do with all of the pieces that we have at our disposal to be able to get that laser cannon on top of any flower shop?
Patrick:The thing that worries me even a little bit more is when you take the box off that exists and you're testing your hypotheses in a way that aren't actually being tested. And you're interpreting results in a way that is not consistent with what the intro the method results in the discussion all put together. And I think that's very common as well. You have a baron and Kenny bolted together tested mediation and then your discussion talks about an upward spiral of increasing negative affectivity. Those are not the same.
Greg:You have the story arc then it starts with your introduction and then by God your brain already has some version of a conclusion that you're hoping for and you need that middle part, you need the whole methodological section, the results section, all of that to rise to make that arc legitimate. And I hate it when there's this falling down in the middle. It makes the conclusions in the end tenuous at best, when it didn't necessarily have to be when there might have been some clever way to reengineer. That middle part, you go
Patrick:up to your shelf, and you've got two separate boxes, and you say, why won't part of this from this box and I want part of this from this box? Because Annie's flower shop needs a laser cannon. You put them together, but then you say, Oh, this laser cannon is awesome. I want to tell other people how to build this themselves. And oh, my God, that's a psych methods paper.
Greg:Right? That's exactly that's exactly
Patrick:it.
Greg:I think you just described most of our career. Well, maybe we can start small with this idea as a theme, right, maybe with some of the earliest models that we learned and how we can think about them in terms of this idea of Legos, or in terms of moving the pieces around to get what you want.
Patrick:All right, let's be selfish. You've got a nice flower shop, but you don't have a laser cannon to protect. And Andy needs a laser cannon. Clearly, you have the kit for the Millennium Falcon that has a whole lot of laser cannons. Because we've only been talking about one laser cannon for Andy's flower shop. My niece Maggie actually had it bristling with laser cannons.
Greg:What about maybe some photon torpedoes from the USS Enterprise, pew pew pew pew pew
Patrick:what we want to do is to say alright, I learned how to use the Legos I've learned how to build the thing that's in the picture. And now I want to start manipulating that toward my own end. So the point is, how can we manipulate what we have in order to get what we need? That I think should be the arc?
Greg:Okay, so if we think about it, maybe in terms of what the parts are, we have on the table to be able to move around? What should we start off with?
Patrick:I was on the back deck last night fall has come in full force to North Carolina and it was absolutely beautiful. And I had my clipboard and my fountain pen. What year is this? $25,000 in computers, and I have my clipboard and fountain pen. And the first thing I put down is let's start easy. How can we manipulate the numerical scales of our measures to achieve things we want? Okay, so what do you mean the scales? One of the first things we teach you about as an undergrad is The Maltese Falcon. Do you remember the Maltese Falcon?
Greg:Not the chin?
Patrick:Chin music.
Unknown:People lose teeth talking like that. You want to hang around? You'll be polite.
Patrick:We have four broad types of scale Noir. Why is it The Maltese Falcon film noir? And why are nominal ordinal interval ratio nominal, we stand in front of you. And we say a nominal variable is name. It's a characteristic. It's a grouping, it has no numerical value, it has no quantitative characteristics. And we hammer you about this. And then we say, well, unless you want to make it zero and one and use it as a predictor in your model. And so I am going to start super simple. We take what we told you was a characterization that has no quantitative value. And we're not only going to assign it a zero and a one, but we are going to interpret the model implied mean of the outcome that's associated with moving on the number line from zero to one. Wait for it for a variable that has no quantitative characteristics. Well, what did we do? Let's pretend that we have a one predictor regression, we know this is a member of the general linear model, we know this is a two group t test. We have our predictor that's this nominal variable that we've assigned zero and one. But now the intercept of that equation is the model implied mean of the outcome when all predictors are zero? Well, that's the mean of the first group. And the regression coefficient associated with the predictor is what is the shift in the model implied mean for a one unit shift in the predictor, while a one unit shift is moving from the control group to the treatment group. So the intercept is the mean of the control group. And our regression coefficient is the difference between the control and the treatment group. So we are manipulating the numerical scaling of our predictor so that I get these numerical values that may have some meaning to me
Greg:and it actually feels even more egregious when someone says I have four levels to my nominal variable, right? It might be religious preference. might be identified ethnicity could be a variety of different things. And we want to build that in as a predictor, right. And so what we learned, and this really is this idea of repurposing things, what we learned as well, if you got four different categories in your nominal variable, the end of your noir, then you can code that with three, quantitative predictors, three predictors, that could be of the form 01. For example, let's imagine just for sake of argument that your four groups are that you have a control group, and then three different treatment groups, you could code that control group as zero and then have three dummy variables that represent the difference between each of the treatment groups going back to the control group. That seems pretty handy. And what if I want to know about the differences among the treatment groups, though? Me saying, Well, I'm sorry, I can't compare the treatment groups to each other, because that's not the way this works, would be an extreme example of you completely letting the statistical tail wag your research question dog here. But what do we do
Patrick:we learn this in grad stats instead of zero, and one, maybe we have negative one, and one, because that's going to compare that to some overall mean, maybe we have negative one and positive point five and positive point five, where we can compare one group mean to the mean of two group means you have a whole host of things that you can do that you can say, well, I'm almost going to use the regression model like a calculator, and I'm going to do whatever comparison I damn well, please.
Greg:And our old friend Cohen did a really nice job of talking about different coding schemes. And these should be things that if you're not immediately familiar with them, I hope that they at least ring bells. There's the contrast, coding effect, coding all different types of coding. And the key is that you don't even just lock into those specific types of codes. But as Patrick said, You do whatever you want. Imagine it's the case in the example that I just gave you that the control group was a complete lack of any dosage of something. But the three treatment groups were all variations in dosage. Well, you could do contrasts that represent trends associated with the increase in dosage. As long as you understand that you can manipulate these weights in any way, as long as it subscribes to certain rules, you can ask any question you want. One of the things that we sort of take for granted, even in a quantitative predictor is the scale that the good Lord has given that particular variable, that variable comes with whatever metric it has that variable comes with whatever zero point it has. And you know what, frankly, those things don't always make sense to you. And one of the ones that I would hone in on for this particular point is, the zero value for a number of variables that we have is a completely meaningless thing. If I have your LSAT score, what does it mean to have zero amount of LSAT, but you literally cannot earn a zero on the SATs score, as long as you sign your name trial problem, congratulations, you got to 200. But when we put that variable as it stands into a regression model, the intercept is like a throwaway parameter for the most part, right? It's just the constant as we get in most of the output. But what if we would like that to represent something meaningful, I sure as heck don't care about the value of an outcome that someone would have given a zero LSAT score. The thing is, you can center that predictor, however you want, you could make a score of 200 be the zero by centering that variable around 200. Subtracting 200 from every score, you could make it around a mean of 500, which is sort of the scaled mean of an essay t score before it drifts after a few years and has to be reined back in. You could pick any point that you want. And you should pick whatever point you want to get the parameter out that you want that answers the research question that you want. And
Patrick:when you start seeing that I've got this handful of laser cannons that I can put on anything you want, you start to see, oh, wow, I can control these in a lot of different ways. We often do mean centering of a continuous predictor, just as Greg is talking about, so that the intercept represents the mean of the outcome app, the mean of the predictor. Okay, that's a nice mean, centering is always nice. But we could mean center a continuous covariant around the mean of one of the groups that we're studying Dan and I teach multi level modeling. And we have an example where we're looking at birth weight and there are three groups of mothers healthy controls, there was a treatment that was discontinued in a treatment that was continued. And then we have continuous covariates. What we centered the covariates, not around the mean of everyone, but around the mean of the healthy controls. So that the zero represents what is the mean for the healthy controls and then we can compare the other groups you can do that you can move to standardizing a neat thing in the SEM is we can get a thing called a partially standardized solution. A usual standardized solution is all predictors and all outcomes are standardized. So while one standard deviation unit in your predictor is associated with a gamma standard deviation, changing your dependent variable, that's fine if you have continuous on both sides, but what if your predictor is a group variable? Well, what is the standard deviation change in moving from control to treatment? Well, the regression model doesn't allow in its native state where you standardize the dependent variable, but you don't standardize the predictor, well, you can do that manually, is you can standardize your y before the regression, regress the standardized y on your raw binary predictor, and then you have your partially standardized solution. I have been in a meeting where somebody said, Well, regression doesn't allow you to do a partially standardized solution, just change the scaling, you can do anything you want. And you
Greg:should do anything that you want, right? Make the method match the question, don't make the question match the method. And so everything we've described so far, has been manipulations around a single variable, but we have systems of variables, right? We have multiple predictors. What are some fun things that we can do to help loosen up the rules when it comes to multiple variables simultaneously?
Patrick:Well, let's say we have x 1x, two and x three predicting y. All right, that's a main effect model. The regression coefficient for x two is the unique relation between x two and y above and beyond the effects of x one and x three. That's great. If that's your question, what if your question is, what is the relation between x two and y as a function of x one? Does the magnitude of x two to y depend upon where you are in x one? That's an interaction? How do we manipulate the model to get to it, we do the sleight of hand that we've done for 50 or 60 years, you multiply x one and x two together, outside of the regression model, you bring in that product as a new predictor. And that represents the conditional relation that's going to allow us to model the interaction between x one and x two. But that's the next thing of the laser cannon. Well, let's combine our variables in ways that allow us to do what we want to
Greg:and what you just described, which, like you said, is something we've been doing for 50 or 60 years very, very clever when we look at it. Now in hindsight, we go Yeah, well, it's kind of obvious. But it wasn't kind of obvious, originally. And now imagine that you're sitting in a meeting with someone and the person says, yes, yes, I really want to get at this kind of moderation or interaction between these two. But this product actually is a very specific type of moderation, where the relation of one of your predictors with your outcome changes linearly as a function of the other variable. What if, after some soul searching, you say, I am interested in moderation, but you know what, I think that the slope of that initial predictor is going to get steeper for a while as the other variable increases. But then I think it's going to kind of slow down, I don't think it's just going to keep ratcheting up, up, up up up overall, as a product would say, one option is for you to say, well, I guess the product term is about as good as I can get. So I'll use that for moderation. But you know what, I don't just want a laser cannon. I want a pink laser cannon that shoots out rainbow colored unicorns. But what are we going to do? You can build in a different type of moderation. I don't have to just do a product of x two with x one, I could do a product of x two squared with x one or the log of x two with x one. What is it about x two that you think governs the particular nature of the moderation that you have operating? That is what you should be building in?
Patrick:That's a testable hypothesis that there's some slowing of those increases? we'll square it, bring it in as a squared variable. You've got your pink laser cannon, doo doo doo doo doo doo, doo doo doo doo. Nope. Really, I'm sorry, you express surprise, really? Okay, so Cluster One, we mess around with the numerical values cluster two, we combine variables in a way that are meaningful to us. We take products, we take powers, we take products of powers, we do whatever we want. So we're back out on the back deck last night. Cluster three, however, you scaled your predictors and however you combined your predictors in a way that's of use to you, you estimate your model, and now you have parameter estimates. We have regression coefficients, we have covariances, we have residual variances. Now let's talk about combining those in a way that's of use to us. Not the variables right there. over in the corner. They got a Coors Light Cross Blue Cross like the world's most refreshing beer, their work is done. And the model has given you parameter estimates and standard errors. Talk to me about fun things we can do with those
Greg:parameter estimates are just such a playground. I think one of the problems with the way we usually look at the instructions that come with these kinds of models is that we try to answer complex questions, one parameter at a time. And a very obvious example of this that people should be familiar with is mediation. Right? So we have some hypothesis that X has an influence on Y and Y has an influence on Z. And we're really interested in the mediating value of y to the point of wanting to understand the magnitude of the indirect effect that X has on z through y. Well away that requires us only to use the instructions that we would be given is to look at well, what's the link between x and y? Okay, that's a part of the story. What's the link between Y and Z? Okay, that's part of the story. But the whole story has to do with a combination of information that you get of those two parameters together, quite literally, the product of those parameters is the indirect effect. So one that we're all familiar with, I hope, or at least in concept is that the information of those two parameters can be brought together? multiplicatively, we go off to the side and we multiply those parameters, because that is what gets at our question.
Patrick:That one is like you have taken apart the Millennium Falcon, and you have all the stuff that went into the Millennium Falcon, which is one of the coolest things you can build in terms of stuff. Those are your parameter estimates. And now we're talking about I wonder how I can combine these, remember, not variables, not numerical values, not products, we have a gamma hat sub one and a gamma hat sub two and a gamma hat sub three, what can I do to make new parameters? So a couple episodes ago, we talked about maximum likelihood estimation. And one thing one of us said, I don't remember who because I don't listen, when you talk, is we have all of these beneficial properties of maximum likelihood estimates when we'd need assumptions, right. They're unbiased, consistent, efficient. And here's a really important one for our conversation. Here they are asymptotically normally distributed. So these are all these wonderful characteristics of maximum likelihood estimates. But a throwaway line is combinations of maximum likelihood estimates are themselves maximum likelihood estimates. So that snapping the laser cannon on to Annie's flower shop, now itself is a maximum likelihood estimate. And under assumptions has all the goodies that go with ml. So you take your gamma hat, one of x to y, you take your gamma hat to from Y to Z, and you multiply those, it's sometimes called a compound parameter for obvious reasons. And that now becomes a new parameter in the model that we didn't have before. Like, that's what's so cool about this is we literally have obtained something from our model results that we did not have as part of the native estimation of that model. When you see that it's the I see dead people. I see dead people. When you lean back and say, Holy crap, you mean I can combine these estimates, I can add them, I can subtract them, I can multiply them, I can make ratios. Oh my gosh, it opens up this huge array of kinds of hypotheses that we can evaluate. Is the difference between two parameters zero, right? A super simple question, we can ask is the value of one parameter, the same as the mean of two other parameters? A lot of us have tested interactions and then done simple intercepts and simple slopes. What is the regression of y on x at high, medium and low values of z? Right? That's a David rugosa. Pick a point. Everybody say that it's a fun word, pick a boy thereupon, pick a boy. Here is the regression of y on x, a standard deviation above z at the mean of Z and a standard deviation below z. Well, where do we get those? Well, we take the regression coefficients for the intercept for the main effect of x for the main effect of z, and for the interaction between x and z, and we create new compound parameters to get those probing of the interactions. Yeah, it's all right
Greg:there in front of you, for you to be creative with and put together in different ways that map onto the research question that you have. It's mostly
Patrick:there are in front of you. We got a point estimate you and I even using our limited quantitative abilities can often multiply two numbers. On a good day, we can add two or three numbers at once. The point estimates are right there and easy to get. What about the standard errors? So we have a regression of y on x at high level of z at the medium level of z and low Z. But what we want to know is is the regression of y on x a standard deviation above z significantly different from zero or not? And in enters one of my very favorite little statistical tricks, and that is delta method
Greg:ooooh....tell us more.
Patrick:Okay, we agreed that you would not talk like that anymore. And by we, I mean,
Greg:say delta.
Patrick:What delta method is, is amazingly cool. Why is it amazingly cool? We're not going to talk about delta method in details. But what it is, is it's an analytic method for estimating the variance of a combination of random variants themselves are normally distributed. Yes. Alright. So what does that mean? Well, we can have gamma one, and we can have gamma hat two, we have a point estimate for that we have a standard error for that. And we actually have a covariance between those parameter estimates. It's an off diagonal element and an asymptotic covariance weight matrix. Long, long, lat long. But if we add those two parameters together, we can use delta method to get what is the variance of the sum of two normally distributed random variants? Well, we have this differentiable function with an infinite number of sums, which we call a Taylor series, the Taylor series, in principle can have an infinite number of songs infinite, it's a lot and I want to go for a run. And so usually, we just take the first or the second Taylor series approximation. And this gives you a standard error, what we often do in practice, we estimate our model, get our results, compute these compound parameters in the computer program will calculate the standard errors for those using the delta method. Now we can bootstrap and we can do like their whole conversations about this, I think that's a really neat thing is not only can we combine these parameters in darn near any way we want, but then we can get a standard error, a critical ratio and a p value. I do
Greg:this all the time. One thing worth mentioning is that not all combinations of parameters are going to have these Taylor series expansions that go on for as far as the eye can see, because some ways that we combine parameters are fairly simple. But some ways that we combine parameters are heinous. And if we kept taking derivative second, third, we would just get these infinite series out. I do a lot of stuff with reliability coefficients and different types of reliability coefficients. And if you take a factor model, for example, factor model has a lot of different moving parts to it, it has a variance of the factor, we might set that to one, we might estimate it their loadings, their air variances, well, how does all that information combined to tell you something about reliability, whether it's reliability of a composite of those indicator variables, or some estimate of actually how stable the factor is itself from sample to sample? Well, that information is a combination of things like loadings or factor variances or air variances. And it's not exactly a pretty combination, I can take any modeling package worth its salt, literally define that parameter that I'm interested in, what's the parameter, it's the reliability, I write out what the function is as a function of these other parameters. And I get out not just a point estimate, I also get out a standard error, whether I get that standard error by a delta method approximation, or I bootstrap it. The point is that I'm not stuck with saying, well, the reliability is kind of going to be related to this loading. And this loading, the loadings are pretty good. So the reliability would be pretty good. No, I compute the parameter I want point estimate interval estimate. And that is all right there at my fingertips, you have
Patrick:shared some code with me that I think it's almost magic where you do a CFA and in a couple of lines of code, you compute not only what omega is based upon the parameters in the model, but you get a standard error and a confidence interval for it. You can't say, oh, I can't report omega because it's not part of the output of program X. Yeah, well, drawing on my clinical training, tough crap, put in two lines of code and compute it. And we're talking about SEM and this just generalizes across almost anything. I mean, you did all these things in logistic regression. You can do it totally across almost anything, but you can't say any more. Well, it's not All right in the output, right, I didn't report it because m plus doesn't have it in the output. Well, then freakin put it in,
Greg:yep, reach across the pile of Legos, pull out some other things, snap them together in the way that you need it. And all we're talking about right now is just within a single group. But you can do this across multiple groups. I just described a way to get reliability. Well, what if I'm getting reliability within one group, and I want to get reliability within another group? And now I want to know if there's a significant difference in the reliability across these groups? Oh, no. What do I do? You code the reliability of group one, you code the reliability of group two, you code the difference between these two reliabilities. Oh, my gosh, I get out the point estimate for the difference in reliability is for the standard error of the difference point estimate interval estimate, do I want to bootstrap it gray bootstrap it, all of this is gettable, you just have to be able to literally think outside your box of pieces, and build it the way you need it.
Patrick:And a lot of this stuff is all online, you've written papers about this that has example code in it. This is not a silly, you know, where you could write a Python script nested within R, that pulls in open MX that you can then take two, you know, it's like at that point, I don't care about the question anymore. It's like, Yeah, forget it, it was not that important. This is you define a new parameter in the model, you write the equation for what that is, that is very straightforward. And then you get the standard error for that this stuff is within reach of everybody who's doing this kind of work.
Greg:One of the questions I get a lot when someone is in my office, and they say, Well, I kind of need to do this. And my reaction is, oh, we just need to build that. And then we go through and figure out how to build whatever we want. One of the questions I get frequently is, is there a citation for that? Or can you give me a paper where someone has done that? My answer is usually I have no idea i because I no longer think that way. Right? I no longer think in terms of this set of things that are up on the shelf, I think in terms of just building whatever we want. I don't know if you handle that in a particular way. But I don't have an answer anymore for Is there a paper for that because to me, you don't need a paper for that to be able to build whatever you want,
Patrick:which was a line that I wrote in my review of your psychometric article 20 years ago, which is, I admittedly cannot identify a citation that has done this work. But then again, I never felt the need for one. Right as we don't have to justify some of this stuff, because it just is here. Here's omega, this is the estimate, here is my model, and I am going to calculate it. Then again, this is not a how to discussion. This is think about all the parts of the Legos in front of you, and how would you combine them? It's so empowering. If there's something you want, and you don't have it, then get a Coors Light turn on Green Day go out in the garage and freakin building.
Greg:Exactly, do you have that time to listen to me whine? Somehow, in all of this, you defined clusters of things, Cluster One was single variables, cluster two was multiple variables, cluster three is systems of parameters that we can push and pull. Am I getting that right? So far?
Patrick:We're listening to me? Oh, it's
Greg:so sweet. All right. So then there has to be a cluster for following all of that. And to me, if I may cluster for is just from the ground up re parameterizing particular modeling framework. So first of all, you and I work in this structural equation modeling world a lot. And I will say that structural equation modeling really is all about re parameterizing. Taking this one thing and putting patterns of constraints on it to get something else out. So if you think about a collection of variables, they have variances, covariances, they also have means if you look at those and you say where are the factor loadings in that, or where is the mediation in that? And the answer is, it's nowhere, right? It's not anywhere until you impose a pattern of constraints on the variances covariances and even means to tease out those things that you want. So all of the things that you and I do really are about re parameterizing it's just that we have so abstracted the way we think about things. We draw a path model and that means a system of constraints, we draw a factor model and that means a system of constraints. But each one of those really is this I think fairly brilliant re parameterization of what is at its fundamental core, just a bunch of variances and covariances and means all of that said I still think there should be an award for the most brilliant re parameterization reconfiguring, taking into the garage, pulling the door down, re sanding, and do you have Any idea who I think that award should go to?
Patrick:Oh, you embarrass me. Go ahead. I have no idea. Well,
Greg:if I tell you the name Bill Meredith
Patrick:No, it's the Lincoln curveball, Meredith and T sec. It is the most creative contribution. I think since Spearman
Greg:just unbelievable. And again, this is one of those things that in hindsight, we look at it, we go, oh, yeah, that's the way we do it. But the idea of literally taking the values of time, whether it's hours or days, or years, or whatever, and hard coding that into a confirmatory factor model to emulate a growth process to me is just like crazy thinking, fantastic.
Patrick:You're not going to let me bring time in as a predictor into the SEM. Okay, I am going to take what you treated as a random variable, right? Think about a multi level model. Yeah, time is a predictor that is a column in your data file, and you are regressing the outcome on the predictor, and obtaining fixed effects and random effects that are associated with that. And he said, I don't need your stinking predictor. I'm gonna take those values, I'm going to fix them in a factor loading matrix. Funny story, I'm gonna get exactly what you get, not kinda not the same discussion, not the same general conclusion, 16 decimals out, I'm gonna get exactly what you get. But now we live inside the SEM.
Greg:And the things that we can do within this framework are phenomenal. You and I had a whole episode, I think that involved some therapy with Dr. Michelle, where we talked a bit about some of the relative merits of the different approaches to being able to get at growth, but your shortcomings notwithstanding the thing about this framework, this whole? I statements, Patrick,
Patrick:I think you're a toll.
Greg:So once we have growth modeling, we already know that there are things that we can do within that framework that we almost take for granted now, right? So if we say, Oh, I don't necessarily want to have the initial time point as my frame of reference, I would like to have the last time point as my frame of reference, or I would like to have some middle time point as my frame of reference. That's really parameterizing the model. You want something quadratic? Well, God bless you throw on a factor Wait, what fits imaginary, that's right, throw one, this latent thing that represents a quadratic code, the loadings to be reflective of that quadratic effect, and darn it if you don't have a quadratic model there. So this is something that we have a certain amount of experience re parameterizing, even within just sort of this basic framework,
Patrick:and what I love is we started with changing the numerical scale, then how do you combine your predictors, then how do you combine your parameters, and now we're leaning back and putting our hands behind our head and saying, You know what, I'm going to take a computer program, and I'm going to treat it like an optimizer. It's no longer an SEM program. This is an optimizer for me, I am going to build the model that I want. And it's going to give me maximum likelihood estimates for it. And you know what it's going to do what I tell it to do, right? I'm going to build a model not only like Meredith, which is amazing. But think about when we talked about her with moderated nonlinear factor analysis, we are literally creating new parameters in the model that we're not using as a calculator, right? That's right. When you describe computing omega and getting a confidence interval, that's actually not a restriction on the model, what we're doing is taking parameters. And we're combining them in ways that are of use to us moderated nonlinear factor analysis and parameter moderation. And that whole expansion from there is I am literally going to impose restrictions in a way that have meaning to me, that gives me a testable hypothesis that the box of a nice flower shop does not. I have had so much fun in my brother's basement with my nieces. I'm not exaggerating, the pile of Legos is five feet across. We put on music and lay on the carpet, and we just talk and we build these things. This final cluster of stuff is you know what anise flower shop doesn't even exist. You start with a single piece and you build that any darn way you please, we can
Greg:operate within an existing framework, right? We can talk about clever ways to build things within a factor framework or clever ways to build things within a mediation and moderation framework. We can talk about clever ways to build things within a growth framework, or we can say exactly what you're talking about, and And that is just, you know, all the pieces are out there in front of me. What do I want to build today,
Insert:Milan hotels, animals, people, boats, skyscrapers and more.
Patrick:When you and I came up through the system, you were living at home, I was temporarily living in the dorms. For those of you at the University of Colorado, I was in the Kittredge complex briefly. We were addicted to David Letterman, David Letterman had the top 10 lists, and he had all these things. And he had Stupid Pet Tricks. And then it generalized to stupid human tricks.
Insert:And it's on the stupid human tricks. Ladies, gentlemen, nice young people come here, and they perform a little tricks that they've learned. And they're not stupid people by any stretch of the imagination, but they have learned stupid tricks.
Patrick:I then was at a conference and it was one of the very first times that I had seen you present. And you gave an entire talk titled something like stupid lizard tricks. And it was human preacher. And you just decided to see how far can we push the envelope on getting Liz roll to swallow a heroin filled condom and get on the plane. Tell us about stupid LISREL tricks.
Greg:I don't remember that pero Winfield kind of, but anyway, they have a better memory than I do. And let me be completely fair about this historically, Chris preacher used to keep a list of stupid lists, rail tricks, which are really just stupid structural equation modeling tricks, ways to coax out of models, things that you want. And there was a time when he and I ping pong back and forth different tricks for getting things that ultimately led to this collaboration that he and I had. And it was within a growth modeling framework. But I think it's really in the spirit of what you're talking about. So I'm going to explain it within the context of a growth modeling framework. Imagine you were looking at nonlinear growth in the form of let's say, a logistic function where people start off slow, then they kind of speed up and then they taper off. Well, what if you're interested in knowing at what time point is it that someone is transitioning from growing to tapering off? Right, and their specific points along the logistic function where that might be happening? And you think, well, first of all, how the heck do I even model logistic growth? And second of all, how do I even get that out of the model? Well, that's the kind of thing that Chris and I are saying, Well, that seems like something someone might want to do. If you think about growth, that is piecewise. People might follow a particular function for a while, and then all of a sudden bend up in a different direction, or bend down in a different direction where your growth function is characterized by these multiple lines that have been spliced together spline, okay, well, that point where they come together, sometimes referred to as a knot. What if you want to estimate where that knot location is? Well, some work by Jeff herring and Bob queue deck and Steven Dutoit laid out how you can make that a parameter in your model. Because if you think about, where's that parameter in a model, how can we trick a model to be able to estimate that and these are all just things that we might want to get out that aren't normal parts of the model? Tessa Johnson and I Intesa our old like one of our original Friends of the pod test, and I had a paper come out a couple of years ago on what we call time to criterion growth models. The idea is that how long do we expect it will take somebody to reach a particular threshold of proficiency? At what time point will that happen? How do we reengineer a growth model to make that a particular parameter in the model, and it's not just a given parameter as though it works for everybody. But it's something that has individual differences with all of these examples, something that Chris and I were talking about a number of years ago, and that he 100% was the brains behind? He and I wrote a couple of papers, one that was a book chapter and then one that was a psych methods article with the underlying theme being how can you get whatever you want out of a growth model? And so in 2012, we talked about ways that you can identify a characteristic of growth that you are interested in that you think that there is a fixed value for that cuts across an entire population. How can you retool a growth model to be able to get that kind of thing out? And we outlined sort of a four step process for doing that. And then in 2015, we said, well, what if that differs for people? What if that's a random value? So for example, think about that, not in a spline model, where if we think growth is characterized by two segments, but those two segments don't necessarily meet in the same location for people, how do we estimate what an average not location is? How do we estimate the variability and not location? And then how do we do cool things like build predictors in a that particular characteristic. And so what Chris and I did, which totally falls in the family of stupid LISREL tricks, stupid structural equation modeling tricks, is we outlined a process where you identify the characteristic that you want, you figure out a way to get it in your model, you figure out a way to parameterize it, estimate it. And the idea wasn't just to be able to get people to estimate random knots or something like that. The idea was to be able to lay out a framework to get people to get whatever they want out of a model. In this case, it was a growth modeling framework.
Patrick:It was also in that talk that you threatened to kill a kitten. For each person who said, like hurt, you have an impact on a room, as I'll give you that. I love that body of work, because instead of saying, what is the latent curve model able to give you you're starting with what do I want from the latent curve model. It's just a different way of thinking and a different way of approaching your data and your research hypotheses, whether you are a Tony Robbins person.
Insert:So if you let someone else take control of your focus, your life will be in someone else's hands,
Patrick:or a Stuart Smalley person. Because I'm good enough.
Insert:I'm smart enough. And doggone it, people like me,
Patrick:or whether you are Maggie, who wants to put a laser cannon on her flower shop.
Maggie:Hi Everyone, this is Maggie. And I really did put a laser cannon on top of Annie's flower shop. Also, Uncle Pat is not very good at Legos, goodbye.
Patrick:All of those are saying this is not testing my hypothesis in the ways that I'd like. And so I'm going to build it myself. And if we have a real point to any of our conversations, this really is an empowerment one of think about the question that you want to empirically evaluate, and then modify whether it be your variables, your parameters, or the very model itself to get what you want. So as we're wrapping up here, if your Christmas timer is going to go off, in start playing your eight track tape
Greg:was a cassette. It wasn't an eight track,
Patrick:but give us a song to go out on what would inspire you.
Greg:The pressure. I don't know. First, I was afraid. I was petrified.
Patrick:I did not ask you to sing it.
Greg:Don't Stop Believin
Patrick:Okay, folks, we so appreciate your time. I know. I know. I texted you about this. I came out in the living room. My daughter was there with her friend. They were doing a crossword puzzle on the floor, listening to my old albums, and they had Steely Dan. They had Yes. Oh, and there was the original Black Sabbath. I am gonna go out on Black Sabbath Iron Man. Awesome. Thank you everybody. We will talk to you next week. Take care thank you so much for listening. You can subscribe to quality food on Apple podcasts, Spotify, or wherever you download instructions on how to build a rocket out of the head of a Greek god. And please leave us a review. You can follow us on Twitter we are at quantity food pod and check out our webpage at quantity pod.org for past episodes played lists shownotes transcripts and other cool stuff. Finally, you can get quantitative themed emerge which is distinctly not available on Amazon Prime day@redbubble.com Where All proceeds go to Donors Choose to support low income schools. You have been listening to quantity food. Your very own intellectual pile of Legos scattered across the living room floor corner today has been brought to you by fat Bear Week, congratulating Greg and Patrick on their strong second place finish only behind bear 747 and wishing them the best of luck next year by the student loan debt relief program, a truly remarkable resource for millions of students, even if a bit unfair to those of us in our generation who paid tuition with live chickens, shiny rocks and seashells. And by the North Carolina State Fair, teaching the next generation of youth about model misspecification one mole at a time. This is most definitely not in PR