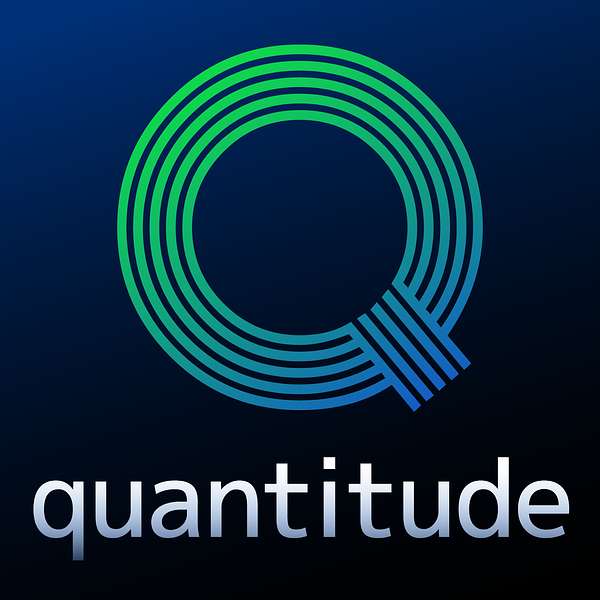
Quantitude
Quantitude
S4E14 Growth Trajectory Estimates...What's My Line?
In this week's episode Patrick and Greg explore all the ways they lie about things when they teach, not the least of which is that there are actually no individual growth trajectories estimated in an individual trajectory growth model. They discuss why this is, how individual trajectory estimates can be obtained, and how these might be used in practice. Along the way they also mention the green light button, developmental milestones, love for semicolons, Jack Nicholson, baskets of data, Oprah, it's all crap, transparencies and dittos, yelling at your steering wheel, libertarians vs. socialists, carts and donkeys, catching squirrels, enemy on the field, being stung, bit, and chased, and persnickety models.
Stay in contact with Quantitude!
- Web page: quantitudepod.org
- TwitterX: @quantitudepod
- YouTube: @quantitudepod
- Merch: redbubble.com
Welcome, my name is Patrick Curran and along with my libertarian friend Greg Hancock, we make up quantity Dude, we are a podcast dedicated to all things quantitative ranging from the irrelevant to the completely irrelevant. In this week's episode, Greg and I Explore all the ways we lie about things when we teach, not the least of which is that there are actually no individual growth trajectories estimated in an individual trajectory growth model, we discuss why this is how individual trajectory estimates can be obtained, and how these might be of use in practice. Along the way, we also discuss the greenlight button, developmental milestones, love for semi colons, Jack Nicholson, baskets of data. Oprah, it's all crap, transparencies and dittos. Yelling at your steering wheel, Libertarians versus socialists, carts and donkeys, catching squirrels, enemies on the field, being stung bit and chased and persnickety models. We hope you enjoyed this week's episode. So both of us have teenage kids. And I don't know what your experience is up north. With increasing frequency things seem to arise at the dinner table where they say you told us this at some point early on. And it turns out that we just figured out that Do you have some of this happening in your own house?
Greg:I mean, there's two ways of looking at it. Right? There's the stuff I told them that wasn't entirely accurate. And then there's stuff I just withheld, telling them. So if you mean things like the world isn't fair, you can't grow up to be whatever you want. And I'm not even sure I'm your dad. Then there are some things that I might have withheld just because I didn't want to depress them the rest of their lives. But are Is there something in particular that you're thinking of that you have had to walk back or that your kids called your BS on silly things
Patrick:come up here at home, and one just made me smile? Because it made me think back to my own dad when I was a kid. At one point, I asked my dad, how did stoplights work? He said, Well, I've got a button in the car and you push the button and it changes the light. And so we'd pull up to the stoplight. We were little kids and my brother and I would say push the button, push the button. And it was really sweet. Because there was a life lesson in it. My dad would say, Oh, we're not in a big hurry. Let's let these other people go first. I'm sure they have more important places to be. And then at some point, he'd say, alright, well, Fair's fair, it's our turn, and he would reach under the dash and the light would turn green. Yeah, so when I was five, but it went into like sixth and seventh and eighth. At one point, my dad, somewhat incredulously said, You're old enough to realize that we don't have a green light button, right? It became a developmental milestone is worried about little Pj is he still thinks at age 19, that I can change the light. What it got me thinking is all the things that we teach kids were either it's just easier to simplify it because you don't want to deal with the complexities, or more importantly, they're not ready to learn the particular thing. But the funny thing is, we do that when we teach grabbed classes
Greg:all the time, right? And I have mixed feelings about it. But there are admittedly a lot of things that I teach early on in a stat sequence that later on, I have to walk back or later on, I sort of have to admit, well, there's really more to the story than that. And I find myself in that situation all the time. Part of the
Patrick:problem is it really is easier to teach something in a particular way. Now, it's not an overt lie. Well, sometimes it is. But it's a simplification picture in your mind's eye, right? We always talk about that. It's like what we're trying to do here or you pan back. The goal here is to, and it's a simplification, but here's part of the problem. We teach semester long classes, I do a little something. And then I pass them off to Bower, Bower didn't know what I talked about, because Heaven forbid we talk to one another and have a cohesive curriculum. It starts getting baked into the system. I don't go back a year later and say, okay, all of us were together last year. And I described this this way. Now, let's dig a little bit deeper into it. Yeah. A funny example I had as I took a wonderful workshop years ago on the science of scientific writing, his name is Goldman and he actually has a whole body of work on how do you write well in a scientific framework, and he had a laugh out loud conversation about the semicolon. Now, you and I have worked a lot together. And you and I have written together you know that I have a very liberal policy on the Oxford comma. And I love semicolons, he had this thing where we should use semicolons more. But when they're introduced in middle school, we're not ready for it, we're not ready to learn how to properly use the semicolon. And so we're not taught it. But by the time we are emotionally ready to use the semicolon, nobody teaches it anymore. It's beyond, it's forgotten. It was just a laugh out loud, funny conversation, because what he did is tied it to kind of like health class and your bodies are changing. I think a really good example of that that is very, very common. It's not just me and you it's very, very common is the first introduction to the multiple regression model. When we talk about Y and Y hat, and the residual E. And the sum of the squared E's I almost every introductory class teaches in a way that the model doesn't actually do it that way.
Greg:I know that when I teach regression, I do it very colloquially, we've got some points there. And we say, hey, try a line. And what would the predicted value? What would the Y hat be for everybody with this particular line? And how do we gauge what the best fit line is in terms of how far the actual points get above and below the line, and we can't just talk about adding those up. And so we talk about why we might square those and sum them up, we wiggle and jiggle until we get that best fit line, that line that minimizes the sum of squared vertical deviations of actual y values from predicted Y values of y's from y hats. And then in the end, I'm saying, See, everybody has a y, but everybody also has a y hat. And I'm seeing all of this stuff, almost like anthropomorphizing the process. But in the end, that's more the statistical puppet show and not exactly what's going on, right?
Patrick:With regression, in fairness to us, you can do it that way, when I teach it, I'm almost like Oprah. You get a residual, residual, it is true, we can do it that way. Everybody has a y. Now everybody has a y hat. And there's a distance between those. And we take Y minus Y hat and get the EES. And we gathered those all together, blah, blah, blah. That's how we describe how we do this. But the actual analytics, that is the getting the regression coefficients, we can do that entirely on covariance matrices. Yeah. But if we have complete data, if you give me a covariance matrix of your outcome, and your predictors with no raw data at all, I can do everything that we do in the regression model. And indeed, however you do that yourself, it is not doing these individual Why Why have E squared, adding them up? It's all the level of the covariance matrix and the mean vector, that to me is kind of like the semi colon, you're not ready to think about x prime x inverse x prime y, when you're very first introduced to it is I feel like Jack Nicholson,
Insert:you want answers? I think I'm entitled you want the truth, you can't handle the truth.
Patrick:But the problem is, is it gets baked in. And then rarely, if ever, does somebody come back and say, you know, funny story, those individual observations and the individual reserves out those don't actually ever exist within the confines of the program, we get the variances of the residuals without ever computing the individual residuals.
Greg:This is all a closed form system that is derived through some optimizations. It's not a wiggle and jiggle optimization like we're accustomed to in a lot of iterative procedures. It is closed form, we do some calculus, we go boom, here's the formula that gives us that and we never touch a Y hat. We never touch a residual. And yet, we keep talking about them as though there's some critical part of the actual process of getting that line in the first place.
Patrick:And I can make it even worse. Okay, please, you and I both teach growth modeling. We've had several episodes related to growth curve analysis, I go full bore Oprah on the growth model is inter individual differences in intra individual change, who I say each of you in the room has a set of repeated measures. Some people have three, some people have four, some people have five, they're in a basket in front of you. Now what I'm going to have each of you do is fit the line that best characterizes your set of repeated measures. Now in your basket is an intercept in a slope. Now I'm going to walk around the room and I'm going to gather all those intercepts together. I'm going to gather all those slopes together and now I've got a distribution of intercepts. Epson, yeah, no, I don't know what that is crap.
Greg:All of it is crap. And you know what, I'm gonna do it till the day I die. I know that it's crap. I know that we don't literally do that. But you can't handle the truth.
Patrick:I would have liked to have seen Oprah and Jack Nicholson. slope, slope, you want a slope, you can handle a slope.
Greg:But it makes total sense, right? If you say, All right, everybody, you've got your own data, Patrick, you have a bunch of repeated measures, I want you to fit your line, go ahead, fit your line, use the regression skills we lied to you about layers, get your slope, get your intercept, you give them back to me. And as you said, I'll put them back in a basket. I love the pedagogical value of that. But you are totally right. That's not how it really works. It's
Patrick:the same answer the semi colon it gets baked in. I do my Oprah, as I'm talking about inter individual variability in these trajectories. Some people start higher, some people start lower, some people increase more rapidly, some less rapidly. And then the big reveal, we can try to predict those. I too am not going to change mostly because I'm old and I can't be fired. I know the slides have already been made. Yeah, I mean, my transparencies are doing just fine.
Greg:I made the dittos.
Patrick:But the problem is, is you walk out of the class without an understanding of Oh, funny story. Every time you've heard somebody wax poetic about individual variability and developmental trajectories of change over time, those do not exist. Yeah, what we do is we look at variances of intercepts, variances of slopes means of those covariance between the two. But it's actually not only kind of like it is exactly the same as the regression model, where we talk about having the variance of the residuals where we never compute the residuals. Here, it's even a little weirder, because we have a variance of the intercepts and the slopes. When we never compute the intercepts and the slopes,
Greg:we tend to teach this thing in a very forward way, you know, imagining we go from the data to the lines, to the characteristics of the lines, the summary statistics, and then doing fun things with those lines. But it works almost completely in the opposite way that we actually have all of the summary stuff to start. And then the question is, do we have a reason? Do we have a need to back off that and talk about individual level things like in regression, I don't have people's residuals to start, although I talk about it as though I do I do the regression, I fit it with that closed form process. And then I can ask what residual each individual has, if I'm interested in that, here we are in a longitudinal setting. But we have this model where we say, oh, yeah, I know what the average slope is, oh, yeah, I know what the variances of slopes and the same thing for intercepts. But now tell me what that means. For an individual. I don't even have that information. It's not like I look at how far their point is above or below a line. I don't even have a line for that individual.
Patrick:And to be super clear, if you're yelling at your steering wheel while listening to this and saying, but you can get residuals estimates and regression, Oh, of course, you can't. That's diagnostics, right. We have residuals and studentized residuals and Studentized deleted residuals, we can get distributions of residuals, we can save them out and subset them, we can look for extreme residuals and outlier detection. But the big point is, we have to do extra work to get those, they do not come out of the standard x prime x inverse x prime y, where we get all of our usual anova tables and our regression coefficients and standardized coefficients and all of that. And that's actually where I want to pivot to is going to the growth model. There is a reason why we teach it about everybody has an intercept, and everybody has a slope. First of all, that's the green light button in my dad's car, which is you're not ready to think about lambda sy lambda prime plus theta apps to get sigma theta hat. It turns out that even though we do not estimate individual intercepts and individual slopes, whether it be an FCM, or an MLM growth model to be super clear, they never exist. In an analysis you want to know a super weirdo thing. All my Oprah you get a trajectory you get a trajectory. If you have complete data, I can estimate the means and variances and covariances of individual trajectories based solely on the covariance matrix and mean vector of the repeated measures.
Greg:You don't have to see any raw data at all It
Patrick:rubs a little bit of the magic off the growth modeling lamp which is Wait a minute, you talked about inter individual differences in intra individual change and blah, blah, blah, I need a covariance matrix. And and
Greg:you never saw an individual, you never saw an individual.
Patrick:Here's the pivot I want to make, just as there are reasons why we want a residual out of the multiple regression model, to do everything that we just talked about, I argue that there are many reasons why it's incredibly helpful to also get an estimate of the trajectories from the model. Because just like the regression, we don't calculate individual intercepts and slopes when doing a standard growth model. But we can use that model to get estimates of the intercepts and the slopes. Well, I
Greg:am going to want to hear this because there are some places where I feel Yeah, absolutely. That makes sense. And then there are other places where I go, Wait, I thought that's what we literally try to avoid with some of these particular models. You know, when I talk about CFA, for example, confirmatory factor analysis, I will often talk about having a model that is a representation of the reason variables relate according some particular theory, or that theory involves one or more underlying latent variables, and students will invariably say, so then we get scores for those factors. First of all, they don't even understand right off the bat that we don't have scores for those, and I remind them, their latent That's what the word laden means. But then somehow, at the end of the process, they're like, okay, it's okay. Okay, so now we get scores. So we can use those for other things? And my answer is usually no, usually, we don't need to. So here we are in a latent growth model setting. And I know that we could talk about things in a multi level model setting as well. And I fit a growth model, I learned so much just by getting these characteristics of the intercepts and slopes and how they covary and how things might predict them. So help me understand under what circumstances getting estimates becomes useful in the way maybe that getting residuals in a regression are useful. It depends.
Patrick:Are you a libertarian or a socialist? Wow. You're just the answer the question.
Greg:I don't have to answer the question. You can't make me answer the question, because my freedom it is my right not to answer, you're
Patrick:a libertarian. Excellent. Let's pan back a little bit. And I'm going to make up some numbers as we have a hypothetical example. So we have a sample of 200 people, we have five repeated measures. Now, anything that we talked about, were almost always in a longitudinal design, different people have different numbers of measures. So maybe have some have three, some have four, some have five, whatever, as you're listening picture in your mind's eye, your own application, where you ever retained your data matrix, where you have five assessments on your outcome. So let's talk about development of reading skills in children. So we have something that we believe to systematically increase on average over time, but there's probably individual variability around if you're a libertarian, which I was when I started learning these growth models, which do an OLS regression for each individual separately. Now, I was all about this in early days. Oh, yeah. And it really was a libertarian kind of thing. These are my repeated measures. I want the line that best characterizes these person one has five repeated measures on reading ability, we're going to regress reading ability on time. Sure. 01234 is the predictor picture a little scatterplot with your five repeated measures, and we're going to fit a line that best characterizes those repeated measures. Well, does my line impact your line? No, not at all. This is an individual regression. That's the libertarian aspect to it. I'm not imposing any functional form that's governed by anybody else. Whatever you do is your right your freedom. You be who you are, we get a regression for person one, we get a regression line for Person two. I'm a SAS guy. So I use SAS terminology, but you do whatever your home program is, you do proc Reg, by ID, we're going to do 200 Or Well, less regressions. And we're going to gather together all the intercepts and the slopes. I actually wrote a paper it was led by a wonderful scientist named Madeline Carrick. And RJ worth was also on this. We'll put this in the show notes. She designed a SAS macro that does this automatically. You just put what is your outcome, what is your time, and it does all the regressions and pulls them all together. It does graphics, things like that. So this is very easy to do. So this is the Oprah Winfrey, you get an intercept, you get an intercept. What is super important to get across here is my intercept and slope is not impacted by your intercept and slope. That's
Greg:right, because it's only informed by your data, nobody else's data anywhere.
Patrick:That's the libertarian. What about the socialist one? Well, we're gonna spend a whole nother episode talking about empirical Bayes estimation and Factor score estimation, because it's a fascinating topic in and of itself. But if you run a growth model in the structural equation model, and we talked about predicted scores as Factor scores, you run it in the multi level model, and we talk about them as a thing called empirical Bayes estimation, we're going to get individual estimates of your intercept and of your slope, but they're going to be a joint combination of your repeated measures, but also the summary statistics of the sample as a whole. And so now, my intercept and slope are impart informed by the means and the variances of everybody else's trajectory,
Greg:in the socialist view of this thing, the intercept and slope, they are factors. And in order to get scores associated with those, and really what we mean is score estimates, because we're not going to be able to get some true intercept and some true slope. In order to do that, I actually need the model and the model itself couldn't have existed without the information from everybody to get what that model is in the first place. And so now, when you estimate my Factor score for an intercept, and a slope and your Factor score for an intercept and slope, it could never have been done without the information from everybody feeding into that model in the first place. I am
Patrick:very fortunate to have a colleague of mine here at Carolina, who actually was the guy who hired me back in the day, but Dave Ellison, I gave a talk to the area, and I did a libertarian kind of view on the individual trajectory estimation. And he, over time has really drawn me to these empirical Bayes estimates, because to me, it just seemed inherently unfair, that your trajectory would somehow impact my trajectory. Yeah, there are many reasons why you would want to do exactly that. There's a thing called a shrunken estimate. And that is, the farther away you are the more sparseness that you have in your data, you're pulled back toward the mean, right? It's like whatever the gangster movie, you tried to leave, and they pull you back in whatever it is. Just when I
Insert:thought I was out, they pull me back in.
Patrick:But you're drawn back to the mean. And that always bothered me deeply. But what you conceptualize is, look, you hooked your wagon to a donkey, that you are sampling from a homogeneous population that is governed by some growth process, and you're observing individual variability in those trajectories around that growth process. And logically, it makes perfect sense to use the information about the sample when you're estimating these individual trajectories. And so just know that if you do an OLS trajectory estimate, that's each case, in isolation of all other cases, if you get a Factor score, or an empirical Bayes estimate out of a model that you fit into the data, that's a joint contribution of the individual data and the characteristics of the sample.
Greg:And I'll tell you what, if there's this libertarian inside you that is bothered by that, it would have to bother you with z scores. Also, because we cannot compute a z score without knowing all the rest of the scores and the distribution to figure out do I have a high Z score? Do I have a low Z score? So the idea of you doing something in reference to all the other information is hard coded in everything we do. From the very first course it doesn't bother me,
Patrick:again, going back to a one predictor regression where you get the residuals out, yeah, you have Y minus Y hat, Y hat is based on x prime x inverse x prime. Why? I mean, it's the same gig totally. Here's something that's really important. It is a double edged sword on this. We are not arguing against the OLS estimates, because what is the trailing edge of getting a Factor score or an empirical Bayes estimate from a model? Well, those means and those variances that you're using for the sample to impart inform your individual trajectory estimate are based on a model that you defined. What if there's a subset of individuals who are governed by a different growth function, who what we want you to think about is what are you trying to achieve when you consider each of these approaches in isolation? And indeed, I gotta tell you in my own work, I use both a lot of times you know, what I'll do is go back to the 200 person five time point example. I don't know what your workflow is on this, Greg, but what I often do is before I fit any models before I consider whether to do an SEM or an MLM or to consider to do a linear or quadratic or whatever it might be, I will do individual regressions, get individual trajectory estimates and plot them one person at a time. And I will page down case by case, and just see what the data look like with that individual line. And they're going to go up and they're going to go down, and they're going to tilt and they're going to do whatever that they do. But it's a model three insight into what am I repeated measures look like? It's just orienting to the data, then a lot of times, I'll just hold the page down key. And it's like one of those old degiro type movies, right, where it's like, man doing somersault. And a lot of times, it's like, oh, okay, I see most of these are linear. I see most of these are going up and down in some generally random way. But a lot of times, you can say, Wow, some of these look like they're linear. And some of these look like a line may not be a good estimate. Yeah, it's no model fitting, it's just okay. Maybe this is a reflection of my own personality. It's orienting to the enemy on the field,
Insert:sir. Overwatch reports and F 14 Tomcat is airborne and on course for our position. Mavericks.
Greg:There's so many ways you could have said that other than that, yeah, yeah. You know, if we think about modeling as this endeavor that we go through, there are some people who might be ill at ease with what you just described, some people might think that that's fishing through the data to get a sense of what the growth process is like. And then subsequently, you've had a growth process to see like how well it fits like, well, of course, you picked it to fit that. On the other hand, I think it's grounding in terms of am I even barking up the right tree here and thinking about this, I think it is a very reasonable first step to, quote, look at your data. The trick here is that you mean looking at some description of your data, you took the spaghetti plot, and you broke it out into one piece of spaghetti at a time as you went page down, page down, page down, we can imagine all of those superimposed onto a particular plot. I think it's a very reasonable first activity, it would be like if you had no variance in a variable, why would you try to predict it? Well, let's take a look at the variance of a variable. We're taking a look at the nature of the growth to try and see whether or not it's even reasonable to talk about growth as a process that describes what's going on. I
Patrick:would never use these individual OLS trajectories to guide the model that I'm going to fit. Sure. But what it is, is it's like a data screening device. Yeah, we should all be looking at univariate distributions and bivariate distributions and potential outliers, before we ever fit a model. Yeah. And you know, where I see the danger is not Oh, I knew it was a linear the whole time, I'm going to do a series of likelihood ratio tests as I build functions anyway. So I think that's a moot point as I'm never gonna be parking or parking or parking or whatever. The bigger danger, and I have found this in my own work as well, is you go straight to the growth model without doing this initial step. And oh, my gosh, your growth model can cloak a problem in a way that you would never know was there, you fit an intercept, only you add a line, you add a curve, you do likelihood ratio test, you back up and say of all the options, linear is the best fit, you could have a subset of cases in there that are doing something very different and not be aware of that.
Greg:Totally. Yeah, I mean, think about it from an outlier perspective, where, when you're talking about univariate, procedures, or even multivariate procedures, you will often try to visualize or compute a way that a case stands out or doesn't stand out relative to the others. How do you do that with regard to align? What do I have to look at and what you're describing is something that makes sense so that you can even visually identify whether it looks like the population you have is homogeneous, or you got some weird folks. And when we say weird, folks, we mean weird folks in terms of a line, which otherwise wouldn't be in your data.
Patrick:Those are the advantages of the individual estimates. Now we go through all our usual model building. Now let's say we do our full bore growth mod, and we build an unconditional growth model. And we identify the optimal trajectory. And we bring in time invariant covariance and time varying covariance, we do everything that we would do. Now we can get model based trajectories, that it is absolutely the individual's raw data, but it's in part influenced by the characteristics of the sample as a whole. And we can gather all of those together and look at them and I gotta tell you, those are the ones I think we should use more in practice. I see the individual OLS as a data screening and initial date. Equality thing, the model implied, I think there are multiple advantages, you can get some pretty opaque results from this model, you get fixed effects, you get random effects, you get covariances of random effects, you get residuals, you get all of our usual parameters. And if you're doing a more complicated model, these tables start breeding like rabbits, where every factor has a mean every factor has a variance, every factor has a covariance with every other factor. And it can get pretty opaque, both in communicating that to a reader, but also, in developing an understanding of the confidence that you have, that you've appropriately modelled the characteristics of the data in your sample, but being able to output these individual trajectory estimates, and then take those out in the garage with your Coors lighting Green Day. And just play around with those, I think is a huge advantage that many people don't capitalize upon when they otherwise could.
Greg:So on the back end, what you're talking about is now actually putting the individual in the individual. Right, right, right, which is, we talk a good game.
Patrick:Exactly. It's the green light button all over again,
Greg:you are all individuals.
Insert:I'm not?
Greg:How do we get it back to that individual level? And why would I want to do that
Patrick:the getting them is easy. In an SEM, any program will read out Factor scores. And the same with the multi level model is any multi level program will give you empirical base estimates at both level one and level two. What we're talking about here is level two empirical Bayes estimates, which are getting case based estimates of the random effects. And those are the intercepts. And those are the slopes. So go to your favorite program, look up how to get these estimates and you got
Greg:and don't forget their estimates, please don't forget, there are only estimates
Patrick:that is huge, right? Because they are latent, right? We can't see it, we're estimating in regression, we actually don't estimate the residual, we compute it. Because we have y and we have Y hat and we take the difference between the two and that's e here. These are estimates. And what that means is there is no single way of going about doing it in any way that we do is flawed in some unknown way. There's an unreliability in the estimate of that. Exactly. And that's reflected in the SEM literature. Is there many, many different ways of getting Factor score estimates, there's not one way. So these are estimates. Now you've done your modeling, you get these case based estimates. Now, what do you do with them? In my own work, I try to do two things. One is I am a massively huge fan of the pokin stuff, then we've talked about this before, as you're going for a hike with your kids and everybody gets a pokin stick, because the world is just an absolute garden of things that need to be poked.
Greg:Did you tell your kids this early that they should go around and poke everything?
Patrick:I mean, oh, and we've been stung and bit and I mean, there's some life lessons in there as well. What is a pokin? Stick is all right. Again, as you're listening, think about something that is near and dear to you. Is it your masters, your dissertation? A manuscript, you've been working on a long time, you've got your final growth model, you've got your tables, you've got your fixed and random effects. How confident are you that you've met all the assumptions of the model that there are no outliers that there's no subgroup heterogeneity, all the things that we worry about? I love using these case based estimates for diagnostics. You've got them in your back pocket. I'm a very pragmatic guy, I've got to see stuff, I've got to touch stuff, I've got to feel things we can talk about the means of the slopes and the variances of the slopes. I want to cut it open and look inside. And when you have all your intercepts and all your slopes, well, now you can go to work with those you can rank order them and look for potential outliers or influential observations. What if there's somebody in there who has a very steep slope relative to everybody else? Well, I might go in and pick that kid off and re estimate the model as a sensitivity analysis to see if my fixed and random effects vary. I gotta tell you these models are persnickety
Greg:as hell surprisingly, you might think that they're robust because they're somehow aggregating overall people. You get a weird line in there and things change.
Patrick:I gotta tell you, you are damn right. I have done a lot of these models over a lot of years and it is not uncommon where you identify by one or two cases that you drop, and the variance estimate of your slopes that you are going to center, your discussion around goes to non significant. These are very sensitive to outliers. Well, if you don't estimate these individual trajectories, you never know those exists. Now, it's a whole nother conversation of Do you delete that case? Do you inform the reader? And again, I don't want to get into that rabbit. Warren of okay, so you find three outliers, what do you do with them, but at least you know, they exist. I have one application where I was so excited in the results of my model, and everything was just how I wanted and I was gonna write this wonderful discussion section, I went through this endeavor that we're discussing. And there were three cases that everybody else was growing, and they were dropping. And you plot these on a single plot, and here are these three kids who start really high, and then drop precipitously. I omitted those three cases, and all my growth effects went away. And what happened is those three kids moved to juvenile detention. That's why their trajectories dropped in the outcome is that they were locked up in juvie. Is that the population to which I want to generalize? Well, no, you would not know that I would have written my discussion of it was the best of times, it was the worst of times in these results that were all driven by three kids.
Greg:And I really want to underscore that thing that you're saying. We're not suggesting that you go in and go, and I don't like that line. And I don't like that line. And I don't like that line. What we're suggesting is that this might be a way to be able to identify cases that are not part of the population to which you wish to generalize. Patrick didn't just look at those lines and go, Oh, looks like juvie to me, Patrick went to those cases, got additional information that helped him to understand why that was going on with those cases, and then made a decision based on the purposes of the study to pull those out. This is not different. In terms of how you handle things with regard to outliers. In other settings, you always have to ask yourself, What am I generalizing to? The challenge is we can't see lines unless we start getting some of this information. And that's what this is helping us to do.
Patrick:And I think that's a really nice way of casting it, you don't have access to these individual trajectories in your fixed and random effects. You get them you look at them. And now you're informed. To me, that's the huge thing. I now know this now, it's an entire different conversation of what do you do with that knowledge? Yeah, but you haven't. And I would infinitely rather have the knowledge and then struggle with what to do with that, rather than not know it at all. And the fun thing about these is, you've got these in your back pocket in your data file. The first column is biological sex. The second column is education. The third column is was I in the treatment and control. The fourth column is my intercept. And the fifth column is my slope. And so now you can get really creative. So sometimes I will rank order the intercepts and the slopes from smallest to highest, it'll pick off the top and bottom 1%. Alright, it's pokin stick, would I write the same discussion section, if I picked off the extremes, if suddenly my theory was supported? When it wasn't before, I'm not going to omit those and never tell the reader blah, blah, blah. This is just know your limitations know the sensitivity of the model, we make assumptions about the distributions of intercepts and slopes, right. These are sometimes buried in the fine print, but in all the models we do we assume that the intercepts are normally distributed, the slopes are normally distributed, the intercept and the slope is linearly related that can be captured in a covariance, do a bivariate plot of intercepts and slopes. picture in your mind's eye, the x axis is intercept, the y axis is the slope, and you've got a scatterplot of how those two relate to one another. These are hugely advantageous for checking model assumptions, we make assumptions about homogeneity of variance. Look at the distribution of intercepts and slopes with a box plot for treatment versus control for male versus female are those box plots more or less similar to one another? It's old school diagnostics. But at the level of the trajectories,
Greg:you made a really good point that we assume that these latent variables might be normally distributed. But imagine, for example, that you are modeling alcohol consumption in adolescence and you run a model that is the equivalent of fitting lines to each of those and your distribution of intercepts. If you drew it out as though it was normal, you would find that some kids are starting off in third Rate drinking negative five drinks per month. And the numbers on the distribution make no sense given your substantive knowledge, obviously. But if you just assume things are normal, then it doesn't make sense. If you do what Patrick's talking about, you might see the heavier skew, right? This isn't just oh, this is something interesting to do. This is part of your understanding of the phenomenon that you're trying to study.
Patrick:To be super clear, this stuff has been around for 20 more years plus, routing, Bush and bright have an entire chapter in their multi level book. It's hierarchical linear modeling, I think it was 2002. It's almost 20 years now. But if diagnostics for a multi level model, all of this stuff applies. Even if you have nested structured data, kids within classrooms, you can get classroom level estimates of the random effects. And they walk through a really nice diagnostics in much the same way that I'm describing now with the growth trajectory. So none of this is new. But that's only half of what I like about it. The first half is how confident are you in the stability of your model, you do whatever you do with those diagnostics. And now let's say you and I have finalized a model that we have confidence in, we believe it's stable, it's not being driven by a small number of outliers. We're meeting the assumptions we're done and done. And now we want to open the cage and release it into the wild. By the way, yesterday, I caught my first squirrel. I sent you a little video clip of that. Yes, you did. Yeah.
Greg:This is what your life becomes, by the way, for those of you who are out there, this is the excitement that goes back and forth between us, Patrick shows me animals. Oh, it's the first squirrel of the season.
Patrick:Yeah, I live on two acres of wooded land, and I've decided to remove all the squirrels. Anyway, we're going to open it and release it into the wild. Now, it is our ethical responsibility to communicate those results to a broad readership, who are going to consume our findings, we give a table of means and variances and covariances. And some are raw and some are standardized. Imagine taking those and then augmenting it with graphics. So one thing I love is the fixed effect is what is the average starting point. And what is the average rate of change make an XY plot where we put the fixed effect on it a starting point of 1.6 100 increases point oh seven per year, but then overlay a random sample of individual trajectories. Oh, these are beautiful plots. And you can augment an obtuse table with these graphics that say here is the model based estimates of the individual variability of the trajectories within the control group. And the panel next to it is within the treatment group, you have a dark line, that's the fixed effect within control and the fixed effect within treatment. And you have the individual trajectories around the control, and you have the individual trajectories around the treatment. It brings your opaque table to life. I like
Greg:that idea a lot, especially because we have an inherent sense of growth modeling. But at the end of it all, we talk a good game about modeling individual processes. But the parameters that we focus on are more descriptions of what's happening across those individuals, rather than taking the individual differences into account. So what you're describing is a way to start visualizing. Are those individual lines really tight to the overall trajectory, are they all over the place that is really valuable information. And it's so much more meaningful to visualize rather than just having a variance of a slope or a variance of an intercept.
Patrick:And it's no different than any of our traditional models that are the same thing. We have a variance of a residual, what are the residuals look like? It's just all we're doing is scaling that up a little bit. It's not even that much. We're instead of individual residuals, we now have individual intercepts, individual slopes, another thumb tack I want to put into maybe a future topic. I will make one recommendation for what not to do with these trajectory estimates. Ooh, and we will come back and revisit it on some future episode.
Greg:cliffhanger. I love it. You
Patrick:are going to have a siren song. Alright, so Greg is Lashed to the mast and is sailing by the sirens so that he can hear their beautiful song
Insert:asleep. My name is Ulysses Everett McGill, asleep about the devil nice three.
Patrick:You've got everybody's intercept. You've got everybody slope and the sirens are singing. Use them as predictor variables, use them as outcome variables and Odysseus is going to go right by To the sirens, and is going to say in 2000 years, we're going to know why not to do that
Insert:join you to ignorant fools and ridiculous superstition. Thank you anyway, you boys are dumber than a bag of hammers.
Patrick:I am going to leave with a cliffhanger, you may be tempted to use those as IVs or DVS and other models and we cannot recommend strongly enough to not do that. And there are statistical reasons that we'll talk about on another day, but do not use them as predictors or outcomes in a subsequent model.
Greg:Wait a minute, do you think that I'm not developmentally ready to be able to handle that information? Is that what you're saying?
Patrick:You know what I'm gonna need to push the episode is over button, because I think we've talked enough so let me get under my desk and Goodbye, everybody.
Greg:Dad, don't push them.
Patrick:Thank you so much for listening. You can subscribe to quantum tude on Apple podcast, Spotify, or wherever you listen to audio intended to punish your children while riding in the backseat of your car. And please leave us a review. You can also follow us on Twitter we are at quantity food pod and check out our webpage at quantity pod.org for past episodes, playlists, show notes, transcripts and other cool stuff. Finally, you can get cornered to the merge to secretly connect with others who also squander their valuable time at Red bubble.com Where All proceeds go to Donors Choose to support low income schools. You have been listening to quantity food, characterized by more fabrications and embellishments than George Santos his resume. quietude has been brought to you by quiet quitting and academia a phenomenon for which our lawyers have stated the quantity of takes no responsibility and vehemently asserts that it is pure coincidence that both words start with a cue by Wednesday's viral Tiktok dance. Honestly, we're just trying to associate us with the video to burrow ourselves into your brain. And we conclude with a quantitative public service announcement. Although Patrick does indeed catch squirrels in his backyard. These are safely captured and are immediately released into Dan Bauer screened in porch. This is most definitely not NPR