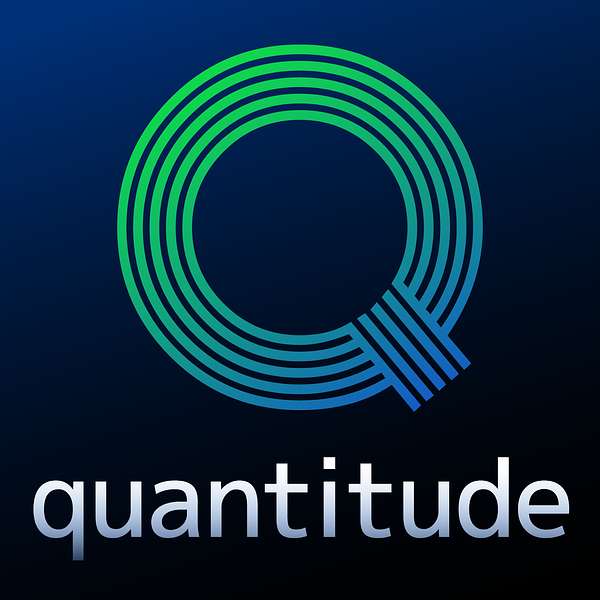
Quantitude
Quantitude
S4E15 Flipping Our Hypotheses to Test Equivalence
In this week's episode Greg and Patrick discuss how we might flip the traditional null and alternative hypothesis testing procedures to move us from tests of literal equality to tests of practical equivalence. Along the way they also discuss tough love, horseshoes and hand-grenades, Patrick’s Driving School, Cheyenne Mountain, So Long and Thanks For All the Fish, isn't that convenient, why people hate us, systolic blood pressure, *real* doctors, I Can’t Drive 55, splash zones, Gallagher, Dilbert, and being precisely equal.
Stay in contact with Quantitude!
- Web page: quantitudepod.org
- TwitterX: @quantitudepod
- YouTube: @quantitudepod
- Merch: redbubble.com
Welcome, my name is Patrick Curran and along with my dolphin loving friend Greg Hancock, we make up quantum tune we're a podcast dedicated to all things quantitative ranging from the irrelevant to the completely irrelevant. In this week's episode Greg and I discuss how we might flip the traditional Knoll and alternative hypothesis testing procedures to move us from tests of literal equality to tests of practical equivalence. Along the way, we also discuss tough love horseshoes and hand grenades. Patrick's driving school Cheyenne Mountain So Long and Thanks for All the Fish. Isn't that convenient? why people hate us systolic blood pressure, real doctors, I can't drive 55 splash zones, Gallagher, Dilbert and being precisely equal. We hope you enjoy this week's episode.
Greg:It would be an understatement to say that you have had a lot going on lately. Am I right? That is right.
Patrick:We've got two root canals a lip surgery and a major shoulder reconstruction.
Greg:Poor guy but if I may, that's not all.
Patrick:No, I got some sad news listeners is a presents over the last three and a half years on the podcast was my 90 year old mom. And indeed mom was on an early episode with Aunt Joanne. Mom through the beauty of your Irish accent kept sending me for a pack of smokes, and was with Aunt Dottie as part of the funeral for MANOVA. But in the first part of December, my mom passed away. So sorry, thank you. She was one of seven born in a period of nine years. Wow. on a dairy farm in Wisconsin in the 1930s. She and her two sisters went to college, the three farm girls pooled their money, bought a car and we're going to drive to California, two or three B teachers. And in Denver had a car accident. My mom was pretty seriously injured. They had to stay in Denver for her to recover and the three of them never left. She was a high school teacher, retired, became a published author and was an absolutely remarkable woman and she will be missed.
Greg:Well, I will always remember that she desk rejected the limerick that I wrote for her to do on our episode.
Patrick:Early on wanted her to read the limerick as part of that episode and Greg wrote it out. I printed it. I was in Denver, my aunt Joanne was there as well gave it to my mum read it and said, Yeah, this is no good. I'm gonna rewrite it come back later this afternoon. And I thought Welcome to where I came from as a human being.
Mom:And oh, Patrick, this is your mother. I have a limerick I would like to share with you and Greg, There once was a quantitative mother whose two sons outdid each other that Pat knew he was through when she said, Why can't you get a real job like your brother? Don't forget to call on a Sunday. Honey, I have a question about My Computer.
Patrick:No offense to your Limerick but I think it came out in
Greg:well, what would we do? Would we raise a pint of Guinness to your to your mom? Is that what we would
Patrick:do? And then you can send me for a pack of smokes. So anyway, thank you for your thoughts, and she will be deeply missed.
Greg:So now that you yourself are getting a little bit older, do you see some of her and you like how you do things? How you parent your kids?
Patrick:Absolutely. There are two things that I see one that lock stock and barrel I got is a sense of tough love. I've told this story before where my kid was running barefoot stubbed their toe ran crying mom brush the hair out of their eyes and said, Well, honey, that's why God made shoes. I got that. Which as you are aware of this story translated where my kids were training for a 5k one of my kids was complaining about how her leg hurt. I said yeah, you hurt when you run, take an Advil go to bed and two days later we found out she had a fracture. So there's that element. But another one that I really admired with her is you can make a lot of progress with close enough that notion of don't let the perfect be the enemy of the good. That that is close enough that we can do that and move on to other things. My Limerick notwithstanding, okay, there is a catalogue for here that it can be within the caliper.
Greg:You're saying it wasn't even close enough. Limerick
Patrick:was outside of the caliper man but a really funny thing happened a while ago where I saw this with my own kids. My kids are 18 now they've been driving for good You're a year and a half. But when we were teaching them to drive, there's the letter of the law and how do you drive, but there's also the spirit of the law. Hmm. And it involves speed limits. And I made a comment at one point when I was teaching the kids how to drive that there's the posted speed limit. But if you have 3545 55, you've got 689 miles an hour that you can go over that before a cop is gonna bother pulling you over to be clear,
Greg:you're offering advice, and you're the one who could wallpaper your entire office with traffic tickets. Am I correct? On that point,
Patrick:I could open my own driver's ed school based on how many I have attended,
Greg:okay, by all means, give your kid advice.
Patrick:The problem was they encoded that as that was actually a law. If you had a posted speed limit, you could go 10 miles an hour over illegally. And this would not have been a problem if one of them had not raised this in driver's ed class as a fact, and they came home and I got excoriated because they got laughed out in the class is that if it's posted 45 Well, legally, you can go 55 Because my dad said so my dad said so. So yes, that notion of how close is close enough?
Greg:What's the old saying close only counts and what was the version of that that you grew up? Hearing close only counts in
Patrick:horseshoes, hand grenades and nuclear war. Wait, you got
Greg:nuclear war at the end that was added. Okay.
Patrick:NORAD is the home of the United States Nuclear tracking, and it's in Cheyenne Mountain. I grew up like 30 minutes from Cheyenne Mountain. And there was always all this talk of Oh, the Russians are going to put a missile through the front door of Cheyenne Mountain. And when the Soviet Union fell, and all the secret documents became available, the Soviet Union couldn't have hit Colorado, much less the front door of Cheyenne Mountain. So yeah, close enough for horseshoes, hand grenades and nuclear war. Okay. You know,
Greg:believe it or not, all of this actually relates to hypothesis testing.
Patrick:Really? All right, I'm gonna refill my coffee here, and I'm gonna see how you're gonna relate my mother to speeding to nuclear war? Go? Okay.
Greg:Let me start with this particular question. When you reject a null hypothesis, like for the difference between two means, what do you actually conclude? What are the words that you teach your class, when you reject the null hypothesis,
Patrick:if you get a P value less than alpha, which we will say is Oh five, I would say it is unlikely that you would have observed a difference between your sample means this large or larger, if there was truly no difference in the population, and therefore you infer that I would have a probabilistic basis to reject the null that they are precisely equal in the population and therefore are different.
Greg:All right, so that there's some difference, because it's kind of hard to believe that there's no difference. You just rejected that. And you would do the same thing for a correlation, right? When you get a statistically significant correlation coefficient or predictor in regression, you wind up saying something that, you know, if it were the case, that that correlation, were really zero in the population or that predictor really had no predictive value and understanding why it would be very unlikely that I would have observed this magnitude of relation. And so I reject that No. Or sometimes we say nil hypothesis, right? Because there's nothing going on in favor of saying there is something going on.
Patrick:Well, that's the cowardly part. We've talked about this before, which is either the means are precisely equal or they're not.
Greg:Yeah. So then let me flip this on you. And I'm not trying to pop quiz you here. I just want to hear the words that you say. So that was when we reject a null hypothesis. What words do you use when you don't show that? That's a good one.
Patrick:Well, from a statistical standpoint, there is insufficient empirical evidence to say that my observed difference between means would have been unlikely given the null hypothesis, that statistical substantively what I would say is from a Popperian standpoint, we have insufficient empirical evidence to falsify or no hypothesis
Greg:Wow, that was a clinic on why people hate us. Did your mom teach you that? Thanks mister see mom
Patrick:also Okay, so for talking parenting. Here's another one I learned from my mom and folks for Those of you have young kids, this is an awesome one. And you can think Dolores, for this. Kids is all about making their own decision. So Greg, it's completely up to you. Do you want to take a bath before dinner? Or do you want to take a bath after dinner? You decide cuz you're a big boy now. I was like, 17, when I was like son of a bitch, I'm taking a bath either way.
Greg:Well, you know, the cagey language that you use, in the end, when you retain the null hypothesis, we find ourselves in this really weird place. So I like what you say that, well, we didn't have enough evidence to reject the null hypothesis, people are often tempted to conclude that there is no difference, then write that if we retain the null hypothesis, and even the language that I'm using is intentionally guarded. Some textbooks will actually say that we accept the null hypothesis, and I hate that language. That's just incorrect. Yeah. Right. Because that almost gives someone license to say, well, I guess the means are equal, then, you know, I grew up softening it to retain but there really is merit in saying clumsily, that we failed to reject the null hypothesis that we failed to find evidence of a difference, which is not the same as saying there's no difference. But I will tell you, there are other times when we do exactly that, we make that exact inference. Think about if you were doing a classic t test, right, just a pooled variance t test. Some people just plow right ahead and do that t test. Other people will say, Well, you know, that rests on an assumption of homogeneity of variance with a Okay, good. So how are you going to test that assumption of homogeneity of variance? And the answer might be doing an F test ratio of the variances of the two independent groups, it might mean doing a Levine's test. But what happens when someone doesn't reject that test? What do they do? They plow right ahead with the pooled variance t test thinking that, Oh, I guess the variances in those populations aren't different. So I've met the assumption that underlies that particular t test. And when they were testing the assumption of homogeneity of variance, they actually took a failure to reject as saying, Oh, I guess things are equal. So we do exactly that.
Patrick:And we also pick and choose, right is that if we fail to reject the null of homogeneity of variance, that's because the variances are equal. But if we fail to reject the equality of the mean, it's because we don't have sufficient power to detect the effects.
Greg:Isn't that convenient? Yeah, we do that with invariance testing, too, right? That we wind up setting up this measurement model for these different groups that we want to know that these variables are loading the same. And in the end, we wind up doing a test where we might have loadings constrained across these populations against a model where the loadings are not constrained. And if we get no significant difference, what do we say they're equivalent across the two groups? There you go. And so we have this logic problem, where we're using the non significance of a test as evidence that there is no difference. And we're doing exactly what you say we're sort of picking and choosing right there are these times where we are kind of sort of hoping for equivalence. So we take that failure to reject as evidence of equivalence. And there are other times where we kind of sort of really want a difference, and we failed to find it. So we say, well, we just didn't have enough power to find it. How would
Patrick:you differentiate equality from equivalents? Because you've been like a dolphin in and out of these terms? Okay. Up, down, up, down, up, down. Have you seen the movie Hitchhiker's Guide to the Galaxy? Read the books saw the movie? Well, the greatest opening film sequence of any movie is that, folks, if you haven't seen this, either watch the movie, which is brilliant, or go to YouTube and watch the opening. It's a song about dolphins, and it's so long, so long, so long. Thanks for all the fish.
Insert:The last ever dolphin message was misinterpreted as a surprisingly sophisticated attempt to do a double backward somersault through a hoop while whistling The Star Spangled Banner. But in fact, the message was this So Long, and Thanks for All the Fish.
Patrick:Thanks for all. So sad that you come to this. Anyway, equality versus equivalence go.
Greg:Oh, so you kind of saw through everything right there. I don't know if you got that from your mom, you must have because you couldn't have come up with that on your own. Yeah, you're exactly right. So when things are equal, or have a quality than they are dead on those means are exactly the same. That correlation is exactly zero. That predictor has exactly no contribution. Those loadings are exactly the same across the different populations. But equivalence to me has a little bit more fuzz and it feels a little bit more like was it Annie? Who was the one who was driving yes and right. And so if we say the speed limited Is this 55? I mean, is it exactly 55? Is it 55 plus or minus five miles an hour plus or minus 10 miles an hour, there's a very specific definition of limit. But in practice, there is this practical equivalence to 55 miles an hour. And I think our hypothesis testing lives are so set up to aim everything at rejection, we forget that there are a lot of cases where we are more interested in the equivalence of things, then we are actually in establishing a difference. But we can't use the hypothesis testing framework the way it's currently set up to try to get at that. And these kinds of ideas of equivalence come up all the time. You know, like when we're testing assumptions of things, or imagine we have intact groups that we are doing as part of a study. But we want to know whether or not those groups are equivalent at baseline. More broadly, if we are in the pharmaceutical industry, and we have a new drug that's a lot cheaper, we might want to know whether that drug performs the same or at least equivalent to some existing drug, does it lower blood pressure, if not exactly the same amount as a more expensive drug? Close enough. So this concept of equivalent exists all the time and things that we do, but we haven't really turned our hypothesis testing lens properly on these kinds of research questions.
Patrick:And the end of the day is how big is big enough right to say that there's a difference that's meaningful and worthwhile,
Greg:which is something that we have to do all the time, when we're talking about power analysis and planning for a study in which our goal, our hope is to be able to find something. But here, we're going to flip it the other way, and talk about what it means for two things to be equivalent. So both of our kids took the LSAT last year. And if they wanted to take a prep course, they could have taken very, very expensive prep courses, they could have taken cheap online stuff, you know, someone might make the claim that our online materials are as good as one of those really expensive courses. Well, what does that mean? Does that mean that the scores you get under one course are exactly the same on average as the other? Or might we call them equivalent? If on average, they don't differ by more than 10 points or 20 points, we could define what that threshold is for what we might call practical equivalence. Or if there are two medications that are supposed to lower systolic blood pressure or diastolic I get those mixed up which one is which one is which? Yeah, which
Insert:shivers the bad one. Hi, this is Dr. David justice, MD board certified pediatric hematology oncology and Transfusion Medicine Physician at Boston Children's Hospital, epidemiological and treatment studies suggest that systolic blood pressure should be the primary target of antihypertensive therapy, although consideration of systolic and diastolic pressure together improves risk prediction. Come on, guys, you can just google this stuff like us
Greg:real doctors do. Well, thank you real doctor. But if there are two medications, we might say, yeah, these medications are practically equivalent if systolic blood pressure is within 10 points, or if you are correlating two variables, and I say I think those variables correlate zero. I mean, they probably don't correlate exactly zero, but maybe you define zero is within plus or minus point one of zero or plus or minus point oh five of zero. So the point is that we can define what we mean by equivalence, we can define that band around your speed, your your speed limit, which seems to only be an interval on the upper end, we don't tend to worry. No,
Patrick:but that's a real thing. I like to think of it as a caliper. Like if you're within this tolerance, however you define that. We're just going to consider those all to be the same. You're going 6566 67. If we had sufficient equipment with sufficient accuracy, we could differentiate 67 from 66 miles an hour, but nobody cares. If you're going above 56 miles an hour and below 74 miles an hour. Nobody cares. Yeah, that caliber, but if you're going slower, you might get pulled over because you're impeding traffic. If you're going faster, you're gonna get pulled over because you're driving too fast. But it seems that Howard Waner It don't make no nevermind. If you're under 10 miles an hour of the posted limit, you're probably going to be fine. Nobody cares.
Greg:And so if we want to do a formal statistical test of this, the null hypothesis significance testing that we're accustomed to doing, the way it is framed, we're always aiming to sort of reject out right using the mean difference example or the correlation example. We're always aiming to reject zero or whatever the value is that we're looking at. But we Imagine flipping that test and thinking instead about what I'm really kind of clumsily calling, rejecting in. Imagine that you have set up a null hypothesis. It's a weird null hypothesis, right? The null hypothesis we're accustomed to doing is something like, I'll do one for correlation. For example, our null hypothesis is that row the population correlation is zero. But we could instead set up a null hypothesis that says the correlation between two variables is point one or greater, or to compound No, I apotheosis, negative point one or lower, which means stronger. So imagine the null hypothesis defines this region that's outside where we would say, oh, yeah, that's a real correlation. And then the alternative to that is that our correlation in the population actually fall somewhere between negative point one zero and positive point one zero, a region where we might say, that is practically equivalent to there being no correlation. Well, if we flip the null and alternative hypotheses like that, we can actually use hypothesis testing procedures to try to test whether or not we could consider that correlation to be practically equivalent to zero, where the difference between two means being practically equivalent to zero,
Patrick:and that map's exactly on to this issue by Irish factor loadings are in O'Shaughnessy, Oh, 305. If you have a factor loadings that has a leading digit of zero, nobody cares, right? Oh, 703, negative Oh, four. It's like, yeah, it's just hovering around zero. Nobody cares. But you make some caliper where if the factor loading is above point one, or below negative point one as a cross loading, then I gotta figure out what to do with that. So we think about this all the time, and indeed, we grouse about it a lot. Because in CFA going to that null hypothesis, we say that factor loading is zero. Yeah, and here we're shrugging you work for State University I work for a state university is to say, I don't believe it's exactly zero in the population. But as long as it has a leading digit of zero in the value, I can sleep at night. So there
Greg:are two things going on here. One is setting up what you think that threshold is, or those boundaries are for defining practical equivalence, and then figuring out the appropriate statistical test for you to do or actually statistical tests, in fact, right, because in the example we were talking about, you could be convinced there actually is a nonzero correlation if it was something sufficiently positive, or if it was something sufficiently negative. So how would we go about figuring that out? Well, there are hypothesis testing ways to do it. And there's sometimes go by the name equivalence tests. But I think there's a very easy way to think about it just in terms of confidence intervals. And the confidence intervals wind up for most things, accomplishing this idea of having two hypothesis tests that are testing inward from the left and inward from the right. So I want to think about things from the perspective of a confidence interval. First, let's think about the difference between two means. Let's think about the LSAT scores. And imagine we have two LSAT prep courses, and we decide if they produce scores with mean differences of less than 10 points, positive or negative, we will go ahead and call them practically equivalent. Well, so if we go ahead and do a study and create a confidence interval for the difference between those two means that confidence interval actually is informative from a hypothesis testing standpoint. Now when I think about this example, I'm going to think about it in a really weird way. You know, I used to take Sydney to dolphin show. I don't even think dolphin shows exist anymore. Like at our Baltimore aquarium, which is a really nice aquarium. There's no more dolphin show. I sticks Sydney to the dolphin shows. I couldn't take the boys to the dolphin show. So whether we're thinking about a dolphin show, or this is so dated a Gallagher comedy show, do you remember Gallagher at all? Oh, yeah,
Patrick:he smashed the watermelons. Exactly. helped me to despatch this melody It's a little known fact is early in his career, he actually smashed dolphins But Peter got to water. Okay.
Greg:That makes what I'm about to say that much more disturbing. Imagine you imagine you and I are sitting in the front row of whether it's a dolphin show or a Gallagher show or an early Gallagher show. Which is the best of both worlds.
Patrick:You did not want to sit in the front row for his early shows.
Greg:You and I are in the splash zone or at least potentially in the splash zone and I am going Have you seated at a seat that is marked with zero, and that zero represents no population difference between these two SATs prep courses on average, and I am going to go sit out at 10 points. And so we have the dolphin show, the Gallagher show or the combination thereof. And in the end, there's a certain splash that occurs as a result of this. This is disturbing to think about. The question is, how big is the splash? Right? Does the splash include zero? Does the splash include 10 points? Does it include one both neither. And those lead us to different potential conclusions, just like a confidence interval would write so we build a confidence interval around the difference between two means, and it might include zero, it might include 10 points, it might include one might include neither, there are four different possibilities for what we're talking about. The first one is, let's imagine you and I both get wet and wet. Let's just keep it at water or watermelon.
Patrick:I caught the blowhole.
Greg:I'm telling you, the art for this episode is just creating itself. Alright, so imagine that you and I are both in the splash zone. Imagine that the competence interval captures both zero and 10 point difference. All right. So if that's the case, essentially, we can't reject zero as a possibility using traditional null hypothesis significance testing. But we also can't reject 10 points. So this is an example that is not conclusive really, in any way. We can't say anything about practical equivalence, we can't say anything about difference. So we're kind of stuck. So now imagine a different scenario where only you are in the splash zone, the Zero gets wet. The point that says there's no difference between the two population means, but I the 10 point difference am outside the splash zone, I am outside the competence interval, that's equivalent to a regular traditional null hypothesis significance test not being statistically significant because it contains zero. But the equivalence test the test of whether or not it differs from 10 points, that is statistically significant. So in that case, what we can say is that we have rejected the idea of it being 10 points in favor of it being something smaller, we have determined no practically important difference between the two. So let's flip that now. And imagine that you didn't get wet, you're not in the splash zone, but I'm in the splash zone. So zero is outside the competence interval. But 10 points is in the confidence interval. That means that a traditional null hypothesis significance test is statistically significant. And it rejects zero, but hypothesis test does not reject 10 points. So in that case, we would say something like, well, there is a difference. But we can't say if it's practically important, right? And I have to be very careful in my language, because I didn't establish that it isn't practically important. I just don't have enough evidence to be able to say that it is trivial or not trivial. So in that case, I wasn't able to establish anything or make any comments specifically about practical equivalence. Exactly. equivalence, yes, but not practical equivalence. And then the final case is when the splashdown doesn't get either of us wet, do you as zero are sitting outside the splash zone I at 10 points. I'm sitting outside the splashdown. So it's a little splash that came between zero and 10 points. And what that means is that a traditional null hypothesis significance test rejected the idea of there being no difference. So we believe that there is a difference. But we also believe because the confidence interval didn't include 10 points, that it is not a practically important difference. So yeah, we have evidence that there's a difference. But we also have statistical evidence that it is not a practically important difference. And so from a practical standpoint, we might be able to consider these two essay T prep courses equivalent. So it's a very easy way of taking confidence intervals to essentially be conducting different types of hypothesis tests, and then being able to reach conclusions about not literally equality, but practical equivalence.
Patrick:And this is such great fun to think about, because one is we're expanding the usual null hypothesis testing in ways that we sometimes don't think about, or in fairness to folks out there are not taught about, but also Greg has been very careful in his language about what is meaningful, and this becomes an inherently subjective, theoretically motivated determination. And this gets really interesting really fast. And it does make me think of Dilbert. So I'm a big Dilbert fan. And I have this cartoon on my door for a while he goes bungee jumping, and the guy is tying them up. And the guy says, How much do you weigh and Dilbert says, Why do you need to know? And he said, well, it determines how much tension I put in and Dilbert says I weigh 700 pounds but I love This topic for these reasons, it's moving beyond the Is it zero? Yes and no. But then it moves us into, well, how big a difference is big enough? And how are you justifying that? Because you might say a 10 point difference is worth the $1,000 LSAT prep course. And I might say, I want 30 point difference. Yeah. Okay. Well, both of those are equally defensible. Yeah, exactly.
Greg:One of the things I really like about this is that it puts the onus on the researcher to define what it means for things to be equivalent. If that seems like too onerous a task, it's exactly what we asked you to do when you're doing a power analysis for a study to decide, what is that minimum detectable effect that you actually care about, we're doing the same thing, it's just that we're doing it for a purpose going the other way, right? Looking at being able to statistically establish something that is under that rather than over that one, although we don't have to, we can use confidence intervals to try to get out that.
Patrick:And I have not always been a big fan of confidence intervals. And the reason is, many people treat them as if there's something unique and novel ly different than a critical ratio. So you have a point estimate a standard error, that gives you a critical ratio, and you look it up in a table and get a P value. And people say no, no, no, or you're a horrible person, you are singularly responsible for everything that's bad in the social sciences. And instead of dividing by the standard error, we should do plus or minus two times the standard error and then see if it contains zero. And I grouse like a grumpy old man, because of course, that is the same thing. You can look at your P value and accept or reject your null hypothesis with a critical ratio. Or you can compute a confidence interval, see if it contains zero, and then accept or reject your null hypothesis is exactly the same thing when used that way. Now, what I'm fascinated with and you actually need to throw me a bone and help me with this. Because as you've been talking, I'm seeing well, there actually is not one test. But there's actually two tests. There's one above and there's one below. Are you in the dolphin Gallagher splash zone? Right, I'm not gonna sleep well tonight, given this whole visualization. But I'm starting to wonder, does our standard confidence interval kinda tell us about both of those at the same time? Or am I thinking about that wrong?
Greg:No, I like how you're thinking about it. When I talked about the confidence interval, I was really just using it as a shortcut way to try to accomplish two hypothesis tests at once. And even though honestly, I talked about it in my example, as informing us about zero, right informing us about that traditional null hypothesis significance test, the two tests that I'm actually referring to are the one above zero and the one below zero. In the LSAT example, we could imagine a positive 10 point difference, or a negative 10 point difference. And really, the competence intervals purpose in the context of equivalence testing, is to conduct a one sided significance test of the positive 10 points in the direction towards zero, and a one sided test of the negative 10 points in the direction of zero. So the confidence interval, whereas we're used to it serving some purpose, that is not really different from a null hypothesis test, here, it actually stands to accomplish both tests of the boundaries of what we would call practical equivalence. So in that sense, I think it's actually really useful as this proxy for those two hypothesis tests. The trick is that the P value that we're accustomed to is the p value associated with that typical test of the null hypothesis of zero. In this particular example, we could imagine two other P values, we could imagine a p value associated with the test of the upper boundary aimed in toward zero, or we could imagine a p value of the test of that lower boundary of practical equivalence, aimed upward towards zero as well, I think in the sense of the confidence interval offering us something different from null hypothesis significance testing, not really, but as a vehicle for helping us to conduct equivalence tests to conduct multiple hypothesis tests simultaneously. I think it actually is insightful. And that's how I was trying to use it here. I don't know if that makes sense.
Patrick:That makes a lot of sense. Thank you for that. Yeah. Where do we go from here? How do we incorporate this into our own work?
Greg:Well, first of all, you don't just incorporate it unless you have a research question or some other type of question that is specifically about equivalence. So it might be the case that you want to test some assumptions prior to doing some other method, whether that assumption is normality, or the assumption is homogeneity of variance or homogeneity of dispersion, like we test with boxes M test, rather than just saying, Well, I didn't get a significant departure from that. So I guess that assumption holds with regard to testing Assam Questions, what we can and maybe should do is figure out what the boundaries are have normal enough or homogeneous enough, and then conduct our test in this flipped way to see whether or not we have violated that assumption. Of course, that requires work on the front end to decide what departures are troublesome, whether it's from normality, or homogeneity of variance. But that's our job. So that's when the tests have to do with testing the assumptions that are associated with other things. But like I said, at the beginning, their actual research questions about equivalence? Is this drug good enough? Is this teaching method good enough, is group therapy as effective or at least close enough to as effective as individual therapy, boy would want to know that because group therapy could be a heck of a lot cheaper? So there are a lot of research questions that are about equivalence. And then it just comes down to the researcher defining in the context of whatever the field is, what stands for equivalence, but you and I know how to embed this in the context of darn near anything, right? We could talk about whether this structural path is zero, or at least close enough, not saying oh, it's not significant, I guess it's zero. But is it practically zero? Or you and I within a structural model could say is this variables value and understanding why practically equivalent to this other predictors value and understanding why that's something you and I know how to do, because we can encode the difference between those two things. Within a structural model. We talked about doing this in our Lego episode, just a few episodes ago, you can code darn near anything you want as a parameter. And then once you have done that, you are able to talk about it in terms of equivalence, we could do it in terms of invariance testing, even if we don't believe that a set of loadings are exactly the same across two populations, is there a way that we could quantify close enough and there are ways that we can quantify close enough, we could quantify it in terms of these loadings are no more than plus or minus 10% of those loadings, that's something we actually could do. These are things where we can re engineer our hypothesis tests to be able to say, All right, I want to see whether or not I can statistically fall within that close enough zone, that practically equivalent zone. And so it has widespread applications. And then we can power for those tests, just like we power for other things. So I think this is a nice complement to the types of tests that we already do very commonly.
Patrick:And we'll put up show notes on this for some of these readings. James Rogers, Kenneth Howard, John Vesey, using significance tests to evaluate equivalence between two experimental groups in 93. So this is 30 years old at this point. And I found this to be a really nice overview of a lot of the things that you've helped walk us through of what do we mean by exact what if it's not exact, what is big enough to be big, and I would recommend looking at that, but really is just thinking about things a little bit differently. I'm looking at the paper, and they have a wonderful figure in here, that corresponds to what you were describing about how you could have a one tailed test on the lower part. And you can have a one tailed test on the upper part. And he shows two distributions, and then combines them into the single distribution. This is really cool stuff.
Greg:I totally agree. So that's equivalence tests. In a nutshell, just taking your good old hypothesis testing skills and turning them in Word on questions of equivalents. It doesn't use any new skills, but it helps you to look at things a little bit differently, and plan to be able to answer those kinds of questions. So it's very, very cool stuff.
Patrick:And I think it's really fun to juxtapose equality with equivalence, because those are not the same. And in the spirit of my middle school English teacher, mom a couple of times during this episode, I have said precisely equal that is sloppy thinking. It is precisely equal is in memory of mom. They are equal. Thanks, everybody.
Greg:Thanks, Mrs. C. Take care, everybody. Take care. Bye bye. Thanks so much for joining us. Don't forget to tell your friends to subscribe to us on Apple podcasts, Spotify, or wherever they go for 100% Dolphin friendly content. You can also follow us on Twitter where we are at quantity pod and visit our website quantity pod.org where you can leave us a message find organized playlists and show notes. Listen to past episodes and other fun stuff. And finally, you can get cool quantity merch like shirts, mugs, stickers and spiral notebooks from Red bubble.com. We're all proceeds from non bootleg authorized merch go to donorschoose.org to help support low income schools. You've been listening to quantitative the podcast where equivalent isn't equal and equal is only equal if it's precisely equal. To close today's episode, rather than having our usual sponsors, I would like to offer a limerick in honor of Mrs. Curran. There once was a mother named Dolores of whose praises We could surely sing a chorus and even without meeting face to face, she has left us all in a better place, giving us Patrick, who will continue to bore us, Mrs. C. I'm sure this Limerick would have been better if you'd been the one to edit it. Cheers. And as I say in Gaelic slung chair