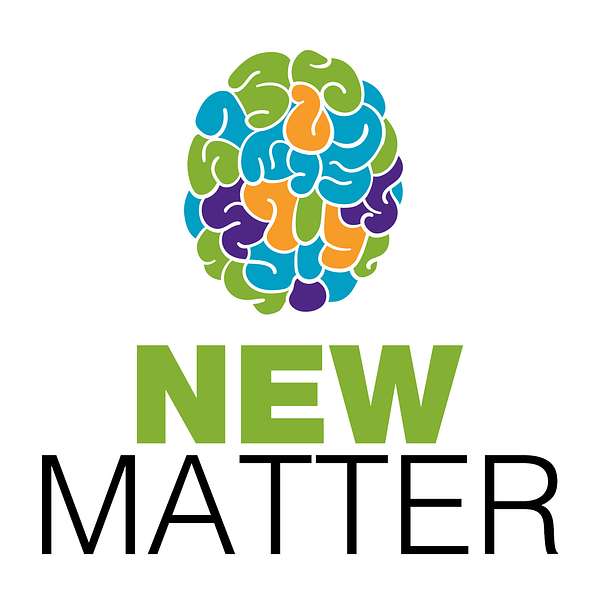
New Matter: Inside the Minds of SLAS Scientists
The official Society for Laboratory Automation and Screening (SLAS) podcast explores advances in automation, cellular imaging, big data and what's coming in the spaces between traditional scientific disciplines. Guests often include members of SLAS along with innovators, leading experts and other members of the global scientific community to highlight technology and even career stories. Episodes are released every week and subscribe to New Matter - available on all podcast players.
New Matter: Inside the Minds of SLAS Scientists
A Lesson in High-Content Image Analysis with Beth Cimini, Ph.D.
In this episode, we're joined by Broad Institute Senior Group Leader and Head of the Cimini Lab Imaging Platform Beth Cimini, Ph.D., for a lesson in high-content image analysis! Cimini is a subject matter expert who shares her knowledge to explain how high-content image analysis works and how it pertains to cell painting.
Key Learning Points:
- The types of research high-content imaging is ideal for
- The process of developing an assay for a high-content imaging approach
- What you need to know to use high-content imaging in your research
About Beth Cimini, Ph.D.
Cimini is a senior group leader, CZI imaging scientist and the head of the Cimini Lab in the Imaging Platform at the Broad Institute in Cambridge, MA. After completing her undergraduate research in visual neuroscience at Boston University, Cimini obtained a Ph.D. in Biochemistry and Molecular Biology at the University of California San Francisco, where she studied the difference between splicing variants of the telomere master scaffolding protein TIN2. She created and directs the Platform's Postdoctoral Training Program in Bioimage Analysis, and also leads the Broad efforts toward community engagement and driving biological projects for the Center for Open Bioimage Analysis.
Stay connected with SLAS:
About SLAS:
SLAS (Society for Laboratory Automation and Screening) is an international professional society of academic, industry and government life sciences researchers and the developers and providers of laboratory automation technology. The SLAS mission is to bring together researchers in academia, industry and government to advance life sciences discovery and technology via education, knowledge exchange and global community building. For more information about SLAS, visit www.slas.org.
SLAS publishes two peer-reviewed and MEDLINE-indexed scientific journals, SLAS Discovery and SLAS Technology. For more information about SLAS and its journals, visit www.slas.org/publications.
For Students:
Visit the SLAS Student Resources to find information on student-focused SLAS awards, grants, scholarships, digital educational content, podcasts and more.
*For a transcript of this episode, please visit this episode's page on Buzzsprout.
Upcoming SLAS Events:
SLAS Europe 2025 Conference and Exhibition
- 20-22 May 2025
- Hamburg, Germany
Hannah Rosen: Hello everyone and welcome to new matter, the SLAS podcast where we interview life science luminaries. I'm your host, Hannah Rosen, and joining us today is Beth Cimini, Senior Group Leader of the Cimini Lab at the Broad Institute. Beth is going to teach us all about high content image analysis. Welcome to the podcast Beth.
Beth Cimini: Thank you so much for having me here.
Hannah Rosen: Now, it's our pleasure! So, to start off could you just kind of give us a little bit about your background in high content image analysis.
Beth Cimini: Yeah, so I came to image analysis via just being a microscopist. My training is just in sort of cell and molecular biology and I wanted to answer very tricky quantitative questions with microscopy and image analysis and found that I actually love doing image analysis and I love teaching others to do it as well. And so when I finished my PhD, I was looking for what to do next and the creators of Cell Profiler, the lead authors, Anne Carpenter, who's at the Broad Institute too, and I was like, oh, teaching people how to do this is a job I... I would love to go and do that. And so, I've been... I've been at the Broad for six years, originally as part of Anne's lab and then my lab split off from hers about a year ago. But I hadn't really thought about high content imaging, so to differentiate that from other microscopy you might normally think about, typically in most microscopy, the way it's done is there are one or two things that, you know, that you care about, and then you design something to look at exactly that. You buy a special probe or you make a special dye and... or fluorescent protein, and then you sort of go look at that thing. With high content image analysis, what we do is sort of the opposite, which is we measure everything and ask questions later, and so the idea is we're not going to make two or three or four measurements from ourselves, we're going to make thousands. And I had never heard of this before starting in Anne's lab, and I was a little bit sort of suspicious that this worked. I was like no, the way we've always done it is good. And then I saw some of the kinds of results you could get, and some of the new experiments you could do by getting thousands of measurements of your cells and then doing things like clustering them. I saw that you could do things that aren't possible looking one or two or three measurements at a time, and so I... I saw the light and was converted.
Hannah Rosen: So yeah, I guess you kind of answered this next question that I had for you already about, you know, when you first became interested in high content image analysis. But can you go into maybe a little bit more detail about, you know, was there any specific project that you were working on that really helped you see the light for high content image analysis?
Beth Cimini: Yeah there were. So, it wasn't a project that I personally worked on, but it was a project that the group was writing up when I started the lab, which was overexpressing... I think it was about 300 different genes in cells, and then using what we call the cell painting assay, which is developed at the Broad by the Carpenter and Triber Labs, and is the most commonly used assay for high content image analysis. And so, this... this is just a bunch of organelle dyes and it's designed to be cheap things that are probably already in the back of your freezer and then just do high content image analysis, measure thousands of features and then cluster things and so they did this on 300 over express genes and what they're able to find is not only could they group genes into pathways based only on these cell painting measurements which aren't specific for any of the pathways. They discovered novel pathway-pathway interactions based purely on the fact that these high content image analysis profiles looked opposite to one another. And that was just entirely blowing my mind because these dyes aren't specific to anything about those pathways, but just by saying, you know, typically when measurement A goes up in condition Y, you know measurement B goes down in condition Z, they actually discovered new biology, and so that was to me, incredibly exciting that, that worked.
Hannah Rosen: Yeah, that is amazing. But you know you say it and, on the one hand, my brain is like that's incredible. But then my brain also at the same time is like, well yeah, that makes a lot of sense that you're gonna learn more about the whole system by actually looking at the whole system instead of just looking at one specific part, right? But it's... it's hard to look at the whole system, I would imagine, in a cell.
Beth Cimini: Yeah, and human brains, it turns out, are actually really bad at assessing information compared to computers. Our brains give an idea of sort of what the general picture is, but they're terrible at details. They can't see textures very well at all, at least compared to the way computers can, and from work that other people have done with high content imaging assays in general, our brains fixate on the wrong details and so our brains get tricked by things that turn out to be sort of red herrings and aren't actually important for understanding the phenotype in question.
Hannah Rosen: That's so interesting. So, when you're performing one of these assays then, and you're relying on, I would assume an algorithm or something to... to analyze these images for you. Do you ever get conflicts between, like, the human researcher and whatever, you know, you're using to analyze the images? Or is it just kind of you put it in a black box and you just believe whatever the... the program kind of spits out at you?
Beth Cimini: Yeah, that's a great question. Typically, where... where that process comes in is where you know at the end of it we come out with these thousands of features from every cell, and then usually the first thing that... when we when we pass this data off to a biologist is they say OK what... what feature was most important between you know, my untreated and my treated, or my, you know, my wild type and my mutant. And so we tell them and then they go look at the cells, they're like, oh, yes, I see that, but it turns out you're brain is really good at tricking you into things. And so we... what we typically actually recommend people do is pull a bunch of treated and a bunch of untreateds, and then have somebody scramble the pictures for them and then go back and check to see if they're right and if they can actually correctly unscramble things. And it turns out that yeah, again, some... some phenotypes really are human visible, and you can see them, but some of them we've had people be like, I can definitely see that and then you scramble and it's like you can definitely not see that. Not because the person's bad at it, but just because the human brain has no access to this information.
Hannah Rosen: That's amazing. So... but it's not that the difference isn't there, it's just that we can't see it, and the computers can. Wow, that's... that's pretty incredible and I wonder, you know, do some people ever come back and they just like don't believe the computer if the computer is like saying that there's this pattern that they're not, they can't confirm for themselves.
Beth Cimini: They don't usually say we think the computer is lying, but one sort of really important thing that any assay in biology does, you know, often the result of the assay is important, but often what's almost as important is that it suggests the next thing to test, or the next thing to try. You know, if you find out that a certain thing causes the cells to be bigger now, maybe you start thinking about is it cell cycle regulated or something where it can... it controls cell size. If you tell somebody that the texture of something is different, it doesn't really give them a next experiment to do, because there's no sort of human intuition about what a change in texture means. And so in that sense, you know, it can often be kind of unsatisfying. It's not usually like, no, you're lying, it's just sort of like oh, what do I do with this?
Hannah Rosen: That's understandable. So what are some of the biggest challenges when it comes to performing high content imaging and analysis?
Beth Cimini: So, one of the biggest challenges is just that high content imaging and high content image analysis is very sensitive, which is great for detecting things you care about and is bad because it can also detect a lot of things that you don't care about. So, it really loves detecting the difference between the edge wells in a multi well plate and the center wells. We've actually even seen it detect which plate is on the bottom of the stack in an incubator versus at the top of the stack in the incubator. And so doing controls and making sure that your controls are set up properly is a huge part of being able to interpret the data that you get out, and so there's always also just the, you know, you're doing something where you have a lot of conditions and a lot doesn't have to be, you know, thousands, it can be a 96 well plate. Figuring out where you can set up such an assay, are you going to be hand pipetting the 96 well plate or are you going to be able to find access to robotics that are going to do some of this work for you, but then also making sure that once you've done all that work that you can interpret it because you've set your controls up correct?
Hannah Rosen: So it sounds like automation is a very helpful and useful tool when setting up these sorts of assays.
Beth Cimini: It definitely can be. I can say we've had collaborators who are really good at just hand pipetting 96 well plates and these poor graduate students have done it and they've done it beautifully. We've gotten great data from it, but if you have access to automation, it will definitely make your life a lot easier.
Hannah Rosen: All right so it helps, but it's not impossible to do without. That's nice, that's good to know. So, what sort of, you know, does high content imaging require any, like, what sort of specialized equipment... equipment does high content imaging require? Like such a specialized microscopes or imaging software?
Beth Cimini: Yeah, so in addition to just sort of again, liquid handling is nice to have, but not essential. You need a microscope that can sort of track over a plate and take automated images in each place, which those are becoming more accessible and cheaper and sort of more common to see. But you... you do need one of those because it... taking even in 96 and then, especially when you get to 384 or 1536 wells, you don't want to go through and be taking one picture from each well by yourself. So, something where you can do scanning, but often that's not too hard to find. The analysis, we typically use an open source image analysis tool that we write called cell profiler, which is, after ImageJ, probably the second or third most popular sort of open-source image analysis software, but it does have some... take some time to sort of, learn to use, and depending on how much you're scaling your experiment, if you're running one 96 well plate you can definitely run that on your local laptop, but if you're saying “oh, I'm going to run one hundred 384 well plates, you can't run that on your laptop and so then you have to think about where am I going to actually do this computational stuff? Do I have access to a cluster at my university? Can I sign up for the cloud in order to run it in the Cloud and then how do I do that? So all of these are... are surmountable challenges, but they're all things you have to think about when you're getting started in terms of how much work and how much time, is this actually going to take?
Hannah Rosen: So is this the sort of thing that somebody might, you know, like outsource to a core facility if they didn't want to invest in all of this technology themselves?
Beth Cimini: Yeah, there are a couple of different places that will do high content imaging assays as a service. My group is totally computational, but we do... we do the image analysis for such screens as a service so people can... can and do just sort of send us their pictures and we send them back, you know, here are some graphs that say exactly what was going on in your plate. It's relatively unusual that certain have a sort of stand-alone image analysis center, but a lot of the places that will do the screening will also do the analysis for you.
Hannah Rosen: Are there a lot of resources out there for people to learn how to do this sort of, you know, either capture or analysis for high content imaging on their own?
Beth Cimini: Yeah, there... there's some really good guidelines out there. The assay guidance manual from NCATS every year has done some good stuff, and there have been some good things in SLAS journals as well. Looking at this, there's actually a whole special interest group from SLAS about high content screening. It's always one of my favorite parts of the SLAS annual meeting is going to the high content screening SIG. One thing that's very important to our group is sort of making all of our methods and all of our tools open source and accessible, and making this assay easy to get into. So, the cell painting assay which I mentioned is the one that we tend to run the most often. There's Nature protocols, paper of the sort of version two of the protocol from 2016, and we're finishing up, but is on Bio Archive now, version three that we made for this large high content imaging consortium that we just finished up. And so, we put a step by step protocol of everything from sort of when you're thinking about setting up the analysis, what are the things you need to test all the way through? Here's how you do the data analysis. So, it's a long protocol, but it is all out there.
Hannah Rosen: So when you do this sort of, you know, high content imaging, presumably it sounds like you're getting a lot of data out of it. So, you know, when you when you're doing this sort of research, is it best to have an idea going in? Cause it... it sounds to me like the point of it is kind of, don't have a question going in, it's just look at everything and then kind of see what happens. But so, what's the best way to, I guess, form an assay for this? You know, do you go in and just say let's collect all this data, store it somewhere and then come back to it, you know, when we have a... a question that we thought of. Or do you kind of go in with kind of a question in mind? You know, like what's the best way to approach this?
Beth Cimini: Yeah, so we've helped people develop a few different variants of these assays over the years, and typically what we recommend is if there's a thing that, you know, that you care about, you know, put in something for that, put in something that's relatively specific to what you care about, but once you're once you've finished that, just keep filling it up until you have all of the dyes that your microscope can reasonably handle. And so, one researcher we've worked with a lot at the Broad, her name is Melina Claussnitzer, and her lab focuses on adipocytes and so they wanted to make an adipocyte specific version of a high content imaging assay. So they took our cell painting assay and they dropped one of the stains out and put their favorite stain in, but they left all of the rest of them intact. And this is a paper that should be out in the next couple of weeks, so hopefully by the time this podcast drops, but did a paper showing that they could see lipid droplet specific phenotypes in patient cells from people who had predispositions genetically to certain conditions related to fat cells, but that also they saw some things that were related to the mitochondria, which you wouldn't necessarily think that that's really the phenotype you're going to be looking for when you're doing a a sort of that cell screening assay, but it turned out that that's where a lot of the... the different features that they found were useful were.
Hannah Rosen: So how do people typically store all of this data? You know, once you... once you collect all this data, it seems a shame, you know, you kind of look through it and then do people say “alright, well I can't... I have no way of storing this data, I guess I'll just get rid of it.” Or are there, you know, kind of high content imaging data storage solutions out there?
Beth Cimini: Yeah, the... the idea of throwing the data away, it just sort of like fills me with pain. But yeah, so depending on, you know, what size plate you're doing and how many plates you're doing. We find that typically a plate of data is between 40 to a couple of hundred gigabytes, and so probably if you're doing, you know, five of these that's fine, but if you're doing hundreds or thousands you're going to need to either talk to your university about getting some space on your network server. You're going to need to sort of invest in some hard drives and maintain it yourself, or move things to the cloud. We have mostly moved stuff to the cloud just because for us the never having to maintain the infrastructure, that someone else maintains the infrastructure, is really helpful. Most cloud storage companies also nowadays will offer storage tiering where essentially the longer the data sits untouched, it will move into tiers that are harder to access it, so you might then, you know, if you want it back again, need to wait three days, but also gets cheaper, so it gets about 20-fold cheaper between when you first upload it and then when it stays forever. And so... so far we've been able to keep all of our data with moving things to sort of low cost, infrequent access cloud storage tiers, but it's absolutely a consideration, how much data can you afford to store.
Hannah Rosen: I'm curious how often do you go back and, you know, if you're working on something, do you... how often do you go back and say “oh yeah, we did the, you... you know... you know, imaging two years ago that would be perfect for this question that we're looking at now” and just kind of pull that data. Does that happen often?
Beth Cimini: It doesn't happen that often. It does happen, and so I alluded to earlier. We were part of a group that put out a giant cell painting, which again is one of our high content imaging assays, public data set that actually just came out this week that had 120,000 compounds in it and 20,000 different genetic perturbations, and so we took some of our older data sets that we had processed 10 years ago and pulled them out and reprocessed them the same way that that public data set had been processed, but the idea being that hopefully, you know, they we will learn how to bioinformatically match up data across, you know, from data we took ten years ago to data we took now, if the features are the same but also just sort of add on to that collection of our data set, more compounds and more things that people can use that would be publicly available.
Hannah Rosen: So, what types of research is high content imaging ideal for?
Beth Cimini: It's really great for cases where you are trying to make associations between things, and so one of the things that we often see it used for is just, you know, I have a bunch of chemicals or genes or things where I know what they are, and I have a bunch of unknowns and so you can then, by having these sort of signposts of, I know that this gene... or this compound you know is a DNA damager, but I have a whole bunch of compounds I'm not really sure what they do, you can then sort of try to match them. And actually, what some research, especially from... from our sister group, the Carpenter-Singh Group, has found is that sometimes we think we know what a compound does, but using cell painting and sort of being able to match it to this huge other database of phenotypes, we actually can find that maybe actually something has more and more targets than we thought of. And actually, it should be annotated as hitting multiple pathways. And so just being able to sort of cluster things and make these sorts of associations is really what high content image analysis is all about. If you already know what you want to find, you don't really need to do high content imaging, but if you just sort of wanna search... search a problem space, that's where high content analysis is really good.
Hannah Rosen: I wonder so, you know, there's a lot, especially you know with drug discovery, there's a lot of kind of in silico work trying to answer some of these questions about, you know, if I have a new compound, what targets could it potentially hit? And kind of just doing that all artificially before you actually run it on a cell. How does this sort of use of high content imaging... is it competing with this method of you know doing the in silico work? Are they complementary? How does that factor into each other?
Beth Cimini: Yeah, so it can be the basis for a lot of in silico work, and that's in fact why we just made a big public data set and released it, is because we want people to be able to say “all right, I care about this gene. I want to see what it looks like in this public data set and then also what compounds look very much like it or look very much opposite to it in this data set.” And so, it's a great tool for if you keep the data and if you keep the data organized and annotated, and that is a huge part of the battle is just sort of knowing what you have and making sure that from lots of experiments from lots of people you actually know what you have. It can be really powerful tool for doing that sort of in silico screening. Other folks in the field have also done things showing that you can then use it to actually predict the results of other assays. So you can, if you have an assay that's sort of really hard and painful to scale, you can try to learn an association between that assay. Say I did 10 compounds of this chemical screen, it's really hard to scale. And see what those 10 compounds look like in cell painting and if the... if you can learn an association between oh, and all of the ones in the chemical assay where it worked, this feature goes up. Now I can go into that database and say alright, where all of the drugs where that feature went up. And so, you can figure out which compounds you should screen in a more enriched way. It's not going to be perfect, but it's going to hopefully give you a higher hit rate than you would have had otherwise.
Hannah Rosen: So, you kind of discussed this a little bit, but I'm wondering if you could give us kind of a step-by-step guide almost of the process for developing an assay for high content... a high content imaging approach.
Beth Cimini: Yeah, so the first thing is, you know, figure out what... figure out what you care about and sort of, what is the problem space that you're searching? The reason to do that is just because you definitely want to make sure that if you can, you have some positive controls in there in terms of a phenotype that you're trying to induce, or you know if you're looking at things like patient cells, you know, can't... do I have some people that I expect to have a... a mutation that will cause an effect or not, just because this is a sort of very associative technique. So, if all you need to figure out that... if the samples that you're going to screen, if they all cluster with each other and all you learn is the clusters, is that enough? Or do you need positive controls that then sort of give you the idea of what the different clusters are. And then it's a matter again of sort of picking out your dyes, which we recommend sort of... just sort of picking as many different organelles as you can fit alongside, you know, any phenotype specific things, you know, if you have a fluorescent protein marker for a thing that you care about, absolutely throw that in. But fill up the spectral space as much as you can. And then in terms of doing the analysis, typically the steps are just sort of find the cells and then measure them. Picking what measurements is something where we've actually found that in general, if you just pick lots, the exact identities aren't that critical from the point of view of distinguishing one sample from another. But again, if there's a particular thing you want to know, if you want to know how things cluster together, and also you know what their mitochondria look like, making sure that you added enough features that sort of describe the thing that you care about. But one thing that we found, and we have... this is the protocol paper that I referenced, here is we... when we were creating this consortium, we... we wanted to make the most perfect version of the assay and so we tested a ton of different things. We changed the concentrations of the dyes, we changed the microscopy settings, and what we found is that basically everything worked. It's like the least exciting paper ever because the conclusions from basically all of the experiments are, yeah, we tried all of these things and they basically all work, do whatever you want. So, the good thing is, this approach is pretty robust, so you don't have to be scared about, oh, I'm gonna do one thing wrong and the whole thing won't work. It will definitely work. It's just a question of, you know, the total success that you're going to have, but it will probably be pretty good no matter what.
Hannah Rosen: So you... you mentioned earlier this... cell painting as the technique that you guys use very often times for high content imaging. Can you go into a little bit more detail about cell painting and, you know, like especially what types of probes do you typically use when you're doing cell painting? Is there one type that's more ideal than another?
Beth Cimini: Yeah, so something in general refers to a specific set of 6 dyes. You have a MitoTracker, wheat germ agglutinin phalloidin, concanavalin A, SYTO 14, and hoechts. And so these stain the nucleus, the RNA, the ER, the Golgi, the actin filaments, and the plasma membrane. And I think I'm probably forgetting one, but in general they're trying to stay in organelles. And so what... the way this dye panel was designed is, first of all, doing small molecule dyes rather than antibodies, and that's just because anytime with a high... with a sort of high throughput assay, you're adding lots of wash steps, your life becomes more painful. And when you're doing lots and lots of wells, you're... if your antibodies are expensive, your life becomes more painful. And so, using small molecule dyes that are cheap and easily available means that the assay can scale a lot more easily than something that's sort of hard to do or expensive. But like I said, those specific dyes can be swapped out if there's something that you really want to want to look at and you're out of spectral space. But it was just essentially trying to fill the spectral space with organelle markers that are small molecules rather than antibodies.
Hannah Rosen: Are there other ways to perform high content imaging without doing cell painting?
Beth Cimini: Yeah, the magic of the approach is definitely not in the specific dyes. You can absolutely do high content screening with any images that you want to take, or even that you already have. There's a paper from the Carpenter Lab a few years ago where they were collaborating with the pharma company and the pharma company literally just pulled images they had already taken and... and used it in the way I mentioned before to try and train a classifier between you know, completely different assays they had run. And these old imaging assays that were literally just sitting on their server, like the microchip pictures, were just sitting on their server already, and so any images that you have, as long as you sort of measure them richly and deeply so that you have lots of measurements and start doing clustering will work, so painting works pretty well and it's at least a sort of benchmark, because then if you want to compare your data to public data, you know that you're using the same dyes, but it... it can really be done with any kind of microscope images you have.
Hannah Rosen: How common is it... you mentioned public data. Is it common for people to make their results from high content screening public?
Beth Cimini: It's still not incredibly common, but it's becoming more so and some of that, I think is... is sort of funding mandates are pushing people to do that, but I think in general people are just sort of realizing the value of open science and the value of, you know, you're making an assay with hundreds or thousands of... of different compounds or genes or whatever in it. There's probably more than you can analyze, and in quite a long time in many sort of grad students or postdocs, so put it out there for somebody else to be able to learn things from it. And so the IDR, the imaging data resource, is one place that for several years now has been collecting these public data sets with annotations, with the idea that then you can go in and sort of reanalyze it. We've recently, because we've started making some data sets that are too big even for the IDR, launched something that we called the cell painting gallery, which right now has only cell painting in it, but is not meant to be only a home for cell painting but where we have big high content screens and things. So we are part of the registry of open data on AWS. So, AWS helped sponsor the resource.
Hannah Rosen: That's great.
Beth Cimini: So there's a group, and I forget which group, called the... the Research Parasite Award, which is sort of a dubious name, but the idea is every year to focus on research that was done using only other people's data and only sort of recycled reused data, and I think that's fantastic because we spend hundreds or thousands or tens of thousands of dollars making these data sets and if it then just sort of sits on some hard drives and nobody ever looks at it again, you know, we're not spending our scientific dollars well. If somebody else has already done the experiment, and they've done it in a way where you can trust it, go use their data. And so, I hope maybe someday I can do something cool enough where I can win one of those.
Hannah Rosen: I think that's a great goal. I love it, yeah. I know myself I have all of the raw data from when I did my PhD on this, just like, giant hard drive that's just... I can't bring myself to get rid of, even though I'm never, ever gonna.... I know I'm never gonna do anything with it, but it just seems such a shame, yeah.
Beth Cimini: No, I've got three of those sitting right next to me right now so... sympathize.
Hannah Rosen: So, you know, is there a way to make high content imaging a high throughput process?
Beth Cimini: Yeah, so high content and throughput... high throughput go really well together. Again, assuming you've got access to the right equipment. So, I mentioned this giant public data set that... that we did. It's, I think somewhere about 3000 plates of 384 or 1536 well plates. If you've got access to the equipment to sort of run things in high throughput, the more samples are in your experiment, the more powerful high content imaging gets because the more things you can cluster against one another and the more things you can compare. High content and high throughput love being put together, but again you have to have access to the right things. Otherwise again, that the grad students will revolt when you ask them to make you know two hundred 384 well plates by hand.
Hannah Rosen: For sure, so if there's a researcher out there listening to this podcast right now and they are really interested in starting to use high content imaging for their research, what would they need to know to get started?
Beth Cimini: I think in general just that it doesn't really take anything special other than the images you already have to do this. The, you know, using specialized assets or specialized techniques might increase the power of what you're doing, but you can do this tomorrow. You can take any images you've already or you've already generated and sort of, instead of analyzing the... the five metrics that you did before, use a program like cell profiler, but also like a lot of other tools too, to output instead hundreds or thousands of measurements and then just cluster stuff and see what happens. If you've only got four samples, you know probably that clustering is not going to be interesting. You could then compare your four samples, if the treatments or whatever you did are in our public data set, you could compare it to our public data set and you could see do your results match our results, or could you train something to match your results to our results in a way that would give you access to more data? It's really just about learning all of the things that are possible. So, there's a group called the CytoData Society that I know is one group that's really pushing approaches in this, but also organizes a big conference every year that you can go to and sort of learn about what are people doing in this high content imaging space and learn about all the different ways to do it. The other thing I would just say is, it's in general a really friendly and positive field, and so there's a lot of, you know, public tutorials and things out there, but also a lot of people like me who are huge dorks and love talking about this stuff and are really excited to talk to people who are getting into this space because we think... we think it's powerful and valuable and we... we want people to get more out of the data they already have. And so, reach out to... to people in the field. There is the image analysis forum image.sc which is where a lot of the open-source image analysis softwarers hangout, definitely also has a cell painting tag and is a place you could go to learn about different open-source tools that allow you to do high content image analysis.
Hannah Rosen: Well, unfortunately we're almost to the end of our time. But before we say goodbye, I wanted to check in with you and see, is there anything that we didn't get a chance to talk about today regarding high content screening that you really, really want to talk about?
Beth Cimini: No other than just try it. You know you've already collected data somewhere. I know you have, so just try it. The worst thing is that, sort of, you've learned and a new way to approach your data and nothing new... no new results come from it, but you'll know how to do it for next time and you might end up discovering connections in your data that you never previously knew were there. One of the nice things with image analysis approaches is it's free. You can't break anything, you can't destroy it, just you know. Try it and see what happens and hopefully you'll find something awesome.
Hannah Rosen: Great. Well Beth, thank You so much for joining us today. I learned a ton talking to you, which is always really exciting for me. But so, thank you for joining us and we look forward to seeing you at a future SLAS event where all the people who are listening can rush up to you and ask you all of their high content analysis questions.
Beth Cimini: Bring it on, I'm looking forward to it. Thank you so much for having me.