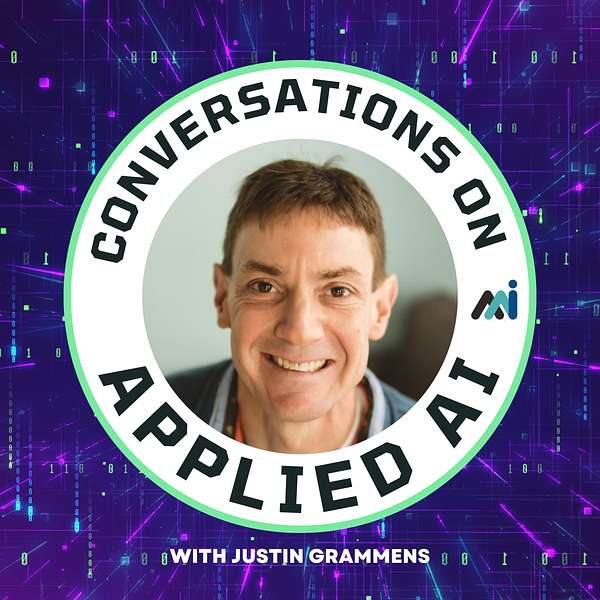
Conversations on Applied AI
Welcome to the Conversations on Applied AI Podcast where Justin Grammens and the team at Emerging Technologies North talk with experts in the fields of Artificial Intelligence and Deep Learning. In each episode, we cut through the hype and dive into how these technologies are being applied to real-world problems today. We hope that you find this episode educational and applicable to your industry and connect with us to learn more about our organization at AppliedAI.MN. Enjoy!
Conversations on Applied AI
Dan McCreary - AI & Enterprise Knowledge Graphs
In this episode, Dan McCreary, Distinguished Engineer in AI and Graph at Optum shares with us his deep experience in Graph Databases, the AI racing league, and why Enterprise Knowledge Graphs will rule the world in the areas of Healthcare and Artificial Intelligence.
Thank you, Dan, for the conversation and to you the listener for your continued support and interest in Artificial Intelligence and how it is being applied to the world in which we live.
Interested in learning more? Please join us at an AppliedAI monthly event!
But there were a lot of unexpected things that we didn't tend to dissipate. And a lot of that has to do with the fact that these graph databases are amazing because there's virtually no penalty for doing joins.
AI Announcer :Welcome to the conversations on applied AI podcast where Justin Grammens and the team at emerging technologies North talk with experts in the field of artificial intelligence and deep learning. In each episode, we cut through the hype and dive into how these technologies are being applied to real world problems today. We hope that you find this episode educational and applicable to your industry and connect with us to learn more about our organization at applied ai.mn. Enjoy.
Justin Grammens :Alright, welcome, everyone. I want to thank Dan McCreary here today our guest our first applied AI podcast, and Dan and I have known each other for many, many years, both sort of been working in the in the local Twin Cities tech community and coming at it from different angles, obviously, but all, you know, sort of under the same sort of common vibe. And I appreciate Dan taking his time here and sort of sharing his experiences with regards to artificial intelligence. Dan, if you wanted to sort of start maybe talk about your background, sort of what you're doing these days, and maybe sort of how you got to this point,
Dan McCreary :happy to so right now I'm working within optim Optimus division of United Health Group. We're doing a lot of the it division. UnitedHealth Group is a very large company, almost 335,000 employees and 32,000 it employees and I work in a very small area, called the advanced technology collaborative, and you achieve a very distributed group. It's not like Amazon or Apple that has very centralized control. It's very distributed. We buy about 40 companies a year and we buy them often just for their data. And the job of the advanced technology collaborative is to scan the horizon for technologies that can be relevant two to five years out and help our business units decide which ones are relevant. The bulk of a lot of our work is directly related to AI. And the rest is almost indirectly related, in some ways getting more data to power engines. And what we're really focusing on now is getting more data, more connected data, and then allow our data scientists, we have 3200 people with the title of data scientist, faster access to high quality connected data, to build machine learning models all over the organization for every possible thing related to healthcare, in the clinics, in claims in providers in networks or contracts. We're trying to be driven by predictive analytics and machine learning.
Justin Grammens :Awesome, very cool. So how would you define AI? I mean, yeah, it's a pretty pretty broad, broad scoping term, in the context of what you guys are doing and maybe just your own personal context, you know, if you're to sort of explain it to a layman or late woman,
Dan McCreary :yeah. lay person. Yes.
Justin Grammens :Lay first and there you go.
Dan McCreary :Yeah, it's is a very broad thing. And I think what's important about the definition of AI is it changes every month. It's something that's always changing based on what people used to think was difficult is now commonplace. And things that are just beyond what we can comprehend are considered AI. So my best example is Alexa, you know, 10 years ago, we couldn't just talk to our home audio system and and do various discussions and get it get the answers back and have it almost be an interactive system. I was just checking to make sure my Alexa was turned off. Talk about always listening is exactly. But it's one of these things where every year what you used to think was AI five years ago, is now just part of every everyday and appliance, it's part of our environment. And there's a lot of things happening behind the scenes. What's important for me about the definition is that a lot of people that are new to the field field are only associating AI with machine learning. And I think that's a big mistake in machine learning, and specifically, deep neural networks have really dominated the industry and a lot of the new developments. But it's not everything, I'd say 80% of innovation is really coming from there. But they're still about 20% of areas that are coming from symbolic reasoning and inference and connecting data sources together and then being able to traverse that and a lot of the predictive algorithms that we see in knowledge graphs, I think those are very complimentary to things that are in AI. So it's a changing field called time. Nice. Yeah,
Justin Grammens :yeah, very good. I think we'll talk a little bit about the knowledge graphs and stuff like that pretty soon here. But one of the things that you and I were sort of kind of going back and forth before we had this podcast was, you know, you talked about optim kind of transitioning from a batch mindset to maybe real time point of care, clinical decision support. Is there is there I guess in one case, maybe you could sort of elaborate a little bit more on this real time aspect. And do you think Think AI needs to be real time to really be AI. That's a little bit of a side question. But yeah, I'd be curious what you think
Dan McCreary :that's really important. So right now in healthcare in the United States, we're dominated by this thing called fee for services. And fee for services is every time you go and have a procedure done, or maybe an eye exam, a health checkout procedure, every time you go to a hospital, they code everything that happens, and they get reimbursed for the number of procedures they do. And the more procedures to do, the more they get reimbursed. So there's no incentive systems in place to limit the number of procedures that are maybe borderline maybe they're not really necessarily, if they're clearly not necessary, that's fraud, but there's a huge amount of edge cases. My wife had a spot of indigestion awhile ago, and she went in and they did $13,000 of highly invasive tests, just to make sure it wasn't heart related problem. And if they really would have looked at the data and her Fitbit data and all those she had a very, very healthy heart, there was no real reason to do $13,000 to test and that's a good example of how the old way is doing not what we're trying to do is move to this thing vertically integrated, where optim doesn't just do the claims for companies, we actually own the clinics, we pay the doctors and the nurses as our staff, they're full time employees, just like Mayo Clinic has full time doctors, and that we also provide the insurance, which means that we are highly incentive to give high quality care, and yet also minimize unnecessary procedures. And that studies in general, those are called a CEOs, accountable care organizations that general studies that a CEOs are about 30% less than fee for services. And so what we're trying to do is transition it and that means we have to buy clinics. But what's important about that is when a physician or physician assistant or nurse makes a judgment About what tests are necessary, what drugs are required, they'd like to be able to use data. And the point is you can't run a batch job overnight and say, according to your symptoms, and your clinical history and your entire genome and all your background, we're going to come up with the right drug for you tomorrow, right? That just doesn't lie, right? It's when the people are using that electronic medical record system. And they say, well, we're going to give you a prescription for an antibiotic. And what they might do is they might have a deal with the local drug company, and they'll give you a very expensive drug. Or what if we could tap into the entire equally effective settings and will give you the least expensive drug. The point is, we have to do that. We have about 100 milliseconds to make sure there's no lag in that drop down list. 100 milliseconds. That's what we call real time. Real Time clinical decision support. Now we're not trying to take over the job of Commissioners. What we're doing is give them a ranked list of options. And the pricing associate with that, to help them make better decisions, and then also explain it right. So we can't just make a recommendation, our AI has to explain why. And they're the reason why is that we use real world evidence. Real World evidence is a term that we're using over and over to say, gee, we have 200 million people in our database. And we've extracted the 20,000 that are most similar to you. And we've looked at their profiles of which drugs are most effective. And here are the ones that we are recommending. And then we let them choose and make those decisions. But there's a lot of decision and data behind all of that information. So it's going to be where we can't just think about it overnight and do analysis, we need to do it in about 100 milliseconds. And that means that our infrastructure has to be much more robust, much richer and be able to do comparison, and we call similarity calculations in real time. Gotcha.
Justin Grammens :Well, yeah, that's that's fascinating. And so how long have you been working Working in healthcare, because there's a lot of terminologies I'm sure you've picked up along the way and acronyms and all that type of stuff. So yeah, if I, if I dive into acronyms without
Dan McCreary :stopping, and I'll try to define them. But I've been on and off in healthcare about 15 years, and then last seven years, really focused in on large, scalable data strategies for optim. And then in the last two years, really shifting towards the clinical. And I just tell you, you know, clinical is an incredibly deep field, so many complicated terminologies and medical systems and things and it just takes a long time to come up to speed. But it's something that I'm really lucky that I can work with a lot of people within optim that are no have to have 20 years experience building clinical decision support systems, and the role of AI and natural language processing is just going to be incredible, as far as helping our clinics and our physicians make really good data driven recommendations in the future.
Justin Grammens :Yeah, yeah, for sure. So when you're when you need to make this decision in 100 milliseconds, obviously, there's a ton of data that you are going to be trying to pull in and analyze. And maybe this is where the Knowledge Graph and all that stuff sort of comes into play. You want explain a little bit how that works?
Dan McCreary :Yeah, absolutely. So let's talk about the old way that we used to pull clinical data in ancient times, stone tablets, right and carved our records in rows and columns in the Sumerian tablets. And those rows were a shoe COEs, a lock 50 bushels of grain right. And we always stored those data in rows and columns. And for the last 5000 years, we have been doing rows and column oriented data. That means going back to punch cards and punch cards going into flat file systems and flat files being won by COBOL, and copy books and things like that. And then somebody said, hey, what if we actually created these IDs in these columns and match them together whenever we run a query and come up with a new idea, and that had this idea of a join, where every time we do a query, we complete Pair all of the data sets and all of those tables, and tried to figure it out in every time we did the query. But that worked as long as you had a few 10s or hundreds of thousands of records, once you start to get the millions of records, joining data gets really expensive. And what's odd about clinical systems is that there's so many relationships, relationship between you and your conditions, your allergies, your drugs, your symptoms, your prescriptions, your providers, just the list of relationship goes on and on and on. And what happened is people found that they just couldn't bring up that data quickly enough by doing joins. So around 2010, the people that kneel for Jay built the first was called index free adjacency graph system. And they start out very small and modestly in a Java JAR file, you just load it in your thing, and it would use memory pointers to hop through relationships. The fastest operations that computers can do are pointer hops. And those systems were wonderful. But they had this unfortunate problem that once you had more pointers that would fit in your RAM, the systems would kind of fall over and die in their query performance. Sure, sure. So it was all loaded in memory. Right, those pointers had to be loaded in memory. If they weren't in memory, then you'd swap out to disk and your query performance just dropped to the floor. So they worked really well in a lot of pilots and just failed when we tried to get the hundreds of millions of patients in there. But about two years ago, this company called Tiger graph really started to develop a distributed graphs. And they were the first company that really completely cleaned the slate, started from scratch, and built a distributed graph systems. And they're not the only ones in the market, but they're the ones that have the most robust security models. And in healthcare, we need very, very robust access control lists. So we've been really going towards these distributed graph systems. And what's interesting is everything we've learned in the Hadoop world. If you ever did Hadoop stuff, they had this thing called map and reduce. map is where you go and grab all the data that's necessary on all the distributed nodes. You do the calculations on all those nodes, and then you reduce the results and collect them all together. And now what Tiger graph and and other companies are doing is they're building that MapReduce into distributed graph. So if I say show me, you have a 200 million patients, show me the 10,000 that have this condition, each of them will go through their distributed graphs, grab the subset, and then bring it back really quickly. And that's how we're able to be able to run these reports and such incredible. The name for that is called h tap, hybrid transaction and analytical processing, because we're getting the speed of the traditional analytics, but we're doing it with in memory pointers in a distributed and scalable way. as we add more patients, as we buy more companies, we just add nodes to clusters. And we don't have to redesign our systems from scratch. So that's really been a big transformation for us at scale is going with distributed Knowledge Graph.
Justin Grammens :Neat, very cool. Yeah. I think some of Google's early stuff was MapReduce. I mean, in fact, I think they're one of the pioneers in that space. Is
Dan McCreary :that? Absolutely, yeah. And just to give Google all the credit that they deserve, they also were the first to really do knowledge graphs at scale. They in 2012, they wrote a paper called things, not strings, where they were allowing you to search the semantic meaning because you might type in a keyword, that keyword is looked up in a graph, it looks all of its aliases, and synonyms and all this other stuff, and it finds all the documents related to the thing you're looking for not just a keyword match. And they showed that those graphs scale back in 2012. So this year, we are eight years later, and most companies have not yet integrated those technologies into their production systems. But Google really proved that these things not only are flexible, great at storing knowledge, but also scale. And I think that's really one of the things that we have to give credit to Google also say that every social networking company in the world LinkedIn, and Facebook book also are all driven by graph databases. And then Amazon now also having a very strong component graph their product graph every time you do a search on Amazon, they're looking for similar members that have similar purchases in the past, and they're recommending things. So the similarity algorithms are core to everything anytime you sell products online. Now, if you don't do real time recommendation in that hundred millisecond window, right, that you're not going to be as competitive as other companies in your sales. You need to have all that information about every one of your customers tied together just like our clinical systems so that you can make product recommendations.
Justin Grammens :Yeah, well in the the sea of data is only going to be getting larger and larger. Right. So if, you know I mean, you might be able to scale today on some other system, but just look another one, two years down the road. I mean, data is coming in exponentially faster. So that's something that's going to, it's going to have to withstand the deluge of data coming.
Dan McCreary :Well, you and I have been doing IoT work now for what five years together. We will Five years ago, Justin that we did our first IoT hack day, something like that.
Justin Grammens :Yeah, I think it was a boy fall of 2015. Yeah, yeah, Yep. Yep, exactly. So yeah.
Dan McCreary :And what that shows you is that the sensors are getting lower cost every year microphones are getting higher quality, the Deep Field listening algorithms. So you can have a little microphone in one part of your house and yet it can understand the sounds across your whole house. That's just amazing technologies that are continuing to get better every year. And the ability for us to do sensors in the home, especially now this isn't the COVID times right where the middle of the COVID pandemic. Wouldn't it be nice if we could stay connected to our seniors, carers providers in their home without having to go into the centralized nursing homes where all the tragic contagion goes back and forth. Those are all things that we it's really we're trying to do to make it easier for people to stay in their home. IoT is going to give us a lot more opportunity. You know, every time they turn on switch, we can monitor that every time They get out of bed, we can do bed sensor monitoring every time that they open their pillbox, we can grab that information. So yes, there's going to be a lot more information about medical adherence in the home. And those are big things that are going to help us in the quality of care for our communities. Yeah. Excellent. Excellent. So I think I've read a couple of your blog posts. And you I think you sort of call the enterprise Knowledge Graph. EKGs. Yes. Right, which I think is great. I love the terminology, I think you mentioned it's also sort of sensing sort of the lifeline or the heartbeat, I guess, right in some way of your heart ation of your organization. And, you know, when we, when we went down this journey, there were a few things we're hoping for, we were hoping for better query performance, we're hoping for easier to tie the state together. But there were a lot of unexpected things that we didn't tend to dissipate. And a lot of that has to do with the fact that these graph databases are amazing because there's virtually no penalty for doing joins, right and when we first started doing this. Remember, I'm an old relational guy, and we try to lose a lot of things. Well, let's just pretend every vertex has a table, and we'll put all the columns together there and blah, blah. And we made a lot of mistakes. What we found was that if we did model the world, in reality, we called nor highly normalized, where every real thing is its own vertex, then we couldn't reuse our models, they would break, they didn't really work correctly. But if we did our job correctly, and we said everything in the real world should be a vertex, it turns out that everybody can share the same model. There are many ways to optimize performance. But there's only one way to model the truth of the world. And once you find that truth, then everybody can use it if there's no penalty for joining. And so we've been just absolutely stunned about how many business units once we model the world, right, can share the same model. They don't need to grab their own set of data and build their own mini data mart and hire them. own analytics and have their own model. And their costs just go up and up and up, because then they have to load the data and maintain the data and check the data quality. If we do that once for everybody costs go down dramatically. So these scalable enterprise knowledge graphs have really started to change the entire cost models. And you know, cost models are really why AI is used in practice, because it's lower cost than doing these rules by hand. It's lower cost than the old manual way. And what we're finding is that the chargeback rates that it has for our business units, sometimes one 10th of the old Hadoop days, where you have to have all the different representations based on your different query performance tools, the star schemas and the OLAP cubes, and blah, blah, blah. Now all of those reports can happen relatively quickly in that 10th of a second interval, with some exceptions, but it's been revolutionary. And what it's going to happen is it's going to start to slowly have Darwin's law apply to relational things. Costs are just too high. And so a lot of organizations that are still trying to there are many resists, you're still building relational systems, and they are going to be very high cost as these scalable graphs come out. It's going to really change the dynamic. And it's going to do it by lower cost and operational systems.
Justin Grammens :What are some examples where you would not want to go graph, I guess? Do you have any of those offhand? Are you just kind of like graph all the time?
Dan McCreary :No, no, no. So if you look at my history, I did a lot of work with this thing called no SQL, right? But we wrote this book called Making Sense of no SQL, my wife and I, and it came up with six architectural patterns. relational is the most common 95% of transactions, at least in Minnesota are still being done on relational and then analytical is OLAP cubes, where we have the pre built aggregates, the star schemas, new track tables, and then the for NO SEQUEL, which are key value store, column, families store, graph and document and what we find is that graph is really good for a lot of things, and it's really Really bad for certain things like a blob store. Now, if you have images, you're never going to want to store your images in a graph. Now you store the URL to those images, so that you can integrate those things and do machine learning on them and pie the list of objects you extract from images. But in graph, Ram is precious, right? It's very precious. Because if you have a big image sitting there in memory, it's going to swamp out 10,000 links that you need to be close. So we really, really keep our Ram are trying to load our graph directly. And so any big blobs in the documents are kind of forbidden graph storage. But the thing is, documents or documents are unique in that they have lots of words in them. And those words have meaning. And they have structure you have words inside of titles. Those words are more important than words and other parts. And when you do a search, you look at relevancy. how relevant is this key word to the whole thing, the structure of where the words in the bathroom are so important. So we don't actually use graphs for doing document ranking. We only use it for things that really are about fast queries and interactive things and analytics. And we also use it to power machine learning, real quick extract, get the data into our models, do the training, find out the insights and pour that data back end. So it is really become a central hub for a lot of things. There's a lot of use cases that are forbidden and some of the analytical things, we've pre calculated some samples, once again, it's not going to replace a Cognos or an OLAP cube or things like that. Those are have other use cases that those are accustomed to.
Justin Grammens :Excellent. Yeah, good. Yeah. Always sort of everyone sort of wants to hop on the new hotness, right. Yeah, the new bandwagon. And I feel I feel that way, in some ways with blockchain. You know, I'm by no means a blockchain expert. But I mean, but over the past couple years has been blockchain has been used for everything and it's like, No, no, no, you don't understand. You know, it's actually not meant to store a whole bunch of data in the blocks.
Dan McCreary :You know, That is a really good example. There is an expression in the graph community called graphs are everywhere. So if you look at the real world, there's a lot of things and those things have relationships. So anytime people come to us and say, gee, we think we can model this graph. That's great. Watching is really odd, because it got so much press. But if you actually look at how to qualify business project for blockchain, you say, if you have groups of people that need to work together, and if they want to trust their own internal computer systems, and if they need a distributed ledger, and if they want that audit ability, and if there's about seven different AMS, all new then would you ever pick watching so it's so funny stories about business units that come into the ATC and say, gee, we have this problem, and we thought blockchain would be really useful. Not even close, but graph is off.
Justin Grammens :Sure after
Dan McCreary :watching is a really good example story of a very hot technology that unfortunately had a very, very narrow set of use cases, less than 1% of use cases that come across my desk are blotching related. And it only has to do right now with when we're trying to build consensus about transactions between organizations. Sure, and those organizations also have to agree on the standards on how to represent those things. And that standardization process only often takes three, four or five years sometimes. So the ROI for blockchain projects still may be there. But none of our businesses want to invest in something where they don't know if they can see the results until five years, probably not a great and so I'd say it is still a great technology for certain things, but we have to narrow down our use cases. So blockchain is a bit extreme graph is the other end of the spectrum, where there are just so many projects that could be using graph for knowledge management today, but that connects well within the company. strengths.
Justin Grammens :So is that is that part of your role, I guess, is educating people on graph or, or just or just all sorts of technologies,
Dan McCreary :all sorts of technologies. So I consider myself a solution architect, Solution Architect is role where the business unit comes to a solution architect and says, we have these problems. And we'd like to work with you to figure out what is a good match. So we go through and read gather the requirements, there's always many requirements to come in. But say we have 1000 requirements come in, well, you might distill it down to 20, what are called architecturally significant requirements. For example, if somebody says, well, the system has to be five nines, well, then a single relational database is never going to work because you have to have replication and you have to be able to upgrade the software without ever shutting it down, or you have to have rolling upgrades in the cluster. So we know that if you have super high availability, but all these no SQL technologies, you can be much better match. So we go through and look for those requirements in the match. Right, not just database and the instructions But the entire stack of tools these days. Gotcha,
Justin Grammens :gotcha. I think you mentioned you've been doing a lot of stuff with NLP, I guess is that is that one of your sort of your your current passions?
Dan McCreary :right it is. And I don't know if you've played with any of these tools Justin lately that are all built around the Bert architecture, the transformer based systems. But it is just amazing to see just in the last six months, the amount of development that's happening, I think, if if you ask me about, you know, where's Skynet gonna evolve from today? It's gonna be built around a lot of the tools that people are trying to do to understand language. And many of the tools that we have developed in NLP, na are now spreading across the industry and other areas. One of my best examples is these things called embeddings. word embeddings. Right. So there's some fantastic demonstrations out there now where we take all of Wikipedia, you load it into an AI model so it's unsupervised learning. You don't have to training set with this is this word label this type of stuff. You just load the things in. And they start to, quote, understand the meaning of these concepts. And you can do things like is a baby older than a grandparent? And they will answer it right. They're starting to have an understanding of all these concepts and how they're related to each other. I have you played with the Okta transformer system? Yes, I did. I did. Yeah.
Justin Grammens :Yeah, that was fascinating. And the thing that's neat is, you don't know what you're gonna get. It's different every time right. So I put in something about will the Internet of Things change the world? And then it just rambled on, right? It basically had a full sort of like paragraph with regards to what it did. And I'm like, Okay, well, I mean, it was it was plausible. I was obviously going to go to a bunch of a bunch of jargon, but it was definitely on track. And then I clicked the Generate again, and it generated something that was like equally well, you know, so I found that pretty neat.
Dan McCreary :Yeah, so that's a really good example of a very large corpus. have text fed into this very large system GPT two done by open AI, and it's really starting to understand things. And what you find is that if you type in something in a totally different domain, so you typed in something about rock music, it will write you an essay about rock music. If you type it about kids fairytales, it'll write you a paragraph about kids. So it knows about all these related concepts. And if you put in the name of rock band, it's going to know similar bands that were also in rock. Right? So that's just really freaky to me about how these neural networks that are behind this are getting close to understanding. Now, the sad thing about that is that they're been using a lot of brute force and massive, massive 10s of billions of parameters in their neural network models. I always remember the human brain is about 82 billion neurons, right? 10 billion is getting in the same order of magnitude, but it takes 10s of thousands of dollars to train those models. Which means that all of the other researchers around the world are falling out of the race, right? It's just Google and Facebook and Amazon, and a few other organizations that can afford to blow $100,000 to build a model are now doing this work. But now we have another innovative group of people that are saying, well, you guys are very wasteful using grid First, if you're really clever, we can prune these networks. And we can do all these other things to make it much smaller, and yet still get close to the same results. And what you see is that there's just tons of innovation happening. So it's not just brute force and pruning. And a lot of the papers that are coming out about making your networks much smaller and yet still precise, I think are great areas for research. And every time some of those new papers come out, I'm very interested to see whether we can, you know, actually train a good natural language system for $10,000 not 100,000.
Justin Grammens :Right, right. I mean, I've always thought that brute force at some point falls down right? I mean, I don't think it's the I don't think it's the answer. The answer is sandal Beal.
Dan McCreary :That's right. Yeah, you can have brute force in the size of data, the number of GPUs you have the size of your network, the number of layers and under printers, all sorts of ways you brute force. And sometimes they have to scale up together. And in general, they are getting better results. But the question is, are they building models that you can actually deploy? Right? Because you can't take 100 billion parameter model and deploy it on a cell phone. Right, right. Right. Well, you can do inferences of huge data center. So we do need to have small, light networks. And you know, what it says to me just means that the human brain is still just an absolutely amazing thing. That every night that we go to sleep, and the theory is that we learn a lot during the day. And at night when we sleep. we prune our neural networks so that they're more efficient. Now, once again, that's the theory there. Yeah, we don't really understand it. But what it shows you is that we are just at the beginning to understand the process of learning and training these artificial neural networks. And there's just such a wonderful opportunity. I almost wish I was a grad student today. So I could spend a lot of time just studying these algorithms and make some innovative contributions. Because a lot of the contributions that are coming in aren't just the PhD researchers deep within DeepMind. At Google, they're coming from Canada. A lot of the people that Geoffrey Hinton trained a lot of the places that are really still looking at it innovatively. So I think there's a great opportunity for young engineers to continue to try to understand the dynamics of these things and to be innovative. I'm just I can't wait to see some of the models. A lot of people are saying that these models as they get better and bigger, we are going to see an inflection point where they really start to understand and we can ask them questions in the chat bot, and they are going to give us answers that are reasoning. Turing test, yes, the Turing test, I think, I think we're 10 years away from being able to have these natural language processing models pass the Turing test. But it's going to be a combination of creativity and symbolic reasoning and inference. And these deep neural networks, it's not just going to be flat, deep neural networks, or other technologies are gonna come.
Justin Grammens :Sure, sure. Fascinating. You talked a little bit about sort of being a grad student these days, I guess. And I know one of one of the things that you are very passionate about, and you've worked very hard here on the community here is helping youngsters learn new technology, whether it be IoT and Arduino is programming of all the various sorts and stuff like that. And you know, I know one of your current passions is AI racing. Lee wanted you to Could you elaborate a little bit about
Dan McCreary :Yeah, absolutely. Well, you were involved in that too. I think you were one of the first people to print a 3d chassis for me, Justin so I did know are your favorite? Yeah, absolutely. So one of the interesting things about this AI recently is part of its p back in what's called the donkey car movement. The donkey car was a car that was created by a bunch of researchers out in the Bay Area. And they asked a simple question, what's the cheapest car that we could build using an RC car and a Raspberry Pi that could take images from a camera and drive around the track. And they started that almost four years ago. And it was very primitive. But it's interesting how many people were interested in that same question. So they had a small group of four people get together in the next month, they got together, they had eight and then doubled to 16. And just recently, I heard that they had a group of 250 people come to a single meetup with cars all trying to race around these tracks. So it's really taken off quite a bit. And it's now a worldwide movement. There's, there's 85 leads around the world in almost every country setting up AI racing tournaments. What's interesting about it is that it's kind of For a lot of people, it's scary, right? When you think of AI, you think of the Terminator, the movie, the horror, and the gore and the science fiction movies that prey on our uncertain views of the future, what is unknown. But in reality, AI can be fun. And it can be very beneficial. And within optim, I have product managers I work with. And I have a lot of product managers said, well, I've heard you're doing work in AI. What exactly is that? And is there any way I can learn more. And so we've decided to let all of our product managers go through this racing need, so that they can just have a feeling of what it is to drive a crowd car around, gather some data, run it through a GPU, build a model, take that model, stick it on your car, and hit the auto drive. And once they do go through that lifecycle becomes a lot less intimidating. It becomes Something they understand all the pieces and the workflows. And those are the same things that we're trying to do to our high schools and the teachers in our high schools that are trying to set these things up. And it goes on to not just AI, but it goes to the broader thing of data literacy. If you ask employers today, what are the most important skills data literacy is something that always comes up they need people that can load data into spreadsheets and do analysis and create plots and charts and trends. Every every part of the company, your marketing, division research and development, their sales, sales, forecasting, all of these things are now data driven. And yet we graduate students in the state of Minnesota, where they can graduate from high school and never have to use a single spreadsheet. It's not part of our graduation standards. So the only people that are really doing this are teachers that take it upon themselves to develop this curriculum. And that's a huge amount of work and they often don't have the support the training In the background to do this. So if we care about our future jobs in Minnesota, we have to understand that these teachers aren't going to magically understand data science, and they will literacy and just transmit it. We as engineers in our community need to be part of that role. And we need to volunteer in schools, help the teachers come up to speed help the students do it. JOHN herkie has been doing some amazing stuff, one of the guys that you and I know in the futurist Academy, and we all need to really take a more active role in the Bay Area. it's different because everybody works in this every day. And so their kids are interested in it and the teachers then get influenced here, we have to work a little harder. We have great analytics, we you know, we have a culture of retail here in Minnesota. We have Best Buy we have target a lot of great SAS developers came out of those things do predictive modeling for inventory management and control and since but are we really making this next leap to machine running an AI, I'm worried. And I think we need to take a much more active role in helping our community come up to speed. I think the AI racing really is one vehicle for doing that. And there are other vehicles too, that we can work on. Justin, you've also done a lot of work with setting up things like hackathons. And I want to acknowledge all the work that you've done to setting those up to get people educated, because that's really about community training.
Justin Grammens :Yeah, yeah, it is. It is. It's about getting people's well. There's a number different aspects to it. It's just it's getting dirty. Right? So hey, I have this idea. Let's just dive in. It's about working together on a team, right? So there, it's not just a one person show. We always have teams teams have to be two or more. Sometimes we grew up to be eight or so. And then, you know, there's the whole aspect of having a deadline. Like people I joke around because my basement is littered with tons of projects and said you have a deadline, they just kind of ended and then the last part is just having fun, right? So so so yeah, I just it's just you know, sort of enjoying And sort of building stuff together. And so yeah, we've had a lot of fun focused on doing IoT hackathons. And I think, as we sort of evolve the group and evolve what we're doing, I see us doing a lot more in sort of the AI space as well.
Dan McCreary :Yeah. So the evolution of these devices, from Arduinos, to Raspberry Pi's the new video Nanos, that now that we have 128 CUDA cores, directly on a $99 edge device. And I think right now you can get something that has almost 10 times that power for about twice as much now for about $200. those devices are just going to continue. And I think our hackathons we need to have teams that are pre trained in how to use Nvidia nano and come in with their data, or show them how to build a machine learning model. And the best thing is cameras, right cameras are, there's so many image recognition where you can have people walk by a camera and count the number of faces that come out. There's those are just out of the box computer vision algorithms. They are so we want to get more of those into our eyes.
Justin Grammens :Yeah, yeah. And it's it's almost magical. When people see it the first time, they're like, oh, wow, that it can really pick that out, you know? And it's like, wow, yeah, yes, actually, that we just saw, I guess collectively have gotten really good at, at getting a lot of images of cats and dogs, you know, and have an N of n. And if human faces, I mean, it's amazing Google Photos, you know, I'll take a picture of one of my kids and it'll realize that it's him. You know, of course, the kids faces change over time, but it's amazing how accurate it is.
Dan McCreary :Yeah. You know, what's amazing about Google Google Photos is every month they add new algorithms to understanding how to label photos. Recently, I typed in sunset, I was looking for a sunset picture for background, and I found not to sunset but other images that were also very relaxing. So I just typed in the word relaxing. So I typed in an emotion into Google Photos, and it could associate emotion with those pictures. Well, that shows you that they're really really, every month they're getting better and better if people haven't played with Google Photos, it is just freaky to see the quality of the labeling that they have for those images, and how they're doing the same embedding for pictures that we're trying to do for our patients, right? break it down into a set of vectors and compare those and distance for similarity. Every word now can be associated with certain concepts that you type in. And you can associate those concepts with a dimension, just pictures. That's really, really cool. Yeah,