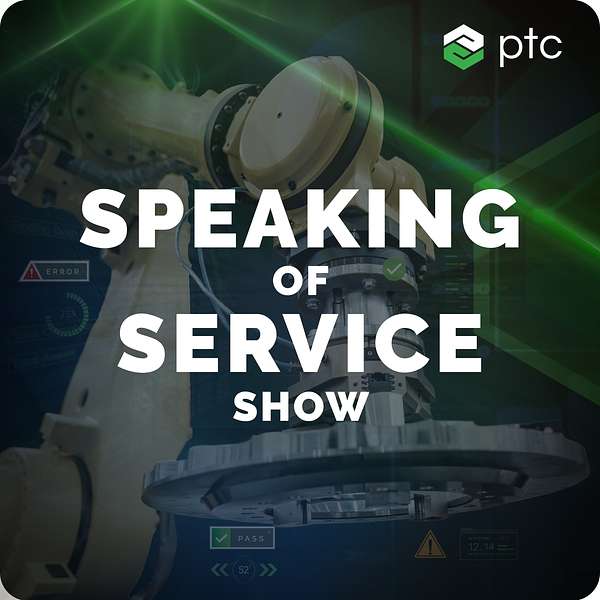
Speaking of Service
Speaking of Service uncovers practical ways to grow service revenue, control costs, and improve customer satisfaction. If you’re looking to innovate, gain a competitive edge, or just learn about the latest service trends, you’ve come to the right place! Also check: www.ptc.com/speakingofservice
Speaking of Service
Value Engineering and the Economic Value of Data
Read Danny´s Perspective on Machine Monitoring and the Journey to Service Performance
How to think differently about the economic value of data and investing in appreciable data assets. In this episode, Chris MacDonald speaks with Danny Jackson, Digital Transformation Director and Brian Allred, Global Director, Data Analytics and Digital Technologies at Autoliv about their digital transformation journey and how they've evaluated the strategic value of data.