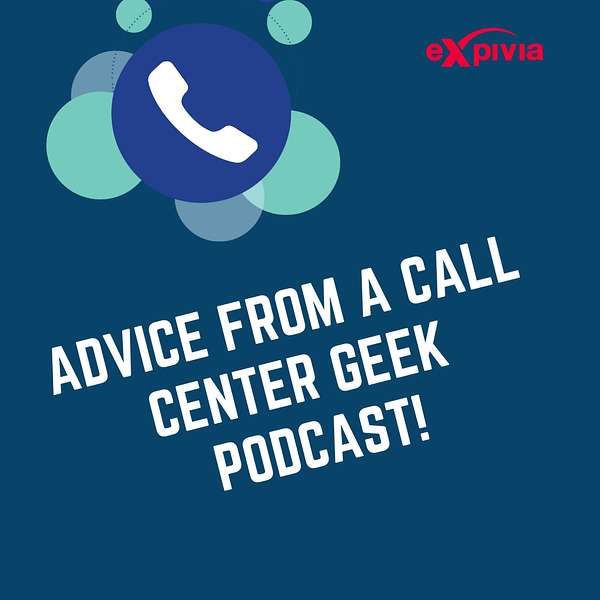
Advice from a Call Center Geek!
Advice from a Call Center Geek is a weekly podcast with a focus on all things call center and contact center. Tom Laird, CEO of 600+ seat award-winning BPO, Expivia Interaction Marketing and Ai auto QA startup OttoQa, ICMI Top 25 Contact Center thought leader discusses topics such as call center operations, hiring, culture, technology, and training while having fun doing it!
Advice from a Call Center Geek!
A Deep Dive into the World of AI & Human Interaction with Deepdesk CEO Robbert Dijkstra
Ready for a peek into the AI-driven future of contact centers? Dive in with our special guest, Robbert Dijkstra of Deepdesk. Discover how AI transforms both the agent and customer experience in digital mediums like chat. Robbert shares how automation not only boosts productivity but also reduces repetitive tasks for agents.
Grasp the crucial role of data in shaping large language models. When integrated with customer interactions and context, the results are a stellar customer experience.
Delve into the upcoming trends in language models for contact centers and the tech advancements businesses can embrace.
Tom Laird’s 100% USA-based, AI-powered contact center. As the only outsourcing partner on the NICE CXone Customer Executive Council, Expivia is redefining what it means to be a CX tech partner. Learn more at expiviausa.com.
Follow Tom: @tlaird_expivia
Join our Facebook Call Center Community: www.facebook.com/callcentergeek
Connect on LinkedIn: https://www.linkedin.com/in/tlairdexpivia/
Follow on TikTok: https://www.tiktok.com/@callcenter_geek
Linkedin Group: https://www.linkedin.com/groups/9041993/
Watch us: Advice from a Call Center Geek Youtube Channel
This is advice from a call center geek a weekly podcast with a focus on all things call center. We'll cover it all, from call center operations, hiring, culture, technology and education. We're here to give you actionable items to improve the quality of yours and your customer's experience. This is an evolving industry with creative minds and ambitious people like this guy. Not only is his passion call center operations, but he's our host. He's the CEO of Expedia Interaction Marketing Group and the call center geek himself, tom Laird.
Speaker 2:Welcome back everybody to another episode of advice from a call center geek the call center, contact center podcast. We try to give you some actionable items to take back in your contact center improve the overall quality, improve the agent experience, hopefully improve that customer experience as well. As you well know, my name is Tom Laird. I am the CEO here at Expedia, interaction, marketing and AutoQA. I have a very special guest. We've been working on this episode for really almost maybe a couple months now to get Robert and his company on to the podcast to talk about a lot of different AI aspects. It's been really fun to see the different perspective people have on AI as it relates to the contact center and to CX in general. These guys have done it in some really cool ways, from the digital side now moving to the voice side. Let's get into this. But before I do that, robert, why don't you give a quick intro to the audience of you and talk about deep desk a little bit and kind of where you guys are at and what you guys do?
Speaker 3:Yeah, thank you, tom. Good morning. My name is Robert. I'm a CEO and founder of deep desk. We started in 2019. We're in the business for five years now. We've basically seen the AI growing, especially in this market, which is now very known, especially with the, the chat GPDs launching last or beginning of this year. We've been in this space for some time now and we're an Amsterdam based company. We're basically focusing on Europe and, since Europe has a bit more of the digital channels already, this is why we focused on these particular channels we launched.
Speaker 3:We're now 20 people running in a few larger contact centers, especially the enterprise larger enterprise in Western Europe. This is basically insurance, banking, maybe telecom, these kind of companies with larger operations. What we built is an agent assist agent assist helping the agents in the contact center, making their work and their life easier. One of the things that we really try to focus on is and we call this thinking human is building technology that is helpful to humans in general. I think we're an early player in terms of the AI and bringing that to the contact center. I think it is a hugely interesting space because there's a really, really an effort going on in contact centers, also in the customer experience in general. Making that connection to your customers is really important. That's why we are trying to help with AI and then especially with the large language models, which we'll talk about as a huge opportunity for even more capabilities there.
Speaker 2:Thank you, guys. Before we or as we go on here again, we're doing this as an AMA. We're on TikTok, on LinkedIn. If you guys have any questions, please throw them out and we'll try to get Robert to give his thoughts on anything that you guys may have as we jump into this. I want to get your take on AI and the speed of it. The cool thing that I thought talking to you guys was I know you're moving to the voice agent assist, but please correct me if I'm wrong you guys started more in the digital meaning chat, meaning those types of things. From now, when people think AI and they think that gender of AI model, that's not something maybe they think about. Can you talk about that? Because I thought it's really cool what you guys do in that space, because I have not seen another company really doing that.
Speaker 3:Yeah, I think what for most people relates to it. As much is like if you do a Google search, it will give you recommendations, auto completing your sentences, these kind of things and this is very much applicable in digital channels chat, messaging, email. This is where we started, especially with Vodafone in the Netherlands, we saw an opportunity to learn from conversations from the past, create an AI model for that and put that into production with the agents there. They liked it a lot. This is five years ago, so we started with a small POC and this was in chat only, so they were doing, I think, tens of thousands of conversations via chat towards their customers. They've now switched to more of a messaging model, which is probably something that happens in the US along the way as well, and so we started building models that could replicate the behaviors that good agents have in the contact centers and then helping agents ramping up faster but also not typing everything the same day every day. So if you have long sentences with questions and everything, if you put that forward as a suggestion, if you just auto complete those, it's really helpful because it takes a little bit away of the pain that the agents have the employees in the contact center doing everything a thousand times a day, it gets really repetitive. So we tried to take that out and also the digital channels.
Speaker 3:I think especially the Netherlands is really forward in bringing those digital channels as a medium for contacting your customers, whereas voice has been declining already and I think that's a trend that you see across Europe and it probably will be more of the digital channels in the US as well, and it's also a space the digital channels are far more eligible, you could say, for automation, if you like, with AI. In voice there's a one-to-one connection between an agent and a customer, whereas many contact centers like they run maybe three, four, five or six conversations simultaneously. And if you can automate within these conversations then you can really well basically enhance productivity, also the quality within these conversations. So we started the other way around.
Speaker 3:We didn't start in voice and it's also, I think, this is what we alluded to a little bit of the difference between the US and Europe, maybe as well in service versus doing outreach as a more of a sales channel.
Speaker 2:I mean you talk about a saving and efficiency. It's almost like you know when, when, when, when and again. This is probably a bad analogy, but when you make an outbound call for a sales call and you get that sale and then you can just send the disclosure, right, so you don't have to have your agent read the disclosure. Like that saved outbound contact centers a huge amount of time. If you have four or five chats and you're, you're able to auto complete so many of those, we're not typing the whole thing. That's crazy efficiencies. It's like making a literally a phone call that much shorter.
Speaker 3:Yeah, exactly, and the opportunity for that in the digital channels is far greater than in the voice channels, even in our opinion. And so, for example, dhl they're almost 50% of over 50% of all the text they type in their contact centers is generated by deep test. And we do this with not so much with the generative AI, but even with AI that has been established before, which is even more cheap and more effective to run. So you don't have to you don't even have to put large language models in there. We do use the large language models, but more for complex use cases. So we just build a stack which is with simple algorithms on the lower end, and then, if, if, the complexes use cases are requiring more and smarter AI, we put those in on top. But those are more costly and also more slow in terms of delivering those suggestions. So we try to make it with very effective algorithms at the bottom end.
Speaker 2:Do you see a difference when it comes to? I mean, I know there is a difference, but when it comes to the back end, that AI or the large language models when you're dealing now, getting into the voice agent assist compared to the digital side, how do those correlate, or do they not correlate, or is it the same thing, but just a different output? What is the difference between those from under the hood?
Speaker 3:From our perspective, what we do with, for example, the auto-completion sort of text recommendations, at this point in time it's still too expensive to do that with a large language model. It's just it's not fast enough and it's too costly. So you better deploy, let's say, more efficient and less expensive models which are perfectly capable. Then, once you start to get into other use cases, building on top of that, maybe summarize. Summarize is a good thing to do with large language. Everyone knows this.
Speaker 3:What we are building now is mechanisms where you say, okay, if this happens in a conversation and the large language model will evaluate that, then give a suggestion, maybe to the agent saying okay, there's an insurance being sold and there's something of a cross-or-enough, so that can be solved with that.
Speaker 3:That's something that we had to train specific models for in the past, but now you can do that with large language models. So the large language model is in real time evaluating what is happening between the customer and the agent, and then you can put forward all sorts of mechanisms saying okay to the agent maybe you should bring forward this opportunity, or maybe you should do this, or maybe you should do a little bit of a compliance check first. So the large language models are enabling different use cases versus that the digital channels, where you have the auto-completions, which are more based on historical information. So it's a combination of both that makes it interesting, and this is also why we say you can use our models for it, but you could also bring your own model, which is something that we offer within the platform as well. If you have trained models, you can integrate those with deep desk as well.
Speaker 2:When you guys started this kind of as your proof of concept five, six years ago on the digital side, as you said, did you see the speed of this, like how fast things are coming? Did you think you guys were way ahead of the curve? Have people caught up? Or does it kind of blow in your mind how fast things have gone in the last, say, 12 to 18 months?
Speaker 3:Yeah, in a way it is, especially since the last maybe 12 months. So, with the explosion and the exposure of GenAI to the general public, we were building the large language models already for our customers in 2019, 2020. So those were more like if you have GPT 3, 3.5, maybe four. Now those were more alike of the GPT 2. You could still run those on, let's say, hardware that is fairly reasonable to get within the contact center space. As with the larger language models GPT 4, you kind of it's hard to run that yourself. You got to get an instance, or maybe you run a little bit, of a smaller model but maybe a 7B or a 30B model.
Speaker 3:But we've been working on this for a very long time already, so we knew what the power was.
Speaker 3:But then, I think just over a year ago, we were looking at these like GPT 3, and we were saying, okay, this is really, really, really getting powerful.
Speaker 3:This is a little bit overwhelming for us as well, and that's also why we started to shift towards okay, how should we build the company from here? Are we, how are we going to embrace these large language models? And I think we did, and this is also what is powering our voice proposition right now, and I think it's really a nice opportunity working with these large language models. So still, it's the first thing that we do now is getting more automation, either to getting the right things in front of the agent or doing RPA kind of things. It will get more interesting over time if we incorporate the context of the customer and the company into these large language models during the conversations that you have as well. So the large language model may know about the customer, which products he or she has, and then being able to put that forward in the conversation as well. So that will be, I think, a really interesting development which we are building towards also with our own large language models.
Speaker 2:And sorry about that. I was trying to move my phone I got there's a couple of questions on TikTok I was looking at, but let's talk about the large language models and in the future of that. I have a kind of a theory, and I don't think it's just my theory, but a lot of the contact center space today. People don't realize how important their data is. So if I'm using a C-Cast platform, a nice CX1 to Genesis, to 5.9, any of those types of things, my data is very hard to access. That, I guess what you see is the progression for, say, a regular contact center that's saying, hey, we want to start to build, we have this huge amount of customer data, we have a huge amount of agent data and how we have dealt with these type of issues. What is your thoughts on starting from scratch, I guess, to build these things out? Where do you see those players? Data is going to be gold to be able to start to access that.
Speaker 3:I know that's a lot in that question, but if you can maybe touch on some of that stuff because I think that's important, yeah, I think what we see happening is that, at the end of the day, it might be a bit different in two or three years, but I think it will end up in the CRM space. So the CX and the CRM will come and join together. This is what you see with Salesforce, this is what you see with Microsoft going that direction. I think what we've chosen as a route is that we bring our technology towards where the agent is doing his or her work, so we make it like little components that you can deploy anywhere.
Speaker 3:We are the platform then to plug into, for example, your knowledge base or this data source. Bringing that together, bringing the information towards the agent. Then the agent is able to handle the questions that the customer has, because all the information is there at the same point. So to us, it's really not so much where, whereas what kind of system do you use? We just deploy the technology as an AI layer on top of the technology stack that you've chosen. But ultimately, I think especially since you're saying the data is where the goal is maybe, I think, if you turn that data into the LLM and then start doing things, that's the magic. I think that's the game that we'll see in the next maybe two or three years.
Speaker 2:Talking to some of my friends in the C-Cast space too. They're talking about. It confuses me and I know it confuses a lot of friends in the space and colleagues. So there's instances of bringing quote-unquote like private chat, gpt to your organization or having some of these other third-party AI models. What is your take on that from? Do you see, like a Walmart, like going back route from their CX to have, like from a privacy stamp or everything? I'm going to have my own yeah.
Speaker 3:I think what is happening, what we see with our customers we are trying to be flexible towards them. So one of our customers is a large bank. They basically have a lot of requirements in terms of security and safety and everything which is obvious for a bank. So we give them the option say, you can go with our models for, let's say, the lower end of the things and then the things that need to run fast. Then there's providers or, like the OpenAI, but also Microsoft and Azure is offering GPT, then it's three and a half or four. Maybe we can provide that service to them via our instance, which Microsoft offers or OpenAI is offering, or they can use their own. This is what we bring, your own model in that sense, so they do have control of that large language model. In that case, then we can build with their large language model towards the use case that they ask us. So we're pretty flexible in it.
Speaker 3:But what I will see and what we are currently doing is like what I've said in the past is I think the large language model is like doing shopping with a truck, whereas if you can have a car as well to do some shopping, and then I think larger language models are good, but for many use cases, the smaller language models which we will deploy ourselves with maybe integrating the data from our customers so bringing all of the data that they have conversations, maybe, but also customer data and putting that into a large language model and then using that as a private version for a bank or an insurance company is really a big opportunity, and we help those customers by providing these types of services and then providing those types of LLMs as well.
Speaker 3:So I think that's a way we will be seeing. So smaller LLMs essentially, which are more focused towards specific use cases, and then you don't have to pull the whole large language model, which is more of a generic model, of course, which has learned from the world. Basically, it should learn from the knowledge within the organization, and I think that's something that we'll see happening, maybe next year already, with vendors doing that.
Speaker 2:And a question I probably should have asked and I normally ask everybody if it's at the beginning, but this might sound a little out of place here but can you define, like, how do you guys at deep desk, how do you guys define AI? Like what is it for you guys Right? Is it this like ultra learning aspect? Is it just really good algorithm? What is your definition of what AI is? It's probability.
Speaker 3:Okay, that's it, I love it. Yeah, and I think we've well. Personally, I have had some sleepless nights over oh, is there an AI which will run away, like this? Discussions with Sam Elton and all these people saying, okay, yeah, this might be something that is really tricky, and it still could be tricky at some point, like I'm not completely sure about the Terminator, kind of story line.
Speaker 3:But I think up until now it's probably probability, which humans are capable of liking a lot, because we see behavior that is human and that's why I think we are so much interested in it.
Speaker 3:It's giving a reflection of humanity back to us and that's also maybe why we are putting more into it than there is actually is, because it's just a prediction of words for a next sentence, that's it's a statistical model with a lot of parameters, so in that sense it's only probability and there's a lot of debate going on on whether this is true and whether it will become at some point maybe like aware or not. But I think we're not there yet. But it's very hard, at least from my perspective, to make, let's say, a good prediction about, okay, when will that be, or will we have like a general AI or these kind of things. But up until now, to me and to us, it's still probability. It's just okay. We predict based on the data and apparently there's a lot of information in that data which we are not able to process as humans, but there is for computers and they have been becoming more powerful every year with Moore's law, etc. So I think we see the results from there.
Speaker 2:That's going to make a great video. I'm excited to chop that one up. Robert, that's, I think, a little bit not a different take, but I think you articulated it how a lot of people think about it, so I appreciate that. Let's talk about your new products. Let's talk about you moving from the digital side and I know we talked a little bit about that already and moving to the voice side from an agent assist, but talk about some of the cool things that you guys have on the plate to kind of put your stamp, kind of in this AI world as it relates to stacks.
Speaker 3:I think what we see I always like to talk from the perspective of our customers we create like slide decks and I say, okay, these are a couple of ideas that we have.
Speaker 3:And then one of the things that is getting a lot of traction that we run with these customers is the knowledge assist, as we call it.
Speaker 3:It's basically we get their data in terms of the knowledge base that they have. This might be a knowledge graph or knowledge base with like documents, instructions or the way they work or operate, and then we train the model with that and basically what we put forward with the agents is a Q&A mechanism, much like chat GPT. They can ask all sorts of questions and it will take into account the context of the conversation they are currently having with the customer. So the large language model is aware of all the knowledge base information and also aware of the conversation going at that moment. This works for voice and for the digital channels as well. So you can implement it as a widget within a CRM system or as a layover, like command K, if you like, and call it and then just ask questions, also repeat questions, just like you would do with chat GPT, for example. It will then give you answers which are really much tailored towards the information that the organization holds. And that's interesting because we have some examples yes, so this is an insurance company.
Speaker 3:They have like an instruction for their people working in their contact center. It's 150 pages and that's hard. It's hard to take in those kind of like these amounts of information, and they do ask this from their people. So we put this into the knowledge graph, saying, okay, this is the knowledge assist and these agents then can ask these questions that they have during the conversations. Towards this, maybe it's a little bit of a chatbot, it's a colleague to them and then answering the questions. But the nice thing is it's kept up to date automatically, because if procedural changes are being set into the system, we get a copy of it and then we update the knowledge as well and the knowledge assist will be capable of giving new answers. It's just generating answers for the customer. In other cases we provide that, for example, within Genesis, one of the customers is using it to have an intent recognition at the beginning of a voice conversation. We then take that intent as in okay, I want to maybe get a new credit card and then put that in into the knowledge assist and it will come up prior to the call even being acknowledged as okay, this is what you should do, and then the agent is already on top of what he or she should do. I think that's really powerful because what we see, if you take the stack of the work that people do in the contact center, and a lot of it is not talking or typing. A lot of it is looking up information, getting records in, getting talks out. So I think we are trying to reduce the number of things that they the time that they spent looking for things, and I think one of the knowledge assist is really powerful in that and it works for digital and voice channels as well.
Speaker 3:The other thing is what we are launching now is routing, so the conversations might come in. We try to assess with a large language model okay, this is a typical question for team A, oh, this is a typical question for team B and if the conversations get in the right place, that's really helpful. We see an uptake from, I think with previous systems, one of our customers was doing 25% of correct routing. With the large language model, we see 75%. So then that really helps in getting the things done right. And the other thing is what we call actions and notifications. We evaluate continuously the conversation that is happening between the customer and the agent and then we can put forward if this and that. So the agent manager or the contact center manager might put in rules saying, okay, if this is happening.
Speaker 3:So for example, if the agent is trying to send a mechanic to maybe somebody at home, it's an expensive transaction the customer needs to sign an approval for that, because otherwise the cost will be for the company and in this case we can just pop up a little message saying okay, you need to make sure that the customer signed off on the mechanic and the cost that come with that. And the power is that these Rules are just prompts. The prompts are being done by the content center manager. They don't need it, they don't need a I data science, they just pull in this information, put in a prompt, saying okay, if this is the case, then I would like you to show a message, or if this is the case, I would like you to run this action. And it's super powerful because you bring back the, the power of the large language model to the business Instead of the IT organization, and I think that's something people have been looking for for a long time as being able to deploy these kind of things in the conflict center.
Speaker 2:Do you think through and in my Just always a question I have for for some of my other, you know, kind of friends that are in or using agent assist or some type of model that's kind of like that. That's that's coming out now is you know what's what? What is too much for the agent, right, like what you know from getting away from, from the just that, but the actual UI, ux of the agent sitting there doing it, how do you think that through of what you give and what you don't give?
Speaker 3:Well, it's an excellent question, because this is why we wait while we came up with a thing human we really tried to take in. Okay, what does this mean for the people doing their work? I think, like in IT, for the last 20 or 30 years We've been building and I don't like to be bragging about it, but it's like not good system for people to use, like it's like building a door with a knob that's not functioning and there's plenty of that in in every industry, like CRM's or CX systems. So we try to focus on that and if you do that, the agents are happier. They will stay longer. If agents are happy, they will provide for happy customers as well, because that's the relationship that they have. So we have a lot of focus on the agent.
Speaker 3:So one of the things that we provided them with is what we call personal collection. We we noticed that many agents in the contact center had lists of sentences that they had on a notepad and we put that into details. We asked them to say, okay, just put it in there and then we'll all complete it for them. It's not even AI, but it's helping them out in doing their work properly. So listening to the, to the user and then implementing those kind of things Even if it's not AI or not super fancy, but just giving them the right tools is helpful in doing your work and we enjoy doing that, because there's beauty in in providing nice tools, at least from my point of view.
Speaker 2:Customers, customers and agents don't care about AI, they just care about the experience. Oh, so you don't need it. Like, yeah, if you can, if you can do something quicker, less expensive, then I'm. I think we are all, everybody in this industry, especially in my, in the BPO space right, you have to be a AI centered BPO context, and you have to say it when I don't even know really what that means. I know that every tool that I have for my partners or my C-Cast partners has AI infusing it. So I guess I'm using that, I'm using agent assist, so I guess I am, but no one cares. Yeah, right, it's just what is the end result and if we can make it better.
Speaker 3:Yeah, I think one of the this is also for DHL at some point the agents, if you turn it off after trial, they become really, really, really, really, really angry because they need to go back to like, okay, well, now we need to start typing again. You know we were used to three months of non typing, whereas we needed don't need it to do. And the other part is I that's good proof. I think the the guys from the DHL Contact Center were asking for t-shirts with deep desk on it because they liked it so much, and I think that that's really proper validation of the product being lost by injuries.
Speaker 2:Where do you see this going? And let's focus on you guys specifically from a from a deep desk standpoint, and again I don't want to like get any secrets or anything that you guys are working on, but you know, as an overall, maybe industry, that you guys and in that kind of that part that you are in in the next, you know, I don't want to say 10 years because that's way too long, but in the next three to five years, what? What are some of the things that you can see kind of coming out that we can all kind of expect?
Speaker 3:Yeah, what I will happen, I think, is General use cases that you should, such as summarization or these kind of things that will be everywhere. It's like the co-pilot Microsoft is offering and just in the, in the general sense, just doing your work, those kind of things. We will be much more focused on the use cases that are specific to the customer contact center, and there are many of those there's. There's so much repetition going on and and I think that the large language models have given us an opportunity to get even more work which is repetitive, which nobody likes, where where nobody gets any interest from To do more automation from that perspective, but what I think where where the real value will be is maybe getting all of the data in one place and then center it and then use that to to to basically get better customer satisfaction. So, because what I think is really that the the sense of the problem is that the company usually has maybe issues or problems in their processes which are leading towards customers reaching out, which is obviously fine. But you should not be solving problems. You should be creating valuable connection towards your customers. I suppose, in terms of having a relationship, that should be the goal. One of our customers of ours is a bank and they are really saying we want to automate the part that it's not interesting and we want to have the agents more time for interaction with the customer. I think large language model will power way more of that and that will create more value.
Speaker 3:At the end of the day, I think what we will do is build our own LLM, which is already around the corner. It will be smaller, but it will be more focused. It will integrate all of the data that our customers have. We bring it together and we'll try to make the experience for the agent better At some point. It might be as good as that. You will be talking as a customer facing application as well. It could be something that is happening for simpler use cases. We never had a really good experience with chatbots in general. There might be a couple of use cases that are very powerful, but those are fairly simple in general. But I think, talking to a large language model with the information, with everything, there would be something that you will be doing in maybe two to three years that might be applicable to every company. But if you merge that with agents and employees, I think that's the most powerful stuff.
Speaker 3:Nobody wants to do the run of the mail stuff that's not what we're here for I think if you can take that out of the equation and get more into the attention towards the customer by having the right information in front of you and helping these customers out, I think that's really powerful.
Speaker 2:I got one or two more questions for you, but I'm going to ask you a selfish question. Being a BPO and being here in the States there is the number one conversation that we're having. Actually, I'm going out to Google tomorrow to talk to a bunch of BPO's on this exact topic. I'd love to get your thoughts on this too. The BPO having an agent in a chair answering calls days only, is done.
Speaker 2:There needs to be what you guys are doing, the incorporation, not only maybe taking. I mean I'm planning on a 20-25% drop in my bond based on customers that want to use that just front end, first touch point generative AI. But I think more customers want to go your route. How do we infuse? How do we help the customer? What do you tell the BPO? Again, I'm with you on this we need to grow up. We're no longer call centers. We need to be technology partners for our clients. That's the route that we're taking, but there's a lot that are not, that are nervous and scared about this. What do you say to the BPO that has had this model forever and now, with technology like agent assist, like all these types of things to infuse with how the agent experience works?
Speaker 3:Well, there's an inherent issue that the business model of BPO is the other way around. So we've been talking to a lot of BPO's in our five year and they have been very much hesitant to implement agent assist, either for the customer that they serve or for themselves, because they just don't feel like we need to turn around. They didn't feel any pressure for that because they're billing hours. That's the principle of it, even if they could make a better book, I think, if they implemented agent assist, but somehow it wasn't getting through. We have done a lot of talks with BPO's and at the end of the day we decided we will do only business with the direct customers because it's easier to explain it to them. It might be a bit of a harsh message, but it just doesn't work out.
Speaker 3:What I think is true for BPO's is being more on the, let's say, partner for IT, like bring new technology in and, I think, expanding into where are humans valuable? So you have humans in a contact center. These are people. That's the value that you have towards the people that are the customers of your customer. So I think somehow finding a route to leverage that more, that these humans are talking to humans, and that should be in the higher let's say conversations. That's where the value is.
Speaker 3:I think many people want to talk to humans still because they like it. It's just a human connection that makes a lot of things stick. But people don't want to talk to a human to change out their credit card. They want to do that process in a different way, like, okay, can we do it automatic because, okay, that's how I like to do it. But I think the shifts should be towards the higher end type of conversation and not so much the drain where every shit goes or what. I'm not sure I am allowed to say that, but I think using the humans in the contact center and then giving them the position to be human towards the customers, I think that's a valuable route.
Speaker 2:This is going to be the first. These types of technology, in my opinion, are going to be the first technology that is not going to be vendor driven. It's going to be customer driven, because I guarantee you and I've not seen it yet, but the next, maybe in 2024, 2025, every RFP I'm going to get is going to be like you must have agent assist right, you must have this right. It's going to be customer driven. It's not something I think that BPO should fight, can fight Just thinking about that model, but again, for when it's customer driven, it's a whole different deal than being vendor driven. I think that might be the first real piece of AI technology that does that.
Speaker 3:Yeah, I agree.
Speaker 2:Yeah, hey, man, I really appreciate this. Can you just maybe give your last pitch? If anybody wants to go to the website, if anybody wants to see anything, if everybody wants to take a demo, also any of your social media stuff too, please? You got the last 30 seconds minute here to do what you need to do.
Speaker 3:Well, we're at deeptestcom. We're very much keen to help you out. Doesn't matter to us when your volumes are. We can plug into the large platforms that are out there and we can help you with our agent assist for digital channels, but also for voice. We'd really like to do that. I think we offer quite an extensive opportunity also in the realm of large language models, which we then have. If you're, let's say, a CEO of a company, this should be on your list, I think, because this is where the money will be the next couple of years.
Speaker 2:Robert, it was great talking to you. It was great talking to you over the last couple of weeks as well. You and your team, and Brendan as well, who's kind of in your leadership team. You guys are really smart. I think you're taking this on in a different way, which is really unique in the space, and taking all the things you guys have learned from the digital side and putting it to voice, where most people have done it the opposite way. I think that's really exciting to see what you guys do.
Speaker 2:Let's definitely stay in touch, but I appreciate it. Thank you so much for coming on, yep, looking forward. Ciao, all right, robert. Thank you, buddy.