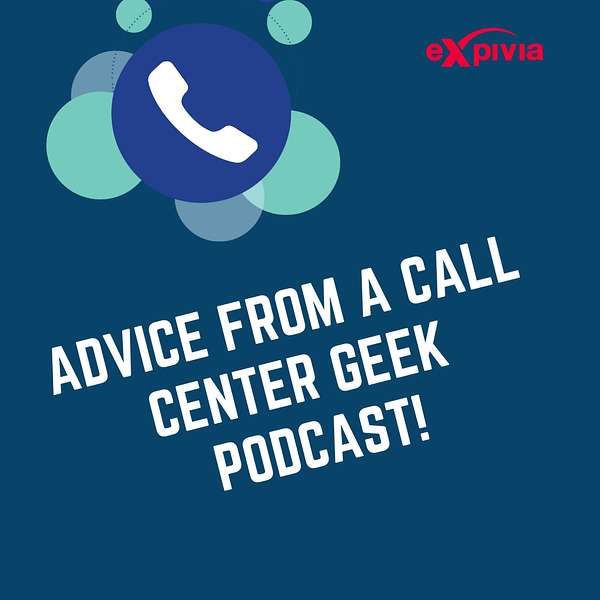
Advice from a Call Center Geek!
Advice from a Call Center Geek is a weekly podcast with a focus on all things call center and contact center. Tom Laird, CEO of 600+ seat award-winning BPO, Expivia Interaction Marketing and Ai auto QA startup OttoQa, ICMI Top 25 Contact Center thought leader discusses topics such as call center operations, hiring, culture, technology, and training while having fun doing it!
Advice from a Call Center Geek!
Everything We Learned from Starting An AI CX Company - OttoQa
Join us as we dive into the journey of founding and growing OttoQa, a cutting-edge AI-powered Customer Experience (CX) company. In this episode, we'll share our firsthand experiences, lessons learned, and the challenges we faced in building a fully automated QA platform. Discover the strategies and insights that helped us innovate in the CX industry, the role of AI in transforming quality assurance, and tips for aspiring entrepreneurs. Whether you're a CX professional, tech enthusiast, or entrepreneur, this podcast is packed with valuable takeaways to inspire and inform your journey. Tune in to explore the future of AI in CX with OttoQa!
Tom Laird’s 100% USA-based, AI-powered contact center. As the only outsourcing partner on the NICE CXone Customer Executive Council, Expivia is redefining what it means to be a CX tech partner. Learn more at expiviausa.com.
Follow Tom: @tlaird_expivia
Join our Facebook Call Center Community: www.facebook.com/callcentergeek
Connect on LinkedIn: https://www.linkedin.com/in/tlairdexpivia/
Follow on TikTok: https://www.tiktok.com/@callcenter_geek
Linkedin Group: https://www.linkedin.com/groups/9041993/
Watch us: Advice from a Call Center Geek Youtube Channel
This is advice from a call center geek a weekly podcast with a focus on all things call center. We'll cover it all, from call center operations, hiring, culture, technology and education. We're here to give you actionable items to improve the quality of yours and your customer's experience. This is an evolving industry with creative minds and ambitious people like this guy. Not only is his passion call center operations, but he's our host. He's the CEO of Expedia Interaction Marketing Group and the call center geek himself, tom.
Speaker 2:Laird. Welcome back everybody to another episode of Advice from a Call Center Geek the call center and contact center podcast. We try to give you some actionable items. Take back in your contact center, improve the overall quality, improve the agent experience. Hopefully can improve the customer experience as well. Oh, how's everybody doing? My name is Tom Laird. Most of you guys know me by now. I would hope.
Speaker 2:It's been a while since we've done a podcast. I have been slammed with Expedia things and with Auto QA really launching and really starting to take off with lots of companies, so I get a lot of questions on auto. I wanted to answer as many of them as I possibly can of my list here and talk about everything that we basically have learned in the last 13, 14, 15 months of starting from scratch with an AI tool, doing it for our internal customers first and then and then moving it to a product that customers are actually paying for or using. It's been really awesome. So I'm live on LinkedIn, live on TikTok. If you guys have any questions, please, please, please ask away. I'm here to answer anything. We'll do this as we normally do as a full AMA, but let's start with some things that I think I didn't think about and, I think, some interesting things, starting with the models that we use. So when ChatGPT 4 came out is really when we started to say, okay, I think we could do something with an AI tool. So, as most of you guys know that are following me, we initially built AutoQA for our internal customers and that's who. We kind of tested it and kind of learned what we needed to do. We thought it was going to be pretty easy. It was nothing easy about it. I know a lot of people just say, well, all you guys are doing is building a GUI on top of a large language model, and I guess, yeah, that's kind of what we're doing, but there's so many intricacies, so many things you need to do about prompting, so many things that our system prompt has to do, that we learned a ton. The main thing that we learned is ChatGPT 4 and ChatGPT 4.0 are not good models for what we are using this for. I don't know if people disagree with this. I've not really talked to nobody's really talked to me about what models they're using in this kind of auto. It's kind of CX space, right. Everybody's kind of hum and hush on that. I'm pretty open with it, although it's cheaper, right.
Speaker 2:When 4.0 came out, it cut our costs in half, but our accuracy was also cut in half. So very quickly the excitement of 4.0 turned into man, this thing. Just, it's almost worse than 4. So that's when we started to say, all right, well, what other models are out there? And we tested pretty much all of them. The ones, the only ones, that we have found to be absolutely the best use case for us have been Anthropix products. Right. So we use Haiku to go do summarizations. Right. So if somebody has 150 forms, we want to summarize the four things that they've done. You know, good or poorly, on those 150 calls that we've evaluated, we just send it out to Haiku. That does a really good job of just kind of summarizing very basic stuff.
Speaker 2:We initially started with Sonnet. We thought Opus was going to be too expensive. Sonnet was a huge step up from 4.0, but it still did not give us the same consistencies. And it's kind of like an oxymoron to say, well, if something's accurate and it's not consistent, is really accurate? And I mean the answer is no. But we would see. You know, out of out of 10 questions that we asked, sonnet would be right eight or nine, but then one time, you know, would just give us something weird. So we worked through a lot and and, to be honest, that's where we learned a lot of things from a prompting standpoint, that would help with consistency. Accuracy is basically just the core prompt and if we're not accurate, then we have a prompting. We're telling it to do something. There's wording wrong, there's something there. But when it came to just the consistency of scoring, that's where some really cool things. We have seven kind of checks within our system prompt to make sure that we are being as accurate as possible with our answers. So I think you guys have heard me talk about this a little bit, but we reiterate every single question.
Speaker 2:So even if it costs us more in tokens, you know, whatever we're doing, our mission is to be the most accurate and the most consistent auto CX, auto QA platform on the on the market. So the one thing that we found is that if we said okay, let's, let's take a question like did the agent, um, did the agent use the proper greeting, so we will say ask that question and answer it large language model but then hold that like in the back of your AI head and then we ask the question again and if both of those match, then boom, we give the answer yes or no or whatever we're scoring. If we still have some type of inconsistency, it's a yes and a no Then we say for a third time, don't ask the question, but go back into the transcript, search for where you need to find that answer right I'm paraphrasing here and then whatever is closest, score that. And we found that to be extremely consistent too. So we do about six or seven of these checks on every single question, with some different techniques based on what we have found to give us the most consistent answers. So it's been really interesting to kind of learn the prompting techniques and some of those things that you can be just. I guess you can just be so creative with this.
Speaker 2:And I think being a contact center, doing a tool like this, like we know what we want, right, we're not just kind of looking for specific keywords. I think I love the phrase that I've been using with this, which is intent and outcomes. Right, we're looking for agent intent and we're looking for did the proper outcome take place? And that's where we can score this thing at a little different level than just again looking for specific keywords Although we're really good at that Like if you have a disclosure and these specific keywords have to be said exactly. Obviously, ai can do that. But AI can also quote, unquote, think right and get deeper into empathy, get deeper into did the statement that the agent made when the customer said this? Did it have an impact on the overall sentiment of the call? Right? You can go a little bit deeper to some of the things that we couldn't do with just analytics and just looking for keywords.
Speaker 2:And I think a lot of the models right now are trying to kind of mesh these two things because they see, with the amount of overhead that some of these larger companies have, that just using the, the large language models can be, can be expensive for them, you know where? For us it's not that bad right, because I don't have a ton of overhead. We, we are doing this kind of in a as a contact center would do it, not as a software company do it, which I don't know if that's good or bad. But I think those are some of the things that we have noticed to make a lot of difference in the actual prompting of the question. So we have found that obviously the system prompt is absolutely vital to tell it the exact outputs that we want. We have found that we can't trust any of the large language models to actually do the scoring, so we do the scoring. We kind of programmatically do it on the back end. Once the answer comes back yes or no, then we scored ourselves with kind of programming. So we know that it's always going to be, you know, spot on from there. You know, tried to do it with the language models but, just again, they're not. They're okay at math, they're not great at math. So you know we had some inconsistencies there early on. That we, that we, we tried to work through the new model that just came out.
Speaker 2:Yesterday, as I recorded this I'm recording this on on the 21st of June 2024, claude 3.5 Sonnet came out and for us it was like Christmas. We didn't know it was coming. We have been using Opus. I guarantee you that there's no one else, no other auto QA platform is using Opus and everybody knows that Opus is the best. It's been beating up ChatGPT 4.0 for a really long time but no one's using it because it's so expensive. That is our core model that we've been using, for everyone is Opus, right, so the most expensive model, but we're getting the best results and we just figured that that'll take care of everything else else. So when 3.5 just came out yesterday, it's about half the cost actually a little bit more than half the cost of Opus, but what we're finding is that it is better than Opus as well. Now we've only been testing this for a day, we've been running models.
Speaker 2:So how we and maybe I'll get into the calibration how we calibrate these calls is a customer will send us 10 scored calls, so we'll have the call and we'll have the maybe it's a PDF or it's a Excel spreadsheet, whatever it is of how the call was scored. We will put that into an Excel spreadsheet as kind of our base. And then what we've been doing normally is just running Opus as the auto brain and then we'll just put in, you know, each question what did the human score? What did auto score, also known as CLOD3, opus, right? Well, how did it score? And then we would calibrate from there. Right, we would tag it blue if we thought that auto was a little bit more correct. We tag it red if we thought the auto was a little bit more correct. We tag it red if we thought the human was a little bit more correct.
Speaker 2:If the human was a little more correct, then we just kind of change and tweak how we're prompting, how we're asking that question. A lot of times it's not the point of being right or wrong, it's being right or wrong compared to the scoring culture of the company. Right, they might be really strict on certain things and they have more leeway in other things where auto initially will be really strict on something. So we just kind of dial that back and we can then match the score and culture of every organization. But what we did, started yesterday, is we'd have a row for the human, a row for we said auto 3.0, meaning Opus 3.0, and then auto 3.5, meaning 3.5, sonnet or sonnet 3.5, depending on how you say it, and it's been really interesting. So a lot of times we have seen that with Cloud 3, opus we match the human a little bit more.
Speaker 2:But 3.5 has been actually we're finding more correct. So we're finding more quote unquote human error in the calls when we're using 3.5. So we're moving to 3.5. It looks like we're going to test this for another day or two as kind of our core model. It's been easier to kind of tweak and also we'll say this we just ran a have a very large customer that we ran the first 10 calls with 3.5, and we were without any tweaking. We were at about 93% or 94% accurate. So we'll be able to get them with our tweaking to 98% how they're scoring, how the human is scoring on their team. So really excited about that I think that's been a little bit of a difference too is that initial run is so much closer now that we have less tweaking, which means less cost for us because we're not running as many kind of calibration runs of the AI. So I think that's kind of been really, really interesting.
Speaker 2:I think you're going to see a war now, or a battle or a sprint or a race whatever you want to call it between Anthropic and OpenAI. You know I would bet you now you're going to see a ChatGPT 5,. You know, within the next month, right, I guarantee that they're pushing it up. You know Opus was the best, but they were so expensive that I don't think anybody was really using them in a fashion like we were. So now to have that cost about it's still a little bit more expensive than ChatGPT 4.0, but it's comparable and it's way better, at least for our use case. I'm not saying 4.0 is bad right, but for the use case that we're using it for it's not even close right. We almost can't even use 4.0 with how poorly it has been able to ask questions of this data.
Speaker 2:So I think that getting into some of the tricks and some of the things that we have found, I think calibration has been something that we're doing and spending a lot of money on every client to get it to the point where we've been fully calibrated. I think it's been really cool to learn some of the prompting tricks. Right, like, like reiteration you know Anthropic likes to use XML text a lot more than than ChatGPT4. So you know, learning that there is a full kind of prompt engineer or a prompt, I guess, helper on the workbench of the anthropic, of open, of of Claude a big problem, but again, I think it goes a little bit too in depth sometimes. We found that the most success we've had is is the human brain when it comes to tweaking a prompt, unless we're way off or something's kind of going crazy, but to get the format right, right with the xml tag, uh tags, I think has been pretty helpful. With that there's, there's just so many more tools on the in the anthropic pool. That I think is cool. One of the things that we're moving to now because visual is starting to come out right and to be good on both of the models is to you know kind of use the forms and kind of feed those forms and let them see the form that a customer has at the beginning.
Speaker 2:To start our initial build out Right. The longest period of time that we have, or the longest length, is the actual initial build out right. So a customer will send us, like their rubric. They'll send us all the questions how do you score these questions? Here's some background on this right. So to build those out takes a little bit of time and we have a we do have an AI tool that we're utilizing to speed that up, but that's something that we're looking to do to make this even quicker. We're currently about 10 days to two weeks. We've been longer for some customers, shorter for others. On the build-out. We won't go to proof of concept until we're at least 96% calibrated. So I think that there's a lot to be said for the tools that are coming out and for how smart the models are getting to be able to start to take other pieces of data input. That will help us kind of as we move forward. I'm very excited.
Speaker 2:The next big step, I think from the model standpoint that we are excited and we are preparing for, is the one thing that we stink at is that we can't see the screen right. We thought that 4.0 was going to be the panacea, that we are going to be able to take the screen recordings and QA what an agent is doing actually on the screen as well, and while we can get data from that right, we haven't really been able to get it to the point where it can QA it. I think that's coming very shortly. That was a little frustrating. That kind of was the oh my God Foros here. Oh my God, I can do this kind of video and this imaging. This is unbelievable. But it's just the processing power. I think that it needed. It just never could get to that point where we could. Put's been a little bit of a struggle there. So we're still sticking with audio. So voice email, chat, sms, help, desk tickets those are all the things that we're really good at QAing. I think the other thing is customers have been extremely helpful with how we should roadmap this thing out. I'm glad that we went out early.
Speaker 2:You know certain things that out early. You know certain things that customers want. You know a lot of different changes to the dashboard that we will be able to see, you know, starting even today with being able to show differentiation how much better or worse an agent is getting. I think those basic type of reporting is important. You know auto-fails were not easy right To do. Auto-fails was something that we're now doing programmatically. So for a section of a QA form we will say we'll just click it into this is an auto-fail section. So if any of them and we'll say we'll click the auto-fail and we'll say yes. So if any of those questions are answered yes, then we will still score the call, but it'll be a zero for the overall call, although you'll be able to still see the sections. So we've been able to tweak some things there. Rated calls we're getting much better at.
Speaker 2:I feel very comfortable now going out with customers and say, hey, I don't want a yes or no, but I want to scale of one through 10, we have one customer that is scoring this zero, one, two or three right, and they get points, you know, based on those different levels and I think we're we're very close and very accurate with those guys. So I think that customer is pretty happy with where things are going, cause that's a little bit more subjective, right, the difference between a two and a three, like they did this, but they did a little better as a three compared to a yes or no. They either did it or they didn't. There's a big difference in kind of how the AI needs to think that through. It's a little bit more subjective, but there's some prompting techniques that we've been able to utilize for that as well. So, yeah, it's been a lot of fun.
Speaker 2:We are getting better and better and better and better and faster and faster of this every single day. I'd love to build this, some of this stuff, out for you guys. Again, remember, no, no, no contracts right now, where there's still no setup. I don't know how much longer we're going to be able to do that for, but right now there's still no setup. Total usage model, right. So no seat licenses or any of that.
Speaker 2:It doesn't cost you anything to run this from us building your form out to a full proof of concept of you being able to do 100 to 200 calls on your own to check it out. So we do all the work for you. All you do is sit back and at the end, score some calls, answer some questions, if we have, and then hopefully we can really do some cool things for you from a cost standpoint, as you know, we can work. The cost of this, compared to a human being, is pennies. So I think that you know you can utilize your teams in a lot of different ways that are more productive as well. So, thank you guys. Again, check us out. Autoqacom, xpeviausacom. Love to talk with you guys either on your outsourcing, also on our AutoQA platform as well.