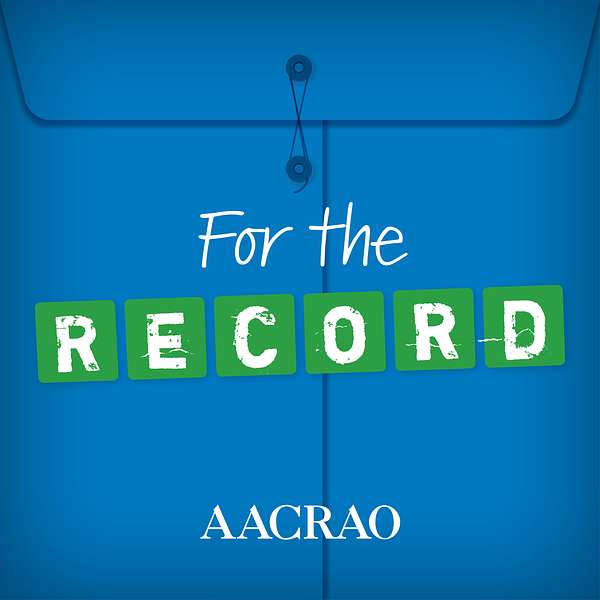
For the Record, An AACRAO Podcast
For the Record, An AACRAO Podcast
Artificial Intelligence in the Registrar’s Office
Artificial Intelligence (AI) is a hot topic everywhere these days. What is it, what are its promises, and what are its current limitations in terms of applicability to the work we do in a registrar’s office. You’ll hear from the Chief AI Officer at George Mason University about the promises and challenges of AI, and from a privacy and information law attorney about some of the considerations we should be making as we pursue any new technology, but AI especially.
Note to listeners: edit implemented on 3/12/25 to remove a small audio gap
Key Takeaways:
- There are many promises of AI, but there are some significant challenges currently, as well. Don’t be swept away by the promises without engaging with the limitations.
- As registrars we have a special responsibility to make sure that the AI we employ maintains the safety, security, and integrity of the data we steward. The Fair Information Practice Principles (FIPPs) can help guide us to positive data governance outcomes.
- Be wary of shiny new things. If the promise of something is too good to be true, it probably is. Explore AI and imagine ways it might be applied in your office, but AI is not the only tool available to us, and (in my opinion) we might be better served by pursuing intelligent automation solutions than artificial intelligence (AI) solutions.
Host:
Doug McKenna
University Registrar, George Mason University
cmckenn@gmu.edu
Guests:
Amarda Shehu, PhD
Chief Artificial Intelligence Officer and Professor, Computer Science, School of Computing, George Mason University
Claire McKenna
University of Notre Dame Law School
Claire McKenna is an attorney with 21 years of experience advising public and private sector clients. Her practice focuses on all aspects of information law, including privacy, security, access, and disclosure.
References and Additional Information:
Fair Information Practice Principles (FIPPs) | FPC.gov
Weapons of Math Destruction by Cathy O'Neil: 9780553418835 | PenguinRandomHouse.com: Books
The Big Switch | Nicholas Carr (Sorry I called you “David,” Nicholas!)
How much electricity does AI consume?
You're listening to For the Record, a registrar podcast sponsored by OCR. I am here to help. This episode is called AI in the registrar's Office. Hello. Welcome to For the Record. I'm your host, Doug McKenna, University registrar at George Mason University in Fairfax, Virginia. I want to kick things off right at the top here by acknowledging that pretty much nothing feels normal anymore. Blaming diversity, equity and inclusion for plane crashes, it's not normal. Having an unelected immigrant billionaire run roughshod over federal agencies, not normal. Thousands of people being laid off, even though it doesn't actually save the government any money, not normal. So I'm going to keep doing episodes of For the Record, and even though it feels weird that every episode isn't just me screaming about everything I perceive is going wrong in the world, I think continuing to talk about issues affecting our work in spite of everything else that's going on is the way that I'm approaching things. I hope that you'll find some solace in that approach, and I hope that you'll give me feedback if you think I'm off base. Today though, we're going to be talking about everyone's favorite topic these days, artificial intelligence, AI. I'm skeptical, which might be surprising to hear considering I'm a big technology person. It's even right there on my LinkedIn profile if you want to check. I strategically, consistently and intentionally apply technology to administrative processes, and if it's on LinkedIn, you know you can trust it. So. But let me clarify, I'm not opposed to AI generally, although some of you didn't grow up scarred by that first Terminator movie, or you weren't radicalized by reading Player Piano by Kurt Vonnegut, and it shows. AI has a lot of issues right now, some of which we'll discuss in this episode. Generative AI is in its infancy, and I question the value of its application to registrars office business practices in its current form. So first, some of my sort of objections, concerns, let's let's say their concerns about artificial intelligence, and I think they're summed up in two quotes. I saw a funny thing on Blue Sky the other day, and it says, It's amazing how Chat GPT knows everything about subjects I know nothing about, but is wrong, like 40% of the time about things I'm an expert on. I'm not gonna think about this any further. And there's another fun perspective offering quote about artificial intelligence that I want to share. I want AI to do my laundry and dishes so that I can do art and writing, not for AI to do my art and writing, so that I can do my laundry and dishes. And that is author and video game enthusiast Joanna Mashika. Mashika. It's M A C I E J E W S K A. And I think that sums it up. These, these are my concerns, and that doesn't even get into the concerns about how much electricity is needed to train an AI model. And so one article that I read from the Verge, I'll link it in the show notes, says that training a large language model like GPT 3, for example, is estimated to use just under 1300 megawatt hours of electricity, about as much power as is consumed annually by 130 US homes. So for context, streaming an hour of Netflix requires 0.8 kilowatt hours of electricity. So that means you'd have to watch 1,625,000 hours of Netflix to consume the same amount of power it takes it takes to train a bot on a large language model, on an LLM. The problem with knowing how much energy it takes to actually run an AI large language model like Chat GPT is that companies have gotten very, very secretive about how much energy their data centers are consuming and what is behind that consumption. It's not nothing. And until we can get to a more sustainable artificial intelligence environment, I'm skeptical of the benefits. I don't think that the benefit of chat GPT right now outweighs. The cost, the technical and environmental cost of getting it to do what you want it to do. And even when you get it to do what you want it to do, as the previous quote pointed out, it's still wrong 40% of the time. It can still make things up. You still have to check it. So I'm skeptical. I could be convinced, but I'm skeptical. But we're going to hear from two guests today. The first is Dr. Amada Shehu, who is the chief artificial intelligence officer at George Mason University, to hear about some of the potential benefits of AI and also some of the challenges. And then we're going to be joined by privacy and information law attorney and McKenna family general counsel, Claire McKenna. Who's gonna provide some perspective on AI from a legal framework. It'll be a lot for one episode, but I think it's worth it. So, without further ado, let's jump in. Right, OK, so happy to be here. I am Amarda Shao. I am uh George Mason University's vice president and chief officer as of September of 2024, a new position for the university, but I have been here since 2008. I am professor of computer science and an associate dean in the College of Engineering and Computing. Fantastic. Thank you for taking time to talk with us today. What is a chief AI officer and what does that position do? You mentioned it's a new position for the university. I did a little digging and it's a new position relatively everywhere. So what, what does it do? Yes, it's a, it's a position that many companies or governmental agencies started in some sense and so it's a position where folks started to think about what it is that they were asked to do or that they wanted to do, but the overarching umbrella is. Due to a recognition of the importance of artificial intelligence to innovation, whether in companies or in government and so in companies, the role is, I would say a little bit more limited than what a role should be for an institute of higher education. In companies it's very much focused on processes, how do we integrate it for efficiencies, for optimizing processes, and then hopefully also for innovating on products and outcomes. At governmental agencies, there's a big overlap with this mission as well. In institutes of higher education, you're right, it's hard to find what it is that a TAA officer does, so in some sense we are defining it here. At Mason, because you, if you go and you look out there, there's probably very few universities where you find this position and in the ones where you find it, it may often be limited to say, uh, schools of medicine, right? So it's not a sort of a widely recognized position, but here at Mason we recognize the opportunity that this position affords not just to integrate AI in our processes, right, in our business operations. Um, but more importantly, to support our students, right, to support students' success, uh, to support and innovate on instruction, on learning, uh, to support research, to catalyze, to grow our research portfolio, to catalyze collaborations across the colleges, to do workforce development, so. We see it as uh a very fundamental, really as fundamental strategy to supporting all those mission areas, all the buckets that an institute of higher education needs to hit, whether that is student success, workforce, research, professional development, right of faculty and staff and partnerships. Right. Sort of a higher level question, when you say artificial intelligence or reference it as AI. What do you mean by that? How do you define artificial intelligence? How are we at Mason and really in higher education, how we think about artificial intelligence? Yeah, that's a, that's another great question because the, the answer to that in some way has been very narrowed, it has been co-opted by what you see coming out of the tech companies, the major tech companies, and typically when Whenever they talk about AI or whenever you see PRPCs on AI, they are very limited. They very much focused on generative AI and even that, if you look carefully it's just generation of text or generation of images or generation of videos. There is a small sort of subdomain of AI. If you think about it, artificial intelligence is simply this idea of utilizing computation and utilizing uh computational techniques and algorithms in order to build agents that can perceive sensory data from the environment and given a particular objective, reach that objective, make rational decisions to reach that objective. So this is when I say uh this is a very dry objective, right? uh or a dry definition, but, but it's, it's important to keep this in mind because when you look at this definition then you see how when folks talk about agentic AI, uh, you struggle to say, well, but Isn't that AI that is AI in AI we have really always wanted to build artificial intelligence agents, right? We always wanted to, we, we, we sought autonomy, right, decision making, and there are many different subvaris within AI that is not just generation of content. But it is, you know, search and optimization, for instance, if you use ways of your, if you use Google Maps, that algorithm that is a part of AI, right, that is artificial intelligence, right, but it's not as appealing or as PR as as Dali. Or or LGBT logic being able to uh from from facts stored in a repository in a knowledge base to be able to deduce, to come up with proofs, to come up with that that you can guarantee, right, things that you can say definitely. And then there is, there are many things under AI, but, but that thing generated AI that most people think AI is, is a very small part of AI, but currently it's seeing a lot of activity. Yeah, it's getting all of the, the big headlines, yeah. Yes. What are sort of the promises of AI you've touched on some of them, but why is there such a push for AI right now? What are the promises that you can see and then what are the challenges to realizing those promises that you see? Lots of challenges, but I'll start with the promises. So let me back up a little bit why there are a lot of promises when, when folks talk about AI sometimes as a technology that is very limiting, uh, because in one sense, AI is a, I would consider it, it's very. Very similar to electricity. So it is something that you can integrate, whether in your processes or in your what you do in your research, you know, in the way that you, you, you try to obtain knowledge and educate yourself. So it becomes this, this fuel in a sense. And that's why it's very difficult. difficult to really sometimes even imagine the impact of AI because it is in itself a supportive thing, right? It's a, it's almost like a a meta technology and you can use it to um speed up the drug discovery process, for instance, right? And so that's one area where I see. A lot of potential AI for health. It's an area where you can very clearly also measure the, the impact, so you can keep yourself honest and say, well, are we really speeding up the process? Are we really generating novel drugs, right, which is a little bit more difficult when you're like, well, everybody's using GPT but you can't quite put metrics on, where is it helping and how much is helping, right? What what process and so AI for health is a big one. the intersection of I would say AI with biotechnology has also a lot of opportunities, but I also see a lot of opportunities even for instance in catalyzing scientific disciplines, advancing discovery within scientific disciplines. So my area of research, uh, when I started was at the intersection of AI and molecular biology, which we used to call bioinformatics, and we still do. And so that intersection is where it was in, in a sense where a lot of AI advances happened because biology was the domain where we originally had more data. We didn't have, you know, Twitter or now X and we didn't have Facebook, right? So that was the domain where suddenly you had data so you could, you could advance these uh AI algorithms. Now we even talk about new materials, for instance, AI. Uh, allowing us to map out the space of possible materials and design materials with specific properties that we care about and so that in itself can transform a lot of areas, right, a lot of um areas of our society, whether you think very narrowly in uh civil engineering, right, building better bridges or better building more resilient buildings, or you think quantum computing where you need uh. Special materials, right, or you think sustainability, right? Or you think climate resilience. So, um, there are a lot of areas now challenges, I, I see a lot of challenges right now. One that gives me a little bit of of pause. Well, one challenge is measuring the impact that I alluded to in the beginning because depending on the application area, it's it's hard sometimes, but I, I think this will be sort of a few. Stories from now we will be able to answer this question. Uh, we've had trouble finding answers to well how is generative actually impacting what you do on a day to day basis, right? So that's very difficult to measure right now, but we can do it. We can keep ourselves honest in this other application domains, and usually that's what I stick to whenever I put something on LinkedIn. I stick to AI for science or AI for biology where it's very easy to show what kind of advances. You're making one challenge, one concern that I have is opening up to everyone, so giving everybody access and it started first people were not really particularly paying attention, but since around 2019 I started getting the sense that a lot of the research that traditionally was happening within the doors of university on advancing of even foundational AI. Technologies and algorithms started then being advanced more rapidly in at Google, right, than alphabet meta, right, and then Nvidia and then Amazon, and that's a great thing that has its own benefits, but those are companies with very specific objectives, right, and you can boil down everything to money, right? And, and we need to make sure that AI research still is happening within. Education because only in a public university I keep saying this to folks it's only the public university where you can explore all angles because you don't just have engineers working towards a product right? you have the philosophers, you have the policy folks, you have the health folks, you have all the different colleges so it is the perfect environment to look at all the dimensions and and think more clearly about what is ethical, what is responsible, right? How should we integrate AI. In education if we decide that that is a good thing, right, can we do it safely? Can we do it in a in a way that helps our students rather than hampers their development? So these are they, they go more to, to access, making sure that everybody's part of the development and the advancement. um, there are many others, right, but they're a little bit more technical, but, uh, feel free to ask me any questions. There was a study that was released actually. I think this week or last week about people who engage with generative AI on a regular basis, have a reduction in their critical thinking skills. So how do we mitigate some of the potential harms, and that's just one example of a potential harm. How do we mitigate some of those things as we move to integrate? Artificial intelligence more broadly or more deeply into things that we're pursuing. Yeah, I, I read that too, um, the what we call cognitive offloading, right? And that that wasn't actually a surprise to me. That's something that we've been talking about in my community for quite a few years now, but in some sense. So I like making these comparisons because then it gets us away from what I call the freak out mode and it, it, it informs us to looks like new but it's not new. This is the calculator all over again, right, right. Well, I use ways. A lot. So, so you will occasionally get folks to say yeah but nobody remembers anymore how to get anywhere, right? So we started having these questions many years ago as we started to integrate these sort of autonomous, right, somewhat autonomous technologies that could make decisions for us, we moved certain things that we had to do in our daily tasks, right? And now you can argue whether that was a good thing or not. A bad thing, but I just like to always keep in mind that whenever you introduce a technology, it adds, but you have to ask the question what does it take away? You need to have a question, OK, it adds exciting, but does it take away anything? And very often we don't have a good understanding of what it takes away until many years have passed, right, and we see its impact because for whatever reasons, lack of imaginations or not having the right people in the room to think about. About this sometimes we just need time to see the impact of uh of that technology and I'm not saying this to sound blase but just to bring this recognition that there's no free lunch whenever you bring a technology you take something away. So I am not surprised because fundamentally, as I tell my kids, we are inherently lazy, we are drawn to comfort, to convenience, right? So when you have a technology. That promises to make things easier for you, it's very easy, right, to, to be lazier, but how do you do it right? This is something that I'm, I'm very happy to say this is exactly where you need research at a public university, right? Where you can study and think through, uh, first, what does it take away and more importantly, how you should use it or what you should use it. So this is another challenge because we always want to integrate. It is a shiny new thing we want integrate. There are benefits. We clearly see benefits. I mean, I use these tools. They help me sometimes to do context switching very fast, but very often I will sort of make a conscious decision and say, no, no, no, here I'm going to stop because I want my voice to come through. And it's not going to be perfect English, but it's going to be my voice. And I will know this is the way I say things and why I say things. So Lots of challenges. I am still optimistic. These are, I would say growing pains in some sense we have to figure out whenever you introduce a new technology, these are the right questions to ask, right? I bet you folks that study the history of technology, they can point to specific uh moments in time when we exactly, you know, we, we would find that OK, wait, it does this bad thing and wait, it does a bad thing and it's important so that you know, we integrate that in the right place. Yeah, I think it's David Carr has a book, The Big Switch, which talks about the implementation of electricity and you referenced how like this is a thing and so similar where there are tons of examples given in that book where people were like, people keep burning down houses with electricity. We've got to ban it. And that clearly didn't happen. So. Understanding how to harness and make the best of something I think is really important. One sort of last question because you talked, well, two really, I would love to hear your thoughts on the application of AI broadly, not necessarily just, you know, a chatbot and a generative AI, but broadly, How do we apply artificial intelligence to make administrative processes better? What are the opportunities there? And then where are we with the development of this technology? Are we at the VHS stage, or have we already gotten to like, like the DVD stage or the Blu-ray? Um, so the answer to that first question is, you tell me, and I'll, I'll explain what I mean here. If you integrate something, you control for it, right? So you make sure, for instance, that there is no leakage of your data, right, that you're not compromising, you know, the security of the environment in which you you operate. So it's completely sandboxed, but it gives you, it gives you a lot of uh freedom, a lot of opportunities. Me sitting on outside and just having this technology, I don't know, I'm not the domain expert in what you do in the day to day, but I'm a firm believer and, and, and studies are starting to show it is that when you give folks a platform and you control, you know, you make sure, as I said, that there's data security, all those privacy issues are are resolved, but when you do that. Then the users themselves come up with new use cases that you would have never thought of. That's when creativity happens and that is the stage where we are in, and that's also why it's very difficult to measure productivity because the platform providers that are developing this, they can think, they are thinking, right? They want to think use cases because it's part of the business model, right? They want to generate revenue, but we're finding. That for instance, the advancement office suddenly realizes that with this thing, they can be more precise or they can do something, right? And so that's what actually excites me. If you give me an interesting and I reduce it to say if you give me, I would say if you give me a medium, not a technology, if you give me a medium, then watch me what I do, but make sure that everything is there are no um. You know, there are no sensitive, uh, there are no risks there. Now, on the second question, Uh, I was struggling VHS, DVD, Blu-ray, um, I would say we're more in the DVD mode right now, right? VHS was sort of very narrow, uh, but with these platforms we're seeing new use cases every day, right? Uh, folks are coming up with them. It feels like every other day, maybe not Blu-ray yet because this is a technology that's still growing. We're still trying to understand, right, what it. Can and cannot do or what we should use it for and what we should not use it for. So if I had to sort of do a multiple choice, maybe I'll get more credit if I pick DVD at this point. Definitely not, not VHS, but we're not at the Blu-ray yet. There's still room to grow, to grow, and at some point we'll just get to streaming and then everyone will have AI everywhere all the time. OK, transitioning from my skepticism, let's talk about some legal things. And now making her triumphant return to the podcast since way back in season 1 episode 7, Claire McKenna.-- It's-- me. I've always been here the whole time but not really. Claire has worked and practiced law for more than 20 years now, which is crazy to think about in a variety of settings at the federal level, state level, in a weird public-private partnership, and then now in like a public entity's just go with that. Yeah, Claire is an outstanding attorney, but she is not your attorney, dear gentle listener. Nothing, Claire is about to say should be considered legal advice. Always consult your institution's Office of General Counsel with any legal questions that you may have. So, hi, Claire. Hello. Thanks for agreeing to be on the podcast and for chatting with me again. Of course, I'm happy to do it. So today's topic, as you know, is artificial intelligence. And from a legal perspective, what kinds of issues do you see with AI and in particular, Generative AI. I think that in many respects, the issues that are presented by AI are the same kinds of issues that we're confronted with any time we're dealing with types of sensitive data. So in your case, it's I guess most obviously, you're dealing with FERPA data and the restrictions that come along with that. But, but in truth, you know, anybody in their workplace deals with a variety of different kinds of sensitive data, whether it's It's just purely internal information, or perhaps you have law enforcement information, depending on your context, there's attorney-client privilege information, I mean, it goes on and on. And so each of those kinds of data sets has their own requirements and restrictions that have to be accounted for in any context, and in particular, when you're thinking about AI because AI presents some new challenges for the use of that data. Yeah. What what are the challenges that you see? And that you are sort of grappling with in your professional sphere. Yeah, I think that, I think what makes AI unique in a way is that it requires so much data to do its job, and that sort of Well, let's say there's a tension there, right, when you're dealing with types of information that have controls, and then you're, you want to put it in a system that depends on sucking up, sort of depends on those controls not existing, right, because it requires so much information, so much disparate information to do its job. And so, I think a way to deal with that is to just kind of think back, right, to what we talked about in season one, which is, let's go. That's why we're here. So yeah, it's really those fair information practice principles and, you know, I think what we talked about then is that those Even though we talked about those principles as significant because they lay the foundation for all privacy laws in regardless of the sector, so they're the foundation of FERPA, of Graham Leach Bliley, of HIPAA, all of those laws are at their at their foundation based on the FIS. But as we also talked about in that session, the PIs are the framework for assessing data use in any context, not just within those specific categories of control data. And so you got to take it back to the FIS when you're thinking about AI and thinking about how to make it. Work with all of your data. So I thought we should probably just remind everybody what the ips are, right? Yeah. So, um, as we already said, there are the fair information practice principles, and there are, let's see, I don't know, a bunch of them. I'll just rattle through them, um, but you could go back and listen to our previous episode too. OK, so the PIPS principles are collection limitation, right? So, right away you can see potentially attention here with how AI works, because the idea of collection limitation is that you collect the minimum amount of information that you need to do the thing, right? Um, now, interestingly, not necessarily attention because if AI needs a lot of data, then, then you've met that collection limitation. An obligation, that's not, it's an inflection point. What do I need to do this job and only collect that? Doesn't mean if you need a lot, you need a lot, but something to think about. Data quality, the data has to be accurate, complete and timely. That's really also, yeah, exactly, you're making a face. That is also something that is at just a critical point when you're talking about AI and that is job it is to suck up a lot of data and that AI. In and of itself doesn't care, isn't doing that quality assessment. It needs you to do that quality assessment. Yeah, it's a big garbage in garbage out kind of a situation. And so we'll talk about chatbots in a minute, but like one of my concerns about chatbots is that it relies heavily on information on your website, for example. or information that you have in a knowledge base. And that is not always up to date and it is not always clean, and it's not always accurate. I hate to admit that on a pod, no one's listening, it's fine. But it's not your, I mean, it's not an indictment. It's just a truism. That Next up. OK, purpose specification. So this is, you know, tell people what how you're going to use their data. We're going to use it for X. And, you know, that, I kind of see this almost as a greater tension than the collection limitation one, because there is such a desire to be like, let's just collect everything and then figure it out later. And no doubt that everything can be useful. But you really have to start with what are we trying to achieve? And it shouldn't be, or at least from a data governance standpoint, it's not a good answer to say, we just, we don't know, but we just want, we'll know it when we see it. Let's just collect everything. Um, and there's no way to control how an AI uses the data that's been collected. So you hope that it gets quote unquote trained. On the right data and then it comes back with the right answers, but there's really no, there are a few guardrails right now. Right. Well, and there may be technological guardrails that are available that would, I wouldn't be the person to opine about that. But, but that really goes to kind of where we started in this little bit of conversation, which is, you have to know what you're trying to do in order to be able to define. What the guardrails are. So, you know, again, so just to say, let's just suck up everything because surely we'll find it to be useful. I mean, maybe that is useful, but it certainly isn't what's contemplated in the fair information practice principles in this concept of use limitation. So, of course, one of the tips is security safeguards. Obviously, I hope that's obvious how important it is to make sure. That data is secure and that it's not susceptible to unauthorized access and use. But yeah, I mean, again, you know, let's just put a question mark there. And let's talk through like one of the things that I've seen a lot recently in all the Zoom meetings or Microsoft Teams meetings is like the automated, oh, we've got chorus AI is recording and we'll provide your meeting summary. I don't know if we want to talk about that now. Yeah. about it now, I mean, I think that's such a great example. And I think it's such a great example, even, you know, to connect with this aspect of the FIS, which maybe I wasn't thinking of it in that way. But, but certainly, I've had this happen, we've all had this happen. I think where we're in a meeting on a video conferencing platform, and you see that automated thing on there. If that, if that happens to you, I I hope that you're asking, what is that? What is doing, because sometimes that is something that is like an open source that is in your meeting recording what you're saying for transcription purposes, or other purposes that maybe you know or don't know. And that might be really helpful, so that no one maybe has to have handwritten notes, I guess. But you have to remember that that is the same thing as grabbing some random. Person off the street, inviting them into your secret, you know, FERPA meeting or, you know, your attorney-client meet or whatever the case may be, and saying, hey, random person, will you please take notes? And you can take those notes with you and do whatever you want with them because the AI wants that data, it makes it smarter. And if you don't have a relationship, either an relationship, a contractual relationship with that AI, you're note taker, then that information is publicly available to anyone. So you really need to scrutinize those kinds of things in order to ensure that you're meeting your obligation to protect your information from unauthorized access and use. Yeah, that was one of the things that just talked about, even though he didn't listen to that part of the conversation was Being able to use AI securely. Yeah. And I think that that is really that idea that you can just tap into an AI and have it take notes from your meeting and give you a summary meeting summary. That's great. If you know who's providing that, if you have contract details that specify how that data can be used, where it's stored, and all those types of things. So Right now off the shelf generic AI note taker that are widely available, I would be willing to bet that most people don't have that level of contractual protection. And so it is just in anywhere. I'm gesticulating maybe at some point this podcast will need to we need to do a video version of the podcast. My grandma always used to say I have a face made for radio. Anyway, are there more? Oh, oh yes, there are, although we're coming to the end of them. OK, so we talked about security and um, so the next one is openness, and this means that you, again, you have to be transparent about your data practices. So a lot of entities, institutions have privacy policies. You have to look at those and think about whether Your current privacy policy contemplates your proposed use in AI, and that includes, I mean, again, this example is is an interesting one about these AI note takers because it is the approximation of just inviting some random person into your, you know,-- internal meeting and then-- potentially making all of that data publicly accessible. Yeah, giving it to them, hey, you want these student lists or whatever the case, whatever the case is. Yeah, exactly. So, so you have to have your privacy policies, your data protection policies, you have to make them available to to the people whose information you're, yeah, whose information you're using and and you have to, you have to comply with them. And so that is part of the analysis that you need to be doing when you're thinking about how to use these tools. Individual participation, this requires That folks um are able to have access to the information that you're keeping about them, be able to correct it, and in some cases be able to request distraction or erasure of that data, yeah, and FERPA works that way as well in many respects. And so, again, depending on the use, your use of AI may not fit within the FERP. A framework, so you might not have sort of that, I guess, established structure, but, but from a good data management, data um governance standpoint, can you think about, can we replicate this in this AI context? Can we give people access to their data so that they understand how the AI did whatever you're asking it to do using data about you, so. And then the last one is accountability, and so, and that's really that there has to be responsibility, maybe it's legal responsibility, um, but there should be responsibility for compliance with your privacy policies, compliance with your data governance policies, compliance with your commitment to allow people to have access to or correct their data, that kind of thing, you know, it has to be meaningful, I guess, at the end of the day is what that one's about. Yeah. So I've said a couple of times that I'm skeptical about the benefits of applying AI to registrars office administrative tasks in particular. And I want to pick up on a thread about using AI in decisional capacities. And so what does the law say about that? Are there laws about that? Is there guidance about that? What do you have to say about that? Yeah, I mean, there's getting to be Laws about that. I, um, there's just a law that was passed by the Virginia State Legislature that addresses that. I, I think Colorado, so, you know, these are starting to pop up in different states. I believe the EU has a framework about this. And really, those, the laws that are emerging, don't care too much about the use of AI for these administrative. tasks, but they do care deeply about what, um, about the use of AI to make decisions about people. So, whether it's, um, you know, eligibility decisions or admissions decisions, healthcare kinds of decisions, I mean, you, you got it. Um, and so, and those types of systems are considered high-risk systems and so that's really where the regulations are coming in. Not surprisingly, what they're kind of doing at this point is requiring some version of compliance with the FES for these kinds of systems. They also present, which I think there's a lot of buzz about these kinds of systems present this additional concern, which is concern about fairness and um discrimination. And you said, you know, garbage in garbage out, that's kind of an element of it, but there, but It's also maybe it's not garbage,-- maybe-- like racism in racism out. Well, but not even maybe not even necessarily intentional racism in racism out. I mean, that's sort of part of the concern. I mean, there's, I read a study that's old now, but that's so interesting about facial recognition technology, right? And so too many or most of the test data was white male faces. And so Literally, these systems were not recognizing female faces or people of color faces. Hey, that's like every other system that's been built in the history of systems. Well, you know, and so I don't know. I, I, let's not, we don't need to ascribe motives, right, to to that. So like your racism and racism, and maybe that probably wasn't, well, I don't, I'm not going to ascribe motives. But if you want a system that is Good at recognizing faces, right? It needs to be able to recognize all different kinds of faces. So that's kind of one that is an example of how it's just the way that a system may how how dependent the systems are on the data in to be able to produce a good, fair, non-discriminatory data out. Yeah. And if this, I'll throw another plug for this book, weapons. of math destruction MAT math destruction about the way that algorithms sort of rule your life and how policing applications in particular, where when you focus on a particular area and you wind up entering more parking tickets or citations or whatever it is, then the algorithm says that area needs more police. And so then you get more. Police there who then find more things that to take or to do whatever and that leads to like this vicious cycle of. Yeah, yeah, that I like that cliche reminds me of that cliche like if you're a hammer, everything's a nail like and that's just sort of just how maybe the developers' point of view because they're a hammer, right? I inadvertently skews the outcome, but you know, as if you're using AI systems for decision making, and those concerns are really come to a critical point, especially if you aren't including humans, you know, what's the check on that, right? Right. All right. What else you got? Well, I guess, you know, the other thing that just I've been reflecting on, which is this is sort of my personal opinion, but we've talked about this is that I think that there, you know, as as entities look forward, I hear people say, All the time, and maybe you do too, like, oh, we need to, we need to think about AI we need to get AI. And I think, you know, I am a, I definitely think that technology is great. And I definitely think that technology can and should be explored and embraced in appropriate circumstances, like I'm not anti-technology, I, you know, I'm not using an abacus still or anything like that. But to say how can we use AI is not, that is not your first question. Your first question is, how can we make our website work better or or, you know, whatever it is, I mean, it is critical that that you're identifying the business need and then maybe you're thinking. about AI is a potential answer to whatever your business need is. But I do feel like there is just kind of this rush to be like, let's do some AI. I feel the same way about blockchain, where like, for a period of time, everyone was like, you got to get on the blockchain. Get on the blockchain. I was like, blockchain is a solution in search of a problem, right? Well, I think, I think that the first what you just said is really true and people are just like grasping at AI as though it is going to solve the sort of structural problems in These administrative problems that are not AI. They're, you know, these, these kinds of solutions take relationship building and personal management, and then also a review of the structure in order to get it to a point where you could train an AI up. To do the particular thing. The other thing is that, and I said this to you yesterday, registrars don't have big data. We are not a big data entity. We have a lot of data, but don't confuse the two. Having a lot of structured data doesn't mean that like we need all these super fancy things. When we think about like, can an AI process these forms faster, it's not. There is a difference between artificial intelligence and intelligent automation. And so in 99% of the use cases that I've been presented as like, oh, you should use AI. Really, I actually need intelligent automation more than I need artificial intelligence, because we have 9 schools. And 2 levels at each school, 3 if you count non-degree. And so this the spectrum of decisions that I need a system to make is not that big. And so I can code, I can't code. Let's not. Someone that I work with can code the decision tree for what should happen. With each of the potentialities at each step of that process, a lot more quickly, a lot more efficiently and a lot more aware of cultural and political sensitivities at my institution, then I could train an AI to do it. It doesn't make sense at this point, because AI is really wasteful. It takes a lot of energy to it takes 10 times. more energy to run an AI search than it does to just run a Google search, even though now every search engine is like trying to use generative AI as part, so just like down a rabbit hole of energy consumption. I'm ranting. I know.-- I know that and you-- never. Well, it also sounds like, I mean, I feel like I, I feel like I need to say you sound like you're anti-technology for the registrar's office and you're, well, no, you're absolutely not. And so I mean, I think my message would be, think about AI think about if, if that is the right solution to the problem that you've you're trying to solve, or, or for whatever your use cases, maybe you don't have a problem, but But walk slowly down that path because there are many, many considerations that need to be accounted for. And, you know, we didn't, I'll just be explicit, I mean, you need to be very careful about open source AI um because you're dealing with sensitive data and that You know, when you input it into an open source AI, you're giving it away. Anybody else who does that same as that same question of that open source AI is gonna get information that now includes what you provided, and because you're dealing with sensitive data, you have to be very thoughtful, so you need to You know, treat AI like you treat any other technology system that you're thinking about deploying in your institution, which means, what are your procurement rules, what are your data security rules, you know, what, how are you sharing the information? How are they protecting the information, what use limitations are they applying it? And then let's just, we'll just add on top of it this additional element where if you are Thinking about using it, you know, for a high risk purpose, a purpose that, you know, is about making decisions that affect individuals, you need to be extra thoughtful and perhaps legally required to ensure that there are guardrails in place, human interventions in place to protect against unfairness, unfair, and or discriminatory outcomes. Word. We're pro technology here. Right, yeah, right, exactly, but it is, but there is, there are many, even though it is so easy, there's so as you said, there's so many open source AI, yeah, of just available, just begging you to put your data in it because they want your data, you know, there are many, this is just like anything else that you do where you have to. Through your process for bringing that technology on board and think about the FIS as part of your deployment and your decision making.-- So-- thank You're welcome for being here.-- Thanks-- for having me here. This is just like the last one where it's just like this is this is what we talk about for fun, so we can just enjoy and bring everyone else in. Yeah. Oh, and the dogs now too. Huge thanks to Amada and Claire for chatting with me about artificial intelligence. Circling back to my skepticism, I definitely see the potential for AI to assist in scientific research, and there may be a time when I'm super on the bus about using it in registrars' offices, beyond the limited scope use cases of a chatbot or something similar. As Claire suggested, as with any new technology, take your time, figure out what problem you're trying to solve and whether the hot new thing is really the best way to address that problem. And then obviously, always understand what free or open source systems are doing with the data you're giving them and always check with your procurement or IT or general counsel's office if you have any questions about any of the applications that are out there, ideally before you start using them. Thanks very much for listening. Reach out to a friend, let them know that you've been thinking about them. Go for a walk, then come back and call your representatives. Oh, and drink some more water. Until next time, I'm Doug McKenna, and this is For the Record. Think about the Phipps. And as part of your deployment and your decision making, so. That is a great button. Think about the Phipps. Yeah. It was a. Yeah.